Answered step by step
Verified Expert Solution
Question
1 Approved Answer
CHAPTER 9 Hypothesis Tests CONTENTS 9.4 POPULATION MEAN: UNKNOWN One-Tailed Test Two-Tailed Test Summary and Practical Advice 9.5 POPULATION PROPORTION Summary 9.6 HYPOTHESIS TESTING AND
CHAPTER 9 Hypothesis Tests CONTENTS 9.4 POPULATION MEAN: UNKNOWN One-Tailed Test Two-Tailed Test Summary and Practical Advice 9.5 POPULATION PROPORTION Summary 9.6 HYPOTHESIS TESTING AND DECISION MAKING 9.7 CALCULATING THE PROBABILITY OF TYPE II ERRORS 9.8 DETERMINING THE SAMPLE SIZE FOR A HYPOTHESIS TEST ABOUT A POPULATION MEAN STATISTICS IN PRACTICE: JOHN MORRELL & COMPANY 9.1 DEVELOPING NULL AND ALTERNATIVE HYPOTHESES The Alternative Hypothesis as a Research Hypothesis The Null Hypothesis as an Assumption to Be Challenged Summary of Forms for Null and Alternative Hypotheses 9.2 TYPE I AND TYPE II ERRORS 9.3 POPULATION MEAN: KNOWN One-Tailed Test Two-Tailed Test Summary and Practical Advice Relationship Between Interval Estimation and Hypothesis Testing Statistics in Practice STATISTICS 349 in PRACTICE JOHN MORRELL & COMPANY* CINCINNATI, OHIO John Morrell & Company, which began in England in 1827, is considered the oldest continuously operating meat manufacturer in the United States. It is a wholly owned and independently managed subsidiary of Smithfield Foods, Smithfield, Virginia. John Morrell & Company offers an extensive product line of processed meats and fresh pork to consumers under 13 regional brands including John Morrell, E-Z-Cut, Tobin's First Prize, Dinner Bell, Hunter, Kretschmar, Rath, Rodeo, Shenson, Farmers Hickory Brand, Iowa Quality, and Peyton's. Each regional brand enjoys high brand recognition and loyalty among consumers. Market research at Morrell provides management with up-to-date information on the company's various products and how the products compare with competing brands of similar products. A recent study compared a Beef Pot Roast made by Morrell to similar beef products from two major competitors. In the three-product comparison test, a sample of consumers was used to indicate how the products rated in terms of taste, appearance, aroma, and overall preference. One research question concerned whether the Beef Pot Roast made by Morrell was the preferred choice of more than 50% of the consumer population. Letting p indicate the population proportion preferring Morrell's product, the hypothesis test for the research question is as follows: H0: p .50 Ha: p .50 The null hypothesis H0 indicates the preference for Morrell's product is less than or equal to 50%. If the *The authors are indebted to Marty Butler, Vice President of Marketing, John Morrell, for providing this Statistics in Practice. Fully cooked entrees allow consumers to heat and serve in the same microwaveable tray. Courtesy of John Morrell's Convenient Cuisine products. sample data support rejecting H0 in favor of the alternative hypothesis Ha , Morrell will draw the research conclusion that in a three-product comparison, their Beef Pot Roast is preferred by more than 50% of the consumer population. In an independent taste test study using a sample of 224 consumers in Cincinnati, Milwaukee, and Los Angeles, 150 consumers selected the Beef Pot Roast made by Morrell as the preferred product. Using statistical hypothesis testing procedures, the null hypothesis H0 was rejected. The study provided statistical evidence supporting Ha and the conclusion that the Morrell product is preferred by more than 50% of the consumer population. The point estimate of the population proportion was p 150/224 .67. Thus, the sample data provided support for a food magazine advertisement showing that in a three-product taste comparison, Beef Pot Roast made by Morrell was \"preferred 2 to 1 over the competition.\" In this chapter we will discuss how to formulate hypotheses and how to conduct tests like the one used by Morrell. Through the analysis of sample data, we will be able to determine whether a hypothesis should or should not be rejected. In Chapters 7 and 8 we showed how a sample could be used to develop point and interval estimates of population parameters. In this chapter we continue the discussion of statistical inference by showing how hypothesis testing can be used to determine whether a statement about the value of a population parameter should or should not be rejected. In hypothesis testing we begin by making a tentative assumption about a population parameter. This tentative assumption is called the null hypothesis and is denoted by H0. We then define another hypothesis, called the alternative hypothesis, which is the opposite of what is stated in the null hypothesis. The alternative hypothesis is denoted by Ha. Chapter 9 350 Hypothesis Tests The hypothesis testing procedure uses data from a sample to test the two competing statements indicated by H0 and Ha. This chapter shows how hypothesis tests can be conducted about a population mean and a population proportion. We begin by providing examples that illustrate approaches to developing null and alternative hypotheses. 9.1 Learning to correctly formulate hypotheses will take some practice. Expect some initial confusion over the proper choice of the null and alternative hypotheses. The examples in this section are intended to provide guidelines. Developing Null and Alternative Hypotheses It is not always obvious how the null and alternative hypotheses should be formulated. Care must be taken to structure the hypotheses appropriately so that the hypothesis testing conclusion provides the information the researcher or decision maker wants. The context of the situation is very important in determining how the hypotheses should be stated. All hypothesis testing applications involve collecting a sample and using the sample results to provide evidence for drawing a conclusion. Good questions to consider when formulating the null and alternative hypotheses are, What is the purpose of collecting the sample? What conclusions are we hoping to make? In the chapter introduction, we stated that the null hypothesis H0 is a tentative assumption about a population parameter such as a population mean or a population proportion. The alternative hypothesis Ha is a statement that is the opposite of what is stated in the null hypothesis. In some situations it is easier to identify the alternative hypothesis first and then develop the null hypothesis. In other situations it is easier to identify the null hypothesis first and then develop the alternative hypothesis. We will illustrate these situations in the following examples. The Alternative Hypothesis as a Research Hypothesis Many applications of hypothesis testing involve an attempt to gather evidence in support of a research hypothesis. In these situations, it is often best to begin with the alternative hypothesis and make it the conclusion that the researcher hopes to support. Consider a particular automobile that currently attains a fuel efficiency of 24 miles per gallon in city driving. A product research group has developed a new fuel injection system designed to increase the miles-per-gallon rating. The group will run controlled tests with the new fuel injection system looking for statistical support for the conclusion that the new fuel injection system provides more miles per gallon than the current system. Several new fuel injection units will be manufactured, installed in test automobiles, and subjected to research-controlled driving conditions. The sample mean miles per gallon for these automobiles will be computed and used in a hypothesis test to determine if it can be concluded that the new system provides more than 24 miles per gallon. In terms of the population mean miles per gallon , the research hypothesis 24 becomes the alternative hypothesis. Since the current system provides an average or mean of 24 miles per gallon, we will make the tentative assumption that the new system is not any better than the current system and choose 24 as the null hypothesis. The null and alternative hypotheses are: H0: 24 Ha: 24 If the sample results lead to the conclusion to reject H0, the inference can be made that Ha: 24 is true. The researchers