Answered step by step
Verified Expert Solution
Question
1 Approved Answer
Curve-fitting Project - Linear Model (due at the end of Week 5) Instructions For this assignment, collect data exhibiting a relatively linear trend, find the
Curve-fitting Project - Linear Model (due at the end of Week 5) Instructions For this assignment, collect data exhibiting a relatively linear trend, find the line of best fit, plot the data and the line, interpret the slope, and use the linear equation to make a prediction. Also, find r2 (coefficient of determination) and r (correlation coefficient). Discuss your findings. Your topic may be that is related to sports, your work, a hobby, or something you find interesting. If you choose, you may use the suggestions described below. A Linear Model Example and Technology Tips are provided in separate documents. Tasks for Linear Regression Model (LR) (LR-1) Describe your topic, provide your data, and cite your source. Collect at least 8 data points. Label appropriately. (Highly recommended: Post this information in the Linear Model Project discussion as well as in your completed project. Include a brief informative description in the title of your posting. Each student must use different data.) The idea with the discussion posting is two-fold: (1) To share your interesting project idea with your classmates, and (2) To give me a chance to give you a brief thumbs-up or thumbs-down about your proposed topic and data. Sometimes students get off on the wrong foot or misunderstand the intent of the project, and your posting provides an opportunity for some feedback. Remark: Students may choose similar topics, but must have different data sets. For example, several students may be interested in a particular Olympic sport, and that is fine, but they must collect different data, perhaps from different events or different gen der. (LR-2) Plot the points (x, y) to obtain a scatterplot. Use an appropriate scale on the horizontal and vertical axes and be sure to label carefully. Visually judge whether the data points exhibit a relatively linear trend. (If so, proceed. If not, try a different topic or data set.) (LR-3) Find the line of best fit (regression line) and graph it on the scatterplot. State the equation of the line. (LR-4) State the slope of the line of best fit. Carefully interpret the meaning of the slope in a sentence or two. (LR-5) Find and state the value of r2, the coefficient of determination, and r, the correlation coefficient. Discuss your findings in a few sentences. Is r positive or negative? Why? Is a line a good curve to fit to this data? Why or why not? Is the linear relationship very strong, moderately strong, weak, or nonexistent? (LR-6) Choose a value of interest and use the line of best fit to make an estimate or prediction. Show calculation work. (LR-7) Write a brief narrative of a paragraph or two. Summarize your findings and be sure to mention any aspect of the linear model project (topic, data, scatterplot, line, r, or estimate, etc.) that you found particularly important or interesting. You may submit all of your project in one document or a combination of documents, which may consist of word processing documents or spreadsheets or scanned handwritten work, provided it is clearly labeled where each task can be found. Be sure to include your name. Projects are graded on the basis of completeness, correctness, ease in locating all of the checklist items, and strength of the narrative portions. Curve-fitting Project - Linear Model (due at the end of Week 5) Instructions For this assignment, collect data exhibiting a relatively linear trend, find the line of best fit, plot the data and the line, interpret the slope, and use the linear equation to make a prediction. Also, find r2 (coefficient of determination) and r (correlation coefficient). Discuss your findings. Your topic may be that is related to sports, your work, a hobby, or something you find interesting. If you choose, you may use the suggestions described below. A Linear Model Example and Technology Tips are provided in separate documents. Tasks for Linear Regression Model (LR) (LR-1) Describe your topic, provide your data, and cite your source. Collect at least 8 data points. Label appropriately. (Highly recommended: Post this