Answered step by step
Verified Expert Solution
Question
1 Approved Answer
AMERICAN JOURNAL OF HUMAN BIOLOGY 14:762-768 (2002) Is Obesity Associated With Poor Sleep Quality in Adolescents? NEERAJ K. GUPTA,1 WILLIAM H. MUELLER,1* WENYAW CHAN,1 AND
AMERICAN JOURNAL OF HUMAN BIOLOGY 14:762-768 (2002) Is Obesity Associated With Poor Sleep Quality in Adolescents? NEERAJ K. GUPTA,1 WILLIAM H. MUELLER,1* WENYAW CHAN,1 AND JANET C. MEININGER2 I School of Public Health, University of Texas Health Science Center at Houston, Houston, Texas 77225 2 School of Nursing, University of Texas Health Science Center at Houston, Houston, Texas 77225 ABSTRACT Obesity has reached epidemic proportions in the U.S. and more developed countries, particularly so among adolescents. A substantial impact on public health could be achieved if other factors causing obesity besides the conventional ones of diet and physical activity could be identified and acted upon. The present study investigates whether there is a link between low sleep quality and obesity in a tri-ethnic, cross-sectional sample (n = 383) of male and female adolescents ages 11-16 years old (Heartfelt Study). Sleep quality was expressed as two variables, total sleep time and sleep disturbance time obtained by 24-hour wrist actigraphy. Percent body fat and body mass index (BMI) were used together to define obesity. The potential influence of demographic and behavioral confounders were considered in models that described the relation of sleep to obesity occurrence. Obese adolescents experienced less sleep than nonobese adolescents (P < 0.01). Foreach hourof lost sleep, the odds of obesity increased by 80%. Sleep disturbance was not directly related to obesity in the sample, but influenced physical activity level (P < 0.01). Daytime physical activity diminished by 3% for every hour increase in sleep disturbance. The above observations were independent of potential confounding variables. Inadequate and poor sleep quality in adolescents may be important factors to consider in the prevention of childhood obesity. Am. J. Hum. Biol. 14:762-768, 2002. ?? 2002 Wiley-Liss, Inc. Obesity is a worldwide health problem, reaching epidemic proportions in the U.S. and more developed countries (Ross et al., 2000), more so in children and adolescents (Troiano et al., 1995; Barth et al., 1997; Troiano and Flegal, 1998). Obesity is associated with a wide range of chronic diseases (Mokdad et al., 1999). Thus, its prevention is an urgent concern for public health. Of the social and behavioral influences on the development of obesity, physical activity and nutrition are the major research focus (Baranowski et al., 2000). However valuable this research, interventions based on it have evidently been unsuccessful in curbing obesity (Nestle and Jacobson, 2000). Other factors may be contributing to obesity in the U.S. and otherindustrial nations. One possible factoris sleep behavior. As the prevalence of obesity has risen there has been a concurrent increase in work hours and sleep debt (Bonnet and Arand, 1995; Spiegel et al., 1999; Leger, 2000). Sleep deprivation could be particularly important for adolescent health, as adolescents have greater sleep needs than prepubertal children (Carskadon, 1990; Kelman, 1999). Yet studies find that adolescents get less sleep than youngerchildren and adults (Mantz et al., 2000). School ?? 2002 Wiley-Liss, Inc. schedules, work hours, later curfew, and television watching are activities common to the lifestyle of urban teenagers that may potentially interfere with sleep needs (Wolfson and Carskadon, 1996, 1998). Sleep debt impacts three hormonal mechanisms in humans that affect metabolic and endocrine functions (Scheen and Van Cauter, 1998): 1) glucose metabolism, 2) serotonin release (Rosmond and Bjorntorp, 2000), and 3) hypocretins (Bourgin et al., 2000; Sutcliffe and de Lecea, 2000). This has lead to the hypothesis that sleep debt might contribute to chronic illness through effects on metabolism (Scheen, 1999; Spiegel et al., 1999). Serotonin (5-hydroxytryptamine) has been implicated in the processes of withinmeal satiation and postmeal satiety concerned with the signals arising from the pattern of food intake. Carbohydrate cravContract