Answered step by step
Verified Expert Solution
Question
1 Approved Answer
data.txt: Country Deaths Deaths-7-day Increase Population Belgium 20,814 260 35 11.48 Slovenia 3,379 148 19 2.09 United Kingdom 98,339 7,042 590 66.83 Czechia 15,453 807
data.txt:
Country Deaths Deaths-7-day Increase Population Belgium 20,814 260 35 11.48 Slovenia 3,379 148 19 2.09 United Kingdom 98,339 7,042 590 66.83 Czechia 15,453 807 84 10.67 Italy 85,881 2,724 420 60.30 Bosnia and Herzegovina 4,593 84 38 3.30 North Macedonia 2,791 65 6 2.08 USA 419,196 19,281 1,910 328.24 Bulgaria 8,880 266 60 6.98 Moldova 3,368 0 3,368 2.66 Hungary 12,024 504 56 9.77 Peru 39,608 838 0 32.51 Panama 5,098 234 35 4.25 Croatia 4,859 175 32 4.07 Spain 56,208 2,035 767 47.08 Mexico 150,273 7,441 659 127.58 France 72,590 2,146 444 67.06 Sweden 11,005 682 0 10.29 Switzerland 9,146 287 81 8.57 Argentina 47,034 968 207 44.94 Portugal 10,721 1,475 252 10.27 Brazil 217,664 6,173 627 211.05 Armenia 3,047 40 8 2.96 Colombia 51,747 2,345 373 50.34 Lithuania 2,664 170 15 2.79 Chile 17,999 426 66 18.95 Poland 35,401 1,703 38 37.97 Romania 17,841 472 65 19.36 Bolivia 10,051 329 66 11.51 Ecuador 14,639 257 16 17.37 Austria 7,451 252 33 8.88 Georgia 3,071 98 16 3.72 Kosovo 1,466 55 19 1.79 Netherlands 13,472 412 38 17.33 Slovakia 4,068 431 0 5.45 South Africa 41,117 2,829 243 58.56 Iran 57,481 508 98 82.91 Germany 53,127 4,130 831 83.13 Ireland 2,977 269 7 4.94 Latvia 1,114 102 3 1.91 Serbia 3,905 114 19 6.94 Puerto Rico 1,778 75 5 3.19 Tunisia 6,287 443 53 11.69 Greece 5,671 153 25 10.72 Ukraine 23,001 964 75 44.39 Costa Rica 2,558 81 40 5.05 Canada 18,868 579 101 37.59 Israel 4,498 418 79 9.05 Russia 68,841 3,209 444 144.37 Albania 1,324 37 9 2.85 Eswatini 493 90 14 1.15 Jordan 4,239 69 15 10.10 West Bank and Gaza 1,796 55 5 4.69 Paraguay 2,632 97 15 7.04 Honduras 3,462 71 15 9.75 Lebanon 2,374 354 54 6.86 Denmark 2,009 173 27 5.82 Iraq 13,000 38 7 39.31 Guatemala 5,469 156 4 16.60 Azerbaijan 3,093 61 11 10.02 Oman 1,522 6 1 4.97 Turkey 25,210 882 137 83.43 Estonia 383 39 7 1.33 Libya 1,782 82 19 6.78 El Salvador 1,572 63 11 6.45 Dominican Republic 2,545 84 14 10.74 Kuwait 954 4 2 4.21 Bahrain 369 7 2 1.64 Morocco 8,172 161 22 36.47 Kyrgyzstan 1,402 10 2 6.46 Saudi Arabia 6,355 20 3 34.27 Belarus 1,658 57 9 9.47 Kazakhstan 2,961 67 3 18.51 Cyprus 188 12 2 1.20 Namibia 319 23 0 2.49 Finland 655 34 11 5.52 Jamaica 338 9 2 2.95 Uruguay 390 60 14 3.46 India 153,587 869 117 1,366.42 Indonesia 28,132 1,542 297 270.63 Norway 548 23 4 5.35 Trinidad and Tobago 134 2 0 1.39 Philippines 10,292 314 50 108.12 Mauritania 417 12 1 4.53 Egypt 9,012 316 53 100.39 Qatar 248 0 0 2.83 United Arab Emirates 798 42 6 9.77 Zimbabwe 1,075 250 70 14.65 Nepal 2,011 42 10 28.61 Algeria 2,866 23 3 43.05 Equatorial Guinea 86 0 0 1.36 Lesotho 134 33 11 2.13 Afghanistan 2,385 39 7 38.04 Gambia 128 0 0 2.35 Botswana 124 36 19 2.30 Pakistan 11,376 273 58 216.57 Syria 890 40 5 17.07 Bangladesh 8,041 99 18 163.05 Japan 5,193 513 74 126.26 Sudan 1,738 135 135 42.81 Venezuela 1,154 38 6 28.52 Zambia 660 82 21 17.86 Australia 909 0 0 25.36 Senegal 582 46 7 16.30 Kenya 1,744 10 0 52.57 Gabon 67 1 0 2.17 Malawi 518 182 10 18.63 South Korea 1,371 71 11 51.71 Nicaragua 168 0 0 6.55 Guinea-Bissau 45 0 0 1.92 Congo (Brazzaville) 117 3 0 5.38 Haiti 243 3 0 11.26 Malaysia 689 70 11 31.95 Yemen 615 3 0 29.16 Uzbekistan 621 1 0 33.58 Ethiopia 2,071 34 5 112.08 Cameroon 462 7 0 25.88 Cuba 197 22 3 11.33 Liberia 84 0 0 4.94 Mali 324 7 1 19.66 Angola 461 19 2 31.83 Rwanda 177 29 3 12.63 Central African Republic 63 0 0 4.75 Sri Lanka 287 14 4 21.80 Ghana 372 14 5 30.42 Mozambique 363 110 58 30.37 Madagascar 279 6 0 26.97 Sierra Leone 77 0 0 7.81 Tajikistan 90 0 0 9.32 Togo 76 2 1 8.08 Somalia 130 0 0 15.44 Mauritius 10 0 0 1.27 Congo (Kinshasa) 661 21 0 86.79 Nigeria 1,507 43 3 200.96 Chad 116 2 0 15.95 Uganda 318 13 1 44.27 Niger 151 5 0 23.31 Guinea 81 0 0 12.77 South Sudan 64 0 0 11.06 Burkina Faso 117 12 5 20.32 Cote d'Ivoire 146 4 0 25.72 Singapore 29 0 0 5.70 New Zealand 25 0 0 4.92 Benin 48 2 0 11.80 China 4,807 7 3 1,397.72 Eritrea 7 1 1 3.50 Thailand 75 4 2 69.63 Papua New Guinea 9 0 0 8.78 Mongolia 2 0 0 3.23 Vietnam 35 0 0 96.46
