Answered step by step
Verified Expert Solution
Question
1 Approved Answer
4/26/2016 Welcome to Lean Six Sigma Black Belt Virtual Class 9: Tuesday, April 26, 2016 Design of Experiments: Part 1 We are entering Week 9.
4/26/2016 Welcome to Lean Six Sigma Black Belt Virtual Class 9: Tuesday, April 26, 2016 Design of Experiments: Part 1 We are entering Week 9. It is suggested (not required) that during Week 9 the following assignments could (depending on the project) be submitted for grading: Pareto, Histogram, SIPOC Expected Variation t-Test and Mystery Tool ANOVA Chi-square Note that not all projects have the same assignments. Of course, you should submit any assignments that you have completed. 2 VERY IMPORTANT DATES! We are half-way through the Course! All project assignments must be 100% correct and complete by midnight on June 17, 2016. All on-line assessment tests must be completed with a score of at least 70% by the end of class at 11:59 PM ET on June 20, 2016. The project files have all been posted on the Discussion Board (Main Classroom). If you have not already done so, be sure to watch the recordings of the week 1 and 2 virtual classes BEFORE you begin submitting project assignments. 1 4/26/2016 3 Tonight's Topics Some Review Chi-square test of independence Introduction to Designed Experiments Fundamental ideas Basic approach DoE Example Full factorial design Analysis (without ANOVA) Interpretation 4 Review: Chi-square test of independence Example: A company wants to determine whether there is any relationship between three proposed work schedules and four different plants. The company takes a random sample of 216 employees and records the number of people who favor each proposed work schedule and their place of employment (that is, Plant 1, 2, 3, or 4). We will use the Chi-square test of independence at an alpha level of 0.05. (adapted from Statistical Methods and Data Analysis by Ott and Longnecker) Ho: Row and Column Variables are independent. That is, \"which work schedule is favored is independent of the plant employed.\" Ha: The Row and Column Variables are dependent (associated). 2 4/26/2016 5 Review: Chi-square test of independence The expected frequencies are found as follows: E ij where: E11 (R i )(C j ) n Ri is the total for Row I and Cj is the total for Column J Row 1Total Column 1 Total 7024 7.78 Overall Total 216 So, the \"observed frequency\" for Work Schedule 1 and Plant 1 is 15 and the \"expected frequency\" is 7.78. We compute the \"expected frequencies\" for each cell. 6 Review: Chi-square test of independence The Chi Square test statistic is found by the following formula: where: O = the observed value of the number in row i and column j E = the expected value of the number in row i and column j Summation = do this for every cell in the table 2 15 7.782 7.78 ... 22 14.17 2 Next, we compare the test statistic (computed value) to the critical value (theoretical value from the Chi-square table). Recall the degrees of freedom for the Chi-square critical value is the number of rows minus one times the number of columns minus 1 (R - 1)(C - 1); df = 6 in this case. Since 27.13 > 12.6, we reject Ho and conclude Work Schedule and Plant are dependent. 14.17 27.13 See page 180 in the BB Text 3 4/26/2016 7 Introduction to Designed Experiments Designed experiments embodies the basic concepts of the scientific method. Questions Note that the scientific method is an iterative process - Knowledge is built upon previous results through the use of experimentation. Hypothesis Deming calls this \"profound knowledge\" which includes: Observation Theory of variation Test Systems theory Psychology Hypothesis accepted, rejected, or modified Epistemology 8 Introduction to Designed Experiments A designed experiment is: a test or series of tests in which purposeful changes are made to the input variables of a process or system so that the experimenter may observe and identify the magnitude of and reasons for changes in the output response. Source: Design and Analysis of Experiments by Douglas Montgomery (Wiley, 2012). \"The processes we have created today as a result of our thinking thus far have problems which cannot be solved by thinking the way we thought when we created them.\" Albert Einstein 4 4/26/2016 9 Introduction to Designed Experiments So, why not just use the old \"one-factor at a time\" approach? It may take numerous \"best guesses\" before an acceptable result is obtained, and by this point the experiment may actually exceed the size and cost of a more formal one. Even when an acceptable solution is found, the experimenter has no guarantee that it is the optimal one without further testing. There is no ability to test for interaction. 10 Introduction to Designed Experiments Efficient experiments require: A solid prior knowledge of the product/process being studied. Clearly defined goals and objectives for the experiment. A statistically and practically valid experimental design. Useful experimental data. Effective data analysis. Action plans for implementation. 