have the statistical support to state that the new fuel injection system increases the mean number of miles per gallon. The production of automobiles with the new fuel injection system should be considered. However, if the sample results lead to the conclusion that H0 cannot be rejected, the researchers cannot conclude 9.1 The conclusion that the research hypothesis is true is made if the sample data provide sufficient evidence to show that the null hypothesis can be rejected. Developing Null and Alternative Hypotheses 351 that the new fuel injection system is better than the current system. Production of automobiles with the new fuel injection system on the basis of better gas mileage cannot be justified. Perhaps more research and further testing can be conducted. Successful companies stay competitive by developing new products, new methods, new systems, and the like, that are better than what is currently available. Before adopting something new, it is desirable to conduct research to determine if there is statistical support for the conclusion that the new approach is indeed better. In such cases, the research hypothesis is stated as the alternative hypothesis. For example, a new teaching method is developed that is believed to be better than the current method. The alternative hypothesis is that the new method is better. The null hypothesis is that the new method is no better than the old method. A new sales force bonus plan is developed in an attempt to increase sales. The alternative hypothesis is that the new bonus plan increases sales. The null hypothesis is that the new bonus plan does not increase sales. A new drug is developed with the goal of lowering blood pressure more than an existing drug. The alternative hypothesis is that the new drug lowers blood pressure more than the existing drug. The null hypothesis is that the new drug does not provide lower blood pressure than the existing drug. In each case, rejection of the null hypothesis H0 provides statistical support for the research hypothesis. We will see many examples of hypothesis tests in research situations such as these throughout this chapter and in the remainder of the text. The Null Hypothesis as an Assumption to Be Challenged Of course, not all hypothesis tests involve research hypotheses. In the following discussion we consider applications of hypothesis testing where we begin with a belief or an assumption that a statement about the value of a population parameter is true. We will then use a hypothesis test to challenge the assumption and determine if there is statistical evidence to conclude that the assumption is incorrect. In these situations, it is helpful to develop the null hypothesis first. The null hypothesis H0 expresses the belief or assumption about the value of the population parameter. The alternative hypothesis Ha is that the belief or assumption is incorrect. As an example, consider the situation of a manufacturer of soft drink products. The label on a soft drink bottle states that it contains 67.6 fluid ounces. We consider the label correct provided the population mean filling weight for the bottles is at least 67.6 fluid ounces. Without any reason to believe otherwise, we would give the manufacturer the benefit of the doubt and assume that the statement provided on the label is correct. Thus, in a hypothesis test about the population mean fluid weight per bottle, we would begin with the assumption that the label is correct and state the null hypothesis as 67.6. The challenge to this assumption would imply that the label is incorrect and the bottles are being underfilled. This challenge would be stated as the alternative hypothesis 67.6. Thus, the null and alternative hypotheses are: H0: 67.6 Ha: 67.6 A manufacturer's product information is usually assumed to be true and stated as the null hypothesis. The conclusion that the information is incorrect can be made if the null hypothesis is rejected. A government agency with the responsibility for validating manufacturing labels could select a sample of soft drinks bottles, compute the sample mean filling weight, and use the sample results to test the preceding hypotheses. If the sample results lead to the conclusion to reject H0, the inference that Ha: 67.6 is true can be made. With this statistical support, the agency is justified in concluding that the label is incorrect and underfilling of the bottles is occurring. Appropriate action to force the manufacturer to comply with labeling standards would be considered. However, if the sample results indicate H0 cannot be rejected, the assumption that the manufacturer's labeling is correct cannot be rejected. With this conclusion, no action would be taken. 352 Chapter 9 Hypothesis Tests Let us now consider a variation of the soft drink bottle filling example by viewing the same situation from the manufacturer's point of view. The bottle-filling operation has been designed to fill soft drink bottles with 67.6 fluid ounces as stated on the label. The company does not want to underfill the containers because that could result in an underfilling complaint from customers or, perhaps, a government agency. However, the company does not want to overfill containers either because putting more soft drink than necessary into the containers would be an unnecessary cost. The company's goal would be to adjust the bottlefilling operation so that the population mean filling weight per bottle is 67.6 fluid ounces as specified on the label. Although this is the company's goal, from time to time any production process can get out of adjustment. If this occurs in our example, underfilling or overfilling of the soft drink bottles will occur. In either case, the company would like to know about it in order to correct the situation by readjusting the bottle-filling operation to the designed 67.6 fluid ounces. In a hypothesis testing application, we would again begin with the assumption that the production process is operating correctly and state the null hypothesis as 67.6 fluid ounces. The alternative hypothesis that challenges this assumption is that 67.6, which indicates either overfilling or underfilling is occurring. The null and alternative hypotheses for the manufacturer's hypothesis test are: H0: 67.6 Ha: 67.6 Suppose that the soft drink manufacturer uses a quality control procedure to periodically select a sample of bottles from the filling operation and computes the sample mean filling weight per bottle. If the sample results lead to the conclusion to reject H0, the inference is made that Ha: 67.6 is true. We conclude that the bottles are not being filled properly and the production process should be adjusted to restore the population mean to 67.6 fluid ounces per bottle. However, if the sample results indicate H0 cannot be rejected, the assumption that the manufacturer's bottle filling operation is functioning properly cannot be rejected. In this case, no further action would be taken and the production operation would continue to run. The two preceding forms of the soft drink manufacturing hypothesis test show that the null and alternative hypotheses may vary depending upon the point of view of the researcher or decision maker. To correctly formulate hypotheses it is important to understand the context of the situation and structure the hypotheses to provide the information the researcher or decision maker wants. Summary of Forms for Null and Alternative Hypotheses The hypothesis tests in this chapter involve two population parameters: the population mean and the population proportion. Depending on the situation, hypothesis tests about a population parameter may take one of three forms: two use inequalities in the null hypothesis; the third uses an equality in the null hypothesis. For hypothesis tests involving a population mean, we let 0 denote the hypothesized value and we must choose one of the following three forms for the hypothesis test. The three possible forms of hypotheses H0 and Ha are shown here. Note that the equality always appears in the null hypothesis H0. H0: 0 Ha: 0 H0: 0 Ha: 0 H0: 0 Ha: 0 For reasons that will be clear later, the first two forms are called one-tailed