strings.txt:
'Enter a file name for writing: ' 'Enter a file name for reading: ' "Error opening file." "{:10s}{:>14s}{:>14s}" "{:10,d}{:>14,.2f}{:>14,.2f}" " Countries with higher death rates than USA per million."Assignment Overview An important question about the pandemic is the death rate which is often calculated as deaths per million of population. That rate is one metric for how well a country has responded to the pandemic. Once you have the ability to read files in Python you can examine raw data to answer such questions. Program Specifications In this project, you will read a file of data with total Covid deaths and the population of countries. You will then calculate the total deaths per million for countries. Assigning deaths to Covid is not consistently handled among countries and often not consistently within countries. However, there is more consistency with first-world countries so we will extract data for the G20 countries. (There isn't anything special about the G20 except that it makes the assignment more interesting.) Since we are dealing with real data there will be complications. For example, there are columns of data that we aren't interested in such as the deaths in the last seven days and there are rows of data that we aren't interested in such as non-G20 countries. There is one header row that describe columns but they are not data so we need to ignore them. Also, some data is in the thousands with commas and Python cannot directly convert a number with commas into a Python number such as an int or float. You will be asked to write a function to handle commas. Deaths-per-Million high-level algorithm Your high-level algorithm will be: 1. Open an input file for reading 2. Open a different file for writing 3. Write header information to the output file. 4. Loop through the input file, reading it line by line a. Call a function to process each line i. Call a function to handle commas b. Calculate deaths/million-population c. If the data is for a G20 country, display it and write it to a file 5. Close both files 6. Display which countries have deaths-per-million worse than the US File Specification The input file named data.txt has one header line and five fixed-field columns Column 0 is a country name in a 25-character field), columns 1, 2, and 3 are integers, and column 4 is a float. The numbers are in 10-character fields. A file such as data.txt with fixed-field columns allows you to use string slicing to extract individual values. Your program must also meet the following specifications: 1. You must have and use at least these four functions-more are fine. A proj05.py file with function stubs is provided. a. def open_file(ch) file_pointer i. If ch is 'w', open a file for writing; otherwise open a file for reading. Repeatedly prompt for a file name until a file is successfully opened. Use the try-except command. Usually, we would use except FileNotFoundError, but that works only for opening a file for reading so we want to use the more general file error for reading and writing so use except IOError. If ch is 'w', the prompt should be Enter a file name for writing: else the prompt should be 'Enter a file name for reading: ii. Parameters: ch iii. Returns: file_pointer iv. Display: prompt and error message as appropriate b. def handle_commas (s, t) int or float or None i. The parameters are s, a string, and T, a string. The expected values of T is the word"int" or "float"; any other value returns None. If the value of T is "int", the string s will be converted to an int and that int value will be returned. Similar for "float". In both cases, you have to remove the commas from s before converting to intor float. If a value of s cannot be converted to an int or float, None will be returned (hint: use try-except) ii. Parameters: str, str iii. Returns: int or float or None iv. Display: nothing c. def process_line (line) str, int , float i. The parameter is a string which is a line from the file. Extract the country (string in a 25-character field), deaths (int in a 10-character field), and population (float in a 10-character field from the END of the line). Strip leading and trailing spaces from the country name. Return the country, deaths, and population. Use the function handle_commas to process the numbers for deaths and population. ii. Parameters: str iii. Returns: str, int , float iv. Display: nothing d. def main() Your main algorithm will be as follows calling the above functions. i. Call open_file to open an input file for reading ii. Call open_file to open a different file for writing iii. Write header information (2 lines) to the output file and to the console. - The header information in the first line is: 'Country', 'Deaths', 'Population', 'Death Rate' - The header information in the second line is: "Millions', 'per Million For both lines, use the following formatting for the headers also provided in the strings.txt file): "{:108}{:>14s}{:>14s)" iv. Loop through the input file, reading it line by line 1. Call a function to process each line a. Call a function to handle commas 2. Calculate deaths/million-population 3. If the data is for a G20 country, display it and write it to the output file. use the following string formatting for the numbers (also provided in the strings.txt file): "{:10,d}{:>14,.20H:>14,.2f}" v. Close both files vi. Display which countries have deaths-per-million worse than the US To determine which countries are worse than the US we provide the deaths-per- million for the US (1277.10). Note that the US rate is rounded to 2 digits after the decimal point. Start with an empty string before the loop and build a comma- separated string of the countries that you will display after the loop. Note that this item is not written to the output file. It is only written to the console. Deliverables The deliverable for this assignment is the following file: proj05.py -- your source code solution Be sure to use the specified file name and to submit it for grading via Mimir before the project deadline Notes and Hints: 1. To clarify the project specifications, sample output is appended to the end of this document. 2. Items 1-9 of the Coding Standard will be enforced for this project-note the change to include more items. 3. You can test functions separately that can be a huge advantage in developing correct code faster! If you cannot figure out how to do that, ask your TA for guidance. 4. We provide a string with the names of G20 countries. Hint use the string operator in to see if a country is in the G20 G20 = "Argentina, Australia, Brazil, Canada, China, France, Germany, India, Indonesia, Italy, Japan, South Korea, Mexico, Russia, Saudi Arabia, South Africa, Turkey, United Kingdom, USA, European Union" 5. We also provide a constant for the US death rate per Millions. Hint use this constant to look for the countries that have death rates high than the US. Note that the constant is rounded to 2 digits after the decimal point. US_RATE = 1277.10 6. Do not hard code your solutions the result is a zero. Hard coding means that your program for the specific tests rather than having a generic solution. Sample Interaction: Function Test handle_commaso S,T: 5 int Instructor: 5 Student : 5 S, T: 5.3 float Instructor: 5.3 Student : 5.3 S,T: 1,234 int Instructor: 1234 Student : 1234 S, T: 1,234.56 float Instructor: 1234.56 Student : 1234.56 S, T: 5.3 xxx Instructor: None Student : None S, T: aaa int Instructor: None Student : None s,T: 1,234.56 int Instructor: None Student : None Function Test process_line 3,596 336 35 11.69 line: Tunisia Instructor: Tunisia 3596 11.69 Student : Tunisia 3596 11.69 4,746 3 0 1,397.72 line: China Instructor: China 4746 1397.72 Student : China 4746 1397.72 140,958 2,836 385 1, 366.42 line: India Instructor: India 140958 1366.42 Student : India 140958 1366.42 Test Case 1 Enter a file name for reading: data.txt Enter a file name for writing: outfile.txt Country Deaths Population