Answered step by step
Verified Expert Solution
Question
1 Approved Answer
Cutting Edge This case was adapted from Hiller, Frederick S. & Mark S. Hillier (2014). Introduction to Management Science: A Modeling and Case Studies Approach
Cutting Edge This case was adapted from Hiller, Frederick S. & Mark S. Hillier (2014). Introduction to Management Science: A Modeling and Case Studies Approach with Spreadsheets, 5th ed., McGraw-Hill/Irwin, pp 429-432. Part 1 Mark Lawrence has been pursuing a vision for more than two years. This pursuit began when he became frustrated in his role as director of Human Resources at Cutting Edge, a large company manufacturing computers and computer peripherals. At that time the Human Resources Department under his direction provided records and benefits administration to the 60,000 Cutting Edge employees throughout the United States, and 35 separate records and benefits administration centers existed across the country. Employees contact these records and benefits centers to obtain information about dental plans and stock options, change tax forms and personal information, and process leaves of absence and retirements. The decentralization of these administration centers caused numerous headaches for Mark. He had to deal with employee complaints often since each center interpreted company policies differently - communicating inconsistent and sometimes inaccurate answers to employees. His department also suffered high operating costs since operating 35 separate centers created inefficiency. His vision? To centralize records and benefits administration by establishing one administration center. This centralized records and benefits administration center would perform two distinct functions: data management and customer service. The data management function would include updating employee records after performance reviews and maintaining the human resource management system. The customer service function would include establishing a call center to answer employee questions concerning records and benefits and to process records and benefits changes over the phone. One year after proposing his vision to management, Mark received the go-ahead from Cutting Edge corporate headquarters. He prepared his \"to do\" list - specifying computer and phone systems requirements, installing hardware and software, integrating data from the 35 separate administration centers, standardizing record-keeping and response procedures, and staffing the administration center. Mark delegated the systems requirements, installation, and integration jobs to a competent group of technology specialists. He took on the responsibility of standardizing procedures and staffing the administration center. Mark had spent many years in human resources and therefore had little problem with standardizing record-keeping and response procedures. He encountered trouble in determining the number of 1 representatives needed to staff the center, however. He was particularly worried about staffing the call center since the representatives answering phones interact directly with customers - the 60,000 Cutting Edge employees. The customer service representatives would receive extensive training so that they would know the records and benefits policies backwards and forwards - enabling them to answer questions accurately and process changes efficiently. Overstaffing would cause Mark to suffer the high costs of training unneeded representatives and paying the surplus representatives the high salaries that go along with such an intense job. Understaffing would cause Mark to continue to suffer the headaches from customer complaints - something he definitely wanted to avoid. The number of customer service representatives Mark needed to hire depended on the number of calls that the records and benefits call center would