Question
Economic historian Fabian Waldinger has done research to understand the consequences of the expulsion of professors from German universities during the period of the rise
Economic historian Fabian Waldinger has done research to understand the consequences of the expulsion of professors from German universities during the period of the rise of the National Socialist German Workers' (Nazi) Party for the productivity and outcomes of Ph.D. students and peer researchers. Waldinger look at the expulsion of high-profile scientists from Germany by the Nazis in the early 1930s and estimate the effects that this had on the researchers remaining in Germany at the time of the expulsion of scientists (so both are related to "peer" effects in productivity). Dismissals were based entirely on family history, religion, and political views and can be thought of as strictly exogenous. The goal of the assignment is to implement the differences-in-differences design (i.e., two-way fixed effects panel data models) to estimate causal effects on the research outcomes of interest. References Waldinger, Fabian (2010). "Quality Matters: The Expulsion of Professors and the Consequences for PhD Student Outcomes in Nazi Germany." Journal of Political Economy, 118(4), 787-831. Waldinger, Fabian (2012). "Peer Effects in Science: Evidence from the Dismissal of Scientists in Nazi Germany." Review of Economic Studies, 79, 838-861. Datasets
2 follow thesuggestions below. The datasets available for the assignment are versions of the ones constructed and used by Waldinger (2015). Dataset #1: Ph.D. Student Researcher Characteristics and Outcomes We will use the following variables in this dataset: Ph.D. Students' Characteristics: phduni: ID of university where student graduated from phdyear: year of graduation female: female student indicator foreign: foreign student indicator Outcomes: ordprof: indicator whether student becomes full professor publishedtop: indicator whether student publishes dissertation in top journal lifetimecit: number of lifetime citations of former student Faculty measures: avg_quality: average faculty quality measured by citations in top journals cohort_profs: students per professor (all mathematics students in department) change_avg_quality: measures how much faculty quality changes due to dismissal. 0 for all pre dismissal years and equal to quality change thereafter (remains 0 for departments without dismissals). change_cohort_profs: measures how much students per professors change due to dismissal Other variables: phduni331 - phduni3333: set of dummy variables equal to 1 for the university that the PhD student graduated from (if she graduated before 1933) or for the university that she attended at the beginning of 1933 (if the student graduated after 1933). fatheroccd1 - fatheroccd27: father occupation code ID's *** Dataset #2: Peer Researcher Characteristics and Outcomes We will use the following three (3) variables in this dataset: Lcit50_peersub_avg_: the peer quality in a person's department pub_: the person's productivity as measured by publications uni_: is the university that the person belongs to
3 Questions We want to test whether the change in faculty quality induced by the Nazi dismissals reduced the productivity of those that were not dismissed. Note: Before starting an analysis, it is good practice to save your control set as a macro so that your specifications are consistent throughout and your code is transparent. Using the global command set a control set to include phd year fixed effects, father occupation fixed effects, the female and foreign student ID variables and the phduni33 ID variables. 1. Using dataset 1, regress each of the three outcomes on the change in department quality and controls stated above. Then, regress each of the outcomes on the change in cohort size and the controls. What can you conclude about productivity spillovers? [5] 2. Explain how this model can be thought of as a difference-in-differences model. [5] 3. What do you think of the standard errors in question (1)? Do you think we should cluster them and why? If we did cluster standard errors, at what level should we cluster? What happens after this step? What does this say about the structure of the observations and about inference via CRVE standard errors? [5] 4. Using dataset 2, regress the number of publications (pub_) on a measure of peer quality at the department level (Lcit50_peersub_avg_), with clustering of standard errors at the level of the university. Is there a positive effect of peer quality on output? Can we interpret the relationship as causal? Why or why not? Explain. [5] 5. Somebody suggests we manually compute the within estimator (based on uni_). Manually program the within-effects estimator for them that controls for university fixed effects. How do the estimates change and why do you think the estimates change? What words of warning would you give them about either the estimates or the standard errors that they'll get using your program? [5] 6. We know there's a better way. Estimate the same model using the dummy variable method. Are the estimates different? How about the standard errors? Why do we see any differences? [5]
Step by Step Solution
There are 3 Steps involved in it
Step: 1
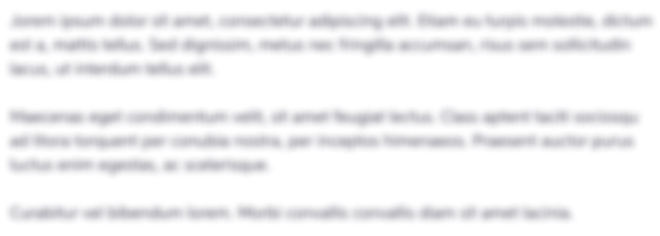
Get Instant Access to Expert-Tailored Solutions
See step-by-step solutions with expert insights and AI powered tools for academic success
Step: 2

Step: 3

Ace Your Homework with AI
Get the answers you need in no time with our AI-driven, step-by-step assistance
Get Started