Answered step by step
Verified Expert Solution
Question
1 Approved Answer
Elena Modesti Final Statistics Paper Social Statistics Professor Charles 4/24/16 The study of the relationship between different social factors is very important in understanding cause
Elena Modesti Final Statistics Paper Social Statistics Professor Charles 4/24/16 The study of the relationship between different social factors is very important in understanding cause and effect, as well as the variables that play a role in the dynamics of society. The two variables we will be exploring in this paper are excessive drinking and annual income. We will define excessive drinking by finding the average number of drinks someone has on a regular basis, and doubling that amount. Annual income will be defined as the amount of money an individual makes from their job in one year. In other words, annual income is an individual's yearly salary. Excessive drinking will be the independent variable in this study, and annual income will be the dependent variable. I want to explore what the relationship is between these two variables and how one affects the other. Excessive drinking is often thought of as an impediment to work place and social functioning, since it's effects don't necessarily jive with the lifestyle required in a high earning career. Although there are certainly other variables that affect individuals yearly earnings, excessive drinking is not conducive to the lifestyle that individuals with high earning jobs usually have. As a result, my broad research question is \"what is relationship between regularly excessive drinking and annual earnings? \"My hypothesis regarding this research question is that there is a negative relationship between an individuals tendency to engage in excessive drinking, and the annual earnings of that same individual. In other words, if an individual is an excessive drinker, then their annual salary is likely to be lower. The competing, or null hypothesis is that there is no relationship between the two variables. Another alternate hypothesis is that there is a positive relationship between excessive drinking and annual salary. However, our predicted outcome is that there is a negative relationship. Studies regarding the relationship between excessive drinking and income have certainly been conducted in the past. Excessive drinking and binge drinking are similar concepts. Binge drinking is especially common in high school and college. The problem begins during adolescence and continues to be a problem in adulthood, according the study \"Vital Signs: Binge Drinking Among High School Students and Adults,\" published in 2009 by Morbidity and Mortality Weekly Report. The report clarifies that most youth who partake in drinking also partake in binge drinking. The two frequently go hand in hand. Other studies focused on the effects of binge drinking claim that drinking and income have a positive relationship, such as the study \"Smoking, Drinking and Income\" published by M. Christopher Auld in 2005. This paper suggests that moderate and heavy drinking can actually increase the chances of a person earning a higher income, but that smoking is negatively correlated with these chances. Further studies explore the consequences of binge drinking during adolescences, highlighting the non conduciveness of binge drinking and later success. The study titled \"Adult Outcomes of Binge Drinking in Adolescence\" by RM Viner and B Taylor in 2007 elucidate that binge drinking at an early age causes more adversity in later life, as well as social exclusion that creates social inequalities in adulthood. All these studies touch on the unique effects excessive drinking has on life trajectories, most of them being negative. However, I want to specifically address how excessive drinking past adolescence effects an individuals career trajectory, specifically their ability to acquire a high earning job. I want to not only contradict that heavy drinking leads to higher earnings, but also confirm that there is a negative relationship between drinking excessively having a high earning job. These studies elucidate the effects excessive drinking, but do not explicitly spell out the relationship excessive drinking has on whether an individual will have a high earning job. That is what I will be arguing in the rest of this paper. I will be using the GSS as a data source. GSS stands for \"The General Social Survey,\" which collects information and keeps a record of the information of residents of the United States. It has been around since 1972, and monitors changes in society as well as the changing complexity of the American population. My variables will be chosen based on how well they can reflect excessive drinking or annual income. The first order of business is to find the mean amount of drinks a random sample of a population has when drinking. This will determine the average amount of drinks a person has when consuming alcohol. In order to define excessive drinking, we will double this mean and use that value as the threshold for excessive drinking. After determining this value, we will take the same population and run a comparison between drinks respondents have on a day when drinking and the respondent's annual income. With this data we can see whether there is a relationship between the two variables, by finding the regression value. If there is a negative