receive. Mark therefore needed to forecast the number of calls that the new centralized center would receive. He approached the forecasting problem by using judgmental forecasting. He studied data from one of the 35 decentralized administration centers and learned that the decentralized center had serviced 15,000 customers and had received 2,000 calls per month. He concluded that since the new centralized center would service four times the number of customers - 60,000 customers - it would receive four times the number of calls - 8,000 calls per month. Mark slowly checked off the items on his \"to do\" list, and the centralized records and benefits center opened one year after Mark had received the go-ahead from corporate headquarters. Now, after operating the new center for 13 weeks, Mark's call center forecasts are proving to be terribly inaccurate. The number of calls the center receives is roughly three times as large as the 8,000 calls per month that Mark had forecasted. Because of demand overload, the call center is slowly going to hell in a handbasket. Customers calling the center must wait an average of five minutes before speaking to a representative, and Mark is receiving numerous complaints. At the same time, the customer service representatives are unhappy and on the verge of quitting because of the stress created by the demand overload. Even corporate headquarters has become aware of the staff and service inadequacies, and executives have been breathing down Mark's neck demanding improvements. 2 Part 2 Mark needed help, and he approached Harry, a corporate analyst, to forecast demand for the call center more accurately. Luckily, when Mark first established the call center, he realized the importance of keeping operational data, and he provided Harry with the number of calls received on each day of the week over the last 13 weeks. The data (refer to Cutting Edge Student File No. 1) begins in week 44 of the last year (2012) and continues to week 5 of the current year (2013). Mark indicates that the days where no calls were received were holidays. As a start, Harry used the data from the past 13 weeks and applied five different time-series forecasting methods in preparing a trial forecast of the call volume for each day of the upcoming week (Week 6). He provided a different forecast for each day of the week by treating the forecast for a single day as being the actual call volume on that day. From plotting the data, Harry could see that demand follows \"seasonal\" patterns within the week. For example, more employees call at the beginning of the week when they are fresh and productive than at the end of the week when they are planning for the weekend. Therefore, Mark prepared and used seasonally adjusted call volumes for the past 13 weeks. After Week 6 ended, Harry compared the five forecasts with the actual volumes and calculated the Mean Absolute Deviation (MAD) values for each method. The result of Harry's work is summarized below: 3 Part 3 After many months of work and with Harry's help, Mark has been able to stabilize the call center operation. Mark now has a better handle on how to forecast the daily call demand and he is able to prepare effective weekly staffing schedules for handling the daily variation in volume. However, Mark is still experiencing difficulty in forecasting the volume from month to month. Cutting Edge has been very active in acquiring new companies while, at the same time, selling off portions of their existing business. Mark believes that this activity is causing fluctuations in call volume because it is affecting the employee head count of Cutting Edge. Mark has assembled monthly data for call volume and head count for the past 18 months (refer to Cutting Edge Student File No. 2). Mark also suspects that there are other factors which may be affecting the call volume, and he has noted these factors on the attached spreadsheet. Based on the upcoming acquisition of Cutter Corp on 7/1/2015, the forecast of head count for July 2015 is 77,000. 