Answered step by step
Verified Expert Solution
Question
1 Approved Answer
An year ago, a group of students HD, RF, LD, and KC designed a project that would allow them to predict the best time taken
Step by Step Solution
There are 3 Steps involved in it
Step: 1
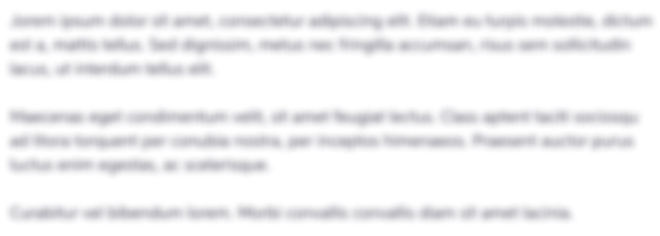
Get Instant Access to Expert-Tailored Solutions
See step-by-step solutions with expert insights and AI powered tools for academic success
Step: 2

Step: 3

Ace Your Homework with AI
Get the answers you need in no time with our AI-driven, step-by-step assistance
Get Started