Millions United Kingdom 98,339 66.83 Italy 85,881 60.30 USA 419, 196 328.24 Mexico 150,273 127.58 France 72,590 67.06 Argentina 47,034 44.94 Brazil 217,664 211.05 South Africa 41,117 58.56 Germany 53, 127 83.13 Canada 18,868 37.59 Russia 68,841 144.37 Turkey 25,210 83.43 Saudi Arabia 6,355 34.27 India 153,587 1,366.42 Indonesia 28, 132 270.63 Japan 5,193 126.26 Australia 909 25.36 South Korea 1,371 51.71 China 4,807 1,397.72 Death Rate per Million 1,471.48 1, 424.23 1,277.10 1,177.87 1,082.46 1,046.60 1,031.34 702.13 639.08 501.94 476.84 302.17 185.44 112.40 103.95 41.13 35.84 26.51 3.44 Countries with higher death rates than USA per million. United Kingdom, Italy Test Case 2 Enter a file name for reading: xxx Error opening file. Enter a file name for reading: yyyy.txt Error opening file. Enter a file name for reading: data.txt Enter a file name for writing: xxx/xxx Error opening file. Enter a file name for writing: outfile.txt Country Deaths Population Death Rate Millions per Million United Kingdom 98,339 66.83 1,471.48 Italy 85,881 60.30 1, 424.23 USA 419, 196 328.24 1,277.10 Mexico 150,273 127.58 1,177.87 France 72,590 67.06 1,082.46 Argentina 47,034 44.94 1,046.60 Brazil 217,664 211.05 1,031.34 South Africa 41, 117 58.56 702.13 Germany 53, 127 83.13 639.08 Canada 18,868 37.59 501.94 Russia 68,841 144.37 476.84 Turkey 25, 210 83.43 302.17 Saudi Arabia 6,355 34.27 185.44 India 153,587 1,366.42 112.40 Indonesia 28,132 270.63 103.95 Japan 5,193 126.26 41.13 Australia 909 25.36 35.84 South Korea 1, 371 51.71 26.51 China 4,807 1,397.72 3.44 Countries with higher death rates than USA per million. United Kingdom, Italy Scoring Rubric Computer Project #05 Scoring Summary General Requirements 5 pts Coding Standard 1-9 (descriptive comments, function header, etc...) Implementation: 0 (7 pts) 0 (7 pts) open_file (manual grading) Function Test handle_commas Function Test process_line 0 (7 pts) (5 pts) Test 1 oooo 0 (5 pts) Test 2 (4 pts) Test 3 (same as Test 1 but checking if output file is correct) Assignment Overview An important question about the pandemic is the death rate which is often calculated as deaths per million of population. That rate is one metric for how well a country has responded to the pandemic. Once you have the ability to read files in Python you can examine raw data to answer such questions. Program Specifications In this project, you will read a file of data with total Covid deaths and the population of countries. You will then calculate the total deaths per million for countries. Assigning deaths to Covid is not consistently handled among countries and often not consistently within countries. However, there is more consistency with first-world countries so we will extract data for the G20 countries. (There isn't anything special about the G20 except that it makes the assignment more interesting.) Since we are dealing with real data there will be complications. For example, there are columns of data that we aren't interested in such as the deaths in the last seven days and there are rows of data that we aren't interested in such as non-G20 countries. There is one header row that describe columns but they are not data so we need to ignore them. Also, some data is in the thousands with commas and Python cannot directly convert a number with commas into a Python number such as an int or float. You will be asked to write a function to handle commas. Deaths-per-Million high-level algorithm Your high-level algorithm will be: 1. Open an input file for reading 2. Open a different file for writing 3. Write header information to the output file. 4. Loop through the input file, reading it line by line a. Call a function to process each line i. Call a function to handle commas b. Calculate deaths/million-population c. If the data is for a G20 country, display it and write it to a file 5. Close both files 6. Display which countries have deaths-per-million worse than the US File Specification The input file named data.txt has one header line and five fixed-field columns Column 0 is a country