4 QNT 5160 Guidelines for Individual Case Assignment: Cutting Edge Instructions This is an individual assignment and therefore must be completed by the individual student without outside assistance. In order to complete the assignment, first read the case write-up for the \"Cutting Edge\" case. Then answer the questions listed below for each part of the case. The Part 1 questions refer to the 2 years leading up to the opening of the new call center. Part 2 questions refer to the first 13 weeks of operation after opening the call center. Part 3 questions refer to the first 18 months of operating the call center. Your answers must be entered directly into this Word document below each question. Insert each answer below each question on this document and use as much space as needed. Questions 3a and 3b each require the completion of an Excel spreadsheet. Submit your completed Word document and these two Excel spreadsheets to the Blackboard assignment box before the posted deadline. You may submit additional Excel spreadsheets if you feel they are necessary to support your answers. Grading A total of 100 percentage points is possible for this assignment. This includes the point values which are assigned to each question (point values are noted next to each question below) plus 10 points which are earned based on following the prescribed assignment format, and the proper writing style and APA format. The percentage points earned on this assignment will be multiplied by 25 to obtain the assignment grade. Part 1 Questions: Question 1a (5 points): Define a problem statement which reflects the challenge facing Mark as he planned for the opening of the new center. Question 1b (5 points): Why was Mark's initial forecast of call volume so far off? What could have been the reasons for this? Question 1c (5 points): What could Mark have done differently to improve his initial forecast? Part 2 Questions: In answering the Part 2 questions, you should download and refer to Student Data File No. 1 which contains the historical data that was used in preparing the forecast results that are reported in Part 2 of the case write-up document. Note that you do not have to prepare any forecasts in answering this question. Hint: it will be helpful for you to review a time-series plot of the 13 weeks of data contained on Student Data File No. 1. Question 2a (5 points): Describe the details of the Last Value method used by Harry and explain its accuracy (MAD value) in comparison with the accuracy of the other methods. 1 Question 2b (5 points): Describe the details of the Averaging method used by Harry and explain its accuracy (MAD value) in comparison with the accuracy of the other methods. Question 2c (5 points): Describe the details of the Moving Average (5 days) method used by Harry and explain its accuracy (MAD value) in comparison with the accuracy of the other methods. Question 2d (5 points): Describe the details of the Exponential Smoothing (alpha = 0.1) method used by Harry and explain its accuracy (MAD value) in comparison with the accuracy of the other methods. Question 2e (5 points): Describe the details of the Exponential Smoothing (alpha = 0.5) method used by Harry and explain its accuracy (MAD value) in comparison with the accuracy of the other methods. Part 3 Questions: In answering the Part 3 questions, you should download and refer to Student Data File No. 2 which contains the historical data that you will need to answer the questions. Question 3a (10 points): Prepare a forecast of call volume for July 2015 by applying Exponential Smoothing (with alpha = 0.5) to the prior 18 months of data. Use the appropriate