Question
Your methodology should contain the following parts or answer the following questions: What type of study are you proposing? What variables will you be measuring?
Your methodology should contain the following parts or answer the following questions:
- What type of study are you proposing?
- What variables will you be measuring?
- What is your hypothesis?
- What types of data will you be using?
- What are the components of your study (research questions, Units of analysis)
Submit a document which outlines your proposed methodology. You can use the responses from the questions presented above as a template for how your document will be organized.
THE PAPER:
Forensic analysis plays a crucial role in criminal investigations, aiding law enforcement agencies in identifying perpetrators and securing convictions. With advancements in technology, particularly in the realm of artificial intelligence (AI), forensic techniques have evolved to incorporate AI-based tools such as facial recognition and automated fingerprint identification systems (AFIS). These technologies promise greater efficiency and accuracy in identifying suspects and analyzing evidence. However, despite the potential benefits, the adoption of AI-based forensic technologies raises significant questions regarding their accuracy, reliability, and legal implications. It is imperative to assess the effectiveness of these technologies and understand how they are perceived by legal professionals and policymakers to ensure fair and just outcomes in criminal proceedings.
Why Study AI-Based Forensic Technologies:
1. Accuracy and Reliability: The accuracy and reliability of AI-based forensic technologies are crucial factors that determine their efficacy in aiding criminal investigations. Assessing the performance of these technologies is essential to ascertain their suitability for use in real-world scenarios.
2. Legal Implications: The integration of AI in forensic analysis raises complex legal questions regarding the admissibility of AI-generated evidence in court proceedings. Understanding how legal professionals and policymakers perceive the use of AI in forensic analysis is essential for developing appropriate regulatory frameworks and ensuring the fairness of legal proceedings.
The comparison between AI-based forensic techniques, like facial recognition systems and Automated Fingerprint Identification Systems (AFIS), and traditional forensic methods such as fingerprint analysis and DNA profiling, is a topic of ongoing discussion and research in the field of forensic science. Here's a deeper dive into the points you've mentioned:
1. Accuracy and Reliability:
AI-based Facial Recognition Systems and AFIS:
- Advantages:
- Speed: AI-based systems can process large amounts of data rapidly, potentially expediting investigations.
- Automation: They can automate repetitive tasks, reducing the workload on human analysts.
- Scalability: These systems can handle a vast amount of data, making them suitable for large-scale investigations.
- Challenges:
- Quality of Input Data: The accuracy of AI systems heavily relies on the quality and quantity of data used for training. Poor-quality or biased training data can lead to inaccurate results and potential biases in identification.
- Algorithm Sophistication: The effectiveness of AI algorithms varies depending on their complexity and how well they generalize to different scenarios. Some systems may struggle with certain conditions, such as poor lighting or facial occlusions.
- Biases in Training Data: If the training data is not diverse and representative of the population, AI systems may exhibit biases, leading to inaccurate or unfair results, particularly for minority groups.
Traditional Forensic Techniques (Fingerprint Analysis and DNA Profiling):
- Advantages:
- Established Methods: Fingerprint analysis and DNA profiling have been extensively validated and accepted in legal proceedings as reliable methods for identifying individuals.
- High Accuracy: These techniques have high accuracy rates when performed by trained forensic experts using standardized protocols.
- Legal Admissibility: Courts have historically accepted fingerprint and DNA evidence as highly reliable and admissible in legal proceedings.
- Challenges:
- Human Error: Despite their reliability, traditional forensic techniques are not immune to human error, such as misinterpretation of evidence or contamination.
- Time-Consuming: Fingerprint analysis and DNA profiling can be time-consuming processes, especially when dealing with large volumes of evidence.
While AI-based forensic technologies hold promises for enhancing the efficiency and accuracy of forensic investigations, they are still evolving, and their reliability depends on various factors. Traditional forensic techniques like fingerprint analysis and DNA profiling remain pillars of forensic science due to their established reliability and acceptance in legal proceedings. The integration of AI into forensic workflows should be accompanied by rigorous validation, transparency, and ongoing evaluation to ensure their effectiveness and fairness in practice.
- Value as Tools: Some legal professionals and policymakers may see AI-based forensic technologies as valuable tools that can enhance the efficiency and accuracy of criminal investigations. These technologies can automate processes, analyze large volumes of data quickly, and potentially identify patterns or evidence that human investigators might miss. This perspective often emphasizes the potential benefits of AI in improving the criminal justice system's effectiveness.
