Question
Calculate and interpret the linear relationship between companies using the correlation coefficient. Here, you should calculate the correlation between pairs of companies. use the following
Calculate and interpret the linear relationship between companies using the correlation coefficient. Here, you should calculate the correlation between pairs of companies. use the following companies: verizon vs AT&T, verizon vs T-mobile, etc. I need to include the section of your R code that allows me to perform the computation.
code example
#####1. Install the Packages if necessary#### if(!require("tidyverse")) install.packages("tidyverse") if(!require("tidyquant")) install.packages("tidyquant") if(!require("lubridate")) install.packages("lubridate") if(!require("PerformanceAnalytics")) install.packages("PerformanceAnalytics") if(!require("openxlsx")) install.packages("openxlsx")
#####2. Load the Packages#### library(tidyverse) library(tidyquant) library(lubridate) library(PerformanceAnalytics) library(openxlsx)
#####3. Data Wrangling####
######3.1.Asset Prices (Stocks) ######## Daily_Price <- c("NFLX","AMZN","AAPL") %>% tq_get(get = "stock.prices", from = "2018-01-01", to = "2023-06-30")
######3.2.Asset Returns (Stocks) ######## Daily_Ret <- c("NFLX","AMZN","AAPL") %>% tq_get(get = "stock.prices", from = "2018-01-01", to = "2023-06-30") %>% group_by(symbol) %>% tq_transmute(select = adjusted, mutate_fun = periodReturn, period = "daily", col_rename = "dRet")
Monthly_Ret <- c("NFLX","AMZN","AAPL") %>% tq_get(get = "stock.prices", from = "2018-01-01", to = "2023-06-30") %>% group_by(symbol) %>% tq_transmute(select = adjusted, mutate_fun = periodReturn, period = "monthly", col_rename = "mRet")
######3.3.Baseline Returns (Portfolio) ######## Monthly_Ret_Port <- "XLK" %>% tq_get(get = "stock.prices", from = "2018-01-01", to = "2023-06-30") %>% tq_transmute(select = adjusted, mutate_fun = periodReturn, period = "monthly", col_rename = "Rb")
######3.4.Join (Merge) ########
Monthly_Ret_Join <- left_join(Monthly_Ret,Monthly_Ret_Port,by="date")
######3.5.Reshape ########
Daily_Ret_Wide <- pivot_wider(Daily_Ret,names_from = symbol, values_from = dRet)%>% mutate(Period = if_else(date<=ymd("2019-12-31"),"Pre-Covid","Post-Covid"))
Monthly_Ret_Wide <- pivot_wider(Monthly_Ret,names_from = symbol, values_from = mRet) %>% left_join(.,Monthly_Ret_Port,by="date")
Monthly_Ret_Wide_TS <- read.zoo(Monthly_Ret_Wide, format = "%Y-%m-%d")
#####4. Analysis####
######4.1.Tables####
Table_summary <- table.Stats(Monthly_Ret_Wide_TS) %>% tibble::rownames_to_column(.,"Statistic")
Table_var <- table.Variability(Monthly_Ret_Wide_TS) %>% tibble::rownames_to_column(.,"Statistic")
Table_semi <- SemiSD(Monthly_Ret_Wide_TS, method = "full") %>% as_tibble(.) %>% mutate(Statistic="Semi-Deviation") %>% dplyr::select(Statistic,everything())
Table_semi2 <- DownsideDeviation(Monthly_Ret_Wide_TS, method = "full") %>% as_tibble(.) %>% mutate(Statistic="Downside-Deviation (MAR=0%)") %>% dplyr::select(Statistic,everything())
Table_summary2 <- table.AnnualizedReturns(Monthly_Ret_Wide_TS) %>% tibble::rownames_to_column(.,"Statistic")
Table_cor <- Monthly_Ret_Join %>% group_by(symbol) %>% summarise(Num_of_Months=n(), mRet_Mean=mean(mRet, na.rm= TRUE), mRet_SD=sd(mRet, na.rm= TRUE), Rb_SD=sd(Rb, na.rm= TRUE), Cov_mRet_Rb=cov(x=mRet,y=Rb, use="pairwise.complete.obs"), Cor_mRet_Rb=cor(x=mRet,y=Rb, use="pairwise.complete.obs"), Cor_mRet_Rb2=Cov_mRet_Rb/(mRet_SD*Rb_SD))
######4.2.Graphs####
chart.Boxplot(Monthly_Ret_Wide_TS)
chart.RiskReturnScatter(Monthly_Ret_Wide_TS)
chart.SnailTrail(Monthly_Ret_Wide_TS, legend.loc = "topleft")
#####5.Export & Communicate####
######5.1.Tables####
wb <- createWorkbook()
addWorksheet(wb,"Daily_Prices") writeData(wb, sheet = "Daily_Prices", x = Daily_Price)
addWorksheet(wb,"Daily_Returns") writeData(wb, sheet = "Daily_Returns", x = Daily_Ret_Wide)
addWorksheet(wb,"Monthly_Returns") writeData(wb, sheet = "Monthly_Returns", x = Monthly_Ret_Wide)
addWorksheet(wb,"T1_summary") writeData(wb, sheet = "T1_summary", x = Table_summary)
addWorksheet(wb,"T2_variability") writeData(wb, sheet = "T2_variability", x = Table_var)
addWorksheet(wb,"T3_semi") writeData(wb, sheet = "T3_semi", x = Table_semi)
addWorksheet(wb,"T4_semi2") writeData(wb, sheet = "T4_semi2", x = Table_semi2)
addWorksheet(wb,"T5_summary2") writeData(wb, sheet = "T5_summary2", x = Table_summary2)
saveWorkbook(wb, "FING6702_Mod6_R_Tables.xlsx", overwrite = TRUE)
remove(wb)
######5.2.Charts####
jpeg("FING6702_Mod6_R_Graph1.jpeg", quality = 75) chart.Boxplot(Monthly_Ret_Wide_TS) dev.off()
jpeg("FING6702_Mod6_R_Graph2.jpeg", quality = 75) chart.RiskReturnScatter(Monthly_Ret_Wide_TS) dev.off()
jpeg("FING6702_Mod6_R_Graph3.jpeg", quality = 75) chart.SnailTrail(Monthly_Ret_Wide_TS, legend.loc = "topleft") dev.off()
Step by Step Solution
There are 3 Steps involved in it
Step: 1
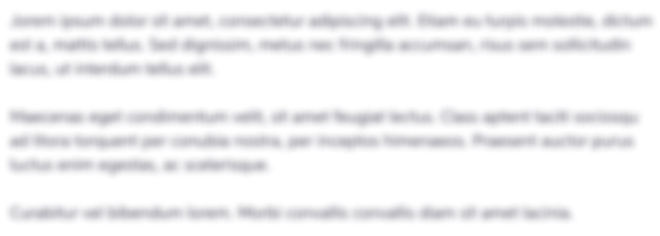
Get Instant Access to Expert-Tailored Solutions
See step-by-step solutions with expert insights and AI powered tools for academic success
Step: 2

Step: 3

Ace Your Homework with AI
Get the answers you need in no time with our AI-driven, step-by-step assistance
Get Started