Which business analytics model(s) is used? Briefly justify why this paper's analysis should be considered as the business analytics model(s) you have selected.
Provide as much information as you can on the data frame such as the number of observations, the number of variables, the names and explanation of the variables and their categories
Provide as much information as you can regarding the data cleaning, handling of missing data, and outliers.
Have they used normalization, standardization, or any other transformation?
If the paper does not have a section on this, mention it with a sentence.
Summarize the descriptive statistics used in the paper. As much as you can provide the insights or the details that have been instrumental in determining what to do in the latter parts of the paper.
How many tables and charts do the paper include?
Considering "which chart to use when?" if possible give one example of a chart for showing the relationship, comparison, distribution, and composition. Here, demonstrate your understanding that the correct chart type was selected or why another chart type would be more appropriate.
Overall, how would you evaluate the charts in terms of graphical excellence. Briefly present your reasoning.
In your own words prepare a summary of the analysis they have done (including the technique they have used) and the insights (results) they have derived.





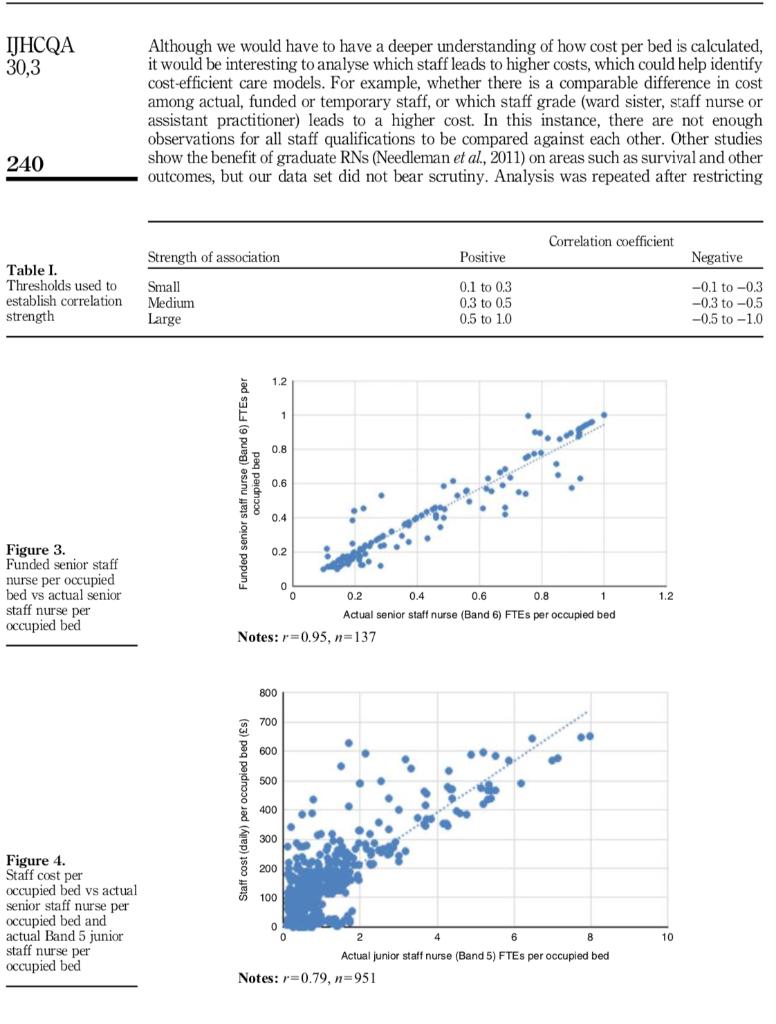






The current issue and full text archive of this journal is available on Emerald Insight at: www.emeraldinsight.com 0952-6862.htm Nurse staffing levels and outcomes mining the UK national data sets for insight Nurse staffing levels and outcomes 235 Received 25 August 2016 Accepted 12 September 2016 Alison Leary School of Health and Social Care, London South Bank University, London, UK Barbara Tomai, Adrian Swift and Andrew Woodward Centre for Workforce Intelligence, Mouchel Management Consulting Limited, London, UK, and Keith Hurst Hurst Research Ltd, Mansfield, UK Abstract Purpose - Despite the generation of mass data by the nursing workforce, determining the impact of the contribution to patient safety remains challenging. Several cross-sectional studies have indicated a relationship between staffing and safety. The purpose of this paper is to uncover possible associations and explore if a deeper understanding of relationships between staffing and other factors such as safety could be revealed within routinely collected national data sets. Design/methodology/approach - Two longitudinal routinely collected data sets consisting of 30 years of UK nurse staffing data and seven years of National Health Service (NHS) benchmark data such as survey results, safety and other indicators were used. A correlation matrix was built and a linear correlation operation was applied (Pearson product-moment correlation coefficient). Findings - A number of associations were revealed within both the UK staffing data set and the NHS benchmarking data set. However, the challenges of using these data sets soon became apparent Practical implications - Staff time and effort are required to collect these data. The limitations of these data sets include inconsistent data collection and quality. The mode of data collection and the itemset collected should be reviewed to generate a data set with robust clinical application. Originality/value - This paper revealed that relationships are likely to be complex and non-linear; however, the main contribution of the paper is the identification of the limitations of routinely collected data. Much time and effort is expended in collecting this data; however, its validity, usefulness and method of routine national data collection appear to require re-examination Keywords Patient safety, Healthcare data, Nursing outcomes, Staffing, Nurse, Acute, Modelling, Safety Paper type Research paper Introduction Nursing care providers, such as registered nurses (RNS) and unregistered healthcare support workers (HCSW), provide most patient contact time in the UK. This workforce represents the largest staff group and subsequently the largest expenditure (NHS Confederation, 2015). Determining the workforce's contribution to patient safety can be challenging, particularly if safety is more than the absence of harm (Reason, 2000). However, we have an opportunity to explore this relationship by mining two large, UK wide data sets, which include data from a National Health Service (NHS) benchmarking and staffing perspective over several years. Our research focusses on understanding how RN and HCSW staffing levels influence patient outcomes and on identifying patterns that could help improve patient care. Nursing work is complex (Hall , 1964; Ebright et al., 2003; Leary et al, 2008; Warren et al, 2012); however, when portrayed as supply and demand, inpatient nursing work is often represented as This work was funded by NHS England. The authors thank Ruth May, Pauline Milne, Vicky Reed, Ruth Auton, Lynne Wigens and Jane Cummings for commissioning the work; however, the conclusions and recommendations expressed in this paper are the authors' alone. International Journal of Health Care Quality Assurance Vol 30 No. 3, 2017 pp. 235-247 Emerald Publishing Limited DOI 10.1108 THCQA-06-20160118 linear tasks that are deterministic. If nursing work is linear, then staffing should be a simple calculation but it is not. Nursing work contains other components that are essential for safety, such as care management, avoiding failure to rescue and vigilance. Failure to match staffing to patient needs, therefore, is associated with increasing mortality (Needleman et al., 2011). Consequently, our aim is to better understand the relationships among healthcare variables by mining national data sets. Studies have looked at the relationship between RN numbers and outcomes (Aiken et al., 2002; Clarke and Aiken, 2003). There is also more data about patient care, experiences and outcomes. There is assumed to be a relationship between safety (using proxy measures such as falls, pressure ulcers or other untoward clinical incidents) and RN presence, but this relationship is not fully understood. Whilst there is no simple relationship between either number, nursing skill mix, outcomes and cost (Griffiths, 2009), there have been numerous statistical studies that demonstrate correlations between RN numbers and adverse outcomes (Clarke and Aiken, 2003; Kane et al., 2007) including failure to rescue deteriorating patients, which suggests a relationship with safety. There are two large data sets in the UK that contain several variables and indicators from NHS trusts. One data set contains routinely collected benchmarking data for most English organisations, with predetermined key performance indicators relating to the facility, patient safety and staffing. We call this the NHS Benchmarking Database. The other data set contains detailed nursing data from several NHS organisations across many years, which we call the UK Nursing Database. Our objective was to analyse these data sets to draw insight and explore potential relationships among them. Both data sets have been curated over several years by one individual, which results in consistency. Our second objective was to find possible intra-data set correlations among variables in the two databases and explore whether additional insight could be drawn from merging them. We highlight results from our correlation analysis and lay out the caveats and challenges to gain insight from these two routinely collected data sets. Methodology Both databases were anonymised and supplied as two comma-separated value files. To carry out the analysis, a correlation matrix was built from all relevant variables from each database. After examining these files, a phased analytical approach was spread across three different stages. The first two