Question
Build a function named firstname_lastname_perceptron.m which takes 3 inputs, i) training features ii) training labels and iii) maximum number of iterations. The function should output
Build a function named firstname_lastname_perceptron.m which takes 3 inputs, i) training features ii) training labels and iii) maximum number of iterations. The function should output the weight vector, accuracy and number of iterations required.
The function should also work for linearly inseparable data and return a linear decision boundary which gives maximum accuracy. You can achieve this by modifying your perceptron function such that it saves weights (W) and accuracy (a) at each iteration and return 'W', 'a' for the iteration where 'a' was maximum.
function [w,a,iter] = firstname_lastname_perceptron(train_features, train_labels, maxiter)
Inputs:
1. train_features: (NxD) Array with D dimensional N data points.
2. train_labels: (Nx1) Array containing labels of N data points. Remember that perceptron algorithm requires your labels to be -1 and 1 one.
3. maxiter: maximum number of iterations.
Outputs:
1. w: weight vector. Bias value is in the weight vector itself. w = [w b]
2. a: accuracy of classification
3. iter: iterations required
Use the following code to generate training data.
train_mean1 = [10,10]; %train data cluster 1 mean
train_sigma1 = [2 -1.5; -1.5 2];
train_mean2 = [7,7]; %train data cluster 2 mean
train_sigma2 = [2 -0.2; -0.2 2];
ntrain1 = 50; %Number of train data points for cluster 1
ntrain2 = 100; % Number of train data points for cluster 2 train_featuresa = mvnrnd(train_mean1,train_sigma1,ntrain1); train_labelsa = 1*ones(ntrain1,1);
train_featuresb = mvnrnd(train_mean2,train_sigma2,ntrain2); train_labelsb = -1*ones(ntrain2,1);
train_features = [train_featuresa; train_featuresb];
train_labels = [train_labelsa; train_labelsb];
The above code would not generate a linearly seperable data everytime. You have to modify your perceptron algorithm such that it gives the best possible decision boundary.
Your function should return a=1 for linearly seperable data and a<1 for linearly inseperable data. Try to achieve maximum accuracy for linearly inseperable data. Your perceptron function will be tested for both linealry seperable and linearly inseperable data.
Hints: You can shuffle your data (training sample,training label) pairs before you start updating the weight vector.
Step by Step Solution
There are 3 Steps involved in it
Step: 1
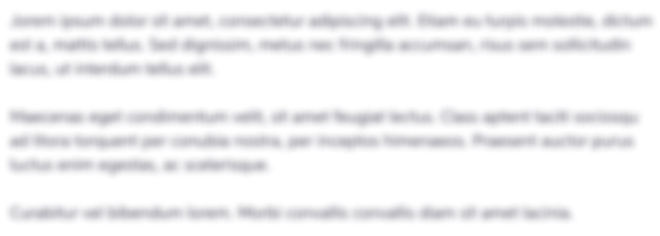
Get Instant Access to Expert-Tailored Solutions
See step-by-step solutions with expert insights and AI powered tools for academic success
Step: 2

Step: 3

Ace Your Homework with AI
Get the answers you need in no time with our AI-driven, step-by-step assistance
Get Started