grant sponsor: The Heartfelt Study was funded by the National Institute of Nursing Research; Contract grant numbers: R55 and R01 NR03052 *Correspondence to: William H. Mueller, PhD, University of Texas Health Science Center at Houston, School of Public Health, P.O. Box 20186, Houston, TX 77225. E-mail: wmueller@ sph.uth.tmc.edu Received 14 September 2001; Accepted 27 May 2002 Published online in Wiley InterScience (www.interscience. wiley.com). DOI: 10.1002/ajhb.10093 2 N.K. GUPTA ET AL. ing, apparently driven by the need for increased serotonin levels and their attendant feelings of increased well-being, has been implicated in obesity (Wurtman and Wurtman, 1995). Normally, serotonin levels are replaced during sleep. Sleep debt and disturbed sleep may thus result in reduced natural levels of serotonin, increasing the need forexogenous sources that can stimulate serotonin release, such as carbohydrates. Sleep debt could thus be a contributor to increasing obesity in the U.S. Sleep apnea commonly occurs in the obese, which would lead to an expectation of greater sleep disturbance and lower physical activity during waking hours in obese persons (Marik, 2000). This could exacerbate further weight gain. The above suggests a potential association of sleep behaviorand obesity in adolescents. The present study investigates whether there is a link between low sleep quality, daytime physical activity, and obesity in a tri-ethnic sample of male and female adolescents 11-16 years old (Heartfelt Study). Hypotheses 1) Sleep quality (sleep duration and sleep disturbance) will not differ systematically with age, gender, and ethnicity and potential behavioral confounders in the sample. 2) Lowerdaytime physical activity will be associated with poorer sleep quality in the sample independent of potential confounders. 3) Obesity will be associated with lower sleep quality (less sleep duration and more sleep disturbance) in the sample independent of potential confounders. MATERIALS AND METHODS The Heartfelt Study examined cardiovascular reactivity and blood pressure in relation to mood in adolescents from three ethnic groups (Meininger et al., 1999). The sample of 383 adolescents, 11-16 years old, was accessed through public middle and high schools in a large, ethnically diverse city in southeast Texas. The sampling plan attempted to obtain nearly equal numbers of children by sex and ethnic group (African American, n = 140; Hispanic, n = 117; and Caucasian, n = 126). Grouped together with Caucasian are 10 children of other ethnicities (mostly Asian). Ethnicity was selfreported. Adolescents were recruited in general assemblies or required classes so groups would not be systematically excluded on the basis of enrollment in particular courses. Body measurements of Heartfelt Study children are similar in central tendency and variation to those matched for age, ethnicity, and genderfrom nationally representative samples (Cho et al., 2001). Those who volunteered to participate were required to have written parent/guardian consent. The Committee forthe Protection of Human Subjects at the University of Texas Health Science Centerat Houston approved the study. Ambulatory monitoring and characterization of sleep patterns Physical activity in the Heartfelt Study was monitored with the Motionlogger Actigraph (model 20.000; Ambulatory Monitoring, Ardsley, NY). The Motionlogger is an instrument the size of a large wristwatch that collects and stores data on subject activity as a continuous time series (Mason and Redeker, 1993). Actigraph measurements are a direct measure of change in acceleration of the device and hence the device wearer. The participant was fitted with the actigraph on the nondominant wrist and worn throughout one 24-hour period except during bathing. The actigraph was used in this study to characterize daytime physical activity and sleep behaviors. Sleep behavior was expressed as two variables: total sleep time (TST) and sleep disturbance time (SDT). Sleep behavior Actigraph methodology has been validated to monitorsleep/wake with a high degree of accuracy (Shinkoda et al., 1998; JeanLouis et al., 1999). The actigraph Action-W program assigns a sleep (=1) or wake (=0) score based on a method