tests. The third form is called a two-tailed test. In many situations, the choice of H0 and Ha is not obvious and judgment is necessary to select the proper form. However, as the preceding forms show, the equality part of the 9.2 Type I and Type II Errors 353 expression (either , , or ) always appears in the null hypothesis. In selecting the proper form of H0 and Ha, keep in mind that the alternative hypothesis is often what the test is attempting to establish. Hence, asking whether the user is looking for evidence to support 0, 0, or 0 will help determine Ha. The following exercises are designed to provide practice in choosing the proper form for a hypothesis test involving a population mean. Exercises 1. The manager of the Danvers-Hilton Resort Hotel stated that the mean guest bill for a weekend is $600 or less. A member of the hotel's accounting staff noticed that the total charges for guest bills have been increasing in recent months. The accountant will use a sample of weekend guest bills to test the manager's claim. a. Which form of the hypotheses should be used to test the manager's claim? Explain. H 0: 600 H a: 600 b. c. SELF test H 0: 600 H a: 600 H 0: 600 H a: 600 What conclusion is appropriate when H0 cannot be rejected? What conclusion is appropriate when H0 can be rejected? 2. The manager of an automobile dealership is considering a new bonus plan designed to increase sales volume. Currently, the mean sales volume is 14 automobiles per month. The manager wants to conduct a research study to see whether the new bonus plan increases sales volume. To collect data on the plan, a sample of sales personnel will be allowed to sell under the new bonus plan for a one-month period. a. Develop the null and alternative hypotheses most appropriate for this situation. b. Comment on the conclusion when H0 cannot be rejected. c. Comment on the conclusion when H0 can be rejected. 3. A production line operation is designed to fill cartons with laundry detergent to a mean weight of 32 ounces. A sample of cartons is periodically selected and weighed to determine whether underfilling or overfilling is occurring. If the sample data lead to a conclusion of underfilling or overfilling, the production line will be shut down and adjusted to obtain proper filling. a. Formulate the null and alternative hypotheses that will help in deciding whether to shut down and adjust the production line. b. Comment on the conclusion and the decision when H0 cannot be rejected. c. Comment on the conclusion and the decision when H0 can be rejected. 4. Because of high production-changeover time and costs, a director of manufacturing must convince management that a proposed manufacturing method reduces costs before the new method can be implemented. The current production method operates with a mean cost of $220 per hour. A research study will measure the cost of the new method over a sample production period. a. Develop the null and alternative hypotheses most appropriate for this study. b. Comment on the conclusion when H0 cannot be rejected. c. Comment on the conclusion when H0 can be rejected. 9.2 Type I and Type II Errors The null and alternative hypotheses are competing statements about the population. Either the null hypothesis H0 is true or the alternative hypothesis Ha is true, but not both. Ideally the hypothesis testing procedure should lead to the acceptance of H0 when H0 is true and the 354 Chapter 9 TABLE 9.1 Hypothesis Tests ERRORS AND CORRECT CONCLUSIONS IN HYPOTHESIS TESTING Population Condition H0 True Ha True Accept H0 Correct Conclusion Type II Error Reject H0 Type I Error Correct Conclusion Conclusion rejection of H0 when Ha is true. Unfortunately, the correct conclusions are not always possible. Because hypothesis tests are based on sample information, we must allow for the possibility of errors. Table 9.1 illustrates the two kinds of errors that can be made in hypothesis testing. The first row of Table 9.1 shows what can happen if the conclusion is to accept H0. If H0 is true, this conclusion is correct. However, if Ha is true, we make a Type II error; that is, we accept H0 when it is false. The second row of Table 9.1 shows what can happen if the conclusion is to reject H0. If H0 is true, we make a Type I error; that is, we reject H0 when it is true. However, if Ha is true, rejecting H0 is correct. Recall the hypothesis testing illustration discussed in Section 9.1 in which an automobile product research group developed a new fuel injection system designed to increase the miles-per-gallon rating of a particular automobile. With the current model obtaining an average of 24 miles per gallon, the hypothesis test was formulated as follows. H0: 24 Ha: 24 The alternative hypothesis, Ha: 24, indicates that the researchers are looking for sample evidence to support the conclusion that the population mean miles per gallon with the new fuel injection system is greater than 24. In this application, the Type I error of rejecting H0 when it is true corresponds to the researchers claiming that the new system improves the miles-per-gallon rating ( 24) when in fact the new system is not any better than the current system. In contrast, the Type II error of accepting H0 when it is false corresponds to the researchers concluding that the new system is not any better than the current system ( 24) when in fact the new system improves miles-per-gallon performance. For the miles-per-gallon rating hypothesis test, the null hypothesis is H0: 24. Suppose the null hypothesis is true as an equality; that is, 24. The probability of making a Type I error when the null hypothesis is true as an equality is called the level of significance. Thus, for the miles-per-gallon rating hypothesis test, the level of significance is the probability of rejecting H0: 24 when 24. Because of the importance of this concept, we now restate the definition of level of significance. LEVEL OF SIGNIFICANCE The level of significance is the probability of making a Type I error when the null hypothesis is true as an equality. 9.2 If the sample data are consistent with the null hypothesis H0 , we will follow the practice of concluding \"do not reject H0 .\" This conclusion is preferred over \"accept H0 ,\" because the conclusion to accept H0 puts us at risk of making a Type II error. Type I and Type II Errors 355 The Greek symbol (alpha) is used to denote the level of significance, and common choices for are .05 and .01. In practice, the person responsible for the hypothesis test specifies the level of significance. By selecting , that person is controlling the probability of making a Type I error. If the cost of making a Type I error is high, small values of are preferred. If the cost of making a Type I error is not too high, larger values of are typically used. Applications of hypothesis testing that only control for the Type I error are called significance tests. Many applications of hypothesis testing are of this type. Although most applications of hypothesis testing control for the probability of making a Type I error, they do not always control for the probability of making a Type II error. Hence, if we decide to accept H0, we cannot determine how confident we can be with that decision. Because of the uncertainty associated with making a Type II error when conducting significance tests, statisticians usually recommend that we use the statement \"do not reject H0\" instead of \"accept H0.\" Using the statement \"do not reject H0\" carries the recommendation to withhold both judgment and action. In effect, by not directly accepting H0, the statistician avoids the risk of making a Type II error. Whenever the probability of making a Type II error has not been determined and controlled, we will not make the statement \"accept H0.