stages analysed possible intra-data set correlations and the third was an inter-data set analysis. However, this proved problematic owing to data set limitations, which we examine in detail. Consequently, we discuss the challenges and caveats when linking two independent databases. Data sets The UK Nursing Database The UK Nursing Database, designed, built and maintained by Keith Hurst, is unique and includes 2,800 acute, mental health and learning disability service wards, departments and community teams. Seven data sets are collected systematically in each ward/ department/team: (1) bed occupancy/daily contact rates, throughput, patient dependency/acuity; (2) staff activity (i.e. direct care, indirect care, associated work and personal time) grade, and ready-for-action (unproductive) time; (3) service quality (care pathway scores); (4) budgeted, actual, temporary and recommended staffing by grade; Nurse staffing levels and outcomes 237 (5) ward design or local population demographics; 6 time-out (annual, study, sick, maternity and compassionate leave); and (7) staffing costs. In the acute wards, for example, 1.8 million staff activities have been logged to date, which are used to: generate the Safer Nursing Care Tool (formerly AUKUH) staffing multipliers (recently endorsed by National Institute for Health and Care Excellence, 2014); and benchmark each ward/department/service's performance against its counterparts. The database's power lies in the way that patient dependency/acuity, staff activity, ward design/locality demographics, workload and staffing can be related to nursing quality: e.g., high workload, understaffed and grade-mixed dilute wards (i.e. proportionally more HCSW) can be connected to low-quality scores. Data collection started in 1985 and around three wards/departments/teams are added to the database each week, which relies on trust managers to volunteer data (75 trusts have contributed data to date). Participating trusts represent all 12 NHS hospital categories: e.g., small peripheral hospitals to specialist services. Because each trust is named in the UK Nursing Database, its data can be connected to corresponding data in the NHS Benchmarking Database. Figure 1 shows a short excerpt for a given NHS code. The NHS Benchmarking Database The NHS Benchmarking Database, funded by NHS England and hosted by West London University, is used mainly to profile NHS trusts. It includes every NHS England acute, mental health/learning disability and community trust. The database, designed, developed and managed by Keith Hurst, contains 1,650 data sets subsumed under 86 workforce planning and development-related categories, such as staff to bed ratios. Data sources include the Health and Social Care Information Centre (now called NHS Digital), Care Quality Commission, Monitor, Department of Health, NHS England, Public Health England and the Office for National Statistics. Indicators include mortality rates, patient experience and staffing data (by professional group). The database is designed to filter high- and low-quality trusts (measured using several unconnected data sets, such as patient satisfaction) and to examine local questions and issues (eg. why are some staff more productive? Why is staff turnover so high?). The database was developed to support trust managers exploring, for example, their staffing levels and to benchmark trusts against comparable providers. Data stop at trust level (unlike data in the UK Nursing Database, which are gathered at ward/department/team level). The NHS Benchmarking Database contains data from about 260 English organisations spanning the years 2005-2014 (at the time of analysis). We focus on 53 performance, staffing and patient satisfaction variables. Throughout the Cler Supl 36 47 1 3 1 2 2 Year 2008 2008 2008 2008 2008 2008 2008 2008 2008 2008 2008 3 Spec Cardiology Cardiology Cardiology Cardiology Cardiology Cardiology Cardiology Cardiology Crit CareCCU CritCareCCU CritCareCCU Crit CareCCU CritCareCCU CritCareCCU EldAcute EldAcute EldAcute EldAcute EldAcute 5 Mnth 2 2 2 2 2 2 2 2 2. 2 4 4 4 4 4 4 4 1 1 1 1 1 1 1 Grade 7 ws nrs spc 7ws 6 sn 5 sn 3 hca 2 hs http 2 CI Oirr 6 wsnrs spc 6 WS 5 sn bnk 5 sn 4 hca 3 hca 7 WS 6WS 5 sn 3 hca 2 hca Patients 31.4 31.4 31.4 31.4 31.4 31.4 31.4 31.4 8.04 8.04 8.04 8.04 8.04 8.04 33 33 33 33 33 Ward 100 100 100 100 100 100 100 100 94 94 94 94 94 94 92 92 92 92 92 Over 94 94 94 94 94 94 94 94 95 95 95 95 95 95 B6 86 86 86 86 ActS SW Bed Cost 84.13105 84.13105 84.13105 84.13105 84.13105 84.13105 84.13105 84.13105 0.11904B 245.2177 0.11904B 245.2177 0.119048 245.2177 0.119048 245.2177 0.119048 245.2177 0.119048 245.2177 81.977 81.977 81.977 81.977 81.977 18 2008 41 5 10 2008 2008 2008 2008 2008 2008 2008 2 3 1 Figure 1. Excerpt from the UK Nursing Database 3 4 text, we refer to this file as benchmarking data. Figure 2 shows an excerpt for the same NHS code as shown in Figure 1. Duplicate entries are due to the different codes (Clinical Commissioning Group, Primary Care Organisation and Local Authority) coterminous the same organisation, which are anonymised to ensure confidentiality. Tagging each organisation with its coterminous bodies (e.g. acute trust, local authority, etc.) enables the reasons behind poor and exemplar performances to be explored: e.g., are emergency department attendances high because patients are dissatisfied with their local - primary care services? Correlation analysis To study correlations among the variables, a matrix was built using Excel's Data Analysis Toolkit. Excel calculates the Pearson product-moment correlation coefficient, which measures linear correlation between two variables and takes values between -1 (negative correlation) and 1 (positive correlation). The thresholds used to establish relationship strengths are shown in Table I. Nursing data re-aggregation was needed to combine multiple observations for a single NHS trust. To accomplish this, the observations were grouped under a unique combination made from organisation name, specialty and year. After observing the correlation matrix, variables that displayed a correlation were highlighted. Scatter-plots were produced to check for compliance with the Pearson product-moment correlation's main assumptions such as: homoscedasticity and outliers. Findings Stage 1 findings UK Nursing Database In this stage, the UK Nursing Database's internal correlations were analysed with focus on correlations between quality scores and the other variables. Quality scores reflect how well patient's needs are assessed and met, ward environment and resources. One area explored was to identify which roles, e.g., ward sister, staff nurse and supporting staff, lead to higher quality scores. However, no correlations involving quality scores and staffing variables were revealed. This finding does not mean that quality scores are independent; staffing levels are likely to influence patient satisfaction (Aiken et al., 2012); however, our data analysis does not reflect that, which is likely to echo data depth and veracity nationally. Routinely collected data in this data set is periodic and collected with varying regularity, i.e., from a daily or shift basis to biannual. Possible correlations between specific activities such as talking directly to patient or helping patient to eat and drink and quality scores were also analysed to explore whether an activity leads to an increase or decrease in patient satisfaction. No correlations were found in these data sets either. However, it is possible that data do not reflect this or that there is a non-linear or multivariate correlation that is not captured by the Pearson product-moment correlation. Medium and strong correlations were found among variables that we would expect to be correlated such as funded and actual full time equivalents (FTE). For example, funded