Answered step by step
Verified Expert Solution
Question
1 Approved Answer
BE562: Problem Set 3 Fall 2016 Due 10/21/2016 8PM 1. Hidden Markov Models and Protein Structure (20 pts) One biological application of hidden Markov models
BE562: Problem Set 3 Fall 2016 Due 10/21/2016 8PM 1. Hidden Markov Models and Protein Structure (20 pts) One biological application of hidden Markov models is to determine the secondary structure (i.e. the general three dimensional shape) of a protein. This general shape is made up of alpha helices, beta sheets, turns and other structures. In this problem we will assume that the amino acid composition of these regions is governed by an HMM. In order to keep this problem relatively simple, we do not use actual transition values or emission probabilities. We will use the state transition probabilities of: Alpha Helix Beta Sheet Turn Other Alpha Helix 0.7 0.3 0.1 0.2 Beta Sheet 0.2 0.6 0.1 0.6 Turn 0.1 0.0 0.1 0.0 Other 0.0 0.1 0.7 0.2 where, for example, P (Alpha Helix Turn) = 0.1. The start state is always Other (accordingly the first emission will be from the Other state) and the emission probabilities are: Amino Acid M L N E A G Alpha Helix 0.50 0.25 00 0.05 0.15 0.02 0.03 Beta Sheet 0.05 0.10 0.35 0.30 0.15 0.05 Turn 0.00 0.20 0.10 0.05 0.20 0.45 Other 0.10 0.10 0.10 0.25 0.10 0.35 (a) Draw this HMM. Include the state transition probabilities and the emission probabilities for each state. (b) What is the probability of the second emitted character being an L? (c) Give the most likely state transition path for the amino acid sequence MLAE using the Viterbi algorithm. What is P (X, Y) for X = MLAE and Y = (the Viterbi path)? (Include the Viterbi algorithm table in your solution.) 1 (d) How many other paths could give rise to the same sequence MLAE? Compute the total probability P (X) for X = MLAE, defined as P (X) = (, ) (although this is not how you should compute it!). Compare this to P (X, Y) for the Viterbi path in part (c). What does this say about the reliability of the Viterbi path? 2. Markov Chains for CpG Classification (20 pts) In this problem, we will simulate and test the use of Markov chains for classifying DNA sequences based on nucleotide frequencies. In these Markov chains, we have four states, one for each nucleotide, and transition probabilities between the states. This is a degenerate case of an HMM in which the state fully determines the emission (thus removing the \"hiddenness\"). Page 50 of Durbin gives the following transition probabilities for the models of being inside a CpG island (M1) and outside a CpG island (M2): M1 A C G T A 0.18 0.171 0.161 0.079 C 0.274 0.367 0.339 0.355 G 0.426 0.274 0.375 0.384 T 0.12 0.188 0.125 0.182 M2 A C G T A 0.3 0.322 0.248 0.177 C 0.205 0.298 0.246 0.239 G 0.285 0.078 0.298 0.292 T 0.21 0.302 0.208 0.292 where, for example, PM 1(A G) = 0.426. We will additionally assume a uniform distribution among nucleotides in the initial state (that is, the chains start in each state with probability 0.25). Write code to (1) generate random sequences from Markov chains defined by M1 and M2 and (2) find the probability of a given sequence being generated by M1 or M2. (a) Simulate 10000 sequences of length 6 from M1. Of the sequences simulated, how often is the probability of the sequence being generated by M2 higher than the probability of it being generated by M1? (b) Now, simulate 10000 sequences of length 6 from M2. Of the sequences simulated, how often is the probability of the sequence being generated by M1 higher than the probability of it being generated by M2? (c) What do the two parts above tell you about the potential for error when classifying sequences into regions of CpG islands using a simple likelihood estimate? (d) If you had a prior on how often a sequence was part of a CpG island, how would you use this information to better classify sequences? In addition to your answers above, include the source code you used in your submission. 3. HMMs, State Durations, and GENSCAN (20 pts) An important use of HMMs is to decode or parse a genome into its biological components, such as 2 exons, introns, CpG islands, etc. In this problem, we will examine how the accuracy of HMM predictions is affected by certain inherent properties of the model. In lecture, we developed an HMM to detect CpG islands that requires eight states in order to capture the CpG dinucleotide bias. To simplify this problem, instead of searching for CpG islands, we will simply search for High-GC (on average 60% G or C) and Low- GC regions (on average 60% A or T). Thus, our model requires only two states. We have provided a program, viterbi.py, which you will complete and use to decode several artificial genomes, and then compare the resulting predictions of High-GC and Low-GC regions to a provided (correct) annotation. More details about this program are included at the end of the problem. (a) In most HMMs, the self-loop transition probabilities akk are large, while the transition probabilities between different states akl are small. Once a Markov chain with these transition probabilities enters state k, it tends to stay in state k for a while. The state duration is