\" In such cases, only two conclusions are possible: do not reject H0 or reject H0. Although controlling for a Type II error in hypothesis testing is not common, it can be done. In Sections 9.7 and 9.8 we will illustrate procedures for determining and controlling the probability of making a Type II error. If proper controls have been established for this error, action based on the \"accept H0\" conclusion can be appropriate. NOTES AND COMMENTS Walter Williams, syndicated columnist and professor of economics at George Mason University, points out that the possibility of making a Type I or a Type II error is always present in decision making (The Cincinnati Enquirer, August 14, 2005). He notes that the Food and Drug Administration runs the risk of making these errors in their drug approval process. With a Type I error, the FDA fails to approve a drug that is safe and effective. A Type II error means the FDA approves a drug that has unanticipated dangerous side effects. Regardless of the decision made, the possibility of making a costly error cannot be eliminated. Exercises SELF test 5. Nielsen reported that young men in the United States watch 56.2 minutes of prime-time TV daily (The Wall Street Journal Europe, November 18, 2003). A researcher believes that young men in Germany spend more time watching prime-time TV. A sample of German young men will be selected by the researcher and the time they spend watching TV in one day will be recorded. The sample results will be used to test the following null and alternative hypotheses. H0: 56.2 Ha: 56.2 a. b. What is the Type I error in this situation? What are the consequences of making this error? What is the Type II error in this situation? What are the consequences of making this error? 6. The label on a 3-quart container of orange juice states that the orange juice contains an average of 1 gram of fat or less. Answer the following questions for a hypothesis test that could be used to test the claim on the label. a. Develop the appropriate null and alternative hypotheses. Chapter 9 356 b. c. Hypothesis Tests What is the Type I error in this situation? What are the consequences of making this error? What is the Type II error in this situation? What are the consequences of making this error? 7. Carpetland salespersons average $8000 per week in sales. Steve Contois, the firm's vice president, proposes a compensation plan with new selling incentives. Steve hopes that the results of a trial selling period will enable him to conclude that the compensation plan increases the average sales per salesperson. a. Develop the appropriate null and alternative hypotheses. b. What is the Type I error in this situation? What are the consequences of making this error? c. What is the Type II error in this situation? What are the consequences of making this error? 8. Suppose a new production method will be implemented if a hypothesis test supports the conclusion that the new method reduces the mean operating cost per hour. a. State the appropriate null and alternative hypotheses if the mean cost for the current production method is $220 per hour. b. What is the Type I error in this situation? What are the consequences of making this error? c. What is the Type II error in this situation? What are the consequences of making this error? 9.3 Population Mean: Known In Chapter 8 we said that the known case corresponds to applications in which historical data and/or other information are available that enable us to obtain a good estimate of the population standard deviation prior to sampling. In such cases the population standard deviation can, for all practical purposes, be considered known. In this section we show how to conduct a hypothesis test about a population mean for the known case. The methods presented in this section are exact if the sample is selected from a population that is normally distributed. In cases where it is not reasonable to assume the population is normally distributed, these methods are still applicable if the sample size is large enough. We provide some practical advice concerning the population distribution and the sample size at the end of this section. One-Tailed Test One-tailed tests about a population mean take one of the following two forms. Lower Tail Test Upper Tail Test H0: 0 Ha: 0 H0: 0 Ha: 0 Let us consider an example involving a lower tail test. The Federal Trade Commission (FTC) periodically conducts statistical studies designed to test the claims that manufacturers make about their products. For example, the label on a large can of Hilltop Coffee states that the can contains 3 pounds of coffee. The FTC knows that Hilltop's production process cannot place exactly 3 pounds of coffee in each can, even if the mean filling weight for the population of all cans filled is 3 pounds per can. However, as long as the population mean filling weight is at least 3 pounds per can, the rights of consumers will be protected. Thus, the FTC interprets the label information on a large can of coffee as a claim by Hilltop that the population mean filling weight is at least 3 pounds per can. We will show how the FTC can check Hilltop's claim by conducting a lower tail hypothesis test. The first step is to develop the null and alternative hypotheses for the test. If the population mean filling weight is at least 3 pounds per can, Hilltop's claim is correct. This establishes the null hypothesis for the test. However, if the population mean weight is less than 3 pounds per can, Hilltop's claim is incorrect. This establishes the alternative 9.3 Population Mean: Known 357 hypothesis. With denoting the population mean filling weight, the null and alternative hypotheses are as follows: H0: 3 Ha: 3 Note that the hypothesized value of the population mean is 0 3. If the sample data indicate that H0 cannot be rejected, the statistical evidence does not support the conclusion that a label violation has occurred. Hence, no action should be taken against Hilltop. However, if the sample data indicate H0 can be rejected, we will conclude that the alternative hypothesis, Ha: 3, is true. In this case a conclusion of underfilling and a charge of a label violation against Hilltop would be justified. Suppose a sample of 36 cans of coffee is selected and the sample mean x is computed as an estimate of the population mean . If the value of the sample mean x is less than 3 pounds, the sample results will cast doubt on the null hypothesis. What we want to know is how much less than 3 pounds must x be before we would be willing to declare the difference significant and risk making a Type I error by falsely accusing Hilltop of a label violation. A key factor in addressing this issue is the value the decision maker selects for the level of significance. As noted in the preceding section, the level of significance, denoted by , is the probability of making a Type I error by rejecting H0 when the null hypothesis is true as an equality. The decision maker must specify the level of significance. If the cost of making a Type I error is high, a small value should be chosen for the level of significance. If the cost is not high, a larger value is more appropriate. In the Hilltop Coffee study, the director of the FTC's testing program made the following statement: \"If the company is meeting its weight specifications at 3, I do not want to take action against them. But, I am willing to risk a 1% chance of making such an error.