senior staff nurse per occupied bed has a large positive correlation with actual senior staff nurse per occupied bed (Figure 3), which implies that managers attempt to staff wards to complement them. As expected, the same behaviour is observed for support staff, ward sisters and assistant practitioners, which implies funded and actual staffing are co-linear. Another strongly positive correlation was found between actual senior staff nurse per occupied bed and daily cost per occupied bed and actual junior staff nurse per occupied bed; in Agenda for Change Bands 5 and 6 (Figures 4 and 5), which confirms that the two most common staff groups drive nursing costs. Organisat Organis at Organisat Organisat Access Admissions- Beds Births-Hspti- ion-CCG ion-PCO ion-Hsptl ion-LA Hsptl (23) CCG (100) Hsptl (27) Attendant (3) Deaths Employment Finance- Morbidity- CCG (30) CCG-MH (9) CCG (84) CCG (123) Quality Quality-PatSat- Staff-Staffing- QOF-CCG ED (64) Hsptl (76) Acute 2,333 1,789.4 35 2.41 1.7168188 8.50607769 3.82 3.82 1,008 1,008 98.25625 98.27697 2,333 30 13.02 1.5596777 30 Acute Acute Acute Acute Acute 845.93 915.64 915.64 915.64 915.64 915.64 1,008 1,008 1,008 3.82 3.82 3.82 3.82 13.02 13.02 1,855.2 1,855.2 1,855.2 1,855.2 1,855.2 98.27697 98.27697 2,333 2,333 2,333 2,333 30 1.5596777 1.5596777 1.5596777 1.5596777 6,060.43532 6,060.43532 6,060.43532 6,060.43532 6,060.43532 6,060.43532 8.50607769 8.50607769 8.50607769 8.50607769 8.50607769 30 13.02 98.27697 98.27697 1,008 30 13.02 IJHCQA 30,3 Although we would have to have a deeper understanding of how cost per bed is calculated, it would be interesting to analyse which staff leads to higher costs, which could help identify cost-efficient care models. For example, whether there is a comparable difference in cost among actual, funded or temporary staff, or which staff grade (ward sister, staff nurse or assistant practitioner) leads to a higher cost. In this instance, there are not enough observations for all staff qualifications to be compared against each other. Other studies show the benefit of graduate RNs (Needleman et al., 2011) on areas such as survival and other outcomes, but our data set did not bear scrutiny. Analysis was repeated after restricting 240 Correlation coefficient Strength of association Positive Negative Table I. Thresholds used to establish correlation strength Small Medium Large 0.1 to 0.3 0.3 to 0.5 0.5 to 1.0 -0.1 to -0.3 -0.3 to -0.5 -0.5 to - 1.0 1.2 1 0.8 Funded senior staff nurse (Band 6) FTEs per occupied bed 0.6 0.4 0.2 Figure 3. Funded senior staff nurse per occupied bed vs actual senior staff nurse per occupied bed 1.2 0 0.2 0.4 0.6 0.8 1 Actual senior staff nurse (Band 6) FTEs per occupied bed Notes: r=0.95, n=137 800 700 600 500 Staff cost (daily) per occupied bed (s) 400 300 200 100 Figure 4. Staff cost per occupied bed vs actual senior staff nurse per occupied bed and actual Band 5 junior staff nurse per occupied bed 10 2 6 8 Actual junior staff nurse (Band 5) FTEs per occupied bed Notes: r=0.79, n=951 data to the last five years (2010-2015) to mitigate time effects. There were no additional Nurse staffing findings and the correlations showed the same behaviour as when all data were included. levels and These results only represent the UK Nursing Database file and therefore should not be outcomes extrapolated to represent the wider health system and workforce. 241 Stage 2 findings - NHS Benchmarking Database Internal correlations in the benchmarking data set were analysed in Stage 2. Some predictable large positive correlations among public health achievement scores and scores for long-term conditions, mental health, musculoskeletal and staff satisfaction were found. Increasing communication between senior manager and staff leads to increased staff satisfaction, i.e., overall engagement and feeling supported by their immediate managers (Figures 6 and 7). Although these insights are valuable, our primary focus was to explore the correlation between safety outcomes such as falls, venous thromboembolism, urinary tract infections (UTI) and those concerning pressure ulcers. No large correlations were found except for a medium strength positive correlation between pressure ulcers and UTI (Figure 8), which may suggest co-morbidity. Figures 9-11 are variables that exhibited large 700 . 600 500 400 Staff cost (daily) per occupied bed (ES) 300 200 100 Figure 5. Staff cost per occupied bed vs "actual senior staff nurse per occupied bed" and "actual Band 6 staff nurse per occupied bed" 5 0 0 2 3 4 Actual senior staff nurse (Band 6) FTEs per occupied bed Notes: r=0.76, n=533 4.1 4 jagement score 3.9 3.8 3.7 3.6 NHS staff survey: overall 3.5 Figure 6. Percentage reporting good communication between senior managers and staff vs overall engagement score and support from immediate managers (overall scores) 60 3.3 0 10 20 30 40 50 NHS staff survey: KF 21. Percentage reporting good communication between senior management and staff Notes: r=0.81, n-238 4.1 IJHCQA 30,3 4 3.9 3.8 242 NHS staff survey: KF 9. Support from immediate managers 3.7 3.6 3.5 3.4 Figure 7. Percentage reporting good communication between senior managers and staff vs overall engagement score and support from immediate managers (focussed question) 60 3. 0 10 20 30 40 50 NHS staff survey: KF 21. Percentage reporting good communication between senior management and staff Notes: r=0.70, n=240 3 2.5 2 2 Safety thermometer, UTI 1.5 1 1 0.5 Figure 8 Urinary tract infections (UTI) 0 2 10 12 4 6 8 Safety thermometer, ulcers , vs ulcers Notes: r=0.41, n=648 4.2 4 4 3.8 3.6 3.4 3.2 Figure 9. Overall engagement vs percentage agreeing that feedback from patients is used to make informed decisions in their department 3 80 30 40 50 60 70 NHS staff survey: KF 29. Percentage agreeing that feedback from patients is used to make informed decisions in their department Notes: r=0.68, n-239 correlations in greatest strength order. These are NHS staff survey: percentage agreeing Nurse staffing that feedback from patients is used to make informed decisions in their department" with levels and "overall engagement score" (Figures 9 and 10) and IAPT (psychological therapy) referrals outcomes moving off sick pay and IAPT referrals completing their treatment" (Figure 11), which indicates an encouraging relationship between these variables and outcome. 243 Findings The UK Nursing Data set contains data regarding patients, quality scores, nurse staffing levels and staff activity for NHS trust sub sets. No correlation was found between quality scores and staffing levels. Quality scores reveal different patient satisfaction aspects, so in reality, there should be a correlation; however, data did not reflect an association. This is contrary to previous findings in other data sets, which suggest a relationship and is therefore explored further here, which is likely to tell us about the current data collection's veracity. No correlation was found between staff activities and quality scores. Positive correlations were found among variables that we expect to be correlated, such as funded vs actual FTEs. Positive correlations were found between actual staff nurse FTES and daily cost per occupied bed. The observations feeding into the correlation analysis spanned 1985-2015. When restricting our observations to the last five years, no significant difference was found from the results spanning 20 years. The second data set we analysed 80 70 NHS staff survey: KF 29. Percentage agreeing that feedback from patients is used to make informed decisions in their department 40 30 Figure 10. Percentage reporting good communication between senior managers and staff vs percentage agreeing that feedback from patients is used to make informed decisions in their department 60 20 10 20 30 40 50 NHS staff survey: KF 21. Percentage reporting good communication between senior management and staff Notes: r=0.64, n=230 1,200 . 