information in the Linear Model Project discussion as well as in your completed project. Include a brief informative description in the title of your posting. Each student must use different data.) The idea with the discussion posting is two-fold: (1) To share your interesting project idea with your classmates, and (2) To give me a chance to give you a brief thumbs-up or thumbs-down about your proposed topic and data. Sometimes students get off on the wrong foot or misunderstand the intent of the project, and your posting provides an opportunity for some feedback. Remark: Students may choose similar topics, but must have different data sets. For example, several students may be interested in a particular Olympic sport, and that is fine, but they must collect different data, perhaps from different events or different gen der. (LR-2) Plot the points (x, y) to obtain a scatterplot. Use an appropriate scale on the horizontal and vertical axes and be sure to label carefully. Visually judge whether the data points exhibit a relatively linear trend. (If so, proceed. If not, try a different topic or data set.) (LR-3) Find the line of best fit (regression line) and graph it on the scatterplot. State the equation of the line. (LR-4) State the slope of the line of best fit. Carefully interpret the meaning of the slope in a sentence or two. (LR-5) Find and state the value of r2, the coefficient of determination, and r, the correlation coefficient. Discuss your findings in a few sentences. Is r positive or negative? Why? Is a line a good curve to fit to this data? Why or why not? Is the linear relationship very strong, moderately strong, weak, or nonexistent? (LR-6) Choose a value of interest and use the line of best fit to make an estimate or prediction. Show calculation work. (LR-7) Write a brief narrative of a paragraph or two. Summarize your findings and be sure to mention any aspect of the linear model project (topic, data, scatterplot, line, r, or estimate, etc.) that you found particularly important or interesting. You may submit all of your project in one document or a combination of documents, which may consist of word processing documents or spreadsheets or scanned handwritten work, provided it is clearly labeled where each task can be found. Be sure to include your name. Projects are graded on the basis of completeness, correctness, ease in locating all of the checklist items, and strength of the narrative portions. (Sample) Curve-Fitting Project - Linear Model: Men's 400 Meter Dash Submitted by Suzanne Sands (LR-1) Purpose: To analyze the winning times for the Olympic Men's 400 Meter Dash using a linear model Data: The winning times were retrieved from http://www.databaseolympics.com/sport/sportevent.htm?sp=ATH&enum=130 The winning times were gathered for the most recent 16 Summer Olympics, post-WWII. (More data was available, back to 1896.) DATA: (LR-2) SCATTERPLOT: Summer Olympics: Men's 400 Meter Dash Winning Times Year 1948 1952 1956 1960 1964 1968 1972 1976 1980 1984 1988 1992 1996 2000 2004 2008 Time (seconds) 46.20 45.90 46.70 44.90 45.10 43.80 44.66 44.26 44.60 44.27 43.87 43.50 43.49 43.84 44.00 43.75 As one would expect, the winning times generally show a downward trend, as stronger co methods result in faster speeds. The trend is somewhat linear. (LR-3) Line of Best Fit (Regression Line) y = 0.0431x + 129.84 where x = Year and y = Winning Time (in seconds) (LR-4) The slope is 0.0431 and is negative since the winning times are generally decreasing. The slope indicates that in general, the winning time decreases by 0.0431 second a year, and so the winning time decreases at an average rate of 4(0.0431) = 0.1724 second each 4-year Olympic interval. (LR-5) Values of r2 and r: r2 = 0.6991 We know that the slope of the regression line is negative so the correlation coefficient r must be negative. r= 0.6991=0.84 Recall that r = 1 corresponds to perfect negative correlation, and so r = (Sample) Curve-Fitting Project - Linear Model: Men's 400 Meter Dash Submitted by Suzanne Sands (LR-1) Purpose: To analyze the winning times for the Olympic Men's 400 Meter Dash using a linear model Data: The winning times were retrieved from http://www.databaseolympics.com/sport/sportevent.htm?sp=ATH&enum=130 The winning times were gathered for the