similar to that of Cole et al. (1992). The program computes the weighted sum of the activity in the current minute, the preceding 4 minutes and the following two minutes as follows: S = + 0.0033 * (1.06 * an4 + 0.54 * an3 + 0.38 * an2 + 0.76 * an1 + 2.3 * an0) + 0.74 * al + 0.67 * a2) where an4-an1 are the activity counts from the prior 4 minutes, an0 is the current minute, and a1 and a2 are the following 2 minutes. The current minute is scored as sleep if S > 1. The numberof minutes scored as sleep by the program during one 24hour period were totaled and converted to hours and this constituted the variable total sleep time (TST). By this method, some sleep hours would have occurred as naps in the daytime; however, 92% of the scores were recorded during nighttime (8 PM to 8 AM). Total sleep disturbance minutes were calculated by addition of actigraph readings scored 0 as awake during periods of sleep over 24 hours, including during daytime naps. However, 94% of the disturbed sleep scores would have been recorded from 8 PM to 8 AM. Each awake epoch during nighttime sleep was counted as 1 minute. These times were converted to sleep disturbance time in hours (SDT). Each actigraphy epoch was also scored automatically for bad or invalid readings. Subjects with more than 10 bad data marks were omitted from the analysis (20 subjects). Daytime physical activity (DPA) The average of the total actigraphy readings for daytime waking hours is an accurate measure of physical activity (Klosch et al., 2001). Compared to other techniques wrist actigraphy has been reported superior in terms of utility and accuracy, distinguishing vigorous (running, climbing stairs, biking) from sedentary activity (reading, watching television, playing video games) (Patterson et al., 1993). Movement is recorded on a relative scale of 0-1,024, with 0 indicating no motion and 1,024 indicating the most vigorous motion. The average of activity counts during nonsleep minutes as scored by the actigraph program is the measure of daytime physical activity (DPA). Physical examination Data on body measurements, bioelectrical impedance, and stage of sexual maturity were collected during a physical exam by a two-person team of nurse practitioners trained in the research protocol (Cho et al., 2001). Body mass index (BMI) was calcu2 2 lated as weight (kg)/height (m ) (Dietz and Robinson, 1998). Fat-free mass was esti- mated using formula #3 in Houtkooper et al. (1992): FFM (kg) = 0.58 RI + 0.24 Weight (kg) + 0.109 Reactance (ohms) - 5.823 where RI is the resistance index (=height2 2 (cm )/resistance (ohms)). This formula is desirable because of its simplicity, low standard errors of estimates, and stability across several samples of children and young adults (Houtkooperet al., 1992). Fat mass (FM) and percent body fat (PBF) were then calculated: FM (kg) = weight (kg)- FFM (kg), and PBF = FM/weight x 100. Body fat variables were treated as both continuous and discrete. The discrete variable is occurrence of obesity (0 = not obese, 1 = obese). This is defined as having a BMI above the 85th percentile for sex and age as per the CDC growth charts, in addition to having a percent body fat of 25% orabove formales or30% orabove forfemales (Williams et al., 1992). Sexual maturity was assessed as breast stages forgirls and genital stages for boys. The five sexual maturity stages were dichotomized as: stages 1-3 less mature (=0) and 4-5 mature (=1) (Mueller et al., 2001). Additional variables Behavioral variables that could potentially affect sleep behaviors include self-reported use of tobacco, alcohol, drugs, birth control pills, and pregnancy status. Descriptive statistics for continuous and categorical variables are shown in Table 1. Statistical methods Hypothesis 1: Correlation and chi-square analysis (for discrete variables) were used to assess the association of obesity, sleep variables, and physical activity with behavioral and demographic variables. Behavioral and demographic variables that are significantly (P < 0.05) related to sleep behavior, activity, or obesity would appear as potential independent variables in tests of subsequent hypotheses. Hypothesis 2: Daytime physical activity is regressed on the sleep quality