Answered step by step
Verified Expert Solution
Question
1 Approved Answer
A Crash Course in Statistics - The t-Test So you took Stats I and Stats II and passed. But do you remember what you did,
A Crash Course in Statistics - The t-Test So you took Stats I and Stats II and passed. But do you remember what you did, how you did it, and why you did it? If you need some basic statistic reminders for the t-Test, then this is the lecture for you! I am going to talk about a t-Test example in this document that corresponds to the example you will see later in Chapter 9 and 10 of your Smith and Davis textbook. The example looks at how quickly a salesperson approaches a potential customer who is dressed in either sloppy or dressy clothes. Although I encourage you to read that chapter to fully understand the t-Test, this mini-lecture will sum up the basics for you a lot earlier in the semester. On the final page are several questions based on this crash course. Answer these questions, and then go into your \"Crash Course in Statistics - The t-Test Quiz #2\" in your blackboard assessments menu and copy over your answer. Each Crash Course Quiz counts 10 points. How, when, and why do a t-Test? Before we get to the example, let me give you some basic information about the t-Test. The tTest is used to compare two means to see if they differ significantly from one another. In this analysis, we need two pieces of information: the means for each group and the t-Test information itself. Do you recall what a mean is? It is the average score. That is, you add up all of the scores and divide by the number of total scores to arrive at the average. Since a t-Test looks at two different conditions, this means you have two means: one for each condition. The means here (and the standard deviation, which we will talk about in Chapter 9) are descriptive statistics. That is, they help describe the data. The t-Test information itself is a test of inferential statistics. That is, we infer significant differences between the two groups. When writing it out, you will see a very common layout for the t-Test, something like: t(14) = 2.61, p = .021. The t tells you this is a t-Test. The 14 tells us our degrees of freedom (more on that in Chapter 9). The 2.61 is the actual number for the t-Test. The p indicates whether it is significant (if it is less than .05, then it is significant). We run a t-Test only under certain conditions. First, our dependent variable (the variable we measure) must be continuous / scaled. That is, the DV has to be along a scale. For example, it can be an attitude (\"On a scale of 1 to 9, how angry are you?\") or a time frame (\"How quickly did the salesperson help the customer on a scale of zero seconds to a thousand seconds?\"). We call these interval or ratio scales. We CANNOT run a t-Test on categorical data. That is, if we have a yes / no question (\"Are you lonely: Yes or No\") or a category based question (\"What is your favorite food: hamburgers, pizza, salad, or tacos?\"), then we cannot run a t-Test. These latter questions are based more on choice of option rather than an actual rating scale, and thus we cannot use a t-Test on them. Second, we run a t-Test when we have two conditions to compare. That is, we compare the mean from Condition A to the mean from Condition B. If our t-Test is significant (p < .05), then we simply see which mean is higher. \"The t-Test was significant, t(14) = 2.61, p = .021, with Condition A (mean = 48.38) less than Condition B (mean = 63.25.\" Let's see how this looks using the example from your textbook (Chapter 9). Textbook Example - Approaching a customer dressed in sloppy versus dressy clothes Here is the basic set-up for our customer clothing study. We randomly assign salespeople to one of our two conditions. They see either a customer who is dressed-up (dressy condition) or a customer who is sloppily dressed (sloppy condition). We measure how quickly it takes the salesperson to approach each customer in seconds. Our independent variable here is the type of clothing (dressy versus sloppy) while our dependent variable is approach time (in seconds). We can predict that salespeople will approach customers who are dressed in dressy clothes faster than customers dressed in sloppy clothes. Our sample is eight participants in the dressy condition and eight participants in the sloppy condition, for a total N of fourteen. Consider the data (you can find this on page 198 in your textbook). Condition A (Dressy Clothes) 37 38 44 47 49 49 54 69 A = 387 M = 48.38 Condition B (Sloppy Clothes) 50 46 62 52 74 69 77 76 B = 506 M = 63.25 , or the symbol for Sigma, means \"the sum of\". Thus A is the sum of the scores for Condition A. That is, 37 + 38 + 44 + 47 + 49 + 49 + 54 + 69 = 387. There are eight scores here, so we divide 387 / 8 = 48.38, giving us our mean for Condition A. We do the same thing for Condition B, giving us a mean of 63.25 (506 / 8 = 63.25). For the first part of our analysis, we compare the means. As you see, 63.25 seconds in Condition B seems a lot slower than 48.38 seconds in condition A. It looks like salespeople respond faster to a dressy customer than a sloppy customer. Thus the means for