most recent 16 Summer Olympics, post-WWII. (More data was available, back to 1896.) DATA: (LR-2) SCATTERPLOT: Summer Olympics: Men's 400 Meter Dash Winning Times Time (seconds) 46.20 45.90 46.70 44.90 45.10 43.80 44.66 44.26 44.60 44.27 43.87 43.50 43.49 43.84 44.00 43.75 47.00 46.50 46.00 Time (seconds) Year 1948 1952 1956 1960 1964 1968 1972 1976 1980 1984 1988 1992 1996 2000 2004 2008 Summer Olympics: Men's 400 Meter Dash Winning Times 45.50 45.00 44.50 44.00 43.50 43.00 1944 1952 1960 1968 1976 1984 1992 2000 2008 Year As one would expect, the winning times generally show a downward trend, as stronger competition and training methods result in faster speeds. The trend is somewhat linear. Page 1 of 4 (LR-3) Summer Olympics: Men's 400 Meter Dash Winning Times 47.00 46.50 y = -0.0431x + 129.84 R = 0.6991 Time (seconds) 46.00 45.50 45.00 44.50 44.00 43.50 43.00 1944 1952 1960 1968 1976 1984 1992 2000 2008 Year Line of Best Fit (Regression Line) y = 0.0431x + 129.84 where x = Year and y = Winning Time (in seconds) (LR-4) The slope is 0.0431 and is negative since the winning times are generally decreasing. The slope indicates that in general, the winning time decreases by 0.0431 second a year, and so the winning time decreases at an average rate of 4(0.0431) = 0.1724 second each 4-year Olympic interval. Page 2 of 4 (LR-5) Values of r2 and r: r2 = 0.6991 We know that the slope of the regression line is negative so the correlation coefficient r must be negative. \u0001 = 0.6991 = 0.84 Recall that r = 1 corresponds to perfect negative correlation, and so r = 0.84 indicates moderately strong negative correlation (relatively close to -1 but not very strong). (LR-6) Prediction: For the 2012 Summer Olympics, substitute x = 2012 to get y = 0.0431(2012) + 129.84 43.1 seconds. The regression line predicts a winning time of 43.1 seconds for the Men's 400 Meter Dash in the 2012 Summer Olympics in London. (LR-7) Narrative: The data consisted of the winning times for the men's 400m event in the Summer Olympics, for 1948 through 2008. The data exhibit a moderately strong downward linear trend, looking overall at the 60 year period. The regression line predicts a winning time of 43.1 seconds for the 2012 Summer Olympics, which would be nearly 0.4 second less than the existing Olympic record of 43.49 seconds, quite a feat! Will the regression line's prediction be accurate? In the last two decades, there appears to be more of a cyclical (up and down) trend. Could winning times continue to drop at the same average rate? Extensive searches for talented potential athletes and improved full-time training methods can lead to decreased winning times, but ultimately, there will be a physical limit for humans. Note that there were some unusual data points of 46.7 seconds in 1956 and 43.80 in 1968, which are far above and far below the regression line. If we restrict ourselves to looking just at the most recent winning times, beyond 1968, for Olympic winning times in 1972 and beyond (10 winning times), we have the following scatterplot and regression line. Page 3 of 4 Summer Olympics: Men's 400 Meter Dash Winning Times 44.80 Time (seconds) 44.60 y = -0.025x + 93.834 R = 0.5351 44.40 44.20 44.00 43.80 43.60 43.40 1968 1976 1984 1992 2000 2008 Year Using the most recent ten winning times, our regression line is y = 0.025x + 93.834. When x = 2012, the prediction is y = 0.025(2012) + 93.834 43.5 seconds. This line predicts a winning time of 43.5 seconds for 2012 and that would indicate an excellent time close to the existing record of 43.49 seconds, but not dramatically below it. Note too that for r2 = 0.5351 and for the negatively sloping line, the correlation coefficient is \u0001 = 0.5351 = 0.73, not as strong as when we considered the time period going back to 1948. The most recent set of 10 winning times do not visually exhibit as strong a linear trend as the set of 16 winning times dating back to 1948. CONCLUSION: I have examined two linear models, using different subsets of the Olympic winning times for the men's 400 meter dash and both have moderately strong negative correlation coefficients. One model uses data extending back to 1948 and predicts a winning time of 43.1 seconds for the 2012 Olympics, and the other model uses data from the most recent 