1,000 800 600 . 400 200 Figure 11. IAPT (psychological therapy) referrals moving off sick pay vs IAPT referrals completing their treatment 0 10 60 70 20 30 40 50 IAPT referrals moving off sick pay Notes: r=0.63, n=154 IJHCQA 30,3 244 contained variables from the NHS Benchmarking Data relating to patient safety and staff survey results for most NHS organisations. Expected positive correlations were found among public health achievement scores and long-term conditions, mental health and musculoskeletal achievement scores, respectively. Increasing communication between senior manager and staff leads to an increasing staff satisfaction. There were no significant findings on which factors play a role in determining patient satisfaction. There was a slight positive correlation between patient satisfaction among the following variables: overall health gain after four common surgical procedures; public health achievement scores; and high dependency and other long-term condition achievement scores. A positive correlation was found between pressure ulcers and UTI. No other correlations were found between pressure ulcers and other data sets. There was a positive correlation between IAPT (psychological therapy) patients completing their treatment and IAPT patients moving off sick pay. A positive correlation was found between staff reporting good communication among senior managers and staff, and the percentage agreeing that feedback from patients is used to make informed decision in their department Further analysis is needed to study correlations arising from the interaction between two or more variables or correlations that are non-linear. To suggest possible interpretations, we need a deeper understanding of variables involved and how data were collected. Further analysis would need to be done to explore correlations arising from interaction within the variables or to capture non-linear relationships. Discussion Several interesting correlations were revealed; however, a stimulating aspect is specifically identifying routinely collected data set's limitations. In Stages 1 and 2, a few strong correlations were observed within the nursing and benchmarking data sets on their own. Stage 3 studied correlations that could arise when linking the two large data sets; however, owing to various analytical barriers, it was not possible to complete this stage. The following summarises the challenges and limitations that prevent analysis when two data sets are combined and the methods by which routine data are collected. The method applied to look for correlations that might identify opportunities to improve care relies on comparing two or more variables. To compare any one variable with a different variable, both variables must be suitably related. The data presented in these data sets appear not to consistently have the prerequisite relationships between data that are required to conduct analyses. The following points describe four limitations in the data that are major barriers to data set analyses. Point in time Data were collected at different times in both data sets. The NHS Benchmarking Data spans 15 years at the time of analysis). Most variables were collected in 2013 and 2014, so they are relatively recent. On the other hand, the UK Nursing Database spans a broader timeline (data were collected between 1985 and 2015). Therefore, most nursing data do not align with respective comparator indicators. Additionally, nursing data correspond to a short time (one month), whereas they are being compared to benchmarking data collated over different time periods. Workforce delivering the care The UK Nursing Data correspond to only a small fraction of the workforce that delivered service over the whole year. The point in time studies cannot represent ward staffing for the whole year. For example, seasonal variations and even time of week activity, and staffing variance is not captured by the workforce data set. Nurse staffing levels and outcomes Organisation The benchmarking data represents NHS trust level while the nursing-workforce data are broken down by clinical specialty (stroke, acute, surgery, etc.) and Agenda for Change Bands (i.e. 1-8 and job title). The UK Nursing Database does not capture every unit in a hospital, while the benchmarking data incorporates the entire hospital . Therefore, comparing a hospital sub-section to the hospital represents a data challenge. For example, it does not make sense to compare nursing data coming from the intensive care unit with the hospital's wider outcomes, which may include long-stay rehabilitation wards. 245 Patient group receiving care The Nursing Database reflects patients that were present during a specific time. The benchmarking database contains data for all patients in the whole facility, making it inappropriate to compare patients from different age and gender groups. For example, it is not possible to compare data from the children's department against variables that are derived from patients in all age groups, or data from the gynaecology department, which are mostly women. Many benchmarking variables are only suitable for comparison with certain variables owing to the need to meet the rules above. Consequently, the intersection between the two data sets is limited, which does not allow robust analyses. Placing these findings in other research contexts, it may be that a bivariate analysis does not offer enough sensitivity owing to their complex underlying relationships. For example, using the NHS Benchmarking Database, if acute trusts are divided into quartiles according to death rates and staff to bed ratios, and the top and bottom quartiles are compared, then there are marked differences. Trusts with the lowest death rates have 3.25 FTE RNs per occupied bed vs 2.53 FTE RNs per occupied bed in acute trusts with the highest death rate. Differences between nurses are repeated across all professional groups (e.g. consultants per occupied bed) (Hurst and Kelley-Patterson, 2016). Since the differences are so marked, then it is odd why Pearson's correlation did not detect such major differences. To support future analysis and add value to possible statistical findings, several factors need to be addressed. A suitably large nursing-workforce data sample should be collected during a consistent timeline so that they can be compared against the benchmarking data. Data collection should target specific research questions, supported by a literature review of existing work in the area. This will decide the required sample size or time. Routine data are currently warehoused in the NHS, but much data collection takes effort and time. The caring data set" should be reviewed, i.e., standard definitions should be set for all data collection before data are collected across multiple sites to ensure that analysis is comparing like with like. The methods we used can be expanded to include regression analysis to understand which staffing configurations lead to a higher patient satisfaction or quality outcomes. Once data integrity is addressed, logistic regression analysis can be used to predict the probability of a patient developing pressure ulcers or other safety outcomes. It should also be possible to develop other methods to mine these data in a way that exposes nursing's non-linear nature and its relationships with outcomes. Other studies suggest a non-linear relationship (Pitkaaho et al., 2014; Staggs and Dunton, 2014). Ultimately, it is possible to develop data sets that link patients, service models and outcomes and use similar methods for future work. Conclusions In this short analysis, correlations were found within the data sets we interrogated. Some were expected, which suggests that our approach and methods are valid, and some are particularly interesting, e.g., the