Answered step by step
Verified Expert Solution
Question
1 Approved Answer
Could you please explain the findings of the study? A systematic review shows no performance benefit of machine learning over logistic regression for clinical prediction
Could you please explain the findings of the study?
A systematic review shows no performance benefit of machine learning over logistic regression for clinical prediction models Evangelia Christodoulou a, Jie Mab, Gary S. Collins b,c, Ewout W. Steyerberg d, Jan Y. Verbakel a,e,f, Ben Van Calster a,d, "Deparbment of Devebpment \& Regene rution, KU Leuven, Herestruat 49 bex 805 , Lewen, 3000 Belgium Oxforl, Windmill Road, Oxforl, OX3 7LD UK 'Oxjord Lhiversity Hospitads NHS Foundation Trust, Oxfard, UK d Department of Biomedical Dota Sciences, Levlen University Medical Centre, Allinusdreef 2, Leiden, 2333 ZA The Netherhands 'Department of Public Health \& Primary Care, KU Leuven, Kapucijnenvoer 33J box 7001, Leaven, 3000 Belgium 4 Nuffield Deparment of Primary Care Health Sciences, Lhivensity of OtJrd, Woodsock Rand, Otord OX2 6GG UK Accepted 5 February 2019; Published online 11 February 2019 Abstract Objectives: The objective of this study was to compare performance of logistic regression (LR) with machine learning (ML) for clinical prediction modeling in the literature. Study Design and Setting: We conducted a Medline literature search (1/2016 to 8/2017) and extracted comparisons between LR and ML models for binary outcomes. Results: We included 71 of 927 studies. The median sample size was 1,250 (range 723,994,872 ), with 19 predictors considered (range 5-563) and eight events per predictor (range 0.3-6,697). The most common ML methods were classification trees, random forests, artificial neural networks, and support vector machines. In 48(68%) studies, we observed potential bias in the validation procedures. Sixty-four (90%) studies used the area under the receiver operating characteristic curve (AUC) to assess discrimination. Calibration was not addressed in 56(79% ) studies. We identified 282 comparisons between an LR and ML model (AUC range, 0.520.99 ). For 145 comparisons at low risk of bias, the difference in logit(AUC) between LR and ML was 0.00 ( 95% confidence interval, 0.18 to 0.18 ). For 137 comparisons at high risk of bias, logit(AUC) was 0.34(0.200.47) higher for ML. Condusion: We found no evidence of superior performance of ML over LR. Improvements in methodology and reporting are needed for studies that compare modeling algorithms. 2019 Elsevier Inc. All rights reserved. Keyworls: Clinical prediction model;; Logistic regression; Machine learning; AUC; Calibration; Reporting 1. Introduction prediction and classification problems. ML methods Clinical risk prediction models are ubiquitous in many include artificial neural networks, support vector machines, medical domains. These models aim to predict a clinically and random forests [2]. Although ML methods have been relevant outcome using person-level information. The tradi- sporadically used for clinical prediction for some time tional approach to develop these models involves the use of [3,4], the growing availability of increasingly large, voluregression models, for example, logistic regression (LR) to minous, and rich data sets such as electronic health records regression models, for example, logistic regression(LR) to data have reignited interest in exploiting these methods predict disease presence (diagnosis) or disease outcomes [57]. (prognosis) [1]. Machine learning (ML) algorithms are Definitions of what constitutes ML and the differences gaining in popularity as an alternative approach for with statistical modelinghave been discussed at length in the literature [8], yet the distinction is not clear-cut [9]. The seminal reference on this issue is Breiman's review * Corresponding author. Tel.: +3216377788; fax: +3216344205. of the "two cultures" [8]. Breiman contrasts theoryE-mail address: ben.vancakter@kuleuven.be (B. Van Calster). based models such as regression with empirical https // doi.org/10.1016/j.jclinepi.2019.02.004 0896435602019 Esevier Inc. All rights reserved. What is new? as ML [13], such as penalized regression (e.g., LASSO, elastic net) or generalized additive models (GAM). We note that these methods do not belong to ML using the "autoKey Findings matic learning from data" definition, and did not classify - Applied studies comparing clinical prediction these as ML in this study. models based on logistic regression and machine Owing to its flexibility, ML is claimed to have better learning algorithms suffered from poor methodol- performance over traditional statistical modeling, and to ogy and reporting, in particular, with respect to better handle a larger number of potential predictors the validation procedure. [57,12,1416]. However, recent research suggested that - The studies rarely assessed whether risk predic- ML requires more data than LR, which contradicts the tions are reliable (calibration), but the area under above claim [17]. Furthermore, ML models are