Answered step by step
Verified Expert Solution
Question
1 Approved Answer
4. In last assignment, we had assumed the multivariate normal model for Y, (Y|X, W) ~ N(XW, 0;I), where Y = [41, 42, ..., YM]
Step by Step Solution
There are 3 Steps involved in it
Step: 1
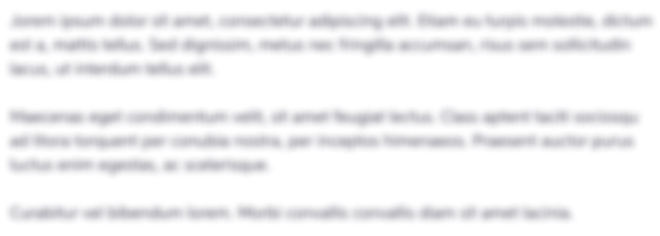
Get Instant Access to Expert-Tailored Solutions
See step-by-step solutions with expert insights and AI powered tools for academic success
Step: 2

Step: 3

Ace Your Homework with AI
Get the answers you need in no time with our AI-driven, step-by-step assistance
Get Started