the total number of consecutive steps at which the Markov chain stays in the same state, before switching to another state (e.g. transitioning into state k and then transitioning out to a different state is a state duration of 1). What is the expected (mean) state duration of state k as a function of the transition probability akk? What is the distribution of state durations P (Dk = d)? (b) Complete the implementation of the Viterbi algorithm in viterbi.py. The skeleton code is explained in the file. Based on the HMM parameters hard-coded into the program, what are the expected state durations for High-GC and Low-GC regions? Apply the finished program to the data file hmmgen, which was generated using the same HMM, and verify that your program achieves 83% accuracy. (c) Now apply your program to the files mystery1, mystery2, and mystery3. How do the (correct) state duration distributions in the mystery sequences differ and what do they have in common? What accuracy levels does your HMM achieve on these sequences? How does each Viterbi predicted state duration distribution differ from the correct distribution? (You don't need to include the plots in your solutions.) (d) Would re-training the HMM parameters according to the procedure described in lecture, using the correct annotations as training data, improve the accuracy of the Viterbi annotation for the mystery sequences? Why or why not? (optional for 10 bonus points) Try to make the decoder perform better by adjusting the hard-coded model parameters. If you succeed, can you explain why? (e) As you are now aware, the length distribution of genomic elements can strongly affect the predictive accuracy of an HMM used to decode them. Unfortunately, most elements in real genomes do not follow the length distribution you derived in part (a). As we covered in lecture, generalized hidden Markov models (GHMMs) allow for an arbitrary length distribution for states. However, as we saw in the lecture, this leads to a substantial increase in the computational complexity of running the Viterbi algorithm. We saw in lecture that Genscan is an example of a GHMM used for gene 3 prediction. By reading the paper below (and associated supplementary material), describe the strategy used by Burge et al to increase the efficiency of Genscan. Burge C, Karlin S. Prediction of complete gene structures in human genomic DNA. J MOL BIO 268(1):78-94, 1997. 4. Gibb's Sampling (20 pts) Implement a Gibbs sampling motif finder. We provide a template file gibbs.py, but you are free to use a programming language of your choice. The Gibbs sampling algorithm can be summarized as follows: Algorithm 1 Chooses motifs of length L from a set of strings S1, . . . ,St 1: function GibbsSampler(S1, . . . , St, L) 2: Choose a substring of length L from each of S1, . . . , St 3: Initialize the PFM 4: 5: 6: while not converged do Choose a sequence at random, Sc 7: Score all substrings of length L from Sc using the PFM 8: 9: Stochastically choose a new substring from Sc with probability proportional to its score 10: return chosen substrings, PFM Many details of this procedure have intentionally been left ambiguous. Specific implementations are described in lecture and discussion, but we encourage you to experiment with different approaches. Make sure to discuss your design decisions in your solutions. Run your code on the four provided data sets. data1 is an artificial data set with a planted, identical motif, data2 is an artificial data set with a degenerate motif, and data3and data4 are real promoter sequences from yeast known to bind the transcription factors ACE2 and MBP1, respectively. The GC content of data1 and data2 is approximately 0.5 and the GC content of data3 and data4 is approximately 0.37. Turn in a copy of the code you added/wrote in addition to what patterns of length 10 your program found consistently for each the four provided data sets. Repeat the procedure several times for each data set until you believe you have found the strongest patterns. The pattern you find in data1 should be very strong. 4 5. HMMs for pairwise sequence alignment (20 points) In lecture 9, we saw how to construct an HMM corresponding to a generative model for the alignment of two sequences given that the sequences were evolutionarily related. This is a generative model for P(X,Y,). (a) What is in P(X,Y,)? (b) Given two sequences {X,Y}, this model could be used to calculate the probability that X and Y are related. What algorithm would you use for this? (c) In lecture 4 we saw that a general S(x,y)=log(Pab/(qaqb)). However, in lecture 9 we calculated the Viterbi recursion for this HMM and compared it to the recursion derived from the finite state machine (FSM - also called a finite state automata (FSA)) model (slide 47) and S(x,y) does not appear to take the expected form. Why not? (d) The following is a generative model for two random sequences - let's call this Prand(X,Y). Given this model, what is the probability of a pair of sequences {X,Y} from this model. (e) We want to correct the HMM Viterbi recursion on slide 47 of lecture 9 so that we get the expected form of S(x,y) when comparing with the FSM approach. In the Viterbi on slide 47, we are calculating: argmax (, , ) Instead what we would like to calculate is: argmax (, , ) (, ) Modify the recursion on slide 47 to calculate this quantity and show that is recovers the expected form of S(x,y). Hint: The key thing to realize is that the Prand is independent of . So we are free to allocate terms of Prand to any terms of P(X,Y,). And the terms of P(X,Y,) correspond to each state of a given . 