\" From the director's statement, we set the level of significance for the hypothesis test at .01. Thus, we must design the hypothesis test so that the probability of making a Type I error when 3 is .01. For the Hilltop Coffee study, by developing the null and alternative hypotheses and specifying the level of significance for the test, we carry out the first two steps required in conducting every hypothesis test. We are now ready to perform the third step of hypothesis testing: collect the sample data and compute the value of what is called a test statistic. Test statistic For the Hilltop Coffee study, previous FTC tests show that the population The standard error of x is the standard deviation of the sampling distribution of x . standard deviation can be assumed known with a value of .18. In addition, these tests also show that the population of filling weights can be assumed to have a normal distribution. From the study of sampling distributions in Chapter 7 we know that if the population from which we are sampling is normally distributed, the sampling distribution of x will also be normally distributed. Thus, for the Hilltop Coffee study, the sampling distribution of x is normally distributed. With a known value of .18 and a sample size of n 36, Figure 9.1 shows the sampling distribution of x when the null hypothesis is true as an equality; that is, when 0 3.1 Note that the standard error of x is given by x n .18 36 .03. Because the sampling distribution of x is normally distributed, the sampling distribution of z 1 x 3 x 0 x .03 In constructing sampling distributions for hypothesis tests, it is assumed that H0 is satisfied as an equality. Chapter 9 358 FIGURE 9.1 Hypothesis Tests SAMPLING DISTRIBUTION OF x FOR THE HILLTOP COFFEE STUDY WHEN THE NULL HYPOTHESIS IS TRUE AS AN EQUALITY ( 3) Sampling distribution of x x = .18 = = .03 n 36 x =3 is a standard normal distribution. A value of z 1 means that the value of x is one standard error below the hypothesized value of the mean, a value of z 2 means that the value of x is two standard errors below the hypothesized value of the mean, and so on. We can use the standard normal probability table to find the lower tail probability corresponding to any z value. For instance, the lower tail area at z 3.00 is .0013. Hence, the probability of obtaining a value of z that is three or more standard errors below the mean is .0013. As a result, the probability of obtaining a value of x that is 3 or more standard errors below the hypothesized population mean 0 3 is also .0013. Such a result is unlikely if the null hypothesis is true. For hypothesis tests about a population mean in the known case, we use the standard normal random variable z as a test statistic to determine whether x deviates from the hypothesized value of enough to justify rejecting the null hypothesis. With x n, the test statistic is as follows. TEST STATISTIC FOR HYPOTHESIS TESTS ABOUT A POPULATION MEAN: KNOWN z x 0 n (9.1) The key question for a lower tail test is, How small must the test statistic z be before we choose to reject the null hypothesis? Two approaches can be used to answer this question: the p-value approach and the critical value approach. p-value approach The p-value approach uses the value of the test statistic z to compute a probability called a p-value. A small p-value indicates the value of the test statistic is unusual given the assumption that H0 is true. p-VALUE A p-value is a probability that provides a measure of the evidence against the null hypothesis provided by the sample. Smaller p-values indicate more evidence against H0. The p-value is used to determine whether the null hypothesis should be rejected. 9.3 WEB file Coffee Population Mean: Known 359 Let us see how the p-value is computed and used. The value of the test statistic is used to compute the p-value. The method used depends on whether the test is a lower tail, an upper tail, or a two-tailed test. For a lower tail test, the p-value is the probability of obtaining a value for the test statistic as small as or smaller than that provided by the sample. Thus, to compute the p-value for the lower tail test in the known case, we must find the area under the standard normal curve for values of z the value of the test statistic. After computing the p-value, we must then decide whether it is small enough to reject the null hypothesis; as we will show, this decision involves comparing the p-value to the level of significance. Let us now compute the p-value for the Hilltop Coffee lower tail test. Suppose the sample of 36 Hilltop coffee cans provides a sample mean of x 2.92 pounds. Is x 2.92 small enough to cause us to reject H0? Because this is a lower tail test, the p-value is the area under the standard normal curve for values of z the value of the test statistic. Using x 2.92, .18, and n 36, we compute the value of the test statistic z. z x 0 n 2.92 3 .1836 2.67 Thus, the p-value is the probability that the test statistic z is less than or equal to 2.67 (the area under the standard normal curve to the left of the test statistic). Using the standard normal probability table, we find that the lower tail area at z 2.67 is .0038. Figure 9.2 shows that x 2.92 corresponds to z 2.67 and a p-value .0038. This p-value indicates a small probability of obtaining a sample mean of x 2.92 (and a test statistic of 2.67) or smaller when sampling from a population with 3. This FIGURE 9.2 p-VALUE FOR THE HILLTOP COFFEE STUDY WHEN x 2.92 AND z 2.67 x = = .03 n x 0 = 3 x x = 2.92 Sampling distribution of z = x - 3 .03 p-value = .0038 z = -2.67 0 z 360 Chapter 9 Hypothesis Tests p-value does not provide much support for the null hypothesis, but is it small enough to cause us to reject H0? The answer depends upon the level of significance for the test. As noted previously, the director of the FTC's testing program selected a value of .01 for the level of significance. The selection of .01 means that the director is willing to tolerate a probability of .01 of rejecting the null hypothesis when it is true as an equality ( 0 3). The sample of 36 coffee cans in the Hilltop Coffee study resulted in a p-value .0038, which means that the probability of obtaining a value of x 2.92 or less when the null hypothesis is true as an equality is .0038. Because .0038 is less than or equal to .01, we reject H0. Therefore, we find sufficient statistical evidence to reject the null hypothesis at the .01 level of significance. We can now state the general rule for determining whether the null hypothesis can be rejected when using the p-value approach. For a level of significance , the rejection rule using the p-value approach is as follows: REJECTION RULE USING p-VALUE Reject H0 if p-value In the Hilltop Coffee test, the p-value of .0038 resulted in the rejection of the null hypothesis. Although the basis for making the rejection decision involves a comparison of the p-value to the level of significance specified by the FTC director, the observed p-value of .0038 means that we would reject H0 for any value of .0038. For this reason, the p-value is also called the observed level of significance. Different decision makers may express different opinions concerning the cost of making a Type I error and may choose a different level of significance. By providing the p-value as part of the hypothesis testing results, another decision maker can compare the reported p-value to his or her own level of significance and possibly make a different decision with respect to rejecting H0. Critical value approach The critical value approach requires that we first determine a value for the test statistic called the critical value. For a lower tail test, the critical value serves as a benchmark for determining whether the value of the test statistic is small enough to reject the null hypothesis. It is the value of the test statistic that corresponds to an area of (the level of significance) in the lower tail of the sampling distribution of the test statistic. In other words, the critical value is the largest value of the test statistic that will result in the rejection of the null hypothesis. Let us return to the Hilltop Coffee example and see how this approach works. In the known case, the sampling distribution for the test statistic z is a standard normal distribution. Therefore, the critical value is the value of the test statistic that corresponds to an area of .01 in the lower tail of a standard normal distribution. Using the standard normal probability table, we find that z 2.33 provides an area of .01 in the lower tail (see Figure 9.3). Thus, if the sample results in a value of the test statistic that is less than or equal to 2.33, the corresponding p-value will be less than or equal to .01; in this case, we should reject the null hypothesis. Hence, for the Hilltop Coffee study the critical value rejection rule for a level of significance of .01 is Reject H0 if z 2.33 In the Hilltop Coffee example, x 2.92 and the test statistic is z 2.67. Because z 2.67 2.33, we can reject H0 and conclude that Hilltop Coffee is underfilling cans. 9.3 FIGURE 9.3 Population Mean: Known 361 CRITICAL VALUE 2.33 FOR THE HILLTOP COFFEE HYPOTHESIS TEST Sampling distribution of x - 0 z= / n = .01 z 0 z = -2.33 We can generalize the rejection rule for the critical value approach