typically the receiver operating characteristic curve (AUC) assessed in terms of discrimination performance (e.g., accuwas almost always provided. racy, area under the receiver operating characteristic [ROC] - The AUC of logistic regression and machine bration) is often not assessed [18]. The claim of improved learning models for clinical risk prediction were performance in clinical prediction is therefore not similar when comparisons were at low risk of bias; established. machine learning (ML) performance was higher in The primary objective of this study was to compare the comparisons that were at high risk of bias. performance of LR with ML algorithms for the developWhat this adds to what was known? ment of diagnostic or prognostic clinical prediction models - ML models do not automatically lead to improved jectives were to describe the characteristics of the studies, performance over traditional methods. the type of ML algorithms that were used, the validation - Model validation procedures are often not sound or process, the modeling aspects of LR and ML, reporting not well reported, which hampers a fair model quality, and risk of bias for comparing performance becomparison in real-world case studies. tween regression and ML [19]. What is the implication and what should change now? 2. Materials and methods - More attention for calibration performance of The study was registered with PROSPERO regression and ML models is urgently needed. (CRD42018068587). We followed the Preferred Reporting - Model development and validation methodologies Items for Systematic reviews and Meta-Analysis (PRISshould be more carefully designed and reported MA) statement. to avoid research waste. - Research should focus more on identifying which 2.1. Identification of studies algorithms have optimal performance for different We searched Medline on August 8th, 2017. We pertypes of prediction problems. formed a sensitive literature search by using a broad working definition of ML (see the search string in Appendix A). We focused on articles published since 2016 (between January 1st, 2016, and August 8th, 2017) to base our analalgorithms such as decision trees, artificial neural networks, ysis on recent studies. support vector machines, or random forests. A useful definition of ML is that it focuses on models that directly and automatically learn from data [10]. By contrast, regression 2.2. Selection of studies models are based on theory and assumptions, and benefit All abstracts were independently screened by two refrom human intervention and subject knowledge for model viewers (E.C. and J.M.); conflicts were resolved by a third specification. For example, ML performs modeling more reviewer (B.V.C. or J.Y.V.). The full text of selected abautomatically than regression regarding the inclusion of stracts were independently assessed for eligibility by three nonlinear associations and interaction terms [11]. To do reviewers (E.C., J.M., B.V.C.), and conflicts were resolved so, ML algorithms are often highly flexible algorithms that by consensus. require penalization to avoid overfitting [12]. Some researchers describe the distinction between statistical modeling and ML as a continuum [5]. Other researchers la- 2.3. Inclusion and exclusion criteria bel any method that deviates from basic regression models Studies were eligible if the article - described the development of a diagnostic or prog- 2.5. Data analysis nostic prediction model for individualized prediction We used descriptive statistics to summarize results. using two or more predictors, - compared prediction models based on LR and ML Within each article, we identified all comparisons between algorithms. LR and ML methods (see Appendix C). We identified mul- Studies were excluded if tiple comparisons within the same article as a result of implementing multiple ML algorithms, developing models for - a new modeling approach was introduced (hence a more than one outcome, developing models based on methodological focus) [20,21], different predictor sets (e.g., once with and once without - models were developed for nonhumans, laboratory measurements), or developing models for - the models made predictions for individual images or several subgroups separately. Although the search string signals rather than participants, contrasted standard LR with penalized methods, we - models were developed based on high-dimensional consider penalized LR (e.g., lasso, ridge, elastic net) to data modalities, be LR rather than ML. Some articles contrasted LR with al- - the primary interest was assessing risk factors rather gorithms that are traditional statistical methods, such as than prediction modeling, discriminant analysis, Poisson regression, generalized esti- - they were reviews of the literature, mating equations, and GAM. We did not classify these al- - studies for which we were unable to obtain the full gorithms as ML. We compared the LR and ML models text. the following order of priority: external validation, internal validation, and training data (no validation). Based