10 Olympic games and predicts 43.5 seconds. My guess is that 43.5 will be closer to the actual winning time. We will see what happens later this summer! UPDATE: When the race was run in August, 2012, the winning time was 43.94 seconds. Page 4 of 4 Curve-Fitting Project - Linear Model: Formula 1 Submitted by Jennifer Tyler (LR-1) Purpose: To analyze the winning times for the Formula 1 racing using a linear model Formula 1 (F1) season 2016 is chosen for linear model to analyse the winning chance for new influence driver Nico Rosberg, this analysis helps in understanding the strengths of Nico Rosberg as he is receiving rival competition from Lewis Hamilton (Team Member - Mercedes). As of Italy race end chart Nico Rosberg lags 2 points for first position, the update of leader's board for first four position is represented below for quick reference to develop linear model. Driver Lewis Hamilton Nico Rosberg Daniel Ricciardo Sebastian Vettel Team Mercedes Mercedes Red bull Ferrari Points 250 248 161 143 Data: The winning times were retrieved from https://www.formula1.com/en/results.html/2016/races/942/spain/race-result.html The data's are collected from official website of Formula1 for authenticity of the work, the source is cited for this work and data's of lap timing of Nico Rosberg is tabulated below, Driver - Nico Rosberg Race Stage Australia Bahrain China Russia Spain Monaco Canada Europe Austria Great Britain Hungary Germany Belgium Italy Total Laps (LR-2) SCATTERPLOT: 57 57 56 53 66 78 70 51 71 52 70 67 44 56 Lap runtime 1:48:15.565 1:33:34.696 1:38:53.891 1:32:41.997 DNF 2:00:22.423 1:32:07.389 1:32:52.366 1:28:04.817 1:35:12.742 1:40:32.092 1:30:59.845 1:44:51.058 1:17:28.089 Position 1 1 1 1 DNF 7 5 1 4 3 2 4 1 1 Total points Points 25 25 25 25 DNF 6 10 25 12 15 18 12 25 25 248 02:09:36.0 01:55:12.0 01:40:48.0 01:26:24.0 01:12:00.0 00:57:36.0 00:43:12.0 00:28:48.0 00:14:24.0 00:00:00.0 0 2 4 6 8 10 12 14 16 Plot Analysis: The spreadsheet is utilized in scatter plot of the lap time, (LR-3) 02:09:36.0 01:55:12.0 01:40:48.0 01:26:24.0 f(x) = 0x + 0.06 R = 0 01:12:00.0 Nico Rosberg F1-2016 Linear (Nico Rosberg F12016) 00:57:36.0 00:43:12.0 00:28:48.0 00:14:24.0 00:00:00.0 0 2 4 6 8 10 12 14 16 The best fit (Regression line) y= 0.00001x + 0.061 (best fit) r2 0.000001 Intercept 0.061 (LR-4) Slope 0.00001and is positive since the winning times are generally increasing. The slope indicates that in general, the winning time increases by 99.9999 . (LR-5)Values of r2 and r: r2 = 0.000001 We know that the slope of the regression line is positive so the correlation coefficient r must be positive. r=-0.000001= 0 so the winning chance is very strong. (LR-6) Prediction: By analysis 2016 season for Formula 1 winning by Nico Rosberg will lead by 12 points. (LR-7) Narrative: The r value is positive so our data chosen for analysis is a good fit. By analysing the data value it is found clear that Nico Rosberg is good to hold pole position no:1 by driving laps below 60 and also in all the available 14 race it is observed that he holds first pole position at ease, by comparing with his teammate Lewis Hamilton he holds no good at endurance run with long laps . One such can be observed with the result of Monaco racing where he ends with position no:7 due to 78 laps endurance run. From the analysis of linear model it has been clear Nico Rosenberg will win this season by 4 points while comparing to Lewis as forthcoming race in Singapore and Malaysia comes with 50 to 60 laps, additionally curve fitting shows he holds r value near to zero shows the chance of winning the series is 99.999% further precise analysis can be carried out by result outcome of Singapore racing. Curve-fitting Project - Linear Model (due at the end of Week 5) Instructions For this assignment, collect data exhibiting a relatively linear trend, find the line of best fit, plot the data and the line, interpret the slope, and use the linear equation to make a prediction. Also, find r2 (coefficient of determination) and r (correlation coefficient). Discuss your findings. Your topic may be that is related to sports, your work, a hobby, or something you find interesting. If you choose, you may use the suggestions described below. A Linear Model Example and Technology Tips are provided