NHS staff survey results showing an apparent relationship between staff satisfaction and senior manager communication. Data available for analysis are vast and we highlight the importance of having an appropriate data set. Most data available to us were collected for other reasons and were not suitable for correlation analysis owing to data inconsistencies. Despite great potential for this analytical method to improve healthcare, evidence-based improvements cannot be gained when routine data are collected so inconsistently. Data for staffing one ward in a trust are not suitable for comparison with outcomes for the whole trust. Future data collection must ensure that all measures and outcomes relate to the same time, patient, staff group and geography. Possibilities and challenges should be examined. As much routine data collection we describe is still collected or inputted manually, urgent questions should be asked regarding efficacy and how staff time is used. We show the potential benefits from further specific studies into correlations between care models or service delivery and outcomes data; however, this analysis will always fundamentally rely on appropriate data being collected and shared for analysis. The current issue and full text archive of this journal is available on Emerald Insight at: www.emeraldinsight.com 0952-6862.htm Nurse staffing levels and outcomes mining the UK national data sets for insight Nurse staffing levels and outcomes 235 Received 25 August 2016 Accepted 12 September 2016 Alison Leary School of Health and Social Care, London South Bank University, London, UK Barbara Tomai, Adrian Swift and Andrew Woodward Centre for Workforce Intelligence, Mouchel Management Consulting Limited, London, UK, and Keith Hurst Hurst Research Ltd, Mansfield, UK Abstract Purpose - Despite the generation of mass data by the nursing workforce, determining the impact of the contribution to patient safety remains challenging. Several cross-sectional studies have indicated a relationship between staffing and safety. The purpose of this paper is to uncover possible associations and explore if a deeper understanding of relationships between staffing and other factors such as safety could be revealed within routinely collected national data sets. Design/methodology/approach - Two longitudinal routinely collected data sets consisting of 30 years of UK nurse staffing data and seven years of National Health Service (NHS) benchmark data such as survey results, safety and other indicators were used. A correlation matrix was built and a linear correlation operation was applied (Pearson product-moment correlation coefficient). Findings - A number of associations were revealed within both the UK staffing data set and the NHS benchmarking data set. However, the challenges of using these data sets soon became apparent Practical implications - Staff time and effort are required to collect these data. The limitations of these data sets include inconsistent data collection and quality. The mode of data collection and the itemset collected should be reviewed to generate a data set with robust clinical application. Originality/value - This paper revealed that relationships are likely to be complex and non-linear; however, the main contribution of the paper is the identification of the limitations of routinely collected data. Much time and effort is expended in collecting this data; however, its validity, usefulness and method of routine national data collection appear to require re-examination Keywords Patient safety, Healthcare data, Nursing outcomes, Staffing, Nurse, Acute, Modelling, Safety Paper type Research paper Introduction Nursing care providers, such as registered nurses (RNS) and unregistered healthcare support workers (HCSW), provide most patient contact time in the UK. This workforce represents the largest staff group and subsequently the largest expenditure (NHS Confederation, 2015). Determining the workforce's contribution to patient safety can be challenging, particularly if safety is more than the absence of harm (Reason, 2000). However, we have an opportunity to explore this relationship by mining two large, UK wide data sets, which include data from a National Health Service (NHS) benchmarking and staffing perspective over several years. Our research focusses on understanding how RN and HCSW staffing levels influence patient outcomes and on identifying patterns that could help improve patient care. Nursing work is complex (Hall , 1964; Ebright et al., 2003; Leary et al, 2008; Warren et al, 2012); however, when portrayed as supply and demand, inpatient nursing work is often represented as This work was funded by NHS England. The authors thank Ruth May, Pauline Milne, Vicky Reed, Ruth Auton, Lynne Wigens and Jane Cummings for commissioning the work; however, the conclusions and recommendations expressed in this paper are the authors' alone. International Journal of Health Care Quality Assurance Vol 30 No. 3, 2017 pp. 235-247 Emerald Publishing Limited DOI 10.1108 THCQA-06-20160118 linear tasks that are deterministic. If nursing work is linear, then staffing should be a simple calculation but it is not. Nursing work contains other components that are essential for safety, such as care management, avoiding failure to rescue and vigilance. Failure to match staffing to patient needs, therefore, is associated with increasing mortality (Needleman et al., 2011). Consequently, our aim is to better understand the relationships among healthcare variables by mining national data sets. Studies have looked at the relationship between RN numbers and outcomes (Aiken et al., 2002; Clarke and Aiken, 2003). There is also more data about patient care, experiences and outcomes. There is assumed to be a relationship between safety (using proxy measures such as falls, pressure ulcers or other untoward clinical incidents) and RN presence, but this relationship is not fully understood. Whilst there is no simple relationship between either number, nursing skill mix, outcomes and cost (Griffiths, 2009), there have been numerous statistical studies that demonstrate correlations between RN numbers and adverse outcomes (Clarke and Aiken, 2003; Kane et al., 2007) including failure to rescue deteriorating patients, which suggests a relationship with safety. There are two large data sets in the UK that contain several variables and indicators from NHS trusts. One data set contains routinely collected benchmarking data for most English organisations, with predetermined key performance indicators relating to the facility, patient safety and staffing. We call this the NHS Benchmarking Database. The other data set contains detailed nursing data from several NHS organisations across many years, which we call the UK Nursing Database. Our objective was to analyse these data sets to draw insight and explore potential relationships among them. Both data sets have been curated over several years by one individual, which results in consistency. Our second objective was to find possible intra-data set correlations among variables in the two databases and explore whether additional insight could be drawn from merging them. We highlight results from our correlation analysis and lay out the caveats and challenges to gain insight from these two routinely collected data sets. Methodology Both databases were anonymised and supplied as two comma-separated value files. To carry out the analysis, a correlation matrix was built from all relevant variables from each database. After examining these files, a phased analytical approach was spread across three different stages. The first two stages analysed possible intra-data set correlations and the third was an inter-data set analysis. However, this proved problematic owing to data set limitations, which we examine in detail. Consequently, we discuss