- Skepticism and Concerns: On the other hand, skepticism can arise due to several concerns. These may include:
- Privacy: Concerns about how AI systems handle sensitive data and the potential for privacy breaches.
- Accuracy: Questions about the reliability and accuracy of AI algorithms, particularly in complex forensic analyses where the stakes are high.
- Biases: Worries about inherent biases in AI algorithms, which could lead to unfair outcomes, particularly for marginalized or vulnerable populations.
- Factors Influencing Acceptance or Skepticism:
- Transparency: The level of transparency in how AI algorithms function and make decisions is crucial. Legal professionals and policymakers may be more accepting of AI technologies if they understand how they work and can verify their outputs.
- Track Record: Real-world applications and case studies demonstrating the effectiveness of AI-based forensic technologies can sway opinions. Positive experiences and success stories can build trust and confidence.
- Ethical Considerations: Concerns about the ethical implications of AI in forensic contexts, such as issues related to consent, fairness, and the potential for unintended consequences.
- Misuse or Abuse: Fears about the misuse or abuse of AI technologies, such as wrongful convictions based on flawed AI analysis or the use of AI for surveillance purposes without appropriate safeguards.
Overall, perceptions of AI-based forensic technologies among legal professionals and policymakers are nuanced and can be influenced by a range of factors, including technological transparency, real-world performance, ethical considerations, and concerns about misuse or abuse. Balancing the potential benefits of these technologies with the need to address their limitations and potential risks is essential for informed decision-making in the criminal justice system.
- Legal Standards and Admissibility:
- Traditional legal standards for evidence admissibility, such as relevance, reliability, and authenticity, may need to be reevaluated in light of AI-generated evidence. Courts may need to establish new criteria to assess the reliability and validity of such evidence.
- Questions may arise regarding the understanding and interpretation of AI-generated evidence by judges and juries who may not be familiar with the underlying technology.
- Data Privacy:
- AI often relies on vast amounts of data to generate insights or predictions. Ensuring the privacy of individuals whose data is used in AI systems is crucial.
- Legal frameworks such as GDPR in Europe or CCPA in California provide guidelines for the collection, storage, and processing of personal data, which must be adhered to when using AI in legal contexts.
- Algorithmic Bias:
- AI algorithms can inherit biases present in the data they are trained on, leading to unfair or discriminatory outcomes. This is particularly concerning in legal contexts where decisions can have profound impacts on individuals' lives.
- Efforts must be made to identify and mitigate biases in AI systems used for generating evidence to ensure fairness and impartiality in legal proceedings.
- Transparency and Accountability:
- There is a need for transparency regarding the methods used to generate AI evidence. Courts, legal professionals, and the public should understand how AI systems arrive at their conclusions.
- Establishing accountability mechanisms is essential to address errors or misconduct related to AI-generated evidence. This may involve ensuring that developers, operators, or users of AI systems can be held responsible for their actions.
- Authentication and Chain of Custody:
- Authenticating AI-generated evidence may pose challenges, especially if the process involves complex algorithms or proprietary software.
- Maintaining a clear chain of custody for AI-generated evidence is essential to establish its reliability and admissibility in court.
- Expert Testimony and Interpretation:
- Legal professionals may need to rely on expert testimony to explain the intricacies of AI technology and the evidence it generates. This highlights the importance of interdisciplinary collaboration between AI specialists and legal experts.
- International and Cross-Jurisdictional Considerations:
- Given the global nature of AI technology and legal systems, harmonizing standards and regulations across jurisdictions is essential to ensure consistency and fairness in the use of AI-generated evidence.
Addressing these legal and ethical implications requires collaboration between policymakers, legal experts, technologists, and ethicists to develop appropriate frameworks and guidelines for the responsible use of AI in legal contexts.
- Inherited Biases from Training Data:
- AI algorithms learn from the data they are trained on. If the training data is biased or lacks diversity, the algorithm will reflect those biases in its decisions.
- For example, facial recognition systems may exhibit racial or gender biases if the training data primarily consists of faces from certain demographics.
- Similarly, Automated Fingerprint Identification Systems (AFIS) may produce false matches if the input data is not diverse or representative of all possible variations in fingerprints.
- Mitigation Strategies:
- Diverse and Representative Training Data: To mitigate biases, it's essential to train AI algorithms on diverse and representative datasets. This means including data from various demographics, ethnicities, genders, and other relevant factors.
