Answered step by step
Verified Expert Solution
Question
1 Approved Answer
CASE 3 LUNA ELECTRONICS B 1 LUNA ELECTRONICS (B) Luna Electronics is an established firm that sells primarily through its catalog operation, though it does
CASE 3 LUNA ELECTRONICS B 1 LUNA ELECTRONICS (B) Luna Electronics is an established firm that sells primarily through its catalog operation, though it does have a small cadre of retail stores. In this regard it has some similarities to the better known Sharper Image operation. Luna has recently produced a potential new product that it calls the \"Holographic Imaging Televiewer (HIT).\" The HIT can be connected to any standard color television, and project a three-dimensional image not unlike 3-D movies of a few decades ago, but without any need for special glasses or other viewer connected paraphernalia. The screen resolution of the HIT greatly exceeds anything out in today's market that is suitable for an individual television set. The procedure for connecting the HIT to the television is very simple, involving a similar process to that of installing a VCR or DVD player. Luna is very excited about this new product, for it was quickly established by the manufacturing division that the HIT can be produced in reasonable quantities, and that it can be sold very profitably. Luna's management believes that there is an enormous market for the HIT, reasonably priced for any household with an income of more than $60,000. However, Luna's management believes that it may be key to capture the market as soon as possible, before Luna's competitors developed a similar product. This is a significant possibility, since the basic technology is sufficiently in the public domain, not allowing Luna to patent it in a way that could withstand competition. 2 Since it will be a number of months before Luna is prepared to sent out its next catalog, it decides to send a special direct-mail offering for the HIT to a subset of its customer database. This customer database (i.e., \"house list\") consists of about 80,000 names, all having been a previous purchaser from Luna. The list has been built up over the years through catalog mailings and occasional special separate offerings to segments of various rented lists. Each time Luna sends out a special (i.e., separate from its regular catalogs) mailing, it includes a cover letter, a brochure indicating the features and benefits of the product, including the price of the product, and a response device (e.g. a coupon to cut out and send back with your order); the offer includes a money-back guarantee within a limited time period. There is no overt charge for postage and handling, a fact for which Luna is well-known, though, of course, these costs are incorporated into the stated price of the product. Luna's direct marketing division was asked to examine the customer database, to identify the characteristics that would allow for a targeting of those names more likely to purchase the HIT in the near future. Lester Retsel, a direct marketing specialist who has been with Luna for ten years, was put in charge. Lester identified the seven characteristics listed in Table 1 (in the order in which they appear on the Luna customer database): Table 1 - Targeting Characteristics 1. Gender 5. Time since most recent Luna purchase 2. Age 6. Number of consecutive non-responses to Luna mailings 3. State of Residence 7. Total dollars of all Luna (mail) purchases 4. Luna credit card holder (yes/no) 3 Luna's management team in charge of the HIT project examined the targeting characteristics. One member of the team, Eve Lebel, wondered why none of the targeting characteristics were specifically connected to the HIT-like products. Lester responded that nearly all of Luna's customers were the type of people who liked electronic gadgets - after all, every name had made at least one purchase from Luna, and had sufficient income to purchase HIT if they liked the concept. He noted that the real issue is to identify who has more proclivity to purchase such a produce by mail, who is a more frequent/recent/dedicated Luna customer, and the mundane issue of which addresses are still correct. Eve was satisfied with that answer, and agreed that these should be the major targeting characteristics to be used to determine whom to mail the HIT offering. Lester was next charged with determining which of the above seven targeting characteristics were the most important targeting characteristics. He decided to address this issue by examining the relationship between each of the seven targeting characteristics and purchase of a similarly priced upscale electronics product. He chose the similarly-priced Luna product he believed was the most similar to the HIT. This turned out to be a television attachment that is built in to many of the higher priced televisions being sold today, but not built in to 99% of the televisions in current use - a \"channel display attachment\" (CDA). A CDA enables the user to form small boxes in each corner of his/her current television screen that display (up to four) additional channels other than the primary channel being watched. Lester believed that whatever relationships existed between the seven above targeting characteristics and the purchase of the CDA should hold also for the purchase of the HIT. 4 Lester's first step was to take the 40,000 names on the Luna customer list that were recently mailed a special offering for the CDA, and derive a separate file of every fifth name; this \"nth name sample\" of 8,000 names was to be used to analyze the data. These 8,000 names had each of the seven targeting characteristics on the database, as well as an indicator whether the name purchased the CDA or not. The next step Lester took was to generate a frequency distribution of each targeting characteristic immediately prior to the mailing in question. The data were routinely coded into a set of mutually exclusive, collectively exhaustive categories. For example, for targeting characteristic 7, total dollars of Luna mail purchases, the categories and frequency distribution is represented in Table 2: Table 2 Frequency Distribution of Total Dollars Categories Percent $0-49 23.4 $50-99 12.2 $100-199 14.1 $200-349 18.0 $350-599 15.3 $600-999 10.9 $1000+ 6.1 100 5 What was of more interest to Lester was the response rate (i.e. the number of responders divided by the number of names mailed) of each purchase category. This is noted in Table 3: Table 3 Response Rate-Total Dollars Categories Response Rate (%) $0-49 .4 $50-99 2.2 $100-199 3.1 $200-349 3.7 $350-599 5.3 $600-999 5.9 $1000+ 4.9 6 Lester considered the frequency distribution and corresponding response rate table for each of the seven targeting characteristics. He noticed what he thought was some sort of pattern for each one of the seven. Of course, the existence of a pattern is equivalent to there being a relationship between the targeting characteristic and the likelihood of response to the offer. Some of the patterns seemed to be somewhat similar to others, while some of them appeared to be stronger than others. Lester decided to continue to include all seven of the targeting characteristics and to use a multiple regression analysis approach. As noted earlier, each of the seven targeting characteristics were represented by a set of mutually exclusive, collectively exhaustive categories. For analysis by multiple regression, targeting characteristic 7, total dollars, was set up as follows: X7a = 1, if name's total dollars is from 50-99; 0 otherwise X7b = 1, if name's total dollars is from 100-199; 0 otherwise X7c = 1, if name's total dollars is from 200-349; 0 otherwise X7d = 1, if name's total dollars is from 350-599; 0 otherwise X7e = 1, if name's total dollars is from 600-999; 0 otherwise X7f = 1, if name's total dollars is 1000+; 0 otherwise 7 The detailed list of categories and variables for the 7 targeting characteristics is described below: Targeting Characteristic 1, Gender, had 2 categories: Male, and Female, and had one variable: X1a Targeting Characteristic 2, Age, had 12 categories: 0-18, 19-24, 25-29, 30-34, 35-39, 40-44, 45-49, 50-54, 55-59, 60-64, 65-69, 70+, and 11 variables: X2a, ..., X2k. Targeting Characteristic 3, State of residence, had 26 categories; some included one state, some two, three, and four states, and 25 variables: X3a, ..., X3y Targeting Characteristic 4, Luna credit card holder, had 2 categories: No, Yes, and 1 variable: X4a Targeting Characteristic 5, Time since most recent Luna purchase, had 7 categories, measured in months: 0-2, 3-5, 6-9, 10-12, 13-18, 19-24, 25+, and 6 variables: X5a, ..., X5f Targeting Characteristic 6, Number of consecutive non-responses to Luna mailings, had 7 categories: 0, 1, 2, 3, 4-5, 6-8, 9+, and 6 variables: X6a, ... X6f. Targeting Characteristic 7, Total Dollars, had 7 categories, as outlined in Table 2, with 6 variables: X7a, ..., X7f 8 (Q1): For each targeting characteristic, the number of categorical/dummy variables is one fewer than the number of categories. Why is this necessary? (Q2): Of the 7 targeting characteristics, three of them (1, 3, and 4) are qualitative/nonnumerical, and must be represented as categorical values. However, the other 4 targeting characteristics are quantitative/numerical in nature and thus can easily be represented by interval-scale/ \"continuous\" variables. Why then did Lester choose to represent them by categorical values? Discuss the advantages and disadvantages of this choice. Lester decided to perform a stepwise regression. (Q3): Should Lester use \"standard\" multiple regression, or did he make a wise choice to use a form of stepwise regression? Discuss the relative merits of each choice. He used a \"pvalue to enter\" of .05, (i.e., bring in a variable only if the variable, upon entering, is significant at a p-value less than or equal to .05), and a \"p-value to be removed\" of .055. (Q4): Why must the p-value to be removed be at least as large as the p-value to enter? 9 The following variables entered the equation: X1a X2c, X2d, X2e, X2f, X2g, X2h, X2i X3a, X3b, X3d, X3h, X3i, X3j, X3k, X3n, X3s, X3y X4a X5c, X5d, X5e, X5f X6c, X6d, X6e, X6f X7c, X7d, X7f (Q5): What is the interpretation of X7c, X7d, and X7f being significant and entering the equation, but X7a, X7b, and X7e not entering the equation? Lester used the regression equation to compute a \"predicted probability of purchase\" for each of the other 32,000 names of the 40,000 names on the original list (from which the 8,000 names used in the regression analysis were culled). He then compared these predicted values with the actual fact of whether each purchased or not. (Q6): Lester had read an article by Denis Sined that suggested that the names that evaluate a prediction equation not include the names from which the regression equation was generated. Do you agree with this suggestion? Why? 10 AFTERMATH The method Lester used to compare the predicted probability of purchase and the actual result of the mailing was to have the 32,000 names arranged in descending order of predicted purchase probability. He then drew up a table showing what he called the \"Pareto Curve,\" the percent of the total purchases of the mailing that each decile of the rank-ordered list garnered. Table 4, below, depicts the results: Table 4-Pareto Curve Top %/decile of rank% of total responses from entire ordered list 32,000 names 25.1* 10 20 44.1 30 58.2 40 69.4 50 78.3 60 85.3 70 91.1 80 96.4 90 98.3 100 100 * For example, the top 10% of the rank-ordered list captured 25.1% of the responses in the total list 11 (Q7): What Graph the curve from the above table. Let % of total response be the vertical axis. would the curve look like had the prediction equation had no value at all? (Q8) What would the curve look like had the prediction been perfect? (Q9): If we were deciding to mail the top portion of the ranked-ordered list, how deeply into the list would we dip if the cost per name to mail is $3 (for postage, brochure, etc.), and the profit per response is assumed to be $72? 12
Step by Step Solution
There are 3 Steps involved in it
Step: 1
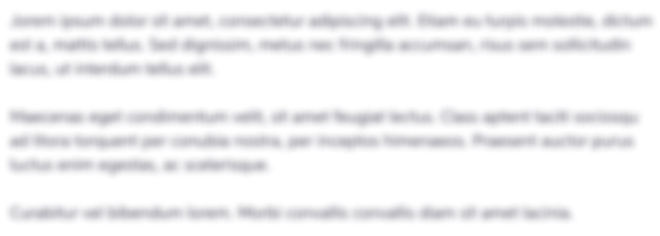
Get Instant Access to Expert-Tailored Solutions
See step-by-step solutions with expert insights and AI powered tools for academic success
Step: 2

Step: 3

Ace Your Homework with AI
Get the answers you need in no time with our AI-driven, step-by-step assistance
Get Started