on the extracted data, we classified ML algorithms into five broad 2.4. Data extraction and risk of bias groups: single classification trees, random forests, artificial We focused on methodological issues of model develop- neural networks, support vector machines, and other algoment and aspects that compromise the comparison of model rithms. We analyzed AUC differences for all comparisons performance between LR and ML algorithms. The list of and with stratification for risk of bias. We performed a extraction items was based on the CHARMS checklist and meta-regression of the difference between logitthe QUADAS risk of bias tool and refined after extensive dis- transformed AUC using a random effect model to take cluscussion among the authors [9,22]. The extracted items tering of comparisons by article into account, and weighted included general study characteristics, applied algorithms by the square root of the validation sample size. Logiand their characteristics, data-driven variable selection, and t(AUC) was used to circumvent the bounded nature of the model performance (Table A.1, Appendix B) [1,2,13,2325]. AUC [26]. From each article, we defined five signaling items to indicate potential bias. We elaborate on these items in Table A.2: 3. Results (1) unclear or biased validation of model performance, Our search identified 927 articles published since be- (2) difference in whether data-driven variable selection tween 1/2016 and 8/2017, of which 802 studies were was performed (yeso) before applying LR and excluded based on title or abstract (Fig. 1). Fifty-four ML algorithms, studies were excluded during full-text screening. Seventy- (3) difference in handling of continuous variables before one studies met inclusion criteria and came from a wide vaapplying LR and ML algorithms, riety of clinical domains, with oncology and cardiovascular (4) different predictors considered for LR and ML medicine as the most common (Table A.3-4) [27-97]. algorithms, (5) whether corrections for imbalanced outcomes where 3.1. General study characteristics used only for LR or only for ML algorithms. The most common designs were cohort (n=39,55%) Most articles developed several LR and/or ML models. and cross-sectional ( n=18,25% ) (Table A.5). Overall, These articles contain multiple comparisons between LR and 50 studies (70%) focused on prognostic outcomes, 19 ML algorithms, and we evaluated the signaling items per com- (27%) on diagnostic outcomes, and two on both. Most parison. Each bias item was scored as no (not present), unclear, studies (n=64,90% ) used existing data, and 27 ( 38% ) or yes (present). We considered a comparison at low risk of bias used hospital-based multicenter data. The median number if the answer was "no" for all five signaling items. If the answer of centers was five (range 2-1,137) (Table A.6). was "unclear" or "yes" for at least one item, we assumed high The median total sample size was 1,250 (range risk of bias. We also summarized the signaling items for each 723,994,872 ), median number of considered predictors study as a whole, by noting the worst case (no, unclear, yes) was 19 (range, 5-563). One hundred and two outcomes across all comparisons in the study. were considered in the 71 articles, the median event rate E. Christodoulou ef al / Joumal of Clinical Epidemiobgy 110 (2019) 12-22 15 Fig. 1. PRISMA flowchart. PRISMA, preferred reporting items for systematic reviews and meta-analysis. was 0.18 (range 0.0020.50 ). We defined the number of machines were used, the Gaussian ("radial basis function") events as the number of participants in the smallest kernel was most often used (n=10). outcome category. Nine articles developed models to predict more than one outcome. The median number of events per predictor in the training data was 8 (range 0.36,697 ) 3.3. Model development (Fig. A.1). Irrespective of algorithm (L.R. vs. M.L.), 14 studies Information on handling of missing data was lacking or (20%) were not clear about how continuous variables were unclear in 32 studies (45\%) (Tables A.7-8). Sixteen studies handled during model development (Table A.10). Discreti(23\%) performed a complete case analysis, 14(20%) relied zation (into two or more categories) was used for some or on ad hoc methods (mean imputation, missing indicator all algorithms in 18 studies (25\%), whereas continuous methods, variable deletion), and nine (11%) used single modeling was observed in 37 studies (52\%), although this or multiple stochastic imputation, albeit poorly was often not explicitly stated. Data-driven variable selecdocumented. tion before any model fitting was reported for 41 studies (58%) Specifically for LR, handling of continuous predictors 3.2. Overview of algorithms was unclear in 47/71 studies (66\%). In 33/47, some or all -four studies used standard (maximum likelihood) nonlinear associations were examined. For one study, it was LR, of which nine also used penalized LR (lasso, ridge, clear that continuous variables were assumed to have linear or elastic net) and one also used boosted LR (Table 1 and associations with the outcome. Discretization of some or all Table A.9). Six studies used only penalized LR, and one continuous predictors was carried out in 20 studies (28\%), study used only bagged LR (classified as ML). whereas nonlinearity was investigated in seven studies Forty-three studies used more than 1ML algorithm. The (10\%). Sixty-three studies (89\%) did not explicitly mention most popular algorithms were classification trees (n=30, whether interaction effects were considered for LR models. 