to handle any level of significance. The rejection rule for a lower tail test follows. REJECTION RULE FOR A LOWER TAIL TEST: CRITICAL VALUE APPROACH Reject H0 if z z where z is the critical value; that is, the z value that provides an area of in the lower tail of the standard normal distribution. The p-value approach to hypothesis testing and the critical value approach will always lead to the same rejection decision; that is, whenever the p-value is less than or equal to , the value of the test statistic will be less than or equal to the critical value. The advantage of the p-value approach is that the p-value tells us how significant the results are (the observed level of significance). If we use the critical value approach, we only know that the results are significant at the stated level of significance. At the beginning of this section, we said that one-tailed tests about a population mean take one of the following two forms: Lower Tail Test Upper Tail Test H0: 0 Ha: 0 H0: 0 Ha: 0 We used the Hilltop Coffee study to illustrate how to conduct a lower tail test. We can use the same general approach to conduct an upper tail test. The test statistic z is still computed using equation (9.1). But, for an upper tail test, the p-value is the probability of obtaining a value for the test statistic as large as or larger than that provided by the sample. Thus, to compute the p-value for the upper tail test in the known case, we must find the area under the standard normal curve to the right of the test statistic. Using the critical value approach causes us to reject the null hypothesis if the value of the test statistic is greater than or equal to the critical value z ; in other words, we reject H0 if z z . Chapter 9 362 Hypothesis Tests Two-Tailed Test In hypothesis testing, the general form for a two-tailed test about a population mean is as follows: H0: 0 Ha: 0 In this subsection we show how to conduct a two-tailed test about a population mean for the known case. As an illustration, we consider the hypothesis testing situation facing MaxFlight, Inc. The U.S. Golf Association (USGA) establishes rules that manufacturers of golf equipment must meet if their products are to be acceptable for use in USGA events. MaxFlight uses a high-technology manufacturing process to produce golf balls with a mean driving distance of 295 yards. Sometimes, however, the process gets out of adjustment and produces golf balls with a mean driving distance different from 295 yards. When the mean distance falls below 295 yards, the company worries about losing sales because the golf balls do not provide as much distance as advertised. When the mean distance passes 295 yards, MaxFlight's golf balls may be rejected by the USGA for exceeding the overall distance standard concerning carry and roll. MaxFlight's quality control program involves taking periodic samples of 50 golf balls to monitor the manufacturing process. For each sample, a hypothesis test is conducted to determine whether the process has fallen out of adjustment. Let us develop the null and alternative hypotheses. We begin by assuming that the process is functioning correctly; that is, the golf balls being produced have a mean distance of 295 yards. This assumption establishes the null hypothesis. The alternative hypothesis is that the mean distance is not equal to 295 yards. With a hypothesized value of 0 295, the null and alternative hypotheses for the MaxFlight hypothesis test are as follows: H0: 295 Ha: 295 If the sample mean x is significantly less than 295 yards or significantly greater than 295 yards, we will reject H0. In this case, corrective action will be taken to adjust the manufacturing process. On the other hand, if x does not deviate from the hypothesized mean 0 295 by a significant amount, H0 will not be rejected and no action will be taken to adjust the manufacturing process. The quality control team selected .05 as the level of significance for the test. Data from previous tests conducted when the process was known to be in adjustment show that the population standard deviation can be assumed known with a value of 12. Thus, with a sample size of n 50, the standard error of x is x WEB file GolfTest n 12 50 1.7 Because the sample size is large, the central limit theorem (see Chapter 7) allows us to conclude that the sampling distribution of x can be approximated by a normal distribution. Figure 9.4 shows the sampling distribution of x for the MaxFlight hypothesis test with a hypothesized population mean of 0 295. Suppose that a sample of 50 golf balls is selected and that the sample mean is x 297.6 yards. This sample mean provides support for the conclusion that the population mean is larger than 295 yards. Is this value of x enough larger than 295 to cause us to reject H0 at the .05 level of significance? In the previous section we described two approaches that can be used to answer this question: the p-value approach and the critical value approach. 9.3 FIGURE 9.4 Population Mean: Known 363 SAMPLING DISTRIBUTION OF x FOR THE MAXFLIGHT HYPOTHESIS TEST x x = = n 12 = 1.7 50 x 0 = 295 p-value approach Recall that the p-value is a probability used to determine whether the null hypothesis should be rejected. For a two-tailed test, values of the test statistic in either tail provide evidence against the null hypothesis. For a two-tailed test, the p-value is the probability of obtaining a value for the test statistic as unlikely as or more unlikely than that provided by the sample. Let us see how the p-value is computed for the MaxFlight hypothesis test. First we compute the value of the test statistic. For the known case, the test statistic z is a standard normal random variable. Using equation (9.1) with x 297.6, the value of the test statistic is z x 0 n 297.6 295 1250 1.53 Now to compute the p-value we must find the probability of obtaining a value for the test statistic at least as unlikely as z 1.53. Clearly values of z 1.53 are at least as unlikely. But, because this is a two-tailed test, values of z 1.53 are also at least as unlikely as the value of the test statistic provided by the sample. In Figure 9.5, we see that the two-tailed p-value in this case is given by P(z 1.53) P(z 1.53). Because the FIGURE 9.5 p-VALUE FOR THE MAXFLIGHT HYPOTHESIS TEST P(z 1.53) = .0630 P(z -1.53) = .0630 -1.53 0 p-value = 2(.0630) = .1260 1.53 z 364 Chapter 9 Hypothesis Tests normal curve is symmetric, we can compute this probability by finding the area under the standard normal curve to the right of z 1.53 and doubling it. The table for the standard normal distribution shows that the area to the left of z 1.53 is .9370. Thus, the area under the standard normal curve to the right of the test statistic z 1.53 is 1.0000 .9370 .0630. Doubling this, we find the p-value for the MaxFlight two-tailed hypothesis test is p-value 2(.0630) .1260. Next we compare the p-value to the level of significance to see whether the null hypothesis should be rejected. With a level of significance of .05, we do not reject H0 because the p-value .1260 .05. Because the null hypothesis is not rejected, no action will be taken to adjust the MaxFlight manufacturing process. The computation of the p-value for a two-tailed test may seem a bit confusing as compared to the computation of the p-value for a one-tailed test. But it can be simplified by following three steps. COMPUTATION OF p-VALUE FOR A TWO-TAILED TEST 1. Compute the value of the test statistic z. 2. If the value of the test statistic is in the upper tail (z 0), find the area under the standard normal curve to the right of z. If the value of the test statistic is in the lower tail (z 0), find the area under the standard normal curve to the left of z. 3. Double the tail area, or probability, obtained in step 2 to obtain the p-value. Critical value approach Before leaving this section, let us see how the test statistic z can be compared to a critical value to make the hypothesis testing decision for a two-tailed test. Figure 9.6 shows that the critical values for the test will occur in both the lower and upper tails of the standard normal distribution. With a level of significance of .05, the