Answered step by step
Verified Expert Solution
Question
1 Approved Answer
Utilising extensive exploratory data analysis ( EDA ) in an analysis is almost always useful. By managing, visualising, and analysing data, EDA can be used
Utilising extensive exploratory data analysis EDA in an analysis is almost always useful. By managing, visualising, and analysing data, EDA can be used to uncover meaningful insights, and allow you to communicate effective findings. Such examples include: Manufacturing process improvementAnalysing production data to identify bottlenecks, defects, and efficiency improvements in manufacturing processes. Retail sales analysisExploring customer purchase patterns and trends to optimise inventory management and marketing strategies. Customer behaviour analysis Investigating customer interaction data to optimise website design, content, and marketing strategies for ecommerce businesses. Financial market insightsInvestigating historical stock market data to identify trading opportunities and risk mitigation strategies. Educational performance evaluationAssessing student performance data to identify areas for improvement in educational curriculum and teaching methodologies. Healthcare data examinationAnalysing patient records to identify correlations between various health factors and outcomes, ultimately enhancing patient care. Social media sentiment analysisUtilising EDA to gauge public sentiment about a product, brand, or political topic by analysing social media data. Environmental data explorationExamining climate data to understand longterm trends, contributing to climate change research and policy development. These examples showcase the versatility of exploratory data analysis in various domains, demonstrating its potential to uncover valuable insights and drive informed decisionmaking.This case study assessment provides you with an opportunity to apply all the techniques you have encountered over the semester and apply them to a realworld dataset. In this assessment, you will need to write a full report which covers the full data science methodology, applied to an analysis of your chosen dataset. This means you will first prepare your dataset, clean it up process it analyse it then train a predictive model on the training dataset and predict values for the test dataset. Finally, you will evaluate your model using the metric RMSE against the test dataset and plot the residuals similar to the lecture content given in module and draw your final conclusions.You can use any models you like, at a minimum using one multiple linear regression model.This assessment will provide evidence towards the following unit learning outcomes:ULO: Detect missing values, outliers and other abnormal data prior to exploratory data analysis.ULO: Identify the hidden underlying structures and patterns of the variables within the data.ULO: Examine ideas and methods used in exploratory data analysis for real world applications.ULO: Demonstrate strong skills in using data visualisation techniques for analysis and communication of findings and results, along with statistical reporting.Assessment instructionsThis case study assessment provides you with an opportunity to apply all the techniques you have encountered across the unit and apply them to a realworld dataset.Note: You have the option to choose your own dataset, but you can also choose to use the default dataset on Ames Housing dataset an American housing dataset:House Prices Advanced Regression Techniques KaggleA brief description of the variables in the dataset can be found in the following document:Variables in the Ames Housing datasetFor example, on the Ames housing dataset if chosen by you when writing the report put yourself into the shoes of a real estate analyst wanting to obtain insights from this dataset to predict house prices. The dataset already has a lot of reports written on it Be inspired by them for EDA but do not focus too much on their modelling. Review and access the following reports on the Ames housing dataset:House Prices Advanced Regression Techniques ReportsKaggleDownload the datasets labelled train.csv and test.csv from the default dataset link. As you are a real estate analyst, your target variable is SalePrice. Note for most of the report, you will only use the train dataset. This includes preprocessing, EDA, and everything else up to and including the creation of a linear model.The linear model will then be trained on the train.csv dataset. You will then predict a set of SalePrice values based on the variable information in the test dataset. You can then compare your predicted values to the real values in the test dataset. Therefore the test.csv dataset is only needed for the Evaluation section of the report.Regardless of which dataset you choose, ensure you understand which variables are explanatory and which are target variables. Also, communicate this in your report.Report structureYour report needs to include the following sections. In each section you will need to give a very brief explanation as to what the section is about, what the purpose of the section is andor describe the key pieces of information in your general approach. For example, in the Data preprocessing section, you would explain what exactly data preprocessing is why you need to clean the data, and describe the key ideas in your approach eg fill in missing values with median based of external controls.
