Question
A correlation study is a type of research method in which a researcher measures two variables to understand and assess the statistical relationship between them.
A correlation study is a type of research method in which a researcher measures two variables to understand and assess the statistical relationship between them. If a linear relationship exists, then linear models can be used to make predictions. Choose one topic from the table of topics. Options for Data Collection: Ask People: Design a survey and ask at least 30 different people to fill out the survey. You can do this in-person, Facebook, Survey Monkey, Google Forms, send via email, etc. Collect Data from Objects: Collect data for objects or observed units (not a person). Find Existing Data: Find the information/data on the Internet. Sources such as the Bureau of Labor Statistics, US Census, sports websites, etc., provide sources of quantitative information. Options for Topic (If you would like to use a different topic, instructor approval is required): Data Collection Option Topics Ask People (at least 30) GPA and Study hours Age and # of tattoos (or # of times eating fast food/week or # of credit cards, ...) Collect Data from Objects (at least 30) Alcohol content and price Fat content and calories for a type of food Find Existing Data (at least 30) Free throws and salary of NBA players Weight of a car and miles per gallon Part I: Introduction: The introduction to your report should include the following: Which topic did you choose and why? Discuss the linear relationship you will be investigating. Clearly explain which variable is the explanatory variable and which variable is the response variable. Part II: Data Collection: Were there any limitations or challenges with data collection? Describe the population from which your sample is drawn. Discuss possible sources of sample bias. Explain. Lastly, include your raw data set at the end of your report. Part III: Descriptive Statistics: Explanatory variable: Include descriptive statistics for your x-variable (explanatory variable). Include the mean, median, mode, range, standard deviation. Provide a histogram, boxplot, or stem-and-leaf for your explanatory variable. Make sure you label and title your graph. Summarize the shape of the graph. Do there appear to be any outliers? Explain. Response variable: Include descriptive statistics for your y-variable (response variable). Include the mean, median, mode, range, standard deviation. Provide a histogram, boxplot, or stem-and-leaf for your response variable. Make sure you label and title your graph. Summarize the shape of the graph. Do there appear to be any outliers? Explain. Include the summary statistics and graphs from StatCrunch in your report with explanation. Part IV: Analysis: Use StatCrunch to complete correlation and regression analysis using your explanatory variable and response variable. Summarize your analysis in your report. The report should include the following: A scatter plot showing the relationship between the explanatory variable and the response variable. Does there appear to be a linear relationship? Explain. Calculate and report the correlation coefficient, r. Does a significant linear relationship exist? Use the table of critical values to make this determination (Table II Appendix A in the text). If a linear correlation exists, is it positive or negative? Explain what this means in the context of your topic. Identify and report the least-squares regression equation. State the slope and explain what the slope means in the context of your topic. Use the regression analysis to predict the value of the response variable by choosing a value for the explanatory variable within the scope of your topic. Report and interpret the coefficient of determination (R2). Part V: Final Conclusion: Discuss whether your linear model is "good". Would you recommend using the linear model you found in part IV? Support your recommendation using your results from part IV. Discuss confounding in observational studies. One cause of confounding in a study is the presence of lurking variables. Discuss any potential lurking variables that could contribute to confounding in your study. Were you surprised by the results of your project or did your analysis verify what you suspected? Why or why not? Make any recommendations for further studies on this topic. Discuss what you have learned by doing this project. What would you do differently the next time you do research? Explain.
Step by Step Solution
There are 3 Steps involved in it
Step: 1
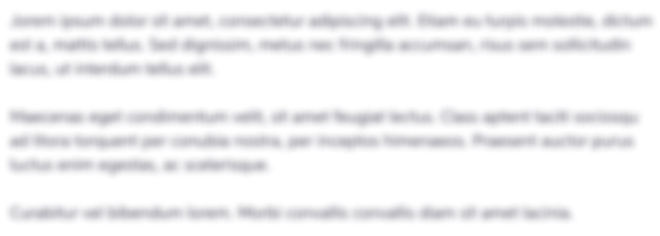
Get Instant Access to Expert-Tailored Solutions
See step-by-step solutions with expert insights and AI powered tools for academic success
Step: 2

Step: 3

Ace Your Homework with AI
Get the answers you need in no time with our AI-driven, step-by-step assistance
Get Started