the challenges and caveats when linking two independent databases. Data sets The UK Nursing Database The UK Nursing Database, designed, built and maintained by Keith Hurst, is unique and includes 2,800 acute, mental health and learning disability service wards, departments and community teams. Seven data sets are collected systematically in each ward/ department/team: (1) bed occupancy/daily contact rates, throughput, patient dependency/acuity; (2) staff activity (i.e. direct care, indirect care, associated work and personal time) grade, and ready-for-action (unproductive) time; (3) service quality (care pathway scores); (4) budgeted, actual, temporary and recommended staffing by grade; Nurse staffing levels and outcomes 237 (5) ward design or local population demographics; 6 time-out (annual, study, sick, maternity and compassionate leave); and (7) staffing costs. In the acute wards, for example, 1.8 million staff activities have been logged to date, which are used to: generate the Safer Nursing Care Tool (formerly AUKUH) staffing multipliers (recently endorsed by National Institute for Health and Care Excellence, 2014); and benchmark each ward/department/service's performance against its counterparts. The database's power lies in the way that patient dependency/acuity, staff activity, ward design/locality demographics, workload and staffing can be related to nursing quality: e.g., high workload, understaffed and grade-mixed dilute wards (i.e. proportionally more HCSW) can be connected to low-quality scores. Data collection started in 1985 and around three wards/departments/teams are added to the database each week, which relies on trust managers to volunteer data (75 trusts have contributed data to date). Participating trusts represent all 12 NHS hospital categories: e.g., small peripheral hospitals to specialist services. Because each trust is named in the UK Nursing Database, its data can be connected to corresponding data in the NHS Benchmarking Database. Figure 1 shows a short excerpt for a given NHS code. The NHS Benchmarking Database The NHS Benchmarking Database, funded by NHS England and hosted by West London University, is used mainly to profile NHS trusts. It includes every NHS England acute, mental health/learning disability and community trust. The database, designed, developed and managed by Keith Hurst, contains 1,650 data sets subsumed under 86 workforce planning and development-related categories, such as staff to bed ratios. Data sources include the Health and Social Care Information Centre (now called NHS Digital), Care Quality Commission, Monitor, Department of Health, NHS England, Public Health England and the Office for National Statistics. Indicators include mortality rates, patient experience and staffing data (by professional group). The database is designed to filter high- and low-quality trusts (measured using several unconnected data sets, such as patient satisfaction) and to examine local questions and issues (eg. why are some staff more productive? Why is staff turnover so high?). The database was developed to support trust managers exploring, for example, their staffing levels and to benchmark trusts against comparable providers. Data stop at trust level (unlike data in the UK Nursing Database, which are gathered at ward/department/team level). The NHS Benchmarking Database contains data from about 260 English organisations spanning the years 2005-2014 (at the time of analysis). We focus on 53 performance, staffing and patient satisfaction variables. Throughout the Cler Supl 36 47 1 3 1 2 2 Year 2008 2008 2008 2008 2008 2008 2008 2008 2008 2008 2008 3 Spec Cardiology Cardiology Cardiology Cardiology Cardiology Cardiology Cardiology Cardiology Crit CareCCU CritCareCCU CritCareCCU Crit CareCCU CritCareCCU CritCareCCU EldAcute EldAcute EldAcute EldAcute EldAcute 5 Mnth 2 2 2 2 2 2 2 2 2. 2 4 4 4 4 4 4 4 1 1 1 1 1 1 1 Grade 7 ws nrs spc 7ws 6 sn 5 sn 3 hca 2 hs http 2 CI Oirr 6 wsnrs spc 6 WS 5 sn bnk 5 sn 4 hca 3 hca 7 WS 6WS 5 sn 3 hca 2 hca Patients 31.4 31.4 31.4 31.4 31.4 31.4 31.4 31.4 8.04 8.04 8.04 8.04 8.04 8.04 33 33 33 33 33 Ward 100 100 100 100 100 100 100 100 94 94 94 94 94 94 92 92 92 92 92 Over 94 94 94 94 94 94 94 94 95 95 95 95 95 95 B6 86 86 86 86 ActS SW Bed Cost 84.13105 84.13105 84.13105 84.13105 84.13105 84.13105 84.13105 84.13105 0.11904B 245.2177 0.11904B 245.2177 0.119048 245.2177 0.119048 245.2177 0.119048 245.2177 0.119048 245.2177 81.977 81.977 81.977 81.977 81.977 18 2008 41 5 10 2008 2008 2008 2008 2008 2008 2008 2 3 1 Figure 1. Excerpt from the UK Nursing Database 3 4 text, we refer to this file as benchmarking data. Figure 2 shows an excerpt for the same NHS code as shown in Figure 1. Duplicate entries are due to the different codes (Clinical Commissioning Group, Primary Care Organisation and Local Authority) coterminous the same organisation, which are anonymised to ensure confidentiality. Tagging each organisation with its coterminous bodies (e.g. acute trust, local authority, etc.) enables the reasons behind poor and exemplar performances to be explored: e.g., are emergency department attendances high because patients are dissatisfied with their local - primary care services? Correlation analysis To study correlations among the variables, a matrix was built using Excel's Data Analysis Toolkit. Excel calculates the Pearson product-moment correlation coefficient, which measures linear correlation between two variables and takes values between -1 (negative correlation) and 1 (positive correlation). The thresholds used to establish relationship strengths are shown in Table I. Nursing data re-aggregation was needed to combine multiple observations for a single NHS trust. To accomplish this, the observations were grouped under a unique combination made from organisation name, specialty and year. After observing the correlation matrix, variables that displayed a correlation were highlighted. Scatter-plots were produced to check for compliance with the Pearson product-moment correlation's main assumptions such as: homoscedasticity and outliers. Findings Stage 1 findings UK Nursing Database In this stage, the UK Nursing Database's internal correlations were analysed with focus on correlations between quality scores and the other variables. Quality scores reflect how well patient's needs are assessed and met, ward environment and resources. One area explored was to identify which roles, e.g., ward sister, staff nurse and supporting staff, lead to higher quality scores. However, no correlations involving quality scores and staffing variables were revealed. This finding does not mean that quality scores are independent; staffing levels are likely to influence patient satisfaction (Aiken et al., 2012); however, our data analysis does not reflect that, which is likely to echo data depth and veracity nationally. Routinely collected data in this data set is periodic and collected with varying regularity, i.e., from a daily or shift basis to biannual. Possible correlations between specific activities such as talking directly to patient or helping patient to eat and drink and quality scores were also analysed to explore whether an activity leads to an increase or decrease in patient satisfaction. No correlations were found in these data sets either. However, it is possible that data do not reflect this or that there is a non-linear or multivariate correlation that is not captured by the Pearson product-moment correlation. Medium and strong correlations were found among variables that we would expect to be correlated such as funded and actual full time equivalents (FTE). For example, funded senior staff nurse per occupied bed has a large positive correlation with actual senior staff nurse per occupied bed (Figure 3), which implies that managers attempt to staff wards to complement them. As expected, the