Excel template from the Hillier text to prepare your forecast and assume that initial call volume is 24,000. Show your forecast below and attach the completed Excel template. Call Volume Forecast for July 2015 (Exponential Smoothing, alpha=0.5): _________________ Question 3b (10 points): Apply Linear Regression to predict call volume from head count using the appropriate Excel template. Show your forecast below and attach the completed Excel template. Call Volume Forecast for July 2015 (Causal Forecasting based on head count): _________________ Question 3c (10 points): Calculate the Mean absolute deviation value of the Exponential Smoothing model (Question 3a) and the Average Estimation Error of the Linear Regression model (Question 3b). Explain the difference between these two values. Mean absolute deviation of Exponential Smoothing model, alpha=0.5: ______________________ Average Estimation Error for Causal Forecasting model based on headcount: __________________ Explanation of the difference in values: 2 Question 3d (20 points): Considering your answers to Questions 3a, 3b and 3c and all the factors that have been described above, prepare your best forecast for July 2015. Show your forecast value below and explain and justify how you came up with this forecast. Call Volume Forecast for July 2015 (My forecast): _________________ Explanation and Justification of Your Method: 3 Cutting Edge Individual Case Assignment, QNT 5160, Fall 2015 Semester File No. 1 (Daily data for 2012 and 2013) Week 44 44 44 44 44 45 45 45 45 Day Mon Tue Wed Thur Fri Mon Tue Wed Thur 45 46 46 46 46 46 47 47 47 47 47 48 48 48 48 48 49 49 49 49 49 50 50 50 50 50 51 51 51 51 Fri Mon Tue Wed Thur Fri Mon Tue Wed Thur Fri Mon Tue Wed Thur Fri Mon Tue Wed Thur Fri Mon Tue Wed Thur Fri Mon Tue Wed Thur Actual Call Volume 1,130 750 920 854 698 1,085 1,012 689 920 755 1,403 1,121 1,050 1,113 1,005 2,652 2,825 1,841 2,012 1,345 954 1,022 1,084 1,321 1,056 941 760 695 1,012 833 922 810 784 789 401 51 52/1 52/1 52/1 52/1 52/1 2 2 2 2 2 3 3 3 3 Fri Mon Tue Wed Thur Fri Mon Tue Wed Thur Fri Mon Tue Wed Thur 429 1,209 789 3 4 4 4 4 4 5 5 5 5 5 6 6 6 6 6 Fri Mon Tue Wed Thur Fri Mon Tue Wed Thur Fri Mon Tue Wed Thur Fri 758 923 878 798 1,012 643 945 689 723 754 798 1,132 890 1,362 1,210 980 950 834 1,012 954 1,346 904 723 698 534 578 487 Cutting Edge Individual Case Assignment, QNT 5160, Fall 2015 Semester File No. 2 (Monthly Data for 2014 and 2015) Year 2014 2014 2014 2014 2014 2014 2014 2014 2014 Month Jan Feb Mar Apr May Jun Jul Aug Sep Actual Call Volume 24,015 25,203 23,589 27,454 28,120 28,321 29,021 26,954 26,456 2014 2014 2014 2015 2015 2015 2015 2015 2015 Oct Nov Dec Jan Feb Mar Apr May Jun 27,120 26,954 27,321 26,456 27,450 31,435 33,124 32,432 31,901 Employee Head Count 62,120 62,152 62,138 68,343 68,120 67,987 67,956 65,342 65,380 65,432 65,423 65,650 65,620 65,610 75,231 75,201 74,978 75,012 QNT 5160, Fall 2015 Semester Notes Centex corporation acquired 4/1/2014 Dental insurance plan changed effective 7/1/2014 Printer division sold to Arconet Corporation 8/1/2014 Major tax law changes signed into law by U.S. President Year-end bonuses announced on 12/10/2014 Paxton Enterprises acquired 3/15/2015 New employee insurance deductions in effect starting 7/1/2015 A 1 B C D E G H I J K L M Answer to Question 3a (instructor) FORECAST FOR JULY 2015 = 2 3 4 5 6 7 8 9 10 11 12 13 14 15 16 17 18 19 20 21 22 23 24 25 26 27 28 29 30 31 32 33 34 35 F Time Period 1 2 3 4 5 6 7 8 9 10 11 12 13 14 15 16 17 18 19 20 21 22 23 24 25 26 27 28 29 30 True Value 24,015 25,203 23,589 27,454 28,120 28,321 29,021 26,954 26,456 27,120 26,954 27,321 26,456 27,450 31,435 33,124 32,432 31,901 Exponential Smoothing Forecast 24,000 24,008 24,605 24,097 25,776 26,948 27,634 28,328 27,641 27,048 27,084 27,019 27,170 26,813 27,132 29,283 31,204 31,818 