Answered step by step
Verified Expert Solution
Question
1 Approved Answer
class, we conside optimizing an Square Error (MSE) loss. practice, there are other choices of loss functions as well. For this problem, we will

class, we conside optimizing an Square Error (MSE) loss. practice, there are other choices of loss functions as well. For this problem, we will consider linear regression using Mean Absolute Error (MAE) as our loss function. Specifically, the MAE loss is given as: JMAE (0) = n 22 i=1 xy). (1) 1 (a) [4 points] Derive the partial derivative MAE() Hint: For this question, you don't need to worry about J=0. You can use the sign function s.t. sign(y-y) +1 y, otherwise." Solution: -1 . (0) 0, 21 = - 00; n 00; n sign(0x)-y)(0x) - y()) sign(0x-y) (b) [2 points] Write the vectorized solution for the gradient of the loss function, i.e., VeJMAE (0). Solution: VeJMAE(0) = 1 sign(x)-y)x() (c) [4 points] Given the following dataset of 8 points, what is the value of of JMAE(0) and JMSE (0) at 0 [1.0, 1.0, 1.0]? How about their gradients Ve.JMAE(0) and V.JMSE (0)? 2 3 4 5 6 7 8 [4,0] [1,1] [0,1] -2,-2] -2,1] [1,0] [1,0] [5,2] [3,0] 12 3 1 6 3 6 8 7 Solution: JMAR (A) 3.375 MSE(A) 10.3125.
Step by Step Solution
There are 3 Steps involved in it
Step: 1
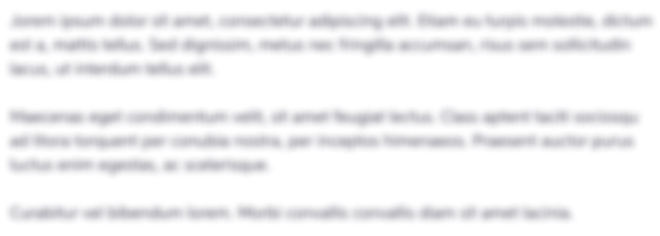
Get Instant Access to Expert-Tailored Solutions
See step-by-step solutions with expert insights and AI powered tools for academic success
Step: 2

Step: 3

Ace Your Homework with AI
Get the answers you need in no time with our AI-driven, step-by-step assistance
Get Started