area in each tail beyond the critical values is /2 .05/2 .025. Using the standard normal probability table, we find the critical values for the test statistic are z.025 1.96 and z.025 1.96. Thus, using the critical value approach, the two-tailed rejection rule is Reject H0 if z 1.96 or if z 1.96 Because the value of the test statistic for the MaxFlight study is z 1.53, the statistical evidence will not permit us to reject the null hypothesis at the .05 level of significance. FIGURE 9.6 CRITICAL VALUES FOR THE MAXFLIGHT HYPOTHESIS TEST Area = .025 Area = .025 -1.96 Reject H0 0 1.96 Reject H0 z 9.3 TABLE 9.2 Population Mean: Known 365 SUMMARY OF HYPOTHESIS TESTS ABOUT A POPULATION MEAN: KNOWN CASE Lower Tail Test Upper Tail Test Two-Tailed Test Hypotheses H 0 : 0 Ha: 0 H 0 : 0 Ha: 0 H 0 : 0 Ha: 0 Test Statistic z Rejection Rule: p-Value Approach Reject H0 if p-value Reject H0 if p-value Reject H0 if p-value Rejection Rule: Critical Value Approach Reject H0 if z z Reject H0 if z z Reject H0 if z z/2 or if z z/2 x 0 n z x 0 n z x 0 n Summary and Practical Advice We presented examples of a lower tail test and a two-tailed test about a population mean. Based upon these examples, we can now summarize the hypothesis testing procedures about a population mean for the known case as shown in Table 9.2. Note that 0 is the hypothesized value of the population mean. The hypothesis testing steps followed in the two examples presented in this section are common to every hypothesis test. STEPS OF HYPOTHESIS TESTING Step 1. Develop the null and alternative hypotheses. Step 2. Specify the level of significance. Step 3. Collect the sample data and compute the value of the test statistic. p-Value Approach Step 4. Use the value of the test statistic to compute the p-value. Step 5. Reject H0 if the p-value . Critical Value Approach Step 4. Use the level of significance to determine the critical value and the rejection rule. Step 5. Use the value of the test statistic and the rejection rule to determine whether to reject H0. Practical advice about the sample size for hypothesis tests is similar to the advice we provided about the sample size for interval estimation in Chapter 8. In most applications, a sample size of n 30 is adequate when using the hypothesis testing procedure described in this section. In cases where the sample size is less than 30, the distribution of the population from which we are sampling becomes an important consideration. If the population is normally distributed, the hypothesis testing procedure that we described is exact and can be used for any sample size. If the population is not normally distributed but is at least roughly symmetric, sample sizes as small as 15 can be expected to provide acceptable results. 366 Chapter 9 Hypothesis Tests Relationship Between Interval Estimation and Hypothesis Testing In Chapter 8 we showed how to develop a confidence interval estimate of a population mean. For the known case, the (1 )% confidence interval estimate of a population mean is given by x z/2 n In this chapter, we showed that a two-tailed hypothesis test about a population mean takes the following form: H0: 0 Ha: 0 where 0 is the hypothesized value for the population mean. Suppose that we follow the procedure described in Chapter 8 for constructing a 100(1 )% confidence interval for the population mean.We know that 100(1 )% of the confidence intervals generated will contain the population mean and 100% of the confidence intervals generated will not contain the population mean. Thus, if we reject H0 whenever the confidence interval does not contain 0, we will be rejecting the null hypothesis when it is true ( 0) with probability . Recall that the level of significance is the probability of rejecting the null hypothesis when it is true. So constructing a 100(1 )% confidence interval and rejecting H0 whenever the interval does not contain 0 is equivalent to conducting a two-tailed hypothesis test with as the level of significance. The procedure for using a confidence interval to conduct a two-tailed hypothesis test can now be summarized. A CONFIDENCE INTERVAL APPROACH TO TESTING A HYPOTHESIS OF THE FORM H0: 0 Ha: 0 1. Select a simple random sample from the population and use the value of the sample mean x to develop the confidence interval for the population mean . x z/2 For a two-tailed hypothesis test, the null hypothesis can be rejected if the confidence interval does not include 0. n 2. If the confidence interval contains the hypothesized value 0, do not reject H0. Otherwise, reject2 H0. Let us illustrate by conducting the MaxFlight hypothesis test using the confidence interval approach. The MaxFlight hypothesis test takes the following form: H0: 295 Ha: 295 2 To be consistent with the rule for rejecting H0 when the p-value , we would also reject H0 using the confidence interval approach if 0 happens to be equal to one of the end points of the 100(1 )% confidence interval. 9.3 Population Mean: Known 367 To test these hypotheses with a level of significance of .05, we sampled 50 golf balls and found a sample mean distance of x 297.6 yards. Recall that the population standard deviation is 12. Using these results with z.025 1.96, we find that the 95% confidence interval estimate of the population mean is n 12 297.6 1.96 50 297.6 3.3 x z.025 or 294.3 to 300.9 This finding enables the quality control manager to conclude with 95% confidence that the mean distance for the population of golf balls is between 294.3 and 300.9 yards. Because the hypothesized value for the population mean, 0 295, is in this interval, the hypothesis testing conclusion is that the null hypothesis, H0: 295, cannot be rejected. Note that this discussion and example pertain to two-tailed hypothesis tests about a population mean. However, the same confidence interval and two-tailed hypothesis testing relationship exists for other population parameters. The relationship can also be extended to one-tailed tests about population parameters. Doing so, however, requires the development of one-sided confidence intervals, which are rarely used in practice. NOTES AND COMMENTS We have shown how to use p-values. The smaller the p-value the greater the evidence against H0 and the more the evidence in favor of Ha. Here are some guidelines statisticians suggest for interpreting small p-values. Less than .01Overwhelming evidence to conclude Ha is true. Between .01 and .05Strong evidence to conclude Ha is true. Between .05 and .10Weak evidence to conclude Ha is true. Greater than .10Insufficient evidence to conclude Ha is true. Exercises Note to Student: Some of the exercises that follow ask you to use the p-value approach and others ask you to use the critical value approach. Both methods will provide the same hypothesis testing conclusion. We provide exercises with both methods to give you practice using both. In later sections and in following chapters, we will generally emphasize the p-value approach as the preferred method, but you may select either based on personal preference. Methods 9. Consider the following hypothesis test: H 0: 20 H a: 20 Chapter 9 368 Hypothesis Tests A sample of 50 provided a sample mean of 19.4. The population standard deviation is 2. a. Compute the value of the test statistic. b. What is the p-value? c. Using .05, what is your conclusion? d. What is the rejection rule using the critical value? What is your conclusion? SELF test 10. Consider the following hypothesis test: H 0: 25 H a: 25 A sample of 40 provided a sample mean of 26.4. The population standard deviation is 6. a. Compute the value of the test statistic. b. What is the p-value? c. At .01, what is your conclusion? d. What is the rejection rule using the critical value? What is your conclusion? SELF test 11. Consider the following hypothesis test: H 0: 15 H a: 15 A sample of 50 provided a sample mean of 14.15. The population standard deviation is 3. a. Compute the value of the test statistic. b. What is the p-value? c. At .05, what is your conclusion? d. What is the rejection rule using the critical value? What is your conclusion? 