42% ), random forests (28,39%), artificial neural networks The remaining eight studies were often unclear on the (26,37%), and support vector machines (24,34%). Of 26 approach for interaction terms (Table A.11). studies using artificial neural networks, 22 used one hidden Penalized LR, as well as many ML algorithms, contains layer, three used multiple hidden layers, and for one study, hyperparameters that determine the complexity/flexibility this was unclear (Table A.9). When support vector of the model. For the most commonly used algorithms, 16 E. Chrissodoubu ef al./ Journad of Clinicad Epidemiology 110 (2019) 12-22 Table 1. Algorithms used in the studies ( n=71 studies) used bootstrapping). Seven studies (10\%) used some form of external validation, most commonly using a chronological split of data into training and test parts. Seven studies (10%) did not validate performance, and for three studies (4%), the approach depended on the algorithm. Importantly, in 48 studies (68%), we observed unclear reporting or potential biases in validation procedures for one or more algorithms. Common reasons were that hyperparameters were tuned or variable selection was performed on all data (or this was not clearly specified), or that not all modeling steps were repeated when resampling was used for validation (Table A.13). The AUC was the most commonly reported performance measure ( 64 studies, 90% ), followed by sensitivity ( 45 , 63% ) and specificity (43, 61\%) (Table A.14). Calibration performance was not discussed in 56 studies (79\%) (Table A.15). Most commonly, calibration was addressed using grouped calibration plots ( n=7 ). Only one study (1\%) evaluated performance in terms of clinical utility using decision curve analysis. In 21 studies, methods were applied to address outcome imbalance, that is, an event rate far from 50% (Table A.16, see Section 4). 3.5. Comparison between performance of LR and ML The most problematic risk of bias item was an unclear/ biased validation procedure (Fig. 2, Table A.17). We identified 282 comparisons between standard/penalized LR (AUC 0.52-0.97) and ML models (AUC 0.580.99 ) in 58 articles. Of the remaining 13 articles, seven did not report AUCs, three reported AUCs for some algorithms only, one reported AUCs to one decimal, one only applied standard and penalized LR, and one only applied bagged LR and random forests. 145 comparisons (51%) were labeled as having low risk of bias. The logit(AUC) was on average 0.25 higher for ML vs. LR (95\% CI 0.120.38 ) (Figs. 3 and 4). However, the logit(AUC) difference was on average 0.00(0.18 to 0.18 ) for comparisons with low risk of bias, and 0.34 higher (0.200.47) for comparisons with high risk of bias. Trees uniformly had worse performance than other ML algorithms. Otherwise, results for different ML algorithms were similar. Finally, Table A.18 reports on additional findings on parameters was not clear in at least half of the studies methodology and reporting that could not be discussed in (Table A.12). It was either unclear whether hyperpara- the main text due to space limitations. meters were tuned or default settings were used, or hyperparameters were said to be tuned but the tuning procedure was not clear. 4. Discussion 3.4. Model validation Our systematic review of studies that compare clinical prediction models using LR and ML yielded the following Twenty-nine studies (41% ) used a single random split of key findings. Reporting of methodology and findings was the data into train-test or train-validate-test parts (Table 2). very often incomplete and unclear; model validation proTwenty-five studies used resampling (35%;15 used cross- cedures still often were poor. Calibration of risk predictions validation, nine used repeated random splitting, and one was seldom examined, and AUC performance of LR and Counts refer to articles. Risk of bias in model validation refers to the first of five bias signaling items that were used in this study. No risk of bias: the item was scored as "no" for all models in the study; unclear: the item was scored as "unclear" for at least one model; yes: the item was scored as "yes"' (bias present) for at least one model. a Table A. 2 describes the five bias items. For bias in model validation, we repeat the description here: We discern two general criteria to assess the validation: first, it should be clear that models are developed using training data only; second, if validation is performed using resampling (repeated data splitting, cross-validation, bootstrapping), it