Question
Develop Model Approach Select Source Task . You must select a related predictive modeling problem with an abundance of data where there is some relationship
Develop Model Approach
- Select Source Task. You must select a related predictive modeling problem with an abundance of data where there is some relationship in the input data, output data, and/or concepts learned during the mapping from input to output data.
- Develop Source Model. Next, you must develop a skillful model for this first task. The model must be better than a naive model to ensure that some feature learning has been performed.
- Reuse Model. The model fit on the source task can then be used as the starting point for a model on the second task of interest. This may involve using all or parts of the model, depending on the modeling technique used.
- Tune Model. Optionally, the model may need to be adapted or refined on the input-output pair data available for the task of interest.
Pre-trained Model Approach
- Select Source Model. A pre-trained source model is chosen from available models. Many research institutions release models on large and challenging datasets that may be included in the pool of candidate models from which to choose from.
- Reuse Model. The model pre-trained model can then be used as the starting point for a model on the second task of interest. This may involve using all or parts of the model, depending on the modeling technique used.
- Tune Model. Optionally, the model may need to be adapted or refined on the input-output pair data available for the task of interest.
This second type of transfer learning is common in the field of deep learning.
Examples of Transfer Learning with Deep Learning
Let's make this concrete with two common examples of transfer learning with deep learning models.
Transfer Learning with Image Data
It is common to perform transfer learning with predictive modeling problems that use image data as input.
This may be a prediction task that takes photographs or video data as input.
For these types of problems, it is common to use a deep learning model pre-trained for a large and challenging image classification task such as the ImageNet 1000-class photograph classification competition.
The research organizations that develop models for this competition and do well often release their final model under a permissive license for reuse. These models can take days or weeks to train on modern hardware.
These models can be downloaded and incorporated directly into new models that expect image data as input.
Three examples of models of this type include:
- Oxford VGG Model
- Google Inception Model
- Microsoft ResNet Model
For more examples, see the Caffe Model Zoo where more pre-trained models are shared.
This approach is effective because the images were trained on a large corpus of photographs and require the model to make predictions on a relatively large number of classes, in turn, requiring that the model efficiently learn to extract features from photographs in order to perform well on the problem.
In their Stanford course on Convolutional Neural Networks for Visual Recognition, the authors caution to carefully choose how much of the pre-trained model to use in your new model.
[Convolutional Neural Networks] features are more generic in early layers and more original-dataset-specific in later layers
Transfer Learning, CS231n Convolutional Neural Networks for Visual Recognition
Transfer Learning with Language Data
It is common to perform transfer learning with natural language processing problems that use text as input or output.
For these types of problems, a word embedding is used that is a mapping of words to a high-dimensional continuous vector space where different words with a similar meaning have a similar vector representation.
Efficient algorithms exist to learn these distributed word representations and it is common for research organizations to release pre-trained models trained on very large corpa of text documents under a permissive license.
Two examples of models of this type include:
- Google's word2vec Model
- Stanford's GloVe Model
These distributed word representation models can be downloaded and incorporated into deep learning language models in either the interpretation of words as input or the generation of words as output from the model.
In his book on Deep Learning for Natural Language Processing, Yoav Goldberg cautions:
... one can download pre-trained word vectors that were trained on very large quantities of text [...] differences in training regimes and underlying corpora have a strong influence on the resulting representations, and that the available pre-trained representations may not be the best choice for [your] particular use case
Step by Step Solution
There are 3 Steps involved in it
Step: 1
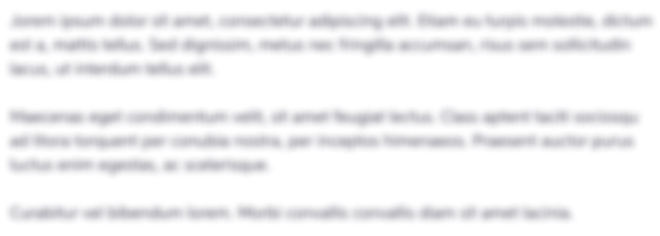
Get Instant Access to Expert-Tailored Solutions
See step-by-step solutions with expert insights and AI powered tools for academic success
Step: 2

Step: 3

Ace Your Homework with AI
Get the answers you need in no time with our AI-driven, step-by-step assistance
Get Started