31,859 #N/A #N/A #N/A #N/A #N/A #N/A #N/A #N/A #N/A #N/A #N/A Forecasting Error 15 1,196 1,016 3,357 2,344 1,373 1,387 1,374 1,185 72 130 302 714 637 4,303 3,841 1,228 83 Smoothing Constant a = Initial Estimate Average = 0.5 24,000 Mean Absolute Deviation MAD = 1,364 31,859 Range Name Alpha Forecast ForecastingError InitialEstimate MAD MSE TrueValue Cells H6 D6:D35 E6:E35 H9 H12 H15 C6:C35 Mean Square Error MSE = 3,452,360 35,000 30,000 25,000 20,000 Value True Value 15,000 Forecast 10,000 5,000 0 Time Period N A 1 B C D E F G H I J K L M N O Answer to Question 3b (instructor only) 2 FORECAST FOR JULY 2015 = Average Estimation Error = 3 4 5 6 7 8 9 10 11 12 13 14 15 16 17 18 19 20 21 22 23 24 25 26 27 28 29 30 31 32 33 34 35 36 37 38 39 40 41 Time Period 1 2 3 4 5 6 7 8 9 10 11 12 13 14 15 16 17 18 19 20 21 22 23 24 25 26 27 28 29 30 Head Count Independent Variable 62,120 62,152 62,138 68,343 68,120 67,987 67,956 65,342 65,380 65,432 65,423 65,650 65,620 65,610 75,231 75,201 74,978 75,012 Call Volume Dependent Variable 24,015 25,203 23,589 27,454 28,120 28,321 29,021 26,954 26,456 27,120 26,954 27,321 26,456 27,450 31,435 33,124 32,432 31,901 Estimate 24,720 24,738 24,730 28,369 28,238 28,160 28,142 26,609 26,631 26,662 26,657 26,790 26,772 26,766 32,409 32,391 32,260 32,280 Average -> Estimation Error 704.70 464.53 1141.26 915.12 118.35 160.65 878.83 344.79 175.50 458.01 297.29 531.16 316.24 683.62 973.53 733.07 171.84 379.10 524.87 Square of Error 496,602 215,792 1,302,464 837,448 14,006 25,809 772,343 118,878 30,799 209,771 88,379 282,135 100,010 467,338 947,756 537,385 29,530 143,715 33,446 525 Linear Regression Line y = a + bx a= -11,710.02 b= 0.59 Estimator If x = 77,000 then y= Range Name a b DependentVariable Estimate EstimationError IndependentVariable SquareOfError x y 33,445.94 Cells J5 J6 D5:D34 E5:E34 F5:F34 C5:C34 G5:G34 J10 J12 35,000 30,000 f(x) = 0.5864410105x - 11710.015904676 25,000 Dependent Variable 20,000 15,000 10,000 5,000 0 60,000 62,000 64,000 66,000 68,000 70,000 Independent Variable 72,000 74,000 76,000 P 1 2 3 4 5 6 7 8 9 10 11 12 13 14 15 16 17 18 19 20 21 22 23 24 25 26 27 28 29 30 31 32 33 34 35 36 37 6,000 38 39 40 41 A 1 2 3 4 5 6 7 8 9 10 11 12 13 14 15 16 17 18 19 20 21 22 23 24 25 26 27 28 29 30 31 32 33 34 B C D E F G H I J K L M Template for Averaging Forecasting Method Time Period 1 2 3 4 5 6 7 8 9 10 11 12 13 14 15 16 17 18 19 20 21 22 23 24 25 26 27 28 29 30 True Value 6809 6465 6569 8266 7257 7064 7784 8724 6992 6822 7949 9650 Averaging Forecast Forecasting Error 6,809 6,637 6,614 7,027 7,073 7,072 7,173 7,367 7,326 7,275 7,336 7,529 344 68 1,652 230 9 712 1,551 375 504 674 2,314 #N/A #N/A #N/A #N/A #N/A #N/A #N/A #N/A #N/A #N/A #N/A #N/A #N/A #N/A #N/A #N/A #N/A Mean Absolute Deviation MAD = 767 Mean Square Error MSE = 1,092,400 Range Name Forecast ForecastingError MAD MSE TrueValue Cells D5:D34 E5:E34 H5 H8 C5:C34 12000 10000 8000 Value 6000 True Value Forecast 4000 2000 0 Time Period N A 1 2 3 4 5 6 7 8 9 10 11 12 13 14 15 16 17 18 19 20 21 22 23 24 25 26 27 28 29 30 31 32 33 34 35 36 37 38 39 40 41 42 43 44 45 46 47 48 49 50 51 52 53 54 55 56 57 58 59 60 61 62 63 64 65 66 67 68 69 70 71 72 73 74 75 B C D E F G H I J K L M N O P Q R Last-Value Forecasting Method with Seasonality for Cutting Edge Week 1 1 1 1 1 2 2 2 2 2 3 3 3 3 3 4 4 4 4 4 5 5 5 5 5 6 6 6 6 6 7 7 7 7 7 8 8 8 8 8 9 9 9 9 9 10 10 10 10 10 11 11 11 11 11 12 12 12 12 12 13 13 13 13 13 14 14 14 14 14 Day Mon Tue Wed Thur Fri Mon Tue Wed Thur Fri Mon Tue Wed Thur Fri Mon Tue Wed Thur Fri Mon Tue Wed Thur Fri Mon Tue Wed Thur Fri Mon Tue Wed Thur Fri Mon Tue Wed Thur Fri Mon Tue Wed Thur Fri Mon Tue Wed Thur Fri Mon Tue Wed Thur Fri Mon Tue Wed Thur Fri Mon Tue Wed Thur Fri Mon Tue Wed Thur Fri True Value Seasonally Adjusted Value #N/A #N/A #N/A #N/A 798 1,325 1,146 1,037 905 1,047 