12. Consider the following hypothesis test: H 0: 80 H a: 80 A sample of 100 is used and the population standard deviation is 12. Compute the p-value and state your conclusion for each of the following sample results. Use .01. a. x 78.5 b. x 77 c. x 75.5 d. x 81 13. Consider the following hypothesis test: H 0: 50 H a: 50 A sample of 60 is used and the population standard deviation is 8. Use the critical value approach to state your conclusion for each of the following sample results. Use .05. a. x 52.5 b. x 51 c. x 51.8 14. Consider the following hypothesis test: H 0: 22 H a: 22 9.3 Population Mean: Known 369 A sample of 75 is used and the population standard deviation is 10. Compute the p-value and state your conclusion for each of the following sample results. Use .01. a. x 23 b. x 25.1 c. x 20 Applications SELF test 15. Individuals filing federal income tax returns prior to March 31 received an average refund of $1056. Consider the population of \"last-minute\" filers who mail their tax return during the last five days of the income tax period (typically April 10 to April 15). a. A researcher suggests that a reason individuals wait until the last five days is that on average these individuals receive lower refunds than do early filers. Develop appropriate hypotheses such that rejection of H0 will support the researcher's contention. b. For a sample of 400 individuals who filed a tax return between April 10 and 15, the sample mean refund was $910. Based on prior experience a population standard deviation of $1600 may be assumed. What is the p-value? c. At .05, what is your conclusion? d. Repeat the preceding hypothesis test using the critical value approach. 16. In a study entitled How Undergraduate Students Use Credit Cards, it was reported that undergraduate students have a mean credit card balance of $3173 (Sallie Mae, April 2009). This figure was an all-time high and had increased 44% over the previous five years. Assume that a current study is being conducted to determine if it can be concluded that the mean credit card balance for undergraduate students has continued to increase compared to the April 2009 report. Based on previous studies, use a population standard deviation $1000. a. State the null and alternative hypotheses. b. What is the p-value for a sample of 180 undergraduate students with a sample mean credit card balance of $3325? c. Using a .05 level of significance, what is your conclusion? 17. Wall Street securities firms paid out record year-end bonuses of $125,500 per employee for 2005 (Fortune, February 6, 2006). Suppose we would like to take a sample of employees at the Jones & Ryan securities firm to see whether the mean year-end bonus is different from the reported mean of $125,500 for the population. a. State the null and alternative hypotheses you would use to test whether the year-end bonuses paid by Jones & Ryan were different from the population mean. b. Suppose a sample of 40 Jones & Ryan employees showed a sample mean year-end bonus of $118,000. Assume a population standard deviation of $30,000 and compute the p-value. c. With .05 as the level of significance, what is your conclusion? d. Repeat the preceding hypothesis test using the critical value approach. 18. The average annual total return for U.S. Diversified Equity mutual funds from 1999 to 2003 was 4.1% (BusinessWeek, January 26, 2004). A researcher would like to conduct a hypothesis test to see whether the returns for mid-cap growth funds over the same period are significantly different from the average for U.S. Diversified Equity funds. a. Formulate the hypotheses that can be used to determine whether the mean annual return for mid-cap growth funds differ from the mean for U.S. Diversified Equity funds. b. A sample of 40 mid-cap growth funds provides a mean return of x 3.4%. Assume the population standard deviation for mid-cap growth funds is known from previous studies to be 2%. Use the sample results to compute the test statistic and p-value for the hypothesis test. c. At .05, what is your conclusion? Chapter 9 370 Hypothesis Tests 19. The U.S. Department of Labor reported the average hourly earnings for U.S. production workers to be $14.32 per hour in 2001 (The World Almanac, 2003). A sample of 75 production workers during 2003 showed a sample mean of $14.68 per hour. Assuming the population standard deviation $1.45, can we conclude that an increase occurred in the mean hourly earnings since 2001? Use .05. 20. For the United States, the mean monthly Internet bill is $32.79 per household (CNBC, January 18, 2006). A sample of 50 households in a southern state showed a sample mean of $30.63. Use a population standard deviation of $5.60. a. Formulate hypotheses for a test to determine whether the sample data support the conclusion that the mean monthly Internet bill in the southern state is less than the national mean of $32.79. b. What is the value of the test statistic? c. What is the p-value? d. At .01, what is your conclusion? WEB file Fowle 21. Fowle Marketing Research, Inc., bases charges to a client on the assumption that telephone surveys can be completed in a mean time of 15 minutes or less. If a longer mean survey time is necessary, a premium rate is charged. A sample of 35 surveys provided the survey times shown in the file named Fowle. Based upon past studies, the population standard deviation is assumed known with 4 minutes. Is the premium rate justified? a. Formulate the null and alternative hypotheses for this application. b. Compute the value of the test statistic. c. What is the p-value? d. At .01, what is your conclusion? 22. CCN and ActMedia provided a television channel targeted to individuals waiting in supermarket checkout lines. The channel showed news, short features, and advertisements. The length of the program was based on the assumption that the population mean time a shopper stands in a supermarket checkout line is 8 minutes. A sample of actual waiting times will be used to test this assumption and determine whether actual mean waiting time differs from this standard. a. Formulate the hypotheses for this application. b. A sample of 120 shoppers showed a sample mean waiting time of 8.5 minutes. Assume a population standard deviation of 3.2 minutes. What is the p-value? c. At .05, what is your conclusion? d. Compute a 95% confidence interval for the population mean. Does it support your conclusion? 9.4 Population Mean: Unknown In this section we describe how to conduct hypothesis tests about a population mean for the unknown case. Because the unknown case corresponds to situations in which an estimate of the population standard deviation cannot be developed prior to sampling, the sample must be used to develop an estimate of both and . Thus, to conduct a hypothesis test about a population mean for the unknown case, the sample mean x is used as an estimate of and the sample standard deviation s is used as an estimate of . The steps of the hypothesis testing procedure for the unknown case are the same as those for the known case described in Section 9.3. But, with unknown, the computation of the test statistic and p-value is a bit different. Recall that for the known case, the sampling distribution of the test statistic has a standard normal distribution. For the unknown case, however, the sampling distribution of the test statistic follows the t distribution; it has slightly more variability because the sample is used to develop estimates of both and . 9.4 Population Mean: Unknown 371 In Section 8.2 we showed that an interval estimate of a population mean for the unknown case is based on a probability distribution known as the t distribution. Hypothesis tests about a population mean for the unknown case are also based on the t distribution. For the unknown case, the test statistic has a t distribution with n 1 degrees of freedom. TEST STATISTIC FOR HYPOTHESIS TEST
Step by Step Solution
There are 3 Steps involved in it
Step: 1
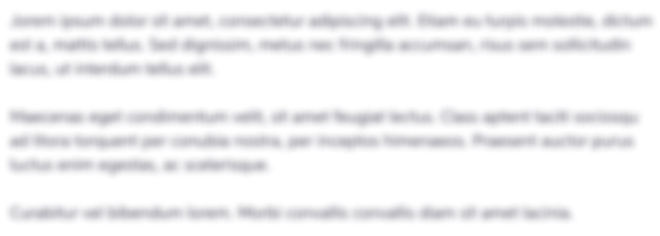
Get Instant Access to Expert-Tailored Solutions
See step-by-step solutions with expert insights and AI powered tools for academic success
Step: 2

Step: 3

Ace Your Homework with AI
Get the answers you need in no time with our AI-driven, step-by-step assistance
Get Started