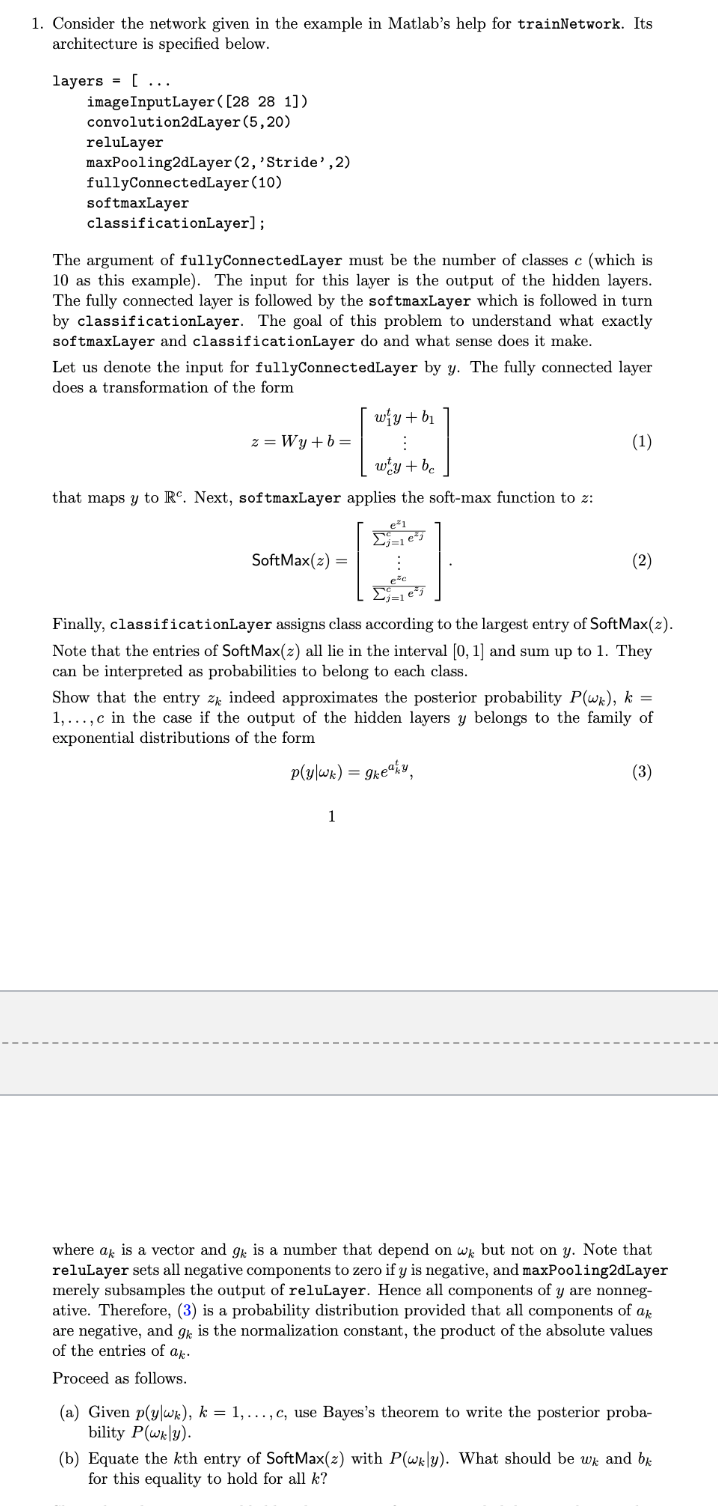
1. Consider the network given in the example in Matlab's help for trainNetwork. Its architecture is specified below. layers = [... image InputLayer([28 28 1]) convolution2dLayer(5,20) reluLayer maxPooling2dLayer(2, 'Stride', 2) fullyConnectedLayer(10) softmaxLayer classificationLayer); The argument of fullyConnectedLayer must be the number of classes c (which is 10 as this example). The input for this layer is the output of the hidden layers. The fully connected layer is followed by the softmaxLayer which is followed in turn by classificationLayer. The goal of this problem to understand what exactly softmaxLayer and classificationLayer do and what sense does it make. Let us denote the input for fullyConnectedLayer by y. The fully connected layer does a transformation of the form wiy + b1 z = Wy+b= : (1) wy+be that maps y to R. Next, softmaxLayer applies the soft-max function to : Dj=es SoftMax(2) = (2) Ees Finally, classificationLayer assigns class according to the largest entry of SoftMax(2). Note that the entries of Soft Max(z) all lie in the interval (0, 1) and sum up to 1. They can be interpreted as probabilities to belong to each class. Show that the entry Zk indeed approximates the posterior probability Plwa), k = 1,...,c in the case if the output of the hidden layers y belongs to the family of exponential distributions of the form p(y|wx) = gke4ly (3) 1 where ak is a vector and gk is a number that depend on wk but not on y. Note that reluLayer sets all negative components to zero if y is negative, and maxPooling2dLayer merely subsamples the output of reluLayer. Hence all components of y are nonneg- ative. Therefore, (3) is a probability distribution provided that all components of ak are negative, and gk is the normalization constant, the product of the absolute values of the entries of ak. Proceed as follows. (a) Given pylwk), k = 1,..., C, use Bayes's theorem to write the posterior proba- bility P( wy). (b) Equate the kth entry of Soft Max(z) with P(wkly). What should be wk and be for this equality to hold for all k? 1. Consider the network given in the example in Matlab's help for trainNetwork. Its architecture is specified below. layers = [... image InputLayer([28 28 1]) convolution2dLayer(5,20) reluLayer maxPooling2dLayer(2, 'Stride', 2) fullyConnectedLayer(10) softmaxLayer classificationLayer); The argument of fullyConnectedLayer must be the number of classes c (which is 10 as this example). The input for this layer is the output of the hidden layers. The fully connected layer is followed by the softmaxLayer which is followed in turn by classificationLayer. The goal of this problem to understand what exactly softmaxLayer and classificationLayer do and what sense does it make. Let us denote the input for fullyConnectedLayer by y. The fully connected layer does a transformation of the form wiy + b1 z = Wy+b= : (1) wy+be that maps y to R. Next, softmaxLayer applies the soft-max function to : Dj=es SoftMax(2) = (2) Ees Finally, classificationLayer assigns class according to the largest entry of SoftMax(2). Note that the entries of Soft Max(z) all lie in the interval (0, 1) and sum up to 1. They can be interpreted as probabilities to belong to each class. Show that the entry Zk indeed approximates the posterior probability Plwa), k = 1,...,c in the case if the output of the hidden layers y belongs to the family of exponential distributions of the form p(y|wx) = gke4ly (3) 1 where ak is a vector and gk is a number that depend on wk but not on y. Note that reluLayer sets all negative components to zero if y is negative, and maxPooling2dLayer merely subsamples the output of reluLayer. Hence all components of y are nonneg- ative. Therefore, (3) is a probability distribution provided that all components of ak are negative, and gk is the normalization constant, the product of the absolute values of the entries of ak. Proceed as follows. (a) Given pylwk), k = 1,..., C, use Bayes's theorem to write the posterior proba- bility P( wy). (b) Equate the kth entry of Soft Max(z) with P(wkly). What should be wk and be for this equality to hold for all k