Question
ASAP!!! Theme: COVID19 CRISIS using math, PLEASE I NEED THE ANSWERS ONLY FOR EXERCISE #2 I want to answer my exercise #2, according to my
ASAP!!! Theme: COVID19 CRISIS using math, PLEASE I NEED THE ANSWERS ONLY FOR EXERCISE #2
I want to answer my exercise #2, according to my reflections and answers to my exercise #1:
Exercise #1:
If the logistic curve is S-shaped and stabilizes, like the classic population and epidemiological curves, what difficulties would we encounter if we tried to apply the same technique to find its best-fit version to our data? Can we do it? Why or why not? Can these difficulties be overcome? How?
If we could find the best-fit logistic curve, what could we project or predict with them that would be useful for the COVID19 crisis?
My reflections and responses to exercise #1:
The difficulty we would encounter in applying the same technique to find the best-fit curve to our data is that we cannot linearize in a "nice" way the functions corresponding to the logistic curve and the Weibull distribution. And by "nice" way I mean like we were able to do for the simple exponential model. Therefore, other more complex methods like the "Nonlinear Least Squares estimation" have to be used.
We could implement this to find the best fits to our data, but I think it will give a not very accurate result. So far, visually our data does not yet have that S shape. Rather we have that initial part of the S, characterized by an exponential growth. Therefore, the best we can do is to extrapolate to get the S that might fit the current data and future data.
If we could find the best fit curves for the COVID19 crisis, we could predict when we would have the maximum growth in cases and when we would have that plateau of confirmed cases and thus be able to overcome our difficulties.
In conclusion, we cannot transform the problem into a linear one, as we did with the exponential curve. We should see a method to work the least squares in these cases. With this method, we can also see that even finding the best fit curves, that maximum growth rate and when it occurs, are very difficult to estimate even with this best fit curve, as it turns out that it depends very sensitively on changes or additions to the data.
Exercise #2:
- Question to answer with good quality explanations: One question to ask is whether it is appropriate when proposing a model to look at daily or cumulative data. For example, we can look at daily admissions to the hospital or we can look at cumulative admissions since some start date. Clearly, the "curves" that generate the data will be different. What do you think, in light of the reflections made in exercise 1? Which type of data is the logistic curve for, daily or cumulative? What would you do to model the other type of data?
Step by Step Solution
There are 3 Steps involved in it
Step: 1
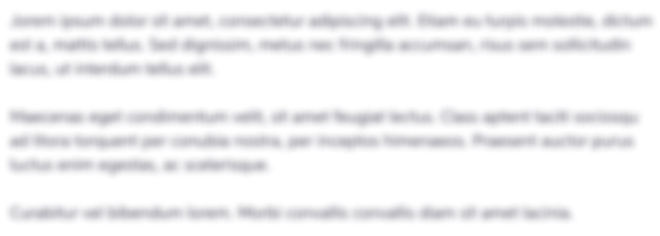
Get Instant Access to Expert-Tailored Solutions
See step-by-step solutions with expert insights and AI powered tools for academic success
Step: 2

Step: 3

Ace Your Homework with AI
Get the answers you need in no time with our AI-driven, step-by-step assistance
Get Started