in separate documents. Tasks for Linear Regression Model (LR) (LR-1) Describe your topic, provide your data, and cite your source. Collect at least 8 data points. Label appropriately. (Highly recommended: Post this information in the Linear Model Project discussion as well as in your completed project. Include a brief informative description in the title of your posting. Each student must use different data.) The idea with the discussion posting is two-fold: (1) To share your interesting project idea with your classmates, and (2) To give me a chance to give you a brief thumbs-up or thumbs-down about your proposed topic and data. Sometimes students get off on the wrong foot or misunderstand the intent of the project, and your posting provides an opportunity for some feedback. Remark: Students may choose similar topics, but must have different data sets. For example, several students may be interested in a particular Olympic sport, and that is fine, but they must collect different data, perhaps from different events or different gen der. (LR-2) Plot the points (x, y) to obtain a scatterplot. Use an appropriate scale on the horizontal and vertical axes and be sure to label carefully. Visually judge whether the data points exhibit a relatively linear trend. (If so, proceed. If not, try a different topic or data set.) (LR-3) Find the line of best fit (regression line) and graph it on the scatterplot. State the equation of the line. (LR-4) State the slope of the line of best fit. Carefully interpret the meaning of the slope in a sentence or two. (LR-5) Find and state the value of r2, the coefficient of determination, and r, the correlation coefficient. Discuss your findings in a few sentences. Is r positive or negative? Why? Is a line a good curve to fit to this data? Why or why not? Is the linear relationship very strong, moderately strong, weak, or nonexistent? (LR-6) Choose a value of interest and use the line of best fit to make an estimate or prediction. Show calculation work. (LR-7) Write a brief narrative of a paragraph or two. Summarize your findings and be sure to mention any aspect of the linear model project (topic, data, scatterplot, line, r, or estimate, etc.) that you found particularly important or interesting. You may submit all of your project in one document or a combination of documents, which may consist of word processing documents or spreadsheets or scanned handwritten work, provided it is clearly labeled where each task can be found. Be sure to include your name. Projects are graded on the basis of completeness, correctness, ease in locating all of the checklist items, and strength of the narrative portions. (Sample) Curve-Fitting Project - Linear Model: Men's 400 Meter Dash Submitted by Suzanne Sands (LR-1) Purpose: To analyze the winning times for the Olympic Men's 400 Meter Dash using a linear model Data: The winning times were retrieved from http://www.databaseolympics.com/sport/sportevent.htm?sp=ATH&enum=130 The winning times were gathered for the most recent 16 Summer Olympics, post-WWII. (More data was available, back to 1896.) DATA: (LR-2) SCATTERPLOT: Summer Olympics: Men's 400 Meter Dash Winning Times Year 1948 1952 1956 1960 1964 1968 1972 1976 1980 1984 1988 1992 1996 2000 2004 2008 Time (seconds) 46.20 45.90 46.70 44.90 45.10 43.80 44.66 44.26 44.60 44.27 43.87 43.50 43.49 43.84 44.00 43.75 As one would expect, the winning times generally show a downward trend, as stronger co methods result in faster speeds. The trend is somewhat linear. (LR-3) Line of Best Fit (Regression Line) y = 0.0431x + 129.84 where x = Year and y = Winning Time (in seconds) (LR-4) The slope is 0.0431 and is negative since the winning times are generally decreasing. The slope indicates that in general, the winning time decreases by 0.0431 second a year, and so the winning time decreases at an average rate of 4(0.0431) = 0.1724 second each 4-year Olympic interval. (LR-5) Values of r2 and r: r2 = 0.6991 We know that the slope of the regression line is negative so the correlation coefficient r must be negative. r= 0.6991=0.84 Recall that r = 1 corresponds to perfect negative correlation, and so r = (Sample) Curve-Fitting Project - Linear Model: Men's 400 Meter Dash Submitted by Suzanne Sands (LR-1) Purpose: To analyze the winning