should be clear that all model building steps are repeated in every train ing data set; ad hoc flaws are documented and tabulated. ML was on average no different when comparisons had low into probabilities [101]. In this article, calibration refers risk of bias. The latter finding is in line with the claim that to evaluation of the reliability of probabilistic (risk) estraditional approaches often perform remarkably well [21]. timates [18]. A transformation of model outcomes into Our findings lead to the following recommendations probabilities is part of model development. Furthermore, (Table A.19). First, fully report on all modeling steps and the ML literature has paid attention to the utility of models. analyses in sufficient detail to maximize transparency and For example cost curves are very similar to decision curve reproducibility. We recommend to adhere to the TRIPOD analysis [102]. Finally, the issue of class imbalance is guidelines [19]. If necessary, include detailed descriptions as Supplementary Material. For complex procedures, a comprehensive flowchart of the development and validation procedures can be insightful-some studies provided this [53]. Second, if model validation is based on resampling, the model development should be based on all available data, and the resampling should then include all modeling steps that were used to build the model to estimate performance. Model development on all data was often not performed. In addition, provide all information on these models to allow independent validation. Third, report training and test performance. The difference between these results is informative. Fourth, evaluate model performance in terms of calibration (whether risk estimates are accurate) and clinical utility for decision-making [18]. Preferably, calibration should be investigated using calibration curves, whereas the Hosmer-Lemeshow test should be avoided [18,98,99]. Clinical utility can be assessed using decision Fig. 2. Summary of the five signaling items at study level ( n=71 ). No curve analysis, which is increasingly used in medical appli- (green): none of the five items were scored as "unclear" or "yes" in cations [100]. the whole study; unclear (orange): at least one item was scored as We found several differences between the ML and statis-__ "unclear" for at least one model; yes (red): at least one itemn was tical literature. In the ML literature, calibration often refers scored as "yes" for at least one model. (F interpretation of the refto the transformation of nonprobabilistic model outcomes version of this article.) 18 E. Chrissodoubu ef al./ Journad of Clinicad Epidemiology 110 (2019) 12-22 Fig. 3. Beeswarm plots of AUC difference (AUC of ML method minus AUC of LR) for all 282 comparisons by ML category, overall (A) and stratified by risk of bias (B). LR, logistic regression; ML, machine learning; RF, random forest; SVM, support vecto machine; ANN, artificial neural network. common in the ML literature [13]. This is motivated by a the standard method for clinical prediction, and more moddominant focus on classification and overall accuracy based ern approaches are often discussed in relation to LR on a 50% risk cutoff. However, adjusting class imbalance [6,7,1417,104,107]. distorts prevalence and yields inadequate risk predictions. Future research should focus more on delineating the This is not acceptable for clinical risk prediction. In partic- type of predictive problems in which various algorithms ular, downsampling is inefficient because it reduces sample have maximal value. For example, the signal-to-noise ratio size. Recent research clearly indicated that this increases may be an important aspect in determining how successful the risk of overfitting [103]. ML will be [2,21,107]. ML tends to work well for problems The comparison of AUC performance between LR and with a strong signal-to-noise ratio [108], for example, handML depends on how one defines risk of bias and ML. We writing recognition, gaming, or electric load forecasting. used five signaling items to consider comparisons as at Clinical prediction problems often have a poor signal-tolow or high risk of bias. These items did not address noise ratio [107]. whether LR models were penalized or included nonlinear A limitation of our study is that it does not investigate and/or interaction effects. Regression is sometimes pre- which factors influence the difference in performance sented as a method that simply assumes linearity and addi- (e.g., sample size, number of predictors, hyperparameter tivity [7,104]. In comparison studies, it is usually tuning). We feel that such a study would be relevant, but implemented as such, for example, in two recent bench- should be performed by comparing different scenarios on mark studies using data set repositories [105,106]. Some the same data sets to avoid confounding [106]. Another criticize that assuming linearity and additivity will reduce limitation is that many studies had a fairly limited number the performance of regression, although