same behaviour is observed for support staff, ward sisters and assistant practitioners, which implies funded and actual staffing are co-linear. Another strongly positive correlation was found between actual senior staff nurse per occupied bed and daily cost per occupied bed and actual junior staff nurse per occupied bed; in Agenda for Change Bands 5 and 6 (Figures 4 and 5), which confirms that the two most common staff groups drive nursing costs. Organisat Organis at Organisat Organisat Access Admissions- Beds Births-Hspti- ion-CCG ion-PCO ion-Hsptl ion-LA Hsptl (23) CCG (100) Hsptl (27) Attendant (3) Deaths Employment Finance- Morbidity- CCG (30) CCG-MH (9) CCG (84) CCG (123) Quality Quality-PatSat- Staff-Staffing- QOF-CCG ED (64) Hsptl (76) Acute 2,333 1,789.4 35 2.41 1.7168188 8.50607769 3.82 3.82 1,008 1,008 98.25625 98.27697 2,333 30 13.02 1.5596777 30 Acute Acute Acute Acute Acute 845.93 915.64 915.64 915.64 915.64 915.64 1,008 1,008 1,008 3.82 3.82 3.82 3.82 13.02 13.02 1,855.2 1,855.2 1,855.2 1,855.2 1,855.2 98.27697 98.27697 2,333 2,333 2,333 2,333 30 1.5596777 1.5596777 1.5596777 1.5596777 6,060.43532 6,060.43532 6,060.43532 6,060.43532 6,060.43532 6,060.43532 8.50607769 8.50607769 8.50607769 8.50607769 8.50607769 30 13.02 98.27697 98.27697 1,008 30 13.02 IJHCQA 30,3 Although we would have to have a deeper understanding of how cost per bed is calculated, it would be interesting to analyse which staff leads to higher costs, which could help identify cost-efficient care models. For example, whether there is a comparable difference in cost among actual, funded or temporary staff, or which staff grade (ward sister, staff nurse or assistant practitioner) leads to a higher cost. In this instance, there are not enough observations for all staff qualifications to be compared against each other. Other studies show the benefit of graduate RNs (Needleman et al., 2011) on areas such as survival and other outcomes, but our data set did not bear scrutiny. Analysis was repeated after restricting 240 Correlation coefficient Strength of association Positive Negative Table I. Thresholds used to establish correlation strength Small Medium Large 0.1 to 0.3 0.3 to 0.5 0.5 to 1.0 -0.1 to -0.3 -0.3 to -0.5 -0.5 to - 1.0 1.2 1 0.8 Funded senior staff nurse (Band 6) FTEs per occupied bed 0.6 0.4 0.2 Figure 3. Funded senior staff nurse per occupied bed vs actual senior staff nurse per occupied bed 1.2 0 0.2 0.4 0.6 0.8 1 Actual senior staff nurse (Band 6) FTEs per occupied bed Notes: r=0.95, n=137 800 700 600 500 Staff cost (daily) per occupied bed (s) 400 300 200 100 Figure 4. Staff cost per occupied bed vs actual senior staff nurse per occupied bed and actual Band 5 junior staff nurse per occupied bed 10 2 6 8 Actual junior staff nurse (Band 5) FTEs per occupied bed Notes: r=0.79, n=951 data to the last five years (2010-2015) to mitigate time effects. There were no additional Nurse staffing findings and the correlations showed the same behaviour as when all data were included. levels and These results only represent the UK Nursing Database file and therefore should not be outcomes extrapolated to represent the wider health system and workforce. 241 Stage 2 findings - NHS Benchmarking Database Internal correlations in the benchmarking data set were analysed in Stage 2. Some predictable large positive correlations among public health achievement scores and scores for long-term conditions, mental health, musculoskeletal and staff satisfaction were found. Increasing communication between senior manager and staff leads to increased staff satisfaction, i.e., overall engagement and feeling supported by their immediate managers (Figures 6 and 7). Although these insights are valuable, our primary focus was to explore the correlation between safety outcomes such as falls, venous thromboembolism, urinary tract infections (UTI) and those concerning pressure ulcers. No large correlations were found except for a medium strength positive correlation between pressure ulcers and UTI (Figure 8), which may suggest co-morbidity. Figures 9-11 are variables that exhibited large 700 . 600 500 400 Staff cost (daily) per occupied bed (ES) 300 200 100 Figure 5. Staff cost per occupied bed vs "actual senior staff nurse per occupied bed" and "actual Band 6 staff nurse per occupied bed" 5 0 0 2 3 4 Actual senior staff nurse (Band 6) FTEs per occupied bed Notes: r=0.76, n=533 4.1 4 jagement score 3.9 3.8 3.7 3.6 NHS staff survey: overall 3.5 Figure 6. Percentage reporting good communication between senior managers and staff vs overall engagement score and support from immediate managers (overall scores) 60 3.3 0 10 20 30 40 50 NHS staff survey: KF 21. Percentage reporting good communication between senior management and staff Notes: r=0.81, n-238 4.1 IJHCQA 30,3 4 3.9 3.8 242 NHS staff survey: KF 9. Support from immediate managers 3.7 3.6 3.5 3.4 Figure 7. Percentage reporting good communication between senior managers and staff vs overall engagement score and support from immediate managers (focussed question) 60 3. 0 10 20 30 40 50 NHS staff survey: KF 21. Percentage reporting good communication between senior management and staff Notes: r=0.70, n=240 3 2.5 2 2 Safety thermometer, UTI 1.5 1 1 0.5 Figure 8 Urinary tract infections (UTI) 0 2 10 12 4 6 8 Safety thermometer, ulcers , vs ulcers Notes: r=0.41, n=648 4.2 4 4 3.8 3.6 3.4 3.2 Figure 9. Overall engagement vs percentage agreeing that feedback from patients is used to make informed decisions in their department 3 80 30 40 50 60 70 NHS staff survey: KF 29. Percentage agreeing that feedback from patients is used to make informed decisions in their department Notes: r=0.68, n-239 correlations in greatest strength order. These are NHS staff survey: percentage agreeing Nurse staffing that feedback from patients is used to make informed decisions in their department" with levels and "overall engagement score" (Figures 9 and 10) and IAPT (psychological therapy) referrals outcomes moving off sick pay and IAPT referrals completing their treatment" (Figure 11), which indicates an encouraging relationship between these variables and outcome. 243 Findings The UK Nursing Data set contains data regarding patients, quality scores, nurse staffing levels and staff activity for NHS trust sub sets. No correlation was found between quality scores and staffing levels. Quality scores reveal different patient satisfaction aspects, so in reality, there should be a correlation; however, data did not reflect an association. This is contrary to previous findings in other data sets, which suggest a relationship and is therefore explored further here, which is likely to tell us about the current data collection's veracity. No correlation was found between staff activities and quality scores. Positive correlations were found among variables that we expect to be correlated, such as funded vs actual FTEs. Positive correlations were found between actual staff nurse FTES and daily cost per occupied bed. The observations feeding into the correlation analysis spanned 1985-2015. When restricting our observations to the last five years, no significant difference was found from the results spanning 20 years. The second data set we analysed 80 70 NHS staff survey: KF 29. Percentage agreeing that feedback from patients is used to make informed decisions in their department 40 30 Figure 10. Percentage reporting good communication between senior managers and staff vs percentage agreeing that feedback from patients is used to make informed decisions in their department 60 20 10 20 30 40 50 NHS staff survey: KF 21. Percentage reporting good communication between senior management and staff Notes: r=0.64, n=230 1,200 . 1,000 800 600 . 