Condition A and Condition B support our prediction, but we are not done yet. Just because the means seem to differ doesn't mean they do differ. To make that assessment, we run the t-Test and look at the p value to see if it is p < .05. We can do this by hand (like you did in Stats I and Stats II) or we can take the easy road and let SPSS calculate it for us. I am going to take the easy road, but keep in mind that we still have to interpret what SPSS tells us. For the next section, I am going to open SPSS and run an independent samples t-Test. I'll use screenshots from SPSS as I go, but feel free to run these analyses yourself. Just set up your SPSS file like mine (I also included this SPSS file for you in blackboard if you prefer to use that, but it is a short data set so I recommend setting up your own SPSS file). I am just going to give you the basics here, but you can refer to other sources to figure out some of the info we get from the t-Test not covered in this lecture (like Levene's test, normality testing, etc.). SPSS - Our Clothing Study 1 Click Analyze > Compare Means > Independent-Samples T Test... on the top menu as shown below. You will be presented with the following: 2 Put the "Clothes (1 Dressy, 2 Sloppy)" variable into the "Test Variable(s):" box and the "Time to help (in seconds)" variable into the "Grouping Variable:" box by highlighting the relevant variables and pressing the buttons. Note that SPSS uses different names for variables. It calls the dependent variable the \"Test Variable(s)\" and it calls the independent variable the \"Grouping Variable\". Just remember that our DV (the test variable) must be scaled in order to run this test (1 to 9, or 1 to 5, or even 0 to 100,000). The IV has to be categorical (dressy versus sloppy, men versus women, old versus young, republican versus democrat, high versus low, etc.). 3 You then need to define the groups (Clothes). Press the button. You will be presented with the following screen: 4 Enter "1" into the "Group 1:" box and enter "2" into the "Group 2:" box. Want to know why we do this? Well, remember that we labelled the Dressy group as "1" and the Sloppy group as "2"? That's why we use 1 and 2. NOTE: If you have more than 2 groups (e.g. a casual clothing (\"3\") group, a no clothing (\"4\") group, a clown clothing (\"5\") group, etc., you could type in "1" to "Group 1:" box and "3" to "Group 2:" box to compare the dressy clothes condition with casual clothes condition. You could also compare the no clothes condition (4) to the clown clothes condition (5) for a very interesting study! Since we only have two groups here, we will stick with 1 and 2 5 Press the button Output of the Independent t-Test in SPSS You will be presented with two tables containing all the data generated by the Independent t-test procedure in SPSS. Group Statistics Table (Or Descriptive Statistics) This table provides useful descriptive statistics for the two groups that you compared including the mean and standard deviation. Remember, these \"describe\" the data for us. As you can see, we have 8 participants in the Dressy condition and 8 participants in the Sloppy condition. The mean for the Dressy condition is 48.38 seconds (SD = 10.11) and the mean for the Sloppy condition is 63.25 seconds (SD = 12.54). We can ignore the Std. Error Mean for now, but we will need the rest of the information in our write up (below), so we'll come back to this table. Independent Samples Test Table This table provides the independent t-test results and Levene's Test for Equality of Variances. We will talk about Levene's test later in Chapter 10, but I want to ignore it for now. Let me make the output a bit larger so we can interpret it properly. I'll remove several unneeded columns. Above is the important information we will need for our t-Test (outlined in the red box - you can match the t-Test info in the red box with the t-Test write up in red text below!). We will use the equal variances assumed row (it doesn't differ much from equal variances not assumed anyway, but I prefer to use the df that has a whole number). What we do now is write it up. For the write up, we are going to need both of our tables - the descriptive table (with our means and standard deviations) as well as our independent samples t-Test table. Let me repost the descriptives table below (the \"group statistics\"). The important info is in the green box. Reporting the output of the independent t-Test We report the statistics in this format: t(degrees of freedom[df]) = t-value, p = significance level. In our case this would be: t(14) = 2.61, p = 0.021, and our means/SDs would be (M = 48.38, SD = 10.11) for the Dressy condition and (M = 63.25, SD = 12.54) for the Sloppy condition. We would report the results of the study as follows: We ran an independent samples t-Test with clothing condition as our independent variable and time to help the customer as our dependent variable. The t-Test was significant, t(14) = 2.61, p = .021. Salespeople approached customers dressed in dressy clothes (M = 48.38, SD = 10.11) significantly faster than customers dressed in sloppy clothes (M = 63.25, SD = 12.54). That's it! Not too hard, right? Just