5 4/26/2016 11 Introduction to Designed Experiments State the problem clearly - in detail and in writing! Step 1 Can the problem be stated simpler? Does restating the problem change anything? Is the stated problem a small symptom of an even larger problem? Is this really the problem you should be working one 12 Introduction to Designed Experiments Set clearly defined goals for the experiment. Step 2 Is it for optimization or screening? What will occur if the problem is solved? How will you know when it is solved? What will be the probable cost in time, money, and energy in solving the problem? Is it worth this? Should the problem be subdivided into smaller parts or combined with other similar problems? 6 4/26/2016 13 Introduction to Designed Experiments Use a valid experimental design. Step 3 The experiment may be simple, one parameter testing, or it may require a complex designed experiment. Either way, think of several ways your decision can be checked out to ensure that it will do what you hope. Should it be discussed with others? Can it be tried on a sample basis? What reaction of results from a test will you accept as a "go/no go" signal? 14 Introduction to Designed Experiments Determine how large a difference in the conditions being compared will be considered to be significant from an engineering point of view. In other words, how small or large a difference do you want the experiment to detect? This will affect your sample size. Consider the risks to both the experiment's results and to your ability to interpret them: Step 3 (continued) - - What are the implications of a failure to detect a significant difference? ( Risk) What are the consequences of incorrectly concluding that a difference is significant when it is not? ( Risk) 7 4/26/2016 15 Introduction to Designed Experiments Step 3 Ensure that the response variable is quantitative (numerical) and meaningful in relation to the process under investigation. The underlying principle behind a designed experiment is to maximize the effect of the tested factors and minimize the effect of the error variance, so it is critical that the responses be measured accurately and precisely. (continued) 16 Introduction to Designed Experiments Step 3 (continued) Keep in mind the potential effects of "synergy" - the end result of combining two or more variables may be greater than the sum of the results of each of the variables when taken alone. Do you have any knowledge about possible interactions of the factors? Do you wish to test for these interactions? 8 4/26/2016 17 Introduction to Designed Experiments Collect useful experimental data. Step 4 Use the simplest possible design. Do not make the experiment overly complicated by trying to test too much all at once. Some of the best experimental results are found with iterative experiments in which a series of smaller, simpler experiments are used to "zero in" on the best problem solution. Initialize the process and verify initialization. Take precautions to preserve the necessary identification of the sample units. 18 Introduction to Designed Experiments Ensure that parts are not \"selected\" for the experiment all parts which are produced during the normal operation of the experimental process are used unless the failure mechanism is definitely unrelated to the experiment (such as power failure, machine breakdown, etc.). Randomize the order of the experiments so that the effect of uncontrolled variation is distributed across the experimental trials and the validity of the estimates is improved. Step 4 (continued) 9 4/26/2016 19 Introduction to Designed Experiments Perform effective data analysis. Keep the following in mind as you analyze the data: Step 5 Not all results that are judged \"statistically significant\" are of \"practical\" significance. The smaller the difference you are trying to detect, the larger the sample size needed and the more reliable the measurement system has to be. 20 Introduction to Designed Experiments HYPOTHESIS: An assertion or belief about the effect of a particular treatment on the process under study. A test of the hypothesis is therefore a test of the validity of this assertion, and is conducted by analyzing the sample data obtained during the experiment. EXPERIMENT: A test or series of tests in which purposeful changes are made to the input (independent variables / factors) of a process or system so that the reasons for changes in the output (response / dependent variables) may be observed and evaluated. DESIGN OF THE EXPERIMENT: The plan for conducting the experiment (factors tested, settings at which they are tested, number of units tested, analysis method, etc.) Basic DoE terminology 10 4/26/2016 21 Introduction to Designed Experiments Basic DoE terminology RESPONSE (OR DEPENDENT) VARIABLE: The output which is being measured / evaluated to determine the effect of the experimental manipulation; the output characteristic of interest. EXPERIMENTAL UNIT: The object upon which the response variable is measured; the sample item. FACTORS (OR INDEPENDENT) VARIABLES: The process conditions/parameters theorized to affect the response variable and which are changed during the experiment to determine the resulting impact on the response variable. LEVELS: The values or intensity settings of the factors / independent variables in the experiment. 