400 200 Figure 11. IAPT (psychological therapy) referrals moving off sick pay vs IAPT referrals completing their treatment 0 10 60 70 20 30 40 50 IAPT referrals moving off sick pay Notes: r=0.63, n=154 IJHCQA 30,3 244 contained variables from the NHS Benchmarking Data relating to patient safety and staff survey results for most NHS organisations. Expected positive correlations were found among public health achievement scores and long-term conditions, mental health and musculoskeletal achievement scores, respectively. Increasing communication between senior manager and staff leads to an increasing staff satisfaction. There were no significant findings on which factors play a role in determining patient satisfaction. There was a slight positive correlation between patient satisfaction among the following variables: overall health gain after four common surgical procedures; public health achievement scores; and high dependency and other long-term condition achievement scores. A positive correlation was found between pressure ulcers and UTI. No other correlations were found between pressure ulcers and other data sets. There was a positive correlation between IAPT (psychological therapy) patients completing their treatment and IAPT patients moving off sick pay. A positive correlation was found between staff reporting good communication among senior managers and staff, and the percentage agreeing that feedback from patients is used to make informed decision in their department Further analysis is needed to study correlations arising from the interaction between two or more variables or correlations that are non-linear. To suggest possible interpretations, we need a deeper understanding of variables involved and how data were collected. Further analysis would need to be done to explore correlations arising from interaction within the variables or to capture non-linear relationships. Discussion Several interesting correlations were revealed; however, a stimulating aspect is specifically identifying routinely collected data set's limitations. In Stages 1 and 2, a few strong correlations were observed within the nursing and benchmarking data sets on their own. Stage 3 studied correlations that could arise when linking the two large data sets; however, owing to various analytical barriers, it was not possible to complete this stage. The following summarises the challenges and limitations that prevent analysis when two data sets are combined and the methods by which routine data are collected. The method applied to look for correlations that might identify opportunities to improve care relies on comparing two or more variables. To compare any one variable with a different variable, both variables must be suitably related. The data presented in these data sets appear not to consistently have the prerequisite relationships between data that are required to conduct analyses. The following points describe four limitations in the data that are major barriers to data set analyses. Point in time Data were collected at different times in both data sets. The NHS Benchmarking Data spans 15 years at the time of analysis). Most variables were collected in 2013 and 2014, so they are relatively recent. On the other hand, the UK Nursing Database spans a broader timeline (data were collected between 1985 and 2015). Therefore, most nursing data do not align with respective comparator indicators. Additionally, nursing data correspond to a short time (one month), whereas they are being compared to benchmarking data collated over different time periods. Workforce delivering the care The UK Nursing Data correspond to only a small fraction of the workforce that delivered service over the whole year. The point in time studies cannot represent ward staffing for the whole year. For example, seasonal variations and even time of week activity, and staffing variance is not captured by the workforce data set. Nurse staffing levels and outcomes Organisation The benchmarking data represents NHS trust level while the nursing-workforce data are broken down by clinical specialty (stroke, acute, surgery, etc.) and Agenda for Change Bands (i.e. 1-8 and job title). The UK Nursing Database does not capture every unit in a hospital, while the benchmarking data incorporates the entire hospital . Therefore, comparing a hospital sub-section to the hospital represents a data challenge. For example, it does not make sense to compare nursing data coming from the intensive care unit with the hospital's wider outcomes, which may include long-stay rehabilitation wards. 245 Patient group receiving care The Nursing Database reflects patients that were present during a specific time. The benchmarking database contains data for all patients in the whole facility, making it inappropriate to compare patients from different age and gender groups. For example, it is not possible to compare data from the children's department against variables that are derived from patients in all age groups, or data from the gynaecology department, which are mostly women. Many benchmarking variables are only suitable for comparison with certain variables owing to the need to meet the rules above. Consequently, the intersection between the two data sets is limited, which does not allow robust analyses. Placing these findings in other research contexts, it may be that a bivariate analysis does not offer enough sensitivity owing to their complex underlying relationships. For example, using the NHS Benchmarking Database, if acute trusts are divided into quartiles according to death rates and staff to bed ratios, and the top and bottom quartiles are compared, then there are marked differences. Trusts with the lowest death rates have 3.25 FTE RNs per occupied bed vs 2.53 FTE RNs per occupied bed in acute trusts with the highest death rate. Differences between nurses are repeated across all professional groups (e.g. consultants per occupied bed) (Hurst and Kelley-Patterson, 2016). Since the differences are so marked, then it is odd why Pearson's correlation did not detect such major differences. To support future analysis and add value to possible statistical findings, several factors need to be addressed. A suitably large nursing-workforce data sample should be collected during a consistent timeline so that they can be compared against the benchmarking data. Data collection should target specific research questions, supported by a literature review of existing work in the area. This will decide the required sample size or time. Routine data are currently warehoused in the NHS, but much data collection takes effort and time. The caring data set" should be reviewed, i.e., standard definitions should be set for all data collection before data are collected across multiple sites to ensure that analysis is comparing like with like. The methods we used can be expanded to include regression analysis to understand which staffing configurations lead to a higher patient satisfaction or quality outcomes. Once data integrity is addressed, logistic regression analysis can be used to predict the probability of a patient developing pressure ulcers or other safety outcomes. It should also be possible to develop other methods to mine these data in a way that exposes nursing's non-linear nature and its relationships with outcomes. Other studies suggest a non-linear relationship (Pitkaaho et al., 2014; Staggs and Dunton, 2014). Ultimately, it is possible to develop data sets that link patients, service models and outcomes and use similar methods for future work. Conclusions In this short analysis, correlations were found within the data sets we interrogated. Some were expected, which suggests that our approach and methods are valid, and some are particularly interesting, e.g., the NHS staff survey results showing an apparent relationship between staff satisfaction and senior manager communication. Data available for analysis are vast and we highlight the importance of having an appropriate data