Utilising extensive exploratory data analysis EDA in an analysis is almost always useful. By managing, visualising, and analysing data, EDA can be used to uncover meaningful insights, and allow you to communicate effective findings. Such examples include: Manufacturing process improvementAnalysing production data to identify bottlenecks, defects, and efficiency improvements in manufacturing processes. Retail sales analysisExploring customer purchase patterns and trends to optimise inventory management and marketing strategies. Customer behaviour analysis Investigating customer interaction data to optimise website design, content, and marketing strategies for ecommerce businesses. Financial market insightsInvestigating historical stock market data to identify trading opportunities and risk mitigation strategies. Educational performance evaluationAssessing student performance data to identify areas for improvement in educational curriculum and teaching methodologies. Healthcare data examinationAnalysing patient records to identify correlations between various health factors and outcomes, ultimately enhancing patient care. Social media sentiment analysisUtilising EDA to gauge public sentiment about a product, brand, or political topic by analysing social media data. Environmental data explorationExamining climate data to understand longterm trends, contributing to climate change research and policy development. These examples showcase the versatility of exploratory data analysis in various domains, demonstrating its potential to uncover valuable insights and drive informed decisionmaking.This case study assessment provides you with an opportunity to apply all the techniques you have encountered over the semester and apply them to a realworld dataset. In this assessment, you will need to write a full report which covers the full data science methodology, applied to an analysis of your chosen dataset. This means you will first prepare your dataset, clean it up process it analyse it then train a predictive model on the training dataset and predict values for the test dataset. Finally, you will evaluate your model using the metric RMSE against the test dataset and plot the residuals similar to the lecture content given in module and draw your final conclusions.You can use any models you like, at a minimum using one multiple linear regression model.This assessment will provide evidence towards the following unit learning outcomes:ULO: Detect missing values, outliers and other abnormal data prior to exploratory data analysis.ULO: Identify the hidden underlying structures and patterns of the variables within the data.ULO: Examine ideas and methods used in exploratory data analysis for real world applications.ULO: Demonstrate strong skills in using data visualisation techniques for analysis and communication of findings and results, along with statistical reporting.Assessment instructionsThis case study assessment provides you with an opportunity to apply all the techniques you have encountered across the unit and apply them to a realworld dataset.Note: You have the option to choose your own dataset, but you can also choose to use the default dataset on Ames Housing dataset an American housing dataset:House Prices Advanced Regression Techniques KaggleA brief description of the variables in the dataset can be found in the following document:Variables in the Ames Housing datasetFor example, on the Ames housing dataset if chosen by you when writing the report put yourself into the shoes of a real estate analyst wanting to obtain insights from this dataset to predict house prices. The dataset already has a lot of reports written on it Be inspired by them for EDA but do not focus too much on their modelling. Review and access the following reports on the Ames housing dataset:House Prices Advanced Regression Techniques ReportsKaggleDownload the datasets labelled train.csv and test.csv from the default dataset link. As you are a real estate analyst, your target variable is SalePrice. Note for most of the report, you will only use the train dataset. This includes preprocessing, EDA, and everything else up to and including the creation of a linear model.The linear model will then be trained on the train.csv dataset. You will then predict a set of SalePrice values based on the variable information in the test dataset. You can then compare your predicted values to the real values in the test dataset. Therefore the test.csv dataset is only needed for the Evaluation section of the report.Regardless of which dataset you choose, ensure you understand which variables are explanatory and which are target variables. Also, communicate this in your report.Report structureYour report needs to include the following sections. In each section you will need to give a very brief explanation as to what the section is about, what the purpose of the section is andor describe the key pieces of information in your general approach. For example, in the Data preprocessing section, you would explain what exactly data preprocessing is why you need to clean the data, and describe the key ideas in your approach eg fill in missing values with median based of external controls.
Step by Step Solution
There are 3 Steps involved in it
Step: 1
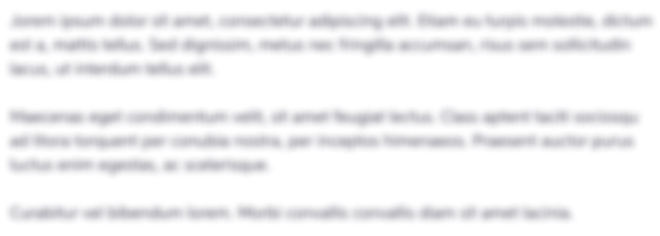
Get Instant Access with AI-Powered Solutions
See step-by-step solutions with expert insights and AI powered tools for academic success
Step: 2

Step: 3

Ace Your Homework with AI
Get the answers you need in no time with our AI-driven, step-by-step assistance
Get Started