22 Introduction to Designed Experiments Basic DoE terminology TREATMENT / TREATMENT COMBINATION: A particular combination of the levels of the factors in the experiment. REPETITION: The number of experimental units tested under each experimental run; also known as sample size. REPLICATION: A completely new run (including new set-up) of a particular treatment combination. MAIN EFFECTS: When there is an optimum level / setting for a tested factor regardless of the levels at which other tested factors are set. INTERACTION: When the optimum level / setting for a tested factor depends upon the level at which other factors are set. 11 4/26/2016 23 Full Factorial Example: Reducing Springs with Cracks with a 23 Full Factorial Quenching Bath Factors Considered: Oil Temperature Steel Temperature Carbon Content Oil Response Variable % of Springs without cracks This is an example that Dr. George Box used often. See Designing Industrial Experiments by Box, Bisgaard, and Fung, BBBF Books, 1990. Also, one of the most important books is Statistics for Experimenters by Box, Hunter, and Hunter, McGraw-Hill. 24 Full Factorial Example: Reducing Springs with Cracks with a 23 Full Factorial Trial % w/o Cracks Steel Temp Carbon Content Oil Temp 1 67 - - - 2 79 + - - 3 61 - + - 4 75 + + - 5 59 - - + 6 90 + - + 7 52 - + + 8 87 + + + 12 4/26/2016 25 Full Factorial Example: Reducing Springs with Cracks with a 23 Full Factorial Trial Res T:Steel Temp C:Carbon Content O:Oil Temp TC TO CO TCO 1 2 3 4 5 6 7 8 67 79 61 75 59 90 52 87 -1 1 -1 1 -1 1 -1 1 -1 -1 1 1 -1 -1 1 1 -1 -1 -1 -1 1 1 1 1 1 -1 -1 1 1 -1 -1 1 1 -1 1 -1 -1 1 -1 1 1 1 -1 -1 -1 -1 1 1 -1 1 1 -1 1 -1 -1 1 23 -5 1.5 1.5 10 0 0.5 Effects Use the SUMPRODUCT Excel Function (and averaging) to generate the effects 26 Full Factorial Example: Reducing Springs with Cracks with a 23 Full Factorial 13 4/26/2016 27 Full Factorial Example: Reducing Springs with Cracks with a 23 Full Factorial 28 VERY IMPORTANT DATES! All project assignments must be 100% correct and complete by midnight on June 17, 2016. All on-line assessment tests must be completed with a score of at least 70% by the end of class at 11:59 PM ET on June 20, 2016. 14 4/26/2016 Goodnight everyone. Next week we conclude our look at Designed Experiments. Next Week 15 5/3/2016 Welcome to Lean Six Sigma Black Belt Virtual Class 10: Tuesday, May 3, 2016 Design of Experiments: Part 2 We are entering Week 10. It is suggested (not required) that during Week 10 the following assignments could (depending on the project) be submitted for grading: Expected Variation t-Test and Mystery Tool ANOVA Chi-square DoE Note that not all projects have the same assignments. Of course, you should submit any assignments that you have completed. 2 VERY IMPORTANT DATES! We are half-way through the Course! All project assignments must be 100% correct and complete by midnight on June 17, 2016. All on-line assessment tests must be completed with a score of at least 70% by the end of class at 11:59 PM ET on June 20, 2016. The project files have all been posted on the Discussion Board (Main Classroom). If you have not already done so, be sure to watch the recordings of the week 1 and 2 virtual classes BEFORE you begin submitting project assignments. 1 5/3/2016 3 Tonight's Topics Some Review 23 Full Factorial Design More on Designed Experiments Replication Use of Center Points Introduction to Fractional Factorial Designs Basic ideas Example Interpretation 4 Review: 23 Full Factorial DoE Example: A process improvement team has been at work on improving the reliability of an electronic controller. The team selects three factors (each at two levels) and replicates the experiment three times (recording the average of the time to failure for each trial). See your Black Belt Online Text (p. 269) for more on this example. With three factors each at two levels, it takes 8 trials to investigate all effects. 2 5/3/2016 5 Review: 23 Full Factorial DoE Using the average response for each trial, we compute the average impact for each factor at the low and high levels and the take the difference. This is the \"effect\" value (effect on the response as a result of going from one level to the next). 