Answered step by step
Verified Expert Solution
Question
1 Approved Answer
Year Close 1983 1258.64 1984 1211.57 1985 1546.67 1986 1895.95 1987 1938.83 1988 2168.57 1989 2753.20 1990 2633.66 1991 3168.83 1992 3301.11 1993 3754.09 1994
Year Close 1983 1258.64 1984 1211.57 1985 1546.67 1986 1895.95 1987 1938.83 1988 2168.57 1989 2753.20 1990 2633.66 1991 3168.83 1992 3301.11 1993 3754.09 1994 3834.44 1995 5117.12 1996 6448.27 1997 7908.25 1998 9181.43 1999 11497.12 2000 10786.85 2001 10021.50 2002 8341.63 2003 10453.92 2004 10783.01 2005 10717.50 2006 12463.15 2007 13264.82 2008 8776.39 2009 10428.05 2010 11557.50 2011 12217.60 2012 13104.10
Using the accompanying table, determine the most appropriate forecasting technique for the accompanying data about the Dow Jones Industrial Average yearly closing value and implement the model. Click here to view the table. Click here to view the data. What is the most appropriate forecasting technique? i Definition O A. Simple moving average OB. Holt-Winters multiplicative seasonality models without trend or multiple regression C. Holt-Winters multiplicative seasonality models with trend OD. Double exponential smoothing O E. Simple exponential smoothing OF. Holt-Winters additive seasonality models with trend O G. Holt-Winters additive seasonality models without trend or multiple regression Use the model forecast the closing value for 2016. Use a=0.8, B = 0.4, and y=0.6, as needed. F2016 - (Round to two decimal places as needed.) Year 1983 1984 1985 1986 1987 1988 1989 1990 1991 1992 1993 1994 1995 1996 1997 1998 1999 2000 2001 2002 2003 2004 2005 2006 2007 2008 2009 2010 2011 2012 i More Info Close 1258.64 1211.57 1546.67 1895.95 1938.83 2168.57 2753.20 2633.66 3168.83 3301.11 3754.09 3834.44 5117.12 6448.27 7908.25 9181.43 11497.12 10786.85 10021.50 8341.63 10453.92 10783.01 10717.50 12463.15 13264.82 8776.39 10428.05 11557.50 12217.60 13104.10 No trend Forecasting Model Choice No Seasonality Seasonality Simple moving average or simple Holt-Winters additive or exponential smoothing multiplicative seasonality models without trend or multiple regression Double exponential smoothing Holt-Winters additive or multiplicative seasonality models with trend Trend Print Done Print Done Using the accompanying table, determine the most appropriate forecasting technique for the accompanying data about the Dow Jones Industrial Average yearly closing value and implement the model. Click here to view the table. Click here to view the data. What is the most appropriate forecasting technique? i Definition O A. Simple moving average OB. Holt-Winters multiplicative seasonality models without trend or multiple regression C. Holt-Winters multiplicative seasonality models with trend OD. Double exponential smoothing O E. Simple exponential smoothing OF. Holt-Winters additive seasonality models with trend O G. Holt-Winters additive seasonality models without trend or multiple regression Use the model forecast the closing value for 2016. Use a=0.8, B = 0.4, and y=0.6, as needed. F2016 - (Round to two decimal places as needed.) Year 1983 1984 1985 1986 1987 1988 1989 1990 1991 1992 1993 1994 1995 1996 1997 1998 1999 2000 2001 2002 2003 2004 2005 2006 2007 2008 2009 2010 2011 2012 i More Info Close 1258.64 1211.57 1546.67 1895.95 1938.83 2168.57 2753.20 2633.66 3168.83 3301.11 3754.09 3834.44 5117.12 6448.27 7908.25 9181.43 11497.12 10786.85 10021.50 8341.63 10453.92 10783.01 10717.50 12463.15 13264.82 8776.39 10428.05 11557.50 12217.60 13104.10 No trend Forecasting Model Choice No Seasonality Seasonality Simple moving average or simple Holt-Winters additive or exponential smoothing multiplicative seasonality models without trend or multiple regression Double exponential smoothing Holt-Winters additive or multiplicative seasonality models with trend Trend Print Done Print DoneStep by Step Solution
There are 3 Steps involved in it
Step: 1
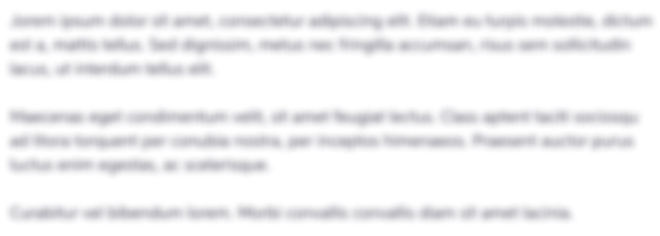
Get Instant Access to Expert-Tailored Solutions
See step-by-step solutions with expert insights and AI powered tools for academic success
Step: 2

Step: 3

Ace Your Homework with AI
Get the answers you need in no time with our AI-driven, step-by-step assistance
Get Started