times for the Olympic Men's 400 Meter Dash using a linear model Data: The winning times were retrieved from http://www.databaseolympics.com/sport/sportevent.htm?sp=ATH&enum=130 The winning times were gathered for the most recent 16 Summer Olympics, post-WWII. (More data was available, back to 1896.) DATA: (LR-2) SCATTERPLOT: Summer Olympics: Men's 400 Meter Dash Winning Times Time (seconds) 46.20 45.90 46.70 44.90 45.10 43.80 44.66 44.26 44.60 44.27 43.87 43.50 43.49 43.84 44.00 43.75 47.00 46.50 46.00 Time (seconds) Year 1948 1952 1956 1960 1964 1968 1972 1976 1980 1984 1988 1992 1996 2000 2004 2008 Summer Olympics: Men's 400 Meter Dash Winning Times 45.50 45.00 44.50 44.00 43.50 43.00 1944 1952 1960 1968 1976 1984 1992 2000 2008 Year As one would expect, the winning times generally show a downward trend, as stronger competition and training methods result in faster speeds. The trend is somewhat linear. Page 1 of 4 (LR-3) Summer Olympics: Men's 400 Meter Dash Winning Times 47.00 46.50 y = -0.0431x + 129.84 R = 0.6991 Time (seconds) 46.00 45.50 45.00 44.50 44.00 43.50 43.00 1944 1952 1960 1968 1976 1984 1992 2000 2008 Year Line of Best Fit (Regression Line) y = 0.0431x + 129.84 where x = Year and y = Winning Time (in seconds) (LR-4) The slope is 0.0431 and is negative since the winning times are generally decreasing. The slope indicates that in general, the winning time decreases by 0.0431 second a year, and so the winning time decreases at an average rate of 4(0.0431) = 0.1724 second each 4-year Olympic interval. Page 2 of 4 (LR-5) Values of r2 and r: r2 = 0.6991 We know that the slope of the regression line is negative so the correlation coefficient r must be negative. \u0001 = 0.6991 = 0.84 Recall that r = 1 corresponds to perfect negative correlation, and so r = 0.84 indicates moderately strong negative correlation (relatively close to -1 but not very strong). (LR-6) Prediction: For the 2012 Summer Olympics, substitute x = 2012 to get y = 0.0431(2012) + 129.84 43.1 seconds. The regression line predicts a winning time of 43.1 seconds for the Men's 400 Meter Dash in the 2012 Summer Olympics in London. (LR-7) Narrative: The data consisted of the winning times for the men's 400m event in the Summer Olympics, for 1948 through 2008. The data exhibit a moderately strong downward linear trend, looking overall at the 60 year period. The regression line predicts a winning time of 43.1 seconds for the 2012 Summer Olympics, which would be nearly 0.4 second less than the existing Olympic record of 43.49 seconds, quite a feat! Will the regression line's prediction be accurate? In the last two decades, there appears to be more of a cyclical (up and down) trend. Could winning times continue to drop at the same average rate? Extensive searches for talented potential athletes and improved full-time training methods can lead to decreased winning times, but ultimately, there will be a physical limit for humans. Note that there were some unusual data points of 46.7 seconds in 1956 and 43.80 in 1968, which are far above and far below the regression line. If we restrict ourselves to looking just at the most recent winning times, beyond 1968, for Olympic winning times in 1972 and beyond (10 winning times), we have the following scatterplot and regression line. Page 3 of 4 Summer Olympics: Men's 400 Meter Dash Winning Times 44.80 Time (seconds) 44.60 y = -0.025x + 93.834 R = 0.5351 44.40 44.20 44.00 43.80 43.60 43.40 1968 1976 1984 1992 2000 2008 Year Using the most recent ten winning times, our regression line is y = 0.025x + 93.834. When x = 2012, the prediction is y = 0.025(2012) + 93.834 43.5 seconds. This line predicts a winning time of 43.5 seconds for 2012 and that would indicate an excellent time close to the existing record of 43.49 seconds, but not dramatically below it. Note too that for r2 = 0.5351 and for the negatively sloping line, the correlation coefficient is \u0001 = 0.5351 = 0.73, not as strong as when we considered the time period going back to 1948. The most recent set of 10 winning times do not visually exhibit as strong a linear trend as the set of 16 winning times dating back to 1948. CONCLUSION: I have examined two linear models, using different subsets of the Olympic winning times for the men's 400 meter dash and both