variables as well as demographic and confounding vari- TABLE I. Variables, codes, and descriptive statistics (Heartfelt Study) Descriptive statistics Variable description Code 2 Body mass index (kg/m ) Percent body fat (%) Daytime physical activity (count) Total sleep time (hours) Sleep disturbance time (hours) Sexual maturity (Tanner Stages 1-5) Age (years) Gender(0 = male, 1 = female) BMI PBF DPA TST SDT SXM AGE SEX Obesity* OBS Caucasian ethnicity African-American ethnicity Hispanic ethnicity Alcohol use Drug use Tobacco use Birth control pill use Pregnancy CCE AAE HPE ALC DRG TBC BCP PRG Frequencies Mean 361 22.43 361 23.16 361 228.64 361 7.68 361 1.17 361 4.00 361 13.00 167 (46.2%) male 194 (53.7%) female 38 (22.8%) males 58 (29.9%) females 96 (26.6%) overall 122 (33.7%) 130 (36.0%) 109 (30.1%) 21 (5.8%) 15 (4.1%) 49 (13.5%) 3 (0.8%) 2 (0.5%) SD Range 5.25 8.66 23.18 0.55 0.51 1.17 1.66 10.9-45.8 1.6-43.9 180-270 5.7-9.1 0.2-2.6 1-5 11-16 *Obesity = BMI above the 85th CDC percentile for age and sex and % body fat equal to or exceeding 25% if male and 30% if female. ables found to be significantly related to used to test this hypothesis treating body DPA in testing hypothesis 1, the full model fat as a continuous variable (BMI, PBF). being: RESULTS Y(DPA) = a + b1 (TST ) + b2 (SDT ) + b3 (SEX Descriptive statistics for the sample are ) shown in Table 1. Average (undisturbed) + b4 (ALC) + b5 (SEX X ALC) sleep time in the sample was 7.68 hours (range: 5.7-9.1 hours) and average sleep disturbance time was 1.17 (range: 0.2-2.6 where variable designations are as in hours). The sample was more or less evenly Table 1. divided by sex and ethnicity. Obesity ocHypothesis 3: Multiple logistic regression currence was substantial at 26.6% overall: analysis is an appropriate way to model the 22.8% in males and 29.9% in females. The effect of sleep quality on a discrete depen- prevalence of unhealthy behaviors was: aldent variable (obesity) (Zar, 1996). Inde- cohol use (5.8%), drug use (4.1%), and topendent variables in the model are sleep bacco (13.5%). Very few children reported behaviors, demographics, and health risk either birth control use or pregnancy, so behaviors, the basic model being: these variables were excluded from further analyses. LOGODDS (OBS) = (intercept) + coefficient (TST ) Hypothesis sleep quality is unrelated to demographic and confounding variables + coefficient (SDT ) (Table 2) + coefficient (AGE) Total sleep and sleep disturbance times + coefficient (SEX ) were, for all practical purposes, not significantly associated with any of the demo+ coefficient (SXM ) graphic or confounding variables whether + coefficient (AAE) by parametric correlation (Table 2), non+ coefficient (HPE) parametric correlation, or chi-square analysis. Fewer hours of sleep were associated with variable designations as in Table 1. with a higherprevalence of obesity (r= Odds ratios and their 95% confidence limits -0.36 in boys and -0.29 in girls). Total sleep are reported. Multiple regression was also TABLE 2. Correlations among study variables TST TST SDT DPA OBS AGE AAE CCE HPE TBC DRG ALC SXM 0.07 0.05 -0.36 -0.10 0.06 0.08 -0.14 0.00 -0.02 0.04 -0.11 SDT DPA OBS AGE AAE CCE HPE TBC DRG ALC SXM -0.06 -0.04 -0.12 -0.29 0.01 -0.04 0.09 -0.05 0.03 -0.24 -0.05 -0.06 0.03 0.02 0.10 -0.10 0.10 0.01 -0.03 -0.11 -0.53 0.16 -0.04 -0.04 0.01 0.01 -0.49 -0.48 0.12 0.04 0.04 0.00 -0.09 -0.14 0.05 0.09 -0.08 0.03 0.02 0.12 -0.10 -0.01 0.00 0.01 0.40 0.03 0.00 0.01 -0.14 -0.08 -0.08 0.08 0.00 0.27 0.09 0.03 -0.13 0.05 -0.17 0.57 0.09 -0.16 0.07 0.06 0.11 -0.08 -0.19 -0.04 -0.09 0.09 0.00 -0.10 -0.07 0.05 0.00 -0.01 -0.09 0.00 -0.01 -0.01 0.03 -0.05 -0.03 -0.19 0.02 0.08 0.05 -0.24 0.19 0.03 0.02 0.09 0.14 0.06 -0.09 0.02 0.02 -0.17 -0.08 0.35 -0.54 -0.50 0.00 0.14 0.00 0.25 -0.47 -0.03 -0.05 0.06 -0.19 0.03 -0.09 -0.06 -0.06 0.34 0.29 0.06 0.37 0.07 0.04 TABLE 3. Multiple regression of daily physical activity (DPA) on sleep disturbance time (SDT), sex (SEX), alcohol use (ALC), and sex X alcohol interaction (SEX * ALC) Variable SDT ALC SEX SEX * ALC Coefficient Standard error P-value -7.48 0.99 0.93 -20.56 2.36 7.41 2.47 10.24 0.0017 0.8928 0.7049 0.0454 Sample size n = 361. 