5 6. Conjugate Priors (12 pts) (a) Assume a binomial distribution with parameter p: (1 ) = ( 1 + 2 ) 1 (1 )2 1 We want to estimate the parameter p which is P(outcome 1). Assume you have a training set of n data points of which n1 are outcome 1 and n2 are outcome 2. Derive the maximum likelihood estimator for p given these data. (b) Recall that the beta distribution is the conjugate prior for the binomial. Beta ( p a, b ) = G(a + b ) a -1 b -1 p (1- p) G(a ) G( b ) Show that if the prior probability of p follows a beta with parameters () and the likelihood of p given the data set from (a) follows a binomial, the posterior probability of p given the data set is a beta distribution with parameters (N1 + , N2 + ) (c) Given a posterior distribution of p that follows beta(), derive the expected value of p. (d) Repeat part (a), but this time assume a multinomial distribution instead of a binomial. (e) The conjugate prior for a multinomial is the Dirichlet. Derive the form of the posterior probability if the likelihood is a multinomial and the prior is a Dirichlet. (f) Derive the expected value for the parameters of a multinomial given a training set and a Dirichlet prior. 6 7. Gibb's Sampling In-depth [Graduate Students Only] (20 pts) Gibbs sampling is one of a family of approaches, called Markov chain Monte Carlo algorithms, for generating samples from a distribution given only limited information about it, such as only its pdf or, in the case of Gibbs sampling, only the conditionals. Gibbs sampling is particularly useful when the conditionals are much easier to formulate than the full joint. Given only a procedural description of Gibbs sampling (e.g. from problem 4), it may not be obvious that it converges to a meaningful answer. In this problem, we'll walk through a simplified version of the algorithm in order to understand how and why it works. We will look for a motif of length (L + 1) in just two sequences, X = x 1 xM +L and Y = y1 yN +L. Let P (I = i, J = j) be the joint probability that a motif starts at position i in sequence X , i 1,. . , M , and at position j in sequence Y , j 1,. . , N . If we knew this distribution, then we could find the most likely argmax positions for a motif as ij P(I=I,J=j). Unfortunately, we do not know P (I = i, J = j). (a) Design expressions for the conditional distributions P (I = i|J = j) and P (J = j|I = i). These expressions need not be closed form. Conceptually, P (I = i|J = j) answers the question: given that some motif appears in Y at yj yj+L, what is the probability that the same motif appears in X at xi xi+L? (Hint: A simple heuristic would define P (I = i|J = j) to be proportional to the percent identity of xi xi+L and yj yj+L.). It is up to you how, or whether, to incorporate a background model. In any case, ensure that for all j, =1 ( = | = ) = 1, and similarly for all i, =1 ( = | = ) = 1. The Gibbs sampler initializes with an arbitrary position in X, which well call I0. We stochastically choose J0 (a position in Y ) according to P (J0 = j|I0 = i), one of the conditional distributions you defined. Then, we choose I1 according to P (I1 = i|J0 = j). Then we choose J1, I2, J2, I3, J3, I4, . . (b) We can view the conditional distributions you defined in part (a) as transition matrices in this sequence of positions, giving the probability of the next choice based on the last choice. Assume you have computed the transition matrices A and B where aji = P (I = i|J = j) and bji =P(J=j | I=i). Give a formula for the transition matrix C where = (+1 = | = ). (c) Now, consider a Markov chain governed by the transition matrix C, which generates the sequence I1, I2, I3, . . . . The states in this Markov chain correspond to positions in the sequence X . (We could similarly derive a Markov chain for Y ). What is (6878 = |0 = 0 ) in terms of C? We now have an exact solution to this motif finding problem for two sequences. In the case of n sequences, we would have n conditional distributions, each dependent on the motif positions in the other n 1 sequences. (d) Describe what the transition matrices from part (b) would be in this case. Would it still be practical to use this approach? Explain your answer. (e) In the two-sequence case, how could you approximate P (I = i) by simulating the Markov chain? Based on this intuition, outline a strategy for estimating the positions of the motif. 7
Step by Step Solution
There are 3 Steps involved in it
Step: 1
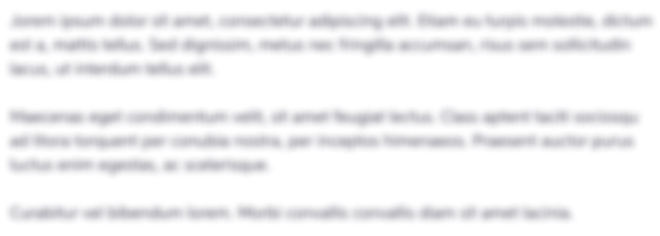
Get Instant Access to Expert-Tailored Solutions
See step-by-step solutions with expert insights and AI powered tools for academic success
Step: 2

Step: 3

Ace Your Homework with AI
Get the answers you need in no time with our AI-driven, step-by-step assistance
Get Started