name in a 25-character field), columns 1, 2, and 3 are integers, and column 4 is a float. The numbers are in 10-character fields. A file such as data.txt with fixed-field columns allows you to use string slicing to extract individual values. Your program must also meet the following specifications: 1. You must have and use at least these four functions-more are fine. A proj05.py file with function stubs is provided. a. def open_file(ch) file_pointer i. If ch is 'w', open a file for writing; otherwise open a file for reading. Repeatedly prompt for a file name until a file is successfully opened. Use the try-except command. Usually, we would use except FileNotFoundError, but that works only for opening a file for reading so we want to use the more general file error for reading and writing so use except IOError. If ch is 'w', the prompt should be Enter a file name for writing: else the prompt should be 'Enter a file name for reading: ii. Parameters: ch iii. Returns: file_pointer iv. Display: prompt and error message as appropriate b. def handle_commas (s, t) int or float or None i. The parameters are s, a string, and T, a string. The expected values of T is the word"int" or "float"; any other value returns None. If the value of T is "int", the string s will be converted to an int and that int value will be returned. Similar for "float". In both cases, you have to remove the commas from s before converting to intor float. If a value of s cannot be converted to an int or float, None will be returned (hint: use try-except) ii. Parameters: str, str iii. Returns: int or float or None iv. Display: nothing c. def process_line (line) str, int , float i. The parameter is a string which is a line from the file. Extract the country (string in a 25-character field), deaths (int in a 10-character field), and population (float in a 10-character field from the END of the line). Strip leading and trailing spaces from the country name. Return the country, deaths, and population. Use the function handle_commas to process the numbers for deaths and population. ii. Parameters: str iii. Returns: str, int , float iv. Display: nothing d. def main() Your main algorithm will be as follows calling the above functions. i. Call open_file to open an input file for reading ii. Call open_file to open a different file for writing iii. Write header information (2 lines) to the output file and to the console. - The header information in the first line is: 'Country', 'Deaths', 'Population', 'Death Rate' - The header information in the second line is: "Millions', 'per Million For both lines, use the following formatting for the headers also provided in the strings.txt file): "{:108}{:>14s}{:>14s)" iv. Loop through the input file, reading it line by line 1. Call a function to process each line a. Call a function to handle commas 2. Calculate deaths/million-population 3. If the data is for a G20 country, display it and write it to the output file. use the following string formatting for the numbers (also provided in the strings.txt file): "{:10,d}{:>14,.20H:>14,.2f}" v. Close both files vi. Display which countries have deaths-per-million worse than the US To determine which countries are worse than the US we provide the deaths-per- million for the US (1277.10). Note that the US rate is rounded to 2 digits after the decimal point. Start with an empty string before the loop and build a comma- separated string of the countries that you will display after the loop. Note that this item is not written to the output file. It is only written to the console. Deliverables The deliverable for this assignment is the following file: proj05.py -- your source code solution Be sure to use the specified file name and to submit it for grading via Mimir before the project deadline Notes and Hints: 1. To clarify the project specifications, sample output is appended to the end of this document. 2. Items 1-9 of the Coding Standard will be enforced for this project-note the change to include more items. 