2 Adjusted R for regression model = 0.037; F4,356 = 4.42 (P = 0.0018). Fig. 1. Numbers of obese children by sex and ethnicity, Heartfelt Study. and sleep disturbance times were uncorrelated. Sleep disturbance, but not total sleep time, was inversely associated with daily physical activity, more strongly in girls (r= -0.19, P < 0.01) than boys (r= -0.12, not significant). Physical activity and obesity occurrence were also minimally affected by demographic and confounding variables, with one exception: Obesity varied significantly (P < 0.01) with ethnicity in girls, African American and Hispanic girls having higherlevels than Caucasian girls (Fig. 1). Physical activity was less in girls who consumed alcohol (r= -0.19, P < 0.01). Obesity prevalence was higher in younger boys (P < 0.01). Hypothesis 2 lower daytime physical activity is associated with poorer sleep quality Mean DPA in the sample was inversely associated with sleep disturbance time (t4 = 3.15, P < 0.01, Table 3). Forevery hourof sleep disturbance there is a 7.4 unit or 3% decrease in DAP. There was also a negative association of DPA with the interaction of SEX * ALC (t4 = 2.00, P < 0.05). Girls using alcohol had a corresponding 20.5 unit or 9% decrease in DPA. The adjusted R2 (x100) for the model is 3.7% (P < 0.01). Hypothesis 3 obesity is associated with lower sleep quality (fewer sleep hours and more disturbed sleep) in the sample independent of age gender ethnicity and other potential confounders Logistic regression of obesity occurrence on the independent variables is shown in Table 4. There is a strong and statistically significant negative association of obesity and total sleep time (P < 0.01). Age and sex were less associated with obesity than their interaction (P < 0.01): Obesity occurs more frequently at younger ages in boys. When body fat is treated as continuous values of BMI orPBF, conclusions are indistinguishable from those reached treating obesity as a dichotomous variable: Higher values are associated with less total sleep time independent of potential confounding variables (analyses not shown). Variable TST AGE SEX SXM CCE HPE AGE * SEX Coefficients (std. err.) O.R. 95% C.I. (low-high) P-value -1.62 (0.28) -0.37 (0.14) -5.49 (2.20) 0.01 (0.13) -0.80 (0.34) 0.24 (0.31) 0.42 (0.16) 0.20 0.69 0.05 1.01 0.44 1.27 1.53 0.11 0.53 0.00 0.78 0.23 0.69 1.11 0.34 0.90 0.31 1.30 0.85 2.32 2.12 0.0000 0.0070 0.0126 0.9375 0.0147 0.4437 0.0091 Parameters: coefficients with standard errors, odds-ratios (O.R.), 95% confidence intervals of O.R., and values for independent variables (n = 361). 2 Chi-square = 64.7793; df = 7; P < 0.0001; Cox and Snell R = 0.16; TST = Total sleep time (hrs); AGE = age (yrs); SEX = sex (male = 0, female = 1); SXM = sexual maturity; CCE = caucasian ethnicity (0 = no, 1 = yes); HPE = Hispanic ethnicity (0 = no, 1 = yes). DISCUSSION None of the behavioral or demographic variables seemed to relate to sleep time or disturbance in a consistent manner. Caucasian girls had a lower occurrence of obesity than girls of other ethnicities and obesity increased in frequency at younger ages in boys. Obesity was not affected by other demographic or behavioral variables (Table 2). Obesity was the single most consistent correlate of sleep time and lack of physical activity the single most consistent correlate of sleep disturbance on initial correlation analysis (Table 2). Multiple regression of physical activity on predictor variables showed that sleep disturbance or wakefulness during nighttime was mildly associated with lowerdaytime physical activity (Table 3). Every hour increase in sleep disturbance was associated with a 7.4 unit or3% decrease in DPA. Although obesity itself was not related to sleep disturbance in the subsequent logistic regression analysis, the data are consistent with a plausible way that sleep disturbance could ultimately affect obesity through reduction in daytime physical activity. The decrease in physical activity was more pronounced in girls who drank alcohol (9%). Sleep time had a significant effect on the prevalence of obesity in the sample independent of potential confounding variables (Table 4). The odds ratio associated with sleep time was 0.20, implying that forevery hourof increased sleep time the