- Regular Audits and Evaluations: Continuous monitoring and auditing of AI algorithms are necessary to identify and address any biases or limitations that may arise over time. Regular evaluations help ensure that the algorithms are performing as intended and not exhibiting discriminatory behavior.
- Transparency and Accountability Mechanisms: Implementing mechanisms for transparency and accountability can help ensure that the decision-making process of AI algorithms is understandable and accountable. This includes providing explanations for the decisions made by the algorithms and allowing for recourse if errors or biases are identified.
- Bias Detection and Correction: Employing techniques such as bias detection algorithms and bias mitigation strategies can help identify and correct biases in AI systems. This may involve adjusting algorithms, retraining models with additional data, or implementing preprocessing techniques to mitigate biases in input data.
Regulatory frameworks for AI-based forensic technologies are essential to ensure they are deployed responsibly and ethically. Here's a more detailed breakdown of the points you've mentioned:
- Transparency, Accountability, and Legal Standards:
- Transparency: Regulations should require transparency in the development, operation, and outcomes of AI systems used in forensic investigations. This includes disclosing the algorithms, data sources, and methodologies used.
- Accountability: Clear lines of accountability should be established to hold developers, operators, and users of AI forensic technologies responsible for their actions and decisions.
- Legal Standards: Regulatory frameworks should align with existing legal standards and ensure that AI systems comply with laws related to evidence, privacy, and due process.
- Guidelines for Development and Deployment:
- Establishing Guidelines: Regulatory bodies should develop guidelines for the development, testing, and deployment of AI systems in forensic contexts. These guidelines should address issues such as accuracy, reliability, bias mitigation, and data security.
- Integrity and Security of Data: Regulations should mandate measures to ensure the integrity and security of data used in forensic investigations, including data collection, storage, sharing, and analysis.
- Independent Oversight and Evaluation:
- Mechanisms for Oversight: Regulatory frameworks should establish mechanisms for independent oversight and evaluation of AI-based forensic technologies. This could involve creating specialized regulatory bodies, expert panels, or auditing processes to monitor compliance and assess the impact of these technologies on legal proceedings and individual rights.
- Evaluation Criteria: Criteria for evaluating the performance, fairness, and ethical implications of AI systems should be defined, and regular audits should be conducted to ensure compliance with regulatory standards.
- Collaborative Approach:
- Multidisciplinary Collaboration: Policymakers, legal experts, technologists, and civil society organizations should collaborate in developing regulatory frameworks for AI-based forensic technologies. This ensures that diverse perspectives are considered and that regulations are comprehensive and effective.
- Stakeholder Engagement: Regulatory processes should involve active engagement with stakeholders, including law enforcement agencies, legal practitioners, technology developers, and advocacy groups, to solicit feedback and address concerns.
- Balancing Benefits and Rights:
- Opportunity and Challenges: The adoption of AI-based forensic technologies offers opportunities to enhance the efficiency and effectiveness of criminal investigations. However, it also raises concerns related to accuracy, fairness, ethics, and privacy.
- Safeguarding Rights: Regulatory frameworks should aim to balance the potential benefits of AI technologies with the need to protect individual rights, ensure fairness in criminal proceedings, and uphold ethical standards.
By prioritizing transparency, accountability, adherence to legal standards, and collaboration among stakeholders, regulatory frameworks can help harness the potential of AI-based forensic technologies while mitigating risks and safeguarding individual rights.
The study of AI-based forensic technologies is essential for evaluating their accuracy, reliability, and legal implications in criminal investigations. By addressing research questions related to the performance of these technologies and the perceptions of legal professionals and policymakers, we can develop informed strategies to harness the potential of AI while upholding fairness and justice in the legal system.
Step by Step Solution
There are 3 Steps involved in it
Step: 1
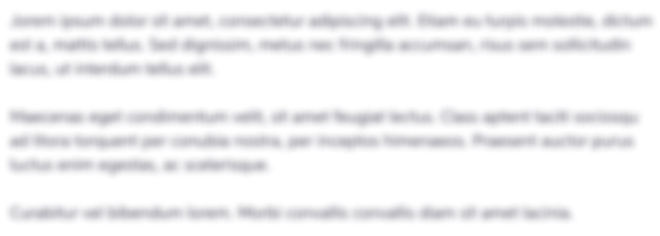
Get Instant Access with AI-Powered Solutions
See step-by-step solutions with expert insights and AI powered tools for academic success
Step: 2

Step: 3

Ace Your Homework with AI
Get the answers you need in no time with our AI-driven, step-by-step assistance
Get Started