1,047 1,047 1,047 1,047 #N/A #N/A #N/A #N/A #N/A #N/A #N/A Seasonally Adjusted Forecast Actual Forecast Forecasting Error #N/A #N/A #N/A #N/A 1,047 1,047 1,047 1,047 1,047 1,325 1,146 1,037 905 798 0 0 0 0 Type of Seasonality Daily Day Mon Tue Wed Thur Fri Seasonal Factor 1.265 1.095 0.990 0.865 0.762 #N/A #N/A #N/A #N/A #N/A #N/A #N/A #N/A #N/A #N/A #N/A #N/A #N/A #N/A #N/A #N/A #N/A #N/A #N/A #N/A #N/A #N/A #N/A #N/A #N/A #N/A #N/A #N/A #N/A #N/A #N/A #N/A #N/A #N/A #N/A #N/A #N/A #N/A #N/A #N/A #N/A #N/A #N/A #N/A #N/A #N/A #N/A #N/A #N/A #N/A #N/A #N/A #N/A #N/A #N/A #N/A #N/A #N/A #N/A #N/A #N/A #N/A #N/A #N/A #N/A #N/A #N/A #N/A #N/A #N/A #N/A #N/A #N/A #N/A #N/A #N/A #N/A #N/A #N/A #N/A #N/A #N/A #N/A #N/A #N/A #N/A #N/A #N/A #N/A #N/A #N/A #N/A #N/A #N/A #N/A #N/A #N/A #N/A #N/A #N/A #N/A #N/A #N/A #N/A #N/A #N/A #N/A Cells G6:G75 H6:H75 K23 K26 K9:K20 F6:F75 E6:E75 D6:D75 K6 1.000 1.000 #N/A #N/A #N/A #N/A #N/A #N/A #N/A Range Name ActualForecast ForecastingError MAD MSE SeasonalFactor SeasonallyAdjustedForecast SeasonallyAdjustedValue TrueValue TypeOfSeasonality 1.000 1.000 1.000 1.000 Seasonally-Adjusted Value Mean Absolute Deviation MAD = 0.0 Mean Square Error MSE = Season ally Adjusted Valu e Season ally Adjusted Forecast 0 1,047 Tim e Period A 1 2 3 4 5 6 7 8 9 10 11 12 13 14 15 16 17 18 19 20 21 22 23 24 25 26 27 28 29 30 31 32 33 34 35 36 37 38 39 40 41 42 43 44 45 46 47 48 49 50 51 52 53 54 55 56 57 58 59 60 61 62 63 64 65 66 67 68 69 70 71 72 73 74 B C D E F G H I J K L M N O P Q Moving-Average Forecasting Method with Seasonality for Cutting Edge Week 1 1 1 1 1 2 2 2 2 2 3 3 3 3 3 4 4 4 4 4 5 5 5 5 5 6 6 6 6 6 7 7 7 7 7 8 8 8 8 8 9 9 9 9 9 10 10 10 10 10 11 11 11 11 11 12 12 12 12 12 13 13 13 13 13 14 14 14 14 Day Mon Tue Wed Thur Fri Mon Tue Wed Thur Fri Mon Tue Wed Thur Fri Mon Tue Wed Thur Fri Mon Tue Wed Thur Fri Mon Tue Wed Thur Fri Mon Tue Wed Thur Fri Mon Tue Wed Thur Fri Mon Tue Wed Thur Fri Mon Tue Wed Thur Fri Mon Tue Wed Thur Fri Mon Tue Wed Thur Fri Mon Tue Wed Thur Fri Mon Tue Wed Thur True Value 945 689 723 754 798 1,019 894 846 760 671 Seasonally Adjusted Value 747 629 730 872 1,047 805 817 854 879 880 Seasonally Adjusted Forecast Actual Forecast Forecasting Error Number of previous periods to consider n= #N/A #N/A #N/A #N/A #N/A 805 817 854 879 880 847 #N/A #N/A #N/A #N/A #N/A #N/A #N/A #N/A #N/A #N/A #N/A #N/A #N/A #N/A #N/A #N/A #N/A #N/A #N/A #N/A #N/A #N/A #N/A #N/A #N/A #N/A #N/A #N/A #N/A #N/A #N/A #N/A #N/A #N/A #N/A #N/A #N/A #N/A #N/A #N/A #N/A #N/A #N/A #N/A #N/A #N/A #N/A #N/A #N/A #N/A #N/A #N/A #N/A #N/A #N/A #N/A #N/A #N/A #N/A #N/A #N/A #N/A #N/A #N/A #N/A #N/A #N/A #N/A #N/A #N/A #N/A #N/A #N/A #N/A #N/A #N/A #N/A #N/A #N/A #N/A #N/A #N/A #N/A #N/A #N/A #N/A #N/A #N/A #N/A #N/A #N/A #N/A #N/A #N/A #N/A #N/A #N/A #N/A #N/A #N/A #N/A #N/A #N/A #N/A #N/A #N/A #N/A #N/A #N/A #N/A #N/A #N/A #N/A #N/A #N/A 5 Type of Seasonality Daily 1,019 894 846 760 671 1,072 0 0 0 0 0 Day Mon Tue Wed Thur Fri Seasonal Factor 1.265 1.095 0.990 0.865 0.762 1.000 1.000 1.000 1.000 1.000 1.000 1.000 Range Name ActualForecast ForecastingError MAD MSE NumberOfPeriods SeasonalFactor SeasonallyAdjustedForecast SeasonallyAdjustedValue TrueValue TypeOfSeasonality Cells G6:G75 H6:H75 K26 K29 K6 K12:K23 F6:F75 E6:E75 D6:D75 K9 1,200 1,000 Seasonally-Adjusted Value 800 Seasonally Adjuste Value 600 Mean Absolute Deviation MAD = 0.0 Mean Square Error MSE = 0.0 Seasonally Adjuste Forecast 400 200 0 Time Period A 75 B 14 C Fri D E F #N/A #N/A G H I J K L M N O P Q R 1 2 3 4 5 6 7 8 9 10 11 12 13 14 15 16 17 18 19 20 21 22 easonally Adjusted 23 alue 24 easonally Adjusted 25 orecast 26 27 28 29 30 31 32 33 34 35 36 37 38 39 40 41 42 43 44 45 46 47 48 49 50 51 52 53 54 55 56 57 58 59 60 61 62 63 64 65 66 67 68 69 70 71 72 73 74 A 1 2 3 4 5 6 7 8 9 10 11 12 13 14 15 16 17 18 