odds of obesity decreases by 80%. Although the study results cannot establish sleep debt as a causative factorin obesity, it does show that the likelihood of obesity in a person with fewerhours of sleep is significantly greater than in individuals who sleep longer. Since extreme cases of sleep debt were not available forthis study, which only covered one 24-hour period in the life of an adolescent, it could not be determined to what extent this relation holds true in general. These results should be interpreted with caution, as additional studies are needed with more sampled days to confirm ordeny an association of low sleep quality and obesity that was found in this study. The data suggest that addressing inadequate and low sleep quality in adolescents may play an important role in the prevention of obesity. Study limitations The study is cross-sectional in design, with attendant limitations forcause and effect reasoning. For a given height and level of physical exertion, fatigue may be greater in the obese child than in the nonobese child. This would have led to an expectation of more, not less sleep in the obese compared to nonobese. Although some studies give plausibility to the argument that sleep debt could be a cause of obesity, it is also possible that low sleep quality fol- lowed as a result of the subjects being obese because of sleep apnea (Marik, 2000). The 24-hour period the actigraph covered in this study would not have allowed forthe most accurate representation of daily sleep habits. Averaging activity measures from recordings taken over a 5-day or more time period would have provided a more realistic picture of daily sleep patterns (Acebo et al., 1999). Nevertheless, the association of sleep time with obesity in this sample was statistically significant to a high degree and occurred in both sexes in a model that included potential confounding factors. Ninety-two percent of recorded sleep time in this study occurred during nighttime, arbitrarily defined as 8 PM to 8 AM. This implies that some of the sleep pertained to daytime naps (8%). It would have been betterto confine the study to purely night hour sleep, but this was the most reliable way to do it given the methodologies available in the Heartfelt Study. Daytime physical activity by actigraph methodology works best in the vigorous range and may be less accurate for intermediate activity levels. Other variables that could play a significant role in the prevention of obesity (e.g., participation in regular exercise or school sports) were not collected in this study and therefore their impact on the sleep behavior/obesity connection could not be assessed. LITERATURE CITED Acebo C, Sadeh A, SeiferR, Tzischinsky O, Wolfson AR, Hafer A, Carskadon MA. 1999. Estimating sleep patterns with activity monitoring in children and adolescents: how many nights are necessary for reliable measures. Sleep 22:95-103. Baranowski T, Mendlein J, Resnicow K, Frank E, Cullen KW, Baranowski J. 2000. Physical activity and nutrition in children and youth: an overview of obesity prevention. Prev Med 31:S1-S10. Barth N, Ziegler A, Himmelmann GW, Coners H, Wabitsch M, Hennighausen K, Mayer H, Remschmidt H, Schafer H, Hebebrand J. 1997. Significant weight gains in a clinical sample of obese children and adolescents between 1985 and 1995. Int J Obes Relat Metab Disord 21:122-126. Bonnet MH, Arand DL. 1995. We are chronically sleep deprived. Sleep 18:908-911. Bourgin P, Huitron-Resendiz S, Spier AD, Fabre V, Morte B, Criado JR, Sutcliffe JG, Henriksen SJ, Lecea L. 2000. Hypocretin-1 modulates rapid eye movement sleep through activation of locus coeruleus neurons. J Neurosci 20:7760-7765. Carskadon MA. 1990. Patterns of sleep and sleepiness in adolescents. Pediatrician 17:5-12. Cho SD, Mueller WH, Meininger JC, Liehr P, Chan W. 2001. Blood pressure and sexual maturity in adolescents: The Heartfelt Study. Am J Hum Biol 13:227234. Cole RJ, Kripke DF, Gruen W, Mullaney DJ, Gillin JC. 1992. Automatic sleep/wake identification from wrist activity. Sleep 15:461-469. Dietz WH, Robinson TN. 1998. Use of the body mass index (BMI) as a measure of overweight in children and adolescents. J Pediatr132:191-193. Houtkooper LB, Going SB, Lohman TG, Roche AF, Van Loan M. 1992. Bioelectrical impedance estimation of fat-free body mass in children and youth: a crossvalidation study. J Appl Physiol 72:366-373. Jean-Louis G, Zizi F, von Gizycki H, Hauri P. 1999. Actigraphic assessment of sleep in insomnia: application of the Actigraph Data Analysis Software (ADAS). Physiol Behav 65:659-663. Kelman BB. 1999. The sleep needs of adolescents. J Sch Nurs 15:14-19. Klosch G, Gruber G, Anderer P, Saletu B. 2001. Activity monitoring in sleep research, medicine and psychopharmacology. Wien Klin Wochensch 113:288-295. LegerD. 