Answered step by step
Verified Expert Solution
Question
00
1 Approved Answer
6. Kernel Functions (50 pts, page 8 & 9) (a) [10 pts, page 8] If k1(x, ) and k2(x, z) are valid kernels and a,
Step by Step Solution
There are 3 Steps involved in it
Step: 1
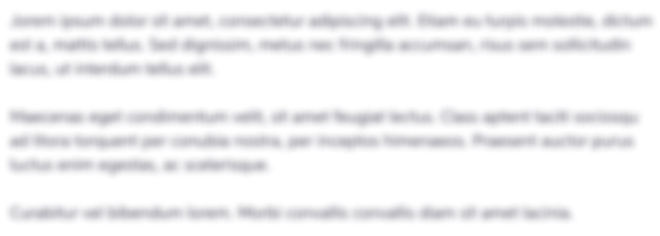
Get Instant Access with AI-Powered Solutions
See step-by-step solutions with expert insights and AI powered tools for academic success
Step: 2

Step: 3

Ace Your Homework with AI
Get the answers you need in no time with our AI-driven, step-by-step assistance
Get Started