19 20 21 22 23 24 25 26 27 28 29 30 31 32 33 34 35 36 37 38 39 40 41 42 43 44 45 46 47 48 49 50 51 52 53 54 55 56 57 58 59 60 61 62 63 64 65 66 67 68 69 B C D E F G H I J K L Seasonal Factors for Cutting Edge Case 44 44 44 44 44 45 45 45 45 45 46 46 46 46 46 47 47 47 47 47 48 48 48 48 48 49 49 49 49 49 50 50 50 50 50 51 51 51 51 51 52/1 52/1 52/1 52/1 52/1 2 2 2 2 2 3 3 3 3 3 4 4 4 4 4 5 5 5 5 5 Week 1 1 1 1 1 2 2 2 2 2 3 3 3 3 3 4 4 4 4 4 5 5 5 5 5 6 6 6 6 6 7 7 7 7 7 8 8 8 8 8 9 9 9 9 9 10 10 10 10 10 11 11 11 11 11 12 12 12 12 12 13 13 13 13 13 Day Mon Tue Wed Thur Fri Mon Tue Wed Thur Fri Mon Tue Wed Thur Fri Mon Tue Wed Thur Fri Mon Tue Wed Thur Fri Mon Tue Wed Thur Fri Mon Tue Wed Thur Fri Mon Tue Wed Thur Fri Mon Tue Wed Thur Fri Mon Tue Wed Thur Fri Mon Tue Wed Thur Fri Mon Tue Wed Thur Fri Mon Tue Wed Thur Fri True Value 1,130 750 920 854 698 1,085 1,012 689 920 755 1,403 1,121 1,050 1,113 1,005 2,652 2,825 1,841 2,012 1,345 954 1,022 1,084 1,321 1,056 941 760 695 1,012 833 922 810 784 789 401 429 1,209 789 1,132 890 1,362 1,210 980 950 834 1,012 954 1,346 904 758 923 878 798 1,012 643 945 689 723 754 798 Range Name SeasonalFactor TrueValue TypeOfSeasonality Type of Seasonality Daily Day Mon Tue Wed Thur Fri Estimate for Seasonal Factor 1.265 1.095 0.990 0.865 0.762 Average Call Volume 1,025 1.238 1.131 0.999 0.850 0.762 Cells G10:G21 D5:D69 F5 1.265 1.095 0.990 0.865 0.762 A 70 B C D E F G H I J K L A 1 2 3 4 5 6 7 8 9 10 11 12 13 14 15 16 17 18 19 20 21 22 23 24 25 26 27 28 29 30 31 32 33 34 35 36 37 38 39 40 41 42 43 44 45 46 47 48 49 50 51 52 53 54 55 56 57 58 59 60 61 62 63 64 65 66 67 68 69 70 71 72 73 74 B C D E F G H I J K L M N O P Q R S T Template for Exponential-Smoothing with Trend Forecasting Method with Seasonality Year 1 1 1 1 2 2 2 2 3 3 3 3 4 4 4 4 5 5 5 5 6 6 6 6 7 7 7 7 8 8 8 8 9 9 9 9 10 10 10 10 11 11 11 11 12 12 12 12 13 13 13 13 14 14 14 14 15 15 15 15 16 16 16 16 17 17 17 17 18 Quarter 1 2 3 4 1 2 3 4 1 2 3 4 1 2 3 4 1 2 3 4 1 2 3 4 1 2 3 4 1 2 3 4 1 2 3 4 1 2 3 4 1 2 3 4 1 2 3 4 1 2 3 4 1 2 3 4 1 2 3 4 1 2 3 4 1 2 3 4 1 True Value 6,809 6,465 6,569 8,266 7,257 7,064 7,784 8,724 6,992 6,822 7,949 9,650 Seasonally Adjusted Value 7,322 7,183 6,635 7,005 7,803 7,849 7,863 7,393 7,518 7,580 8,029 8,178 #N/A #N/A #N/A #N/A #N/A #N/A #N/A #N/A #N/A #N/A #N/A #N/A #N/A #N/A #N/A #N/A #N/A #N/A #N/A #N/A #N/A #N/A #N/A #N/A #N/A #N/A #N/A #N/A #N/A #N/A #N/A #N/A #N/A #N/A #N/A #N/A #N/A #N/A #N/A #N/A #N/A #N/A #N/A #N/A #N/A #N/A #N/A #N/A #N/A #N/A #N/A #N/A #N/A #N/A #N/A #N/A #N/A Latest Trend -54 -90 -243 -102 167 187 179 13 32 34 155 176 Estimated Trend 0 -16 -38 -100 -100 -20 42 83 62 53 47 80 108 Seasonally Adjusted Forecast 7,500 7,430 7,318 7,013 6,910 7,158 7,407 7,627 7,619 7,642 7,670 7,858 8,062 #N/A #N/A #N/A #N/A #N/A #N/A #N/A #N/A #N/A #N/A #N/A #N/A #N/A #N/A #N/A #N/A #N/A #N/A #N/A #N/A #N/A #N/A #N/A #N/A #N/A #N/A #N/A #N/A #N/A #N/A #N/A #N/A #N/A #N/A #N/A #N/A #N/A #N/A #N/A #N/A #N/A #N/A #N/A #N/A #N/A #N/A #N/A #N/A #N/A #N/A #N/A #N/A #N/A #N/A #N/A #N/A Actual Forecast 6,975 6,687 7,245 8,276 6,427 6,442 7,333 9,000 7,085 6,877 7,594 9,272 7,498 Forecasting Error 166 222 676 10 830 622 451 276 93 55 355 378 Smoothing Constant a = b = Initial Estimate Average = Trend = 0.3 0.3 7,500 0 Type of Seasonality Quarterly Quarter 1 2 3 4 Seasonal Factor 0.93 0.90 0.99 1.18 1.00 1.00 1.00 1.00 1.00 1.00 1.00 1.00 Mean Absolute Deviation MAD = 345 Range Name ActualForecast Alpha Beta ForecastingError InitialEstimateAverage InitialEstimateTrend MAD MSE SeasonalFactor SeasonallyAdjustedForecast SeasonallyAdjustedValue TrueValue TypeOfSeasonality Cells I6:I75 M5 M6 J6:J75 M9 M10 M30 M33 M16:M27 H6:H75 E6:E75 D6:D75 M13 9,000 8,000 7,000 Seasonally-Adjusted Value 6,000 5,000 Seasonally Adjusted Value 4,000 Seasonally Adjusted Forecast 3,000 Mean