2000. Public health and insomnia: economic impact. Sleep 23(Suppl 3):S69-S76 Mantz J, Muzet A, Winter AS. 2000. The characteristics of sleep-wake rhythm in adolescents aged 15-20 years. A survey made at school during ten consecutive days. Arch Pediatr 7:256-262. Marik PE. 2000. Leptin, obesity and obstructive sleep apnea. Chest 118:569-571. Mason DJ, RedekerN. 1993. Measurement of activity. Nurs Res 42:87-92. Meininger JC, Liehr P, Mueller WH, Chan W, Smith GL, Portman RJ. 1999. Stress-induced alterations of blood pressures and 24 h ambulatory blood pressure in adolescents. Blood Pres Monit 4:115-120. Mokdad AH, Serdula MK, Dietz WH, Bowman BA, Marks JS, Koplan JP. 1999. The spread of the obesity epidemic in the United States, 1991-1998. JAMA 282:1519-1522. Mueller WH, Cho SD, Meininger JC, Liehr P, Chan W. 2001. Strategies for combining and scaling sexual maturity indicators: Heartfelt Study. Ann Hum Biol 28:422-430. Nestle M, Jacobson MF. 2000. Halting the obesity epidemic: a public health policy approach. Public Health Rep 115:12-24. Patterson SM, Krantz DS, Montgomery LC, Deuster PA, Hedges SM, Nebel LE. 1993. Automated physical activity monitoring: validation and comparison with physiological and self-report measures. Psychophysiology 30:296-305. Rosmond R, Bjorntorp P. 2000. The role of antidepressants in abdominal obesity. Med Hypoth 54:990-994. Ross R, Janssen I, Tremblay A. 2000. Obesity reduction through lifestyle modification. Can J Appl Physiol 25:1-18. Scheen AJ. 1998. Clinical study of the month. Does chronic sleep deprivation predispose to a metabolic syndrome? Rev Med Liege [in French] 54:898-900. Scheen AJ, Van CauterE. 1998. The roles of time of day and sleep quality in modulating glucose regulation: clinical implications. Horm Res 49:191-201. Shinkoda H, Matsumoto K, Hamasaki J, Seo YJ, Park YM, Park KP. 1998. Evaluation of human activities and sleep-wake identification using wrist actigraphy. Psychiatry Clin Neurosci 52:157-159. Spiegel K, Leprout R, Van Cauter E. 1999. Impact of sleep debt on metabolic function. Lancet 354:14351439. Sutcliffe JG, de Lecea L. 2000. The hypocretins: excitatory neuromodulatory peptides for multiple homeostatic systems, including sleep and feeding. J Neurosci Res 62:161-168. Troiano RP, Flegal KM. 1998. Overweight children and adolescents: description, epidemiology, and demographics. Pediatrics 101:497-504. Troiano RP, Flegal KM, Kuczmarski RJ, Campbell SM, Johnson CL. 1995. Overweight prevalence and trends forchildren and adolescents: the National Health and Nutritional Surveys, 1963-1991. Arch Pediatr Adolesc Med 149:1085-1091. Williams DP, Going SB, Lohman TG, Harsha DW, Srinivasan SR, Webber LS, Berenson GS. 1992. Body fatness and risk for elevated blood pressure, total cholesterol, and serum lipoprotein ratios in children and adolescents. Am J Public Health 82:358-363. Wolfson A, Carskadon M. 1996. Early school times affect sleep and daytime functioning in adolescents. Sleep Res 25:117. Wolfson AR, Carskadon MA. 1998. Sleep schedules and daytime functioning in adolescents. Child Dev 69:875-877. Wurtman RJ, Wurtman JJ. 1995. Brain serotonin, carbohydrate-craving, obesity and depression. Obes Res 3(Suppl 4):477S-480S. ZarJH. 1996. Biostatistical analysis, 3rd ed. Upper Saddle River, NJ: Prentice-Hall
Step by Step Solution
There are 3 Steps involved in it
Step: 1
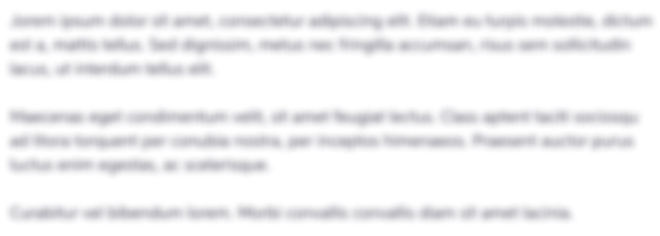
Get Instant Access to Expert-Tailored Solutions
See step-by-step solutions with expert insights and AI powered tools for academic success
Step: 2

Step: 3

Ace Your Homework with AI
Get the answers you need in no time with our AI-driven, step-by-step assistance
Get Started