6 Review: 23 Full Factorial DoE A normal probability plot of the effects shows that the factor A (Conductor Material) and the interaction of A and C (Conductor Material and Cooling Method appear to be significant) 3 5/3/2016 7 Review: 23 Full Factorial DoE Since factor A (Conductor Material) is involved in an interaction, we need to analyze the interaction with factor C (Cooling Method) in order to ascertain the optimum levels. The highest average time to failure occurs when factor A is at the high level (Material B) and factor C is at its high level (New). Factor B (Case Design) did not have a significant impact on the response variable (average time to failure). 8 Review: 23 Full Factorial DoE Since the experiment was replicated, the standard analysis would be ANOVA. The ANOVA confirms that factor and A and the interaction of factor A and C is significant, and using alpha of 0.05, it identifies the interaction of A and B as significant. ANOVA is the preferred method for analysis when appropriate. 4 5/3/2016 9 The Role of Replication Recall that \"replication\" is the completely new run of the experimental conditions (including set-ups, etc.) Replicating an experiment will increase the likelihood that we identify significant effects. In an experiment to determine how to make the best tasting brownies, the factors were: Factors Levels Type of Pan: Aluminum vs. Glass Stirring Method: Spoon vs. Electric Mixer Brand of Mix: Cheap vs. Expensive The response variable was \"Taste\" with higher scores being better. 10 The Role of Replication According to the results of the ANOVA, the only effect that is significant at the 0.05 level is the interaction of Pan Type and Stirring Method. 5 5/3/2016 11 The Role of Replication Note the Total Degrees of Freedom here is 23. This comes from the \"total number of experiments run - 1\" (24 - 1). 12 The Role of Replication If we had not replicated we would only have 7 total degrees of freedom (8 - 1). We would therefore not be able to test for every effect because there are 7 effects and each requires 1 degree of freedom to test it. That would leave 0 degrees of freedom for the error term, which is not allowed. Without replication, we miss the significant interaction effect. 6 5/3/2016 13 The Role of Replication When you don't replicate, your Beta risk (committed when we accept the null hypothesis when it is in fact false) is increased for several reasons: The extra variation from the terms now included in the error term inflates the Sums of Squares for the error term (increases the \"noise" measure for the experiment). The error degrees of freedom is now smaller, which makes the Mean Square for the error term larger. (Mean Square = Sums of Squares / df) The overall F ratio for each term is therefore smaller since you are dividing by a larger number (the Mean Square for Error). 14 Center Points: checking linearity Center points are experiments run at the midpoint of all the factor levels (the \"0\" level). They therefore typically only have meaning with quantitative factors that can be set at the exact midpoint. They are used to detect the presence of curvature in the model without the expense of a 3 level design. If the nonlinearity effect is significant, the linear plots for the main effects are suspect. The significance of the main effects is therefore not reliable and interpolation of the linear model is not advised. Center points require additional experimental runs and therefore also increase the degrees of freedom and the estimate of the experimental error. Every experiment has only one set of center points. At least 4 center point replicates are recommended to have a moderate ability to detect nonlinearity. SOURCE: An Introduction to Design of Experiments by Larry S. Barrentine. 7 5/3/2016 15 Center Points: checking linearity Example: A two factor experiment with Temperature set at 350 and 400 degrees, Pressure set at 50 and 70 psi, and 4 center point replications. The response variable is thickness. The question: is the response linear between the two levels, or is there curvature? 375 60 Response, y - Factor + 16 Center Points: checking linearity We can use the tradition ANOVA method to help us detect the presence of curvature. The results show that curvature is significant, so either Temperature or Pressure (or both) has a nonlinear effect. 8 5/3/2016 17 Center Points: checking linearity What do you do if curvature is significant? The test for nonlinearity does NOT tell which factors contain the curvature, only that it exists with at least 1 factor. Attempt to determine which factors are likely to have a curvilinear relationship with the response variable through research, etc. Otherwise significant nonlinearity must be investigated by further experimentation at three levels. 18 Advantages/Disadvantages of Full Factorial Designs Advantages relatively straightforward to design and use allow for the investigation of all main effects and all interactions Disadvantages can become very large quickly; for 2 5 32 trials 3 81 trials 4 5 factors, 4 factors, example 2 levels 3 levels 9 5/3/2016 19 Introduction to Fractional Factorials An alternative to Full Factorial designs is a class of experiments known as Fractional Factorial designs. Fractional Factorial Designs An experimental design in which a specific (and smaller) portion of the Full Factorial is tested. Note that since not all trials are run, some information cannot be obtained. 