have moderately strong negative correlation coefficients. One model uses data extending back to 1948 and predicts a winning time of 43.1 seconds for the 2012 Olympics, and the other model uses data from the most recent 10 Olympic games and predicts 43.5 seconds. My guess is that 43.5 will be closer to the actual winning time. We will see what happens later this summer! UPDATE: When the race was run in August, 2012, the winning time was 43.94 seconds. Page 4 of 4 Curve-Fitting Project - Linear Model: Formula 1 Submitted by Jennifer Tyler (LR-1) Purpose: To analyze the winning times for the Formula 1 racing using a linear model Formula 1 (F1) season 2016 is chosen for linear model to analyse the winning chance for new influence driver Nico Rosberg, this analysis helps in understanding the strengths of Nico Rosberg as he is receiving rival competition from Lewis Hamilton (Team Member - Mercedes). As of Italy race end chart Nico Rosberg lags 2 points for first position, the update of leader's board for first four position is represented below for quick reference to develop linear model. Driver Lewis Hamilton Nico Rosberg Daniel Ricciardo Sebastian Vettel Team Mercedes Mercedes Red bull Ferrari Points 250 248 161 143 Data: The winning times were retrieved from https://www.formula1.com/en/results.html/2016/races/942/spain/race-result.html The data's are collected from official website of Formula1 for authenticity of the work, the source is cited for this work and data's of lap timing of Nico Rosberg is tabulated below, Driver - Nico Rosberg Race Stage Australia Bahrain China Russia Spain Monaco Canada Europe Austria Great Britain Hungary Germany Belgium Italy Total Laps (LR-2) SCATTERPLOT: 57 57 56 53 66 78 70 51 71 52 70 67 44 56 Lap runtime 1:48:15.565 1:33:34.696 1:38:53.891 1:32:41.997 DNF 2:00:22.423 1:32:07.389 1:32:52.366 1:28:04.817 1:35:12.742 1:40:32.092 1:30:59.845 1:44:51.058 1:17:28.089 Position 1 1 1 1 DNF 7 5 1 4 3 2 4 1 1 Total points Points 25 25 25 25 DNF 6 10 25 12 15 18 12 25 25 248 02:09:36.0 01:55:12.0 01:40:48.0 01:26:24.0 01:12:00.0 00:57:36.0 00:43:12.0 00:28:48.0 00:14:24.0 00:00:00.0 0 2 4 6 8 10 12 14 16 Plot Analysis: The spreadsheet is utilized in scatter plot of the lap time, (LR-3) 02:09:36.0 01:55:12.0 01:40:48.0 01:26:24.0 f(x) = 0x + 0.06 R = 0 01:12:00.0 Nico Rosberg F1-2016 Linear (Nico Rosberg F12016) 00:57:36.0 00:43:12.0 00:28:48.0 00:14:24.0 00:00:00.0 0 2 4 6 8 10 12 14 16 The best fit (Regression line) y= 0.00001x + 0.061 (best fit) r2 0.000001 Intercept 0.061 (LR-4) Slope 0.00001and is positive since the winning times are generally increasing. The slope indicates that in general, the winning time increases by 99.9999 . (LR-5)Values of r2 and r: r2 = 0.000001 We know that the slope of the regression line is positive so the correlation coefficient r must be positive. r=-0.000001= 0 so the winning chance is very strong. (LR-6) Prediction: By analysis 2016 season for Formula 1 winning by Nico Rosberg will lead by 12 points. (LR-7) Narrative: The r value is positive so our data chosen for analysis is a good fit. By analysing the data value it is found clear that Nico Rosberg is good to hold pole position no:1 by driving laps below 60 and also in all the available 14 race it is observed that he holds first pole position at ease, by comparing with his teammate Lewis Hamilton he holds no good at endurance run with long laps . One such can be observed with the result of Monaco racing where he ends with position no:7 due to 78 laps endurance run. From the analysis of linear model it has been clear Nico Rosenberg will win this season by 4 points while comparing to Lewis as forthcoming race in Singapore and Malaysia comes with 50 to 60 laps, additionally curve fitting shows he holds r value near to zero shows the chance of winning the series is 99.999% further precise analysis can be carried out by result outcome of Singapore racing
Step by Step Solution
There are 3 Steps involved in it
Step: 1
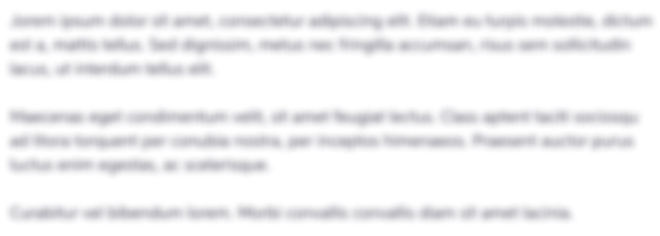
Get Instant Access to Expert-Tailored Solutions
See step-by-step solutions with expert insights and AI powered tools for academic success
Step: 2

Step: 3

Ace Your Homework with AI
Get the answers you need in no time with our AI-driven, step-by-step assistance
Get Started