remember the basics here: we use a t-Test to look at the differences between two means to see if the means differ significantly. We need two SPSS tables to make this assessment: the descriptive statistics tables (\"group statistics\") and the t-Test table (\"Independent Samples t-Test\"). Crash Course In Statistics - The t-Test - Quiz #2 Instructions: Imagine you randomly assign participants to complete either a hard anagram solving task (\"Please rearrange the letters CINERAMA to form a new word\". This, of course, is difficult but not impossible, as it can be rearranged to AMERICAN) versus an easy anagram solving task (\"Please rearrange the letters BAT to form a new word\". This is very easy, as it can be rearranged to TAB). You then measure participant's levels of frustration in completing the anagram solving task on a scale ranging from 1 (very frustrating) to 9 (not at all frustrating). Using these charts, answer the questions on the final page. Transfer those answers to your Crash Course in Statistics - The t-Test Quiz #2 in Blackboard (2 points per question). 1). What is the independent variable in this study? A. Level of frustration B. Whether they can rearrange the word CINERAMA C. Whether they can rearrange the word BAT D. Which problem solving task they received (hard versus easy anagram) E. There is too little information in this study to determine the independent variable 2). What is the dependent variable in this study? A. Level of frustration B. Whether they can rearrange the word CINERAMA C. Whether they can rearrange the word BAT D. Which problem solving task they received (hard versus easy anagram) E. There is too little information in this study to determine the dependent variable You run a t-Test on this data set and get the following SPSS output. Using this output, interpret the information. 3). Choose the correct means and standard deviations for easy and hard conditions. A. The easy condition has a mean of 1.39 and standard deviation of 0.50 while the hard condition has a mean of 8.37 and a standard deviation of 1.30. B. The easy condition has a mean of 8.37 and standard deviation of .050 while the hard condition has a mean of 1.39 and a standard deviation of 1.30. C. The easy condition has a mean of 1.57 and standard deviation of 0.24 while the hard condition has a mean of 37.02 and a standard deviation of 0.09. D. The easy condition has a mean of 27.89 and standard deviation of 59 while the hard condition has a mean of 27.55 and a standard deviation of 37.02. E. The easy condition has a mean of 8.37 and standard deviation of 1.30 while the hard condition has a mean of 1.39 and a standard deviation of 0.50. 4). Is the t-Test significant, and how would you write that out in APA format? A. Yes, the t-Test is significant, t(27.89) = 59, p < .001 B. Yes, the t-Test is significant, t(59) = 27.89, p < .001 C. No, the t-Test is not significant, t(27.89) = 59, p > .001 D. No, the t-Test is not significant, t(59) = 27.89, p > .001 E. It is impossible to tell from this data set if the t-Test is significant 5). Finally, which of the following is the correct results as you would write them in an APA formatted results section. A. We ran an independent samples t-Test with anagram condition as our independent variable and frustration as our dependent variable. The t-Test was significant, t(59) = 27.89, p < .001. Participants were more frustrated in the hard anagram condition (M = 8.37, SD = 1.30) than the easy anagram condition (M = 1.39, SD = 0.50). B. We ran an independent samples t-Test with anagram condition as our independent variable and frustration as our dependent variable. The t-Test was not significant, t(59) = 27.89, p > .05. Participants were no more frustrated in the hard anagram condition (M = 1.39, SD = 0.50) than the easy anagram condition (M = 8.37, SD = 1.30). C. We ran an independent samples t-Test with anagram condition as our independent variable and frustration as our dependent variable. The t-Test was significant, t(59) = 27.89, p < .001. Participants were more frustrated in the hard anagram condition (M = 1.39, SD = 0.50) than the easy anagram condition (M = 8.37, SD = 1.30). D. We ran an independent samples t-Test with anagram condition as our independent variable and frustration as our dependent variable. The t-Test was not significant, t(27.89) = 59, p > .05. Participants were no more frustrated in the hard anagram condition (M = 1.39, SD = 0.50) than the easy anagram condition (M = 8.37, SD = 1.30). E. We ran an independent samples t-Test with anagram condition as our independent variable and frustration as our dependent variable. The t-Test was significant, t(27.89) = 59, p < .001. Participants were more frustrated in the hard anagram condition (M = 1.39, SD = 0.50) than the easy anagram condition (M = 8.37, SD = 1.30)
Step by Step Solution
There are 3 Steps involved in it
Step: 1
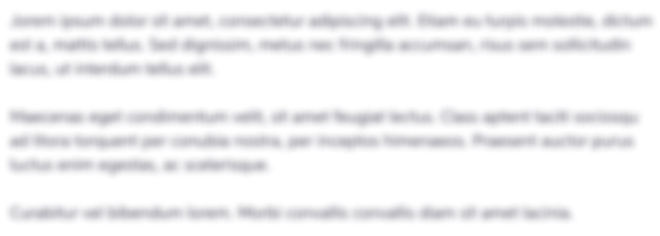
Get Instant Access to Expert-Tailored Solutions
See step-by-step solutions with expert insights and AI powered tools for academic success
Step: 2

Step: 3

Ace Your Homework with AI
Get the answers you need in no time with our AI-driven, step-by-step assistance
Get Started