3. You can test functions separately that can be a huge advantage in developing correct code faster! If you cannot figure out how to do that, ask your TA for guidance. 4. We provide a string with the names of G20 countries. Hint use the string operator in to see if a country is in the G20 G20 = "Argentina, Australia, Brazil, Canada, China, France, Germany, India, Indonesia, Italy, Japan, South Korea, Mexico, Russia, Saudi Arabia, South Africa, Turkey, United Kingdom, USA, European Union" 5. We also provide a constant for the US death rate per Millions. Hint use this constant to look for the countries that have death rates high than the US. Note that the constant is rounded to 2 digits after the decimal point. US_RATE = 1277.10 6. Do not hard code your solutions the result is a zero. Hard coding means that your program for the specific tests rather than having a generic solution. Sample Interaction: Function Test handle_commaso S,T: 5 int Instructor: 5 Student : 5 S, T: 5.3 float Instructor: 5.3 Student : 5.3 S,T: 1,234 int Instructor: 1234 Student : 1234 S, T: 1,234.56 float Instructor: 1234.56 Student : 1234.56 S, T: 5.3 xxx Instructor: None Student : None S, T: aaa int Instructor: None Student : None s,T: 1,234.56 int Instructor: None Student : None Function Test process_line 3,596 336 35 11.69 line: Tunisia Instructor: Tunisia 3596 11.69 Student : Tunisia 3596 11.69 4,746 3 0 1,397.72 line: China Instructor: China 4746 1397.72 Student : China 4746 1397.72 140,958 2,836 385 1, 366.42 line: India Instructor: India 140958 1366.42 Student : India 140958 1366.42 Test Case 1 Enter a file name for reading: data.txt Enter a file name for writing: outfile.txt Country Deaths Population Millions United Kingdom 98,339 66.83 Italy 85,881 60.30 USA 419, 196 328.24 Mexico 150,273 127.58 France 72,590 67.06 Argentina 47,034 44.94 Brazil 217,664 211.05 South Africa 41,117 58.56 Germany 53, 127 83.13 Canada 18,868 37.59 Russia 68,841 144.37 Turkey 25,210 83.43 Saudi Arabia 6,355 34.27 India 153,587 1,366.42 Indonesia 28, 132 270.63 Japan 5,193 126.26 Australia 909 25.36 South Korea 1,371 51.71 China 4,807 1,397.72 Death Rate per Million 1,471.48 1, 424.23 1,277.10 1,177.87 1,082.46 1,046.60 1,031.34 702.13 639.08 501.94 476.84 302.17 185.44 112.40 103.95 41.13 35.84 26.51 3.44 Countries with higher death rates than USA per million. United Kingdom, Italy Test Case 2 Enter a file name for reading: xxx Error opening file. Enter a file name for reading: yyyy.txt Error opening file. Enter a file name for reading: data.txt Enter a file name for writing: xxx/xxx Error opening file. Enter a file name for writing: outfile.txt Country Deaths Population Death Rate Millions per Million United Kingdom 98,339 66.83 1,471.48 Italy 85,881 60.30 1, 424.23 USA 419, 196 328.24 1,277.10 Mexico 150,273 127.58 1,177.87 France 72,590 67.06 1,082.46 Argentina 47,034 44.94 1,046.60 Brazil 217,664 211.05 1,031.34 South Africa 41, 117 58.56 702.13 Germany 53, 127 83.13 639.08 Canada 18,868 37.59 501.94 Russia 68,841 144.37 476.84 Turkey 25, 210 83.43 302.17 Saudi Arabia 6,355 34.27 185.44 India 153,587 1,366.42 112.40 Indonesia 28,132 270.63 103.95 Japan 5,193 126.26 41.13 Australia 909 25.36 35.84 South Korea 1, 371 51.71 26.51 China 4,807 1,397.72 3.44 Countries with higher death rates than USA per million. United Kingdom, Italy Scoring Rubric Computer Project #05 Scoring Summary General Requirements 5 pts Coding Standard 1-9 (descriptive comments, function header, etc...) Implementation: 0 (7 pts) 0 (7 pts) open_file (manual grading) Function Test handle_commas Function Test process_line 0 (7 pts) (5 pts) Test 1 oooo 0 (5 pts) Test 2 (4 pts) Test 3 (same as Test 1 but checking if output file is correct)
Step by Step Solution
There are 3 Steps involved in it
Step: 1
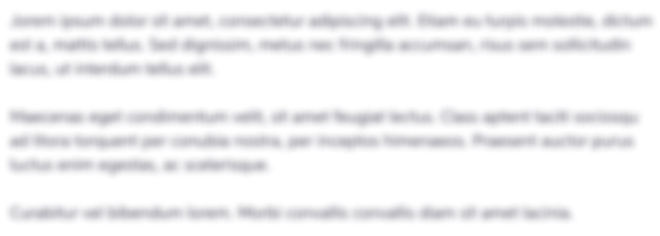
Get Instant Access to Expert-Tailored Solutions
See step-by-step solutions with expert insights and AI powered tools for academic success
Step: 2

Step: 3

Ace Your Homework with AI
Get the answers you need in no time with our AI-driven, step-by-step assistance
Get Started