Question
*You need to explain these changes in p-values in terms of changes in sums of squares and/or degrees of freedom for the factor and/or the
*You need to explain these changes in p-values in terms of changes in sums of squares and/or degrees of freedom for the factor and/or the residual. The options in the quiz will be:
- higher explained MSS because of higher explained SS
- higher explained MSS because of lower degrees of freedom
- lower residual MSS
- higher explained MSS because of higher explained SS, and also lower residual MSS
- higher explained MSS because of lower degrees of freedom, and also lower residual MSS
- higher explained MSS because of higher explained SS and lower degrees of freedom, and also lower residual MSS. (MSS means mean sum of squares and SS means sum of squares)
(I'm supposed to match the above answers to questions 1-11 but I don't get it)
The open black circle is the brine outlet, the 10 sites represented by the black dots are in a ring 50m from the outlet, the 10 sites represented by the red dots are in a ring 500m from the outlet and the 10 sites represented by the blue dots are in a ring 1000m from the outlet. The location of the sites around the ring is chosen at random, while ensuring that the sites are within areas previously reported as being good quality seagrass meadows. She carries out a relative biomass estimate at each site, based on the density of the visible leaves, and also records the depth below sea level of each site. The data she collects is in the 'gabys data.xls' file.
- First, use a one-way ANOVA (not a regression) to look at whether there is a relationship between the distance of the sites from the outlet, and their depth ie. is there a general trend in topology that could confound our results. Is there a significant relationship? Record the p-value you get for this test. If there is a significant relationship, use a boxplot and a TukeyHSD test to decide where the deepest sites are located (at which distance(s)).
- Use a one-way ANOVA (not a regression) to look at whether there is a relationship between the distance of the sites from the outlet, and their relative biomass. Is there a significant relationship? Record the p-value you get for this test. If there is a significant relationship, use a boxplot and a TukeyHSD test to decide where the sites with greatest biomass are located (at which distance(s)).
- Use a linear regression analysis to look at whether there is a relationship between the distance of the sites from the outlet, and their relative biomass. Is there a significant relationship? Record the F-value you get for this test. If there is a significant relationship, does biomass increase or decrease with greater distance?
- Use a linear regression analysis to look at whether there is a relationship between the distance of the sites from the outlet, and their relative biomass. Is there a significant relationship? Record the F-value you get for this test. If there is a significant relationship, does biomass increase or decrease with greater distance?
- Is there a difference in the conclusions to be drawn from the ANOVA test and the regression test on the effect of distance on biomass?
- Use a linear regression analysis to look at whether there is a relationship between the depth of the sites, and their relative biomass. Is there a significant relationship? Record the F-value you get for this test. If there is a significant relationship, does biomass increase or decrease with greater depth?
- Now, use an ANCOVA analysis with an interaction looking at the effects of both distance (as a factor) and depth (as a covariate). Since depth is the covariate and not the main thing we are interested in, we normally put this as the first explanatory variable in the linear model, so its effect is taken out first. Make sure you do it that way this time. Which of the main effects and interactions are significant (at =0.05)?
- Now try fitting the same linear model but with distance as the first explanatory variable. Notice that it makes a difference to the results! Which effect was significant in one of these models but not in the second? And the p-value of which effect did not change at all between the models?
- Now try fitting a linear model with depth as the first explanatory variable and distance as the second, and an interaction, but with both of the variables as covariates, not factors (a multiple regression). This is possible because both variables are numeric. Notice that it also makes a difference to the results, with all the p-values being lower now, compared to the ANCOVA analysis with depth first! Why is the p-value for depth smaller?* Why is the p-value for distance smaller?* Why is the p-value for the interaction smaller?*
- Does the result we got in part e) for the (non) significance of depth contradict the result we get here? Why/ why not?
- Since the last model has a smaller residual mean squares error, we can conclude that it is the best model (there are actually many ways to decide which is the best model, but this is one possible way). Given the significant interaction in this model, and carefully considering the data, what conclusion could we draw regarding the effects of salinity and depth on seagrass biomass (assuming that greater distance from the outlet does indeed mean less effect of salinity)?
Step by Step Solution
There are 3 Steps involved in it
Step: 1
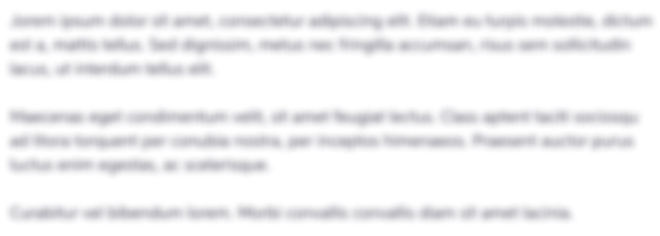
Get Instant Access to Expert-Tailored Solutions
See step-by-step solutions with expert insights and AI powered tools for academic success
Step: 2

Step: 3

Ace Your Homework with AI
Get the answers you need in no time with our AI-driven, step-by-step assistance
Get Started