Square Error MSE = 180,796 2,000 1,000 0 Time Period A 75 B 18 C 2 D E #N/A F G H #N/A I J K L M N O P Q R S T U 1 2 3 4 5 6 7 8 9 10 11 12 13 14 15 16 17 18 19 20 21 22 23 24 25 26 27 djusted Value 28 29 Forecast djusted 30 31 32 33 34 35 36 37 38 39 40 41 42 43 44 45 46 47 48 49 50 51 52 53 54 55 56 57 58 59 60 61 62 63 64 65 66 67 68 69 70 71 72 73 74 A 1 2 3 4 5 6 7 8 9 10 11 12 13 14 15 16 17 18 19 20 21 22 23 24 25 26 27 28 29 30 31 32 33 34 35 B C D E F G H I J K L M Template for Exponential Smoothing Forecasting Method Time Period 1 2 3 4 5 6 7 8 9 10 11 12 13 14 15 16 17 18 19 20 21 22 23 24 25 26 27 28 29 30 True Value 6,809 6,465 6,569 8,266 7,257 7,064 7,784 8,724 6,992 6,822 7,949 9,650 Exponential Smoothing Forecast 7,500 7,155 6,810 6,689 7,478 7,367 7,216 7,500 8,112 7,552 7,187 7,568 8,609 #N/A #N/A #N/A #N/A #N/A #N/A #N/A #N/A #N/A #N/A #N/A #N/A #N/A #N/A #N/A #N/A #N/A Forecasting Error 691 690 241 1,577 221 303 568 1,224 1,120 730 762 2,082 Smoothing Constant a = Initial Estimate Average = 0.5 7,500 Mean Absolute Deviation MAD = 851 Range Name Alpha Forecast ForecastingError InitialEstimate MAD MSE TrueValue Cells H6 D6:D35 E6:E35 H9 H12 H15 C6:C35 Mean Square Error MSE = 1,013,449 12,000 10,000 8,000 Value 6,000 True Value Forecast 4,000 2,000 0 Time Period N A 1 B C D E F G H I J K L M N O Template for Exponential Smoothing Forecasting Method with Trend 2 3 4 5 6 7 8 9 10 11 12 13 14 15 16 17 18 19 20 21 22 23 24 25 26 27 28 29 30 31 32 33 34 35 36 37 38 39 40 Time Period 1 2 3 4 5 6 7 8 9 10 11 12 13 14 15 16 17 18 19 20 21 22 23 24 25 26 27 28 29 30 True Value 6,809 6,465 6,569 8,266 7,257 7,064 7,784 8,724 6,992 6,822 7,949 9,650 Latest Trend -207.30 -291.84 -221.32 335.23 35.57 -23.40 193.95 459.45 -113.06 -190.92 145.57 641.63 Estimated Trend 0.00 -62.19 -131.09 -158.16 -10.14 3.57 -4.52 55.02 176.35 89.53 5.40 47.45 225.70 Exponential Smoothing Forecast 7,500 7,231 6,870 6,621 7,105 7,154 7,122 7,376 7,957 7,757 7,482 7,669 8,489 #N/A #N/A #N/A #N/A #N/A #N/A #N/A #N/A #N/A #N/A #N/A #N/A #N/A #N/A #N/A #N/A #N/A Forecasting Error 691 766 301 1,645 152 90 662 1,348 965 935 467 1,981 Smoothing Constants a = 0.3 b = 0.3 Initial Estimates Average = Trend = 7,500 0 Mean Absolute Deviation MAD = 833.4 Range Name Alpha Beta EstimatedTrend Forecast ForecastingError InitialEstimateAverage InitialEstimateTrend LatestTrend MAD MSE TrueValue Cells J6 J7 E6:E35 F6:F35 G6:G35 J10 J11 D6:D35 J14 J17 C6:C35 Mean Square Error MSE = 1,007,557.6 12,000 10,000 8,000 True Value Value 6,000 Forecast 4,000 2,000 0 1 2 3 4 5 6 7 8 9 10 11 12 1314 15 16 1718 19 20 21 22 2324 25 26 2728 29 30 Time Period P A 1 2 3 4 5 6 7 8 9 10 11 12 13 14 15 16 17 18 19 20 21 22 23 24 25 26 27 28 29 30 31 32 33 34 B C D E F G H I J K L M Template for Last-Value Forecasting Method Time Period 1 2 3 4 5 6 7 8 9 10 11 12 13 14 15 16 17 18 19 20 21 22 23 24 25 26 27 28 29 30 True Value 6,809 6,465 6,569 8,266 7,257 7,064 7,784 8,724 6,992 6,822 7,949 9,650 Last-Value Forecast Forecasting Error 6,809 6,465 6,569 8,266 7,257 7,064 7,784 8,724 6,992 6,822 7,949 9,650 344 104 1,697 1,009 193 720 940 1,732 170 1,127 1,701 #N/A #N/A #N/A #N/A #N/A #N/A #N/A #N/A #N/A #N/A #N/A #N/A #N/A #N/A #N/A #N/A #N/A Mean Absolute Deviation MAD = 885 Mean Square Error MSE = 1,150,777 Range Name Forecast ForecastingError MAD MSE TrueValue Cells D5:D34 E5:E34 H5 H8 C5:C34 12,000 10,000 8,000 Value 6,000 True Value Forecast 4,000 2,000 0 Time Period N
Step by Step Solution
There are 3 Steps involved in it
Step: 1
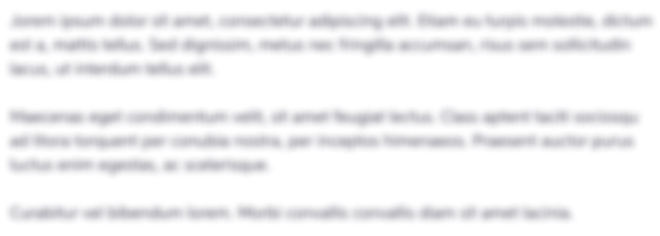
Get Instant Access to Expert-Tailored Solutions
See step-by-step solutions with expert insights and AI powered tools for academic success
Step: 2

Step: 3

Ace Your Homework with AI
Get the answers you need in no time with our AI-driven, step-by-step assistance
Get Started