20 Introduction to Fractional Factorials Level of Knowledge HIGH MODERATE LOW Recommendation Employ full factorials to fully investigate factors and interactions; optimize. Employ fractional factorials to assess factors and some interactions. Employ fractional factorials to screen out unimportant factors and to provide a foundation for further experimentation. See Improving Quality Through Planned Experimentation by Moen, Nolan, and Provost, McGraw-Hill, 2012. Great book. 10 5/3/2016 21 Introduction to Fractional Factorials Consider an experiment in which there are 3 factors, each at 2 levels. In a Full Factorial design, this would be designated as a 23 design, and would require 8 different experimental treatments. Assuming, however, that the experimenter is only able to run 4 experiments, this would become a Fractional Factorial design designated as: 23-1= 4 treatment combinations The base is still the number of levels for the factors -- in this example, 2. The exponent is now written as the result of the original number of factors -- in this example, 3 -- less whatever number is necessary to achieve the new experiment size - which in this example is 4. Since 22 = 4, the exponent is written as "3-1". Since the Fractional Factorial size, 4, is half of the Full Factorial size, 8, this is also known as a "one-half 23 design". 22 Introduction to Fractional Factorials Recall that fractional factorial designs sacrifice some effect information since less than the full number of treatment combinations is utilized. Confounding is the name given to this loss of information. A confounded design is one in which some effects are measured by the same experimental results as others. The effects of factors or interactions confounded together are then indistinguishable from each other. 11 5/3/2016 23 Introduction to Fractional Factorials Alias Sets for a 24-1 Fractional Factorial Design Using D=ABC as the Design Generator A = BCD B = ACD C = ABD D = ABC AB = CD AC = BD BC = AD ABCD = 1 24 Introduction to Fractional Factorials Design resolution captures the amount of confounding that takes place in a DoE. Resolution II Designs Main effects are confounded with each other. [A = B] Resolution III Designs Main effects are not confounded with each other, but twofactor interactions can be. [A = AB; BC = AD] Resolution IV Designs Main effects are not confounded with each other or with two-factor interactions, but two-factor interactions may be confounded with each other. [A = ACD; AC = BD] Resolution V Designs No main effect or two-factor interaction is confounded with any other main effect or two-factor interaction. [A = ACD; AC = BCD] 12 5/3/2016 25 Introduction to Fractional Factorials Example of a Fractional Factorial design: a 24-1 Note that 4 factors are examined in only 8 trials. AC BC ABC= D 1 1 1 -1 -1 -1 1 1 -1 1 -1 1 -1 1 -1 -1 -1 -1 1 1 -1 -1 1 1 -1 1 -1 1 -1 -1 7 -1 1 1 -1 -1 1 -1 8 1 1 1 1 1 1 1 A B C 1 -1 -1 -1 2 1 -1 -1 3 -1 1 -1 4 1 1 5 -1 6 AB The pattern of this design matrix is usually called the experiment in standard order. 26 Fractional Factorial Example A B C D (-) Level (+) Level Response: Force 90 min 120 min required to separate. copper nickel See Designing for Quality by D2A H1E R. H. Lochner and J. E. Matar tin silver Factors Cure time Conductor material Adhesive type IC post coating Trial # A 1 2 3 4 5 6 7 8 + + + + - great book! FACTORS B C + + + + + + + + D 1 + + + + 73.0 87.7 80.5 79.8 85.2 78.0 78.4 90.2 REPLICATES 2 3 4 73.2 86.4 81.4 77.8 85.0 75.5 72.8 87.4 72.8 86.9 82.6 81.3 80.4 83.1 80.5 92.9 72.2 87.9 81.3 79.8 85.2 81.2 78.4 90.0 5 RESPONSE Average 76.2 86.4 82.1 78.2 83.6 79.9 67.9 91.1 73.5 87.1 81.6 79.4 83.9 79.5 75.6 90.3 13 5/3/2016 27 Fractional Factorial Example Output from Minitab Source DF A (Cure Time) B (Conductor Material) C (Adhesive Type) D (IC Post Coating) A*B A*C A*D Error 1 1 1 1 1 1 1 32 SS 295.94 5.33 38.42 758.64 6.72 0.63 2.70 196.24 MS 295.94 5.33 38.42 758.64 6.72 0.63 2.70 6.13 F P 48.26 0.87 6.26 123.71 1.10 0.10 0.44 0.0000 0.3580 0.0180 0.0000 0.3030 0.7520 0.5110 28 VERY IMPORTANT DATES! All project assignments must be 100% correct and complete by midnight on June 17, 2016. All on-line assessment tests must be completed with a score of at least 70% by the end of class at 11:59 PM ET on June 20, 2016. 14 5/3/2016 Goodnight everyone. Next week is a quick review of Statistical Process Control. Next Week 15
Step by Step Solution
There are 3 Steps involved in it
Step: 1
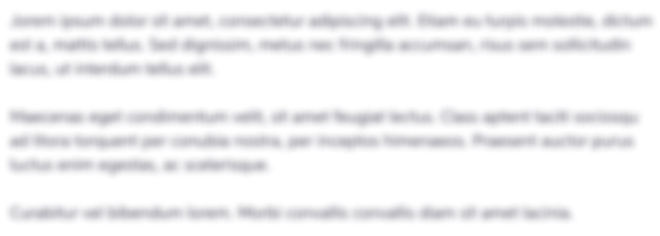
Get Instant Access to Expert-Tailored Solutions
See step-by-step solutions with expert insights and AI powered tools for academic success
Step: 2

Step: 3

Ace Your Homework with AI
Get the answers you need in no time with our AI-driven, step-by-step assistance
Get Started