this may depend of events per considered predictor, a common problem on sample size. Regarding the definition of ML, we used despite repeated warnings [1,17,99,103,109]. This issue ura broad approach: we focused on alternative algorithms gently needs better consideration. Some researchers claim for LR, hereby only excluding classical statistical algo- that ML will not outperform LR when only a limited set rithms (we also excluded GAM, although some may see of prespecified predictors is considered, and that the advanthis as an ML method). The rationale is that LR has been tage of ML lies in better handling a huge amount of E. Christodbulou ef al / Joumal of Clinical Epidemiobgy 110 (2019) 12-22 19 - review \& editing. Ewout W. Steyerberg: Conceptualiza- Fig. 4. Differences in discriminative ability between LK and ML Supplementary data models, overall and aocording to risk of bias ( n=282 comparisons). Supplementary data related to this article can be found at When LR was compared with traditional statistical methods (discrim- https://doi.org/10.1016/j.jclinepi.2019.02.004. inant analysis, Poisson regression, generalized estimating equations, generalized additive models), these methods were not included as "Other ML methods" and were thus excluded from this plot. LR, logis- References tic regersion; RF, random forest; SVM, support vector machine; ANN, artificial neural network. [1] Steyerberg EW. Clinical prediction models New York, NY: Springer, 2009. [2] Hastie T, Tibshirani R, Friedman J. The elements of statistical predictors [3,7,12,15,16,104]. Unfortunately, all 23 comlearning: data mining, inference, and prediction. 2nd ed. New York, parisons that we identified from the seven included studies NY: Springer, 2009. with >100 predictors were at high risk of bias. Neverthe- [3] Kononenko I. Machine leaming for medical diagnosis: history, state less, their median AUC difference was 0.005. In contraof the art and perspective. Artif Intell Med 2001;23:89-109. diction with the aforementioned claim, recent research [4] Lisboa PJ, Taktak AFG. The use of artificial neural networks in desuggests that ML requires more data than LR [17]. A final cision support in cancer. a systematic review. Neural Netw 2006;19: limitation is that conducting a decent and detailed system408-15. atic review on this broad topic was time-consuming. In the [5] Beam AL, Kohane IS. Big data and machine learning in health care. IAMA 2018;319:1317-8. meantime, new studies will have been published. Although [6] Chen JH, Asch SM. Machine learning and prediction in medicine there is the potential that methodology and reporting has - beyond the peak of inflated expectations N Engl J Med 2017; improved, such improvements are slow even when longer 376.25079. periods are considered [110-112]. [7] Goldstein BA, Navar AM, Carter RE. Moving beyond regression In conclusion, evidence is lacking to support the claim techniques in cardiovascular risk prediction: applying machine that clinical prediction models based on ML lead to better learning to address analytic challenges Eur Heart J 2017;38: AUCs than clinical prediction models based on LR. Report180614. [8] Breiman L Statistical modeling, the two cultures (with comments ing of articles that compare both types of algorithms needs and a rejoinder by the author). Stat Sci 2001;16:199-231. to improve. Correct validation procedures are needed [113], [9] Moons KGM, de Groot IAH, Bouwmeester W, Vergouwe Y, with assessment of calibration and clinical utility in addiMallett S, Altman DG, et al. Critical appraisal and data extraction tion to discrimination, to define situations where modern for systematic reviews of prediction modelling stulies: the methods have advantages over traditional approaches. CHARMS checklist. PLoS Med 2014;11:e1001744. [10] Mitchell TM. Machine learning. New York, NY: McGraw Hill; 1997. [11] Boulesteix AL, Schmid M. Machine learning verass statistical modeling. Biom J 2014:56:588-93. [12] Deo RC, Nallamothu BK. Leaming about machine leaming: the promise and piffalls of big data and the electronic health record. CRediT authorship contribution statement Circ Cardiovasc Qual Outcomes 2016;9:618-20. Evangelia Christodoulou: Conceptualization, Investiga- [13] He H, Garcia EA. Leaming from imbalanced data. IEEE Trans tion, Formal analysis, Data curation, Writing - original Knowl Data Eng 2008:21:1263-84. draft. Jie Ma: Investigation, Writing - review \& editing. [14] Pochet NLMM, Suykens IAK. Support vector machines versus loGary S. Collins: Conceptualization, Data curation, Writing gistic regression: improving prospective performance in clinical decision-making. Ultrasound Obstet Gynecol 2006;27:607-8Step by Step Solution
There are 3 Steps involved in it
Step: 1
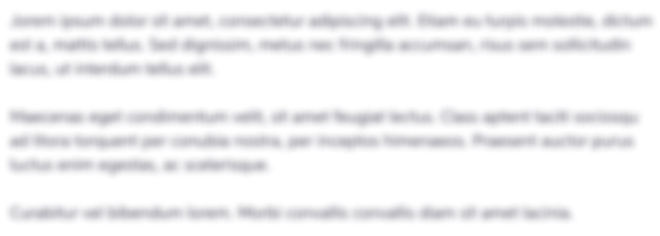
Get Instant Access to Expert-Tailored Solutions
See step-by-step solutions with expert insights and AI powered tools for academic success
Step: 2

Step: 3

Ace Your Homework with AI
Get the answers you need in no time with our AI-driven, step-by-step assistance
Get Started