Answered step by step
Verified Expert Solution
Question
1 Approved Answer
Data Acquisition and Initial Analysis: Retrieve the MNIST dataset. Perform exploratory data analysis to understand the dataset's structure, including i . how many images ii
Data Acquisition and Initial Analysis:
Retrieve the MNIST dataset.
Perform exploratory data analysis to understand the dataset's structure, including
i how many images
ii how many features and the range of feature values eg histogram of the data value
relating it to realworld, such as real images.
iii. how many categorieslabels discrete or continuous type and what they are?
iv visualize at least three randomly selected samples within each category feel the variance
of the data
v visualize more data samples to see whether there are bad data samples need to be
removed. What bad data samples do you think can be
Data Preparation and Manipulation:
Apply dimensionality reduction techniques PCA and tSNE to the MNIST dataset and visualize the
results.
Split the dataset into training samples and testing samples sets.
Machine Learning Model Implementation:
Train a Random Forest classifier on the original dataset and record its performance.
Use PCA to reduce the datasets dimensionality to Train a new Random Forest classifier on the
reduced dataset and see how long it takes. Was training much faster? Then, evaluate the classifier on
the test set. How does it compare to the previous classifier?
Critical Evaluation and Conclusion:
Provide a comprehensive evaluation of the performance of the models.
Summarize findings and insights.
Research Question: Explore how various image preprocessing methods eg normalization, binarization,
noise reduction, and image augmentation influence the performance of at least two different machine
learning models eg Convolutional Neural Networks and Random Forest classifiers trained on the MNIST
dataset. Analyze the models' accuracy, training time, and ability to generalize to test data. Discuss your
findings' implications for designing machine learning pipelines in digit recognition tasks.
Reflect on the composition and diversity of the MNIST dataset, considering its impact on the training process
and model performance. Explore how the inclusion of a more diverse set of handwriting samples eg
different handwriting styles, inclusion of characters from nonLatin alphabets, or samples from wider age
groups might affect the accuracy and generalizability of machine learning models trained for digit
recognition tasks.
Structure
Prepare a jupyter notebook for this assignment. The structure of the Jupyter notebook should alternate texts and
python codes and cover topics listed the in specific tasks above. Always refer to textbook handson machine
learning with ScikitLearn, Keras & TensorFlow for coding help.
How do I submit?
Prepare Your Submission: Ensure your Jupyter notebook ipynb is complete with all required work.
Step by Step Solution
There are 3 Steps involved in it
Step: 1
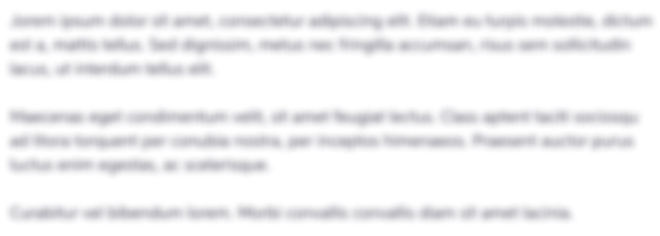
Get Instant Access to Expert-Tailored Solutions
See step-by-step solutions with expert insights and AI powered tools for academic success
Step: 2

Step: 3

Ace Your Homework with AI
Get the answers you need in no time with our AI-driven, step-by-step assistance
Get Started