relationship, the regression value will be closer to -1 than 1. If there is a positive relationship, the regression value will be closer to +1. We will use \"drinkday\" as a variable to measure drinking and \"rincome\" to measure the respondent's income. It's also important to calculate the coefficient of determination to see what percent of the variation in dependent variable (excessive drinking) can be explained by the independent variable (annual income.) In order to reject the null hypothesis that there is no significant relationship between excessive drinking and annual income, we will analyze the p-value in the ANOVA table for the regression analysis. If the pvalue is less than the given level of significance, 0.05, we will be able to reject the null hypothesis. After running the two variables drinkday and rincome in SPSS, I was able to make a number of important conclusions in determining the relationship between excessive drinking and income. In this case we have two variables. \"Respondents income,\" which is in ratio scale but grouped in categories in this case, and \"How many drinks r have on a day when drinking\" which is a discrete variable. The mean for the GSS year for respondents was 1993.02. To begin, the descriptive statistics for drinkday were able to tell me the mean amount of drinks people have on a day of drinking. However, in order to eliminate the effects outliers have on the data set, I am going to focus on the mode as a measure of central tendency. For this particular data set in the GSS, the mode number of drinks people had on a day when drinking was zero. If we further analyze the descriptive statistics for the data set, we can also determine the frequency, percentage and cumulative percentage for the each category in the beginning of the analysis. For example, form the results we can see that 1248 (about 2.1%) of the respondents have incomes less than $1000, 1782 (almost 3%) have in between $1000 to $2999 and so on. We can also determine the percentage distribution for the number of drinks. According to the data, it looks like 59,351 respondents answered \"not applicable\" when asked how many drinks they have on a day when drinking. This accounts for about 99.6% of the respondent pool. 119 participants have 1 drink on a day when drinking which is about .2%, and 66 have 2 drinks which is .1%. It continues on to 12 drinks. We can also analyze the cross-tabulation between the two variables, which shows whether there is an association between the variables. However, there are better ways to determine whether there is an association between the two variables so I will focus on those measures. Furthermore, an ANOVA table was run to see whether there is a significant difference between the mean number of drinks respondents have on a day when drinking in different categories. The ANOVA table generates the P-value between the two variables. If the P value is less than or equal to our significance level, which is 0.05, we reject the null hypothesis in favor of the alternative hypothesis. Here, the p-value is 0.016 which is smaller than .05 implying that we must reject the null hypothesis and conclude that there is a significant difference in group means. ANOVA: How many drinks r have on a day when drinking Sum of Df Mean Square F Sig. 24.488 15 1.633 .016 50172.034 59583 .842 Squares Between Groups Within 1.939 Groups Total 50196.522 59598 The regression analysis is arguably the most important part of the data generated because it answers the question we want to answer. The regression analysis gives the relationship between the two considered variables. Model Summary: Model R R square Adjusted R square Std. Error of the Estimate 1 .009 .000 .000 16.029 Predictors: (Constant), How many drinks r have on a day when drinking To begin, if we analyze the scatter gram made on SPSS between the two variables, we can see that the correlation coefficient value of 0.009 is significant at the .05 significance level. However, this is not practically significant. The scatter gram shows that there are quite a lot of outliers in this dataset, which is affecting our results. On the x axis we have the respondent's income which seems to be clustered mostly in the $10,000 and below, but we also have a group of outlier's clustered in the $100,000 range. This cluster certainly affected the mean income, which was around 8.49. This number is coded as somewhere in the range of $8,000 to $9999. Based on the graph, this mean makes sense. The median income was 8, which is lower because outliers are no longer affecting the central measure of tendency. If we look at our regression model for the two variables, we can see that it is significant at the . 05 level and the p-value is .037. Although the p-value is smaller than .05, thus the null hypothesis can be rejected. However, the R^2 and the adjusted R^2 indicate that this model is not accurate, and therefore it is difficult to make predictions based on the model. Both values are 0 indicating that 0 percent of the independent variable can be explained by the dependent variable. However, the slope and intercept of the scatter plot are significant. The slope value of 1.49 implies that one extra drink is expected to increase the income by 0.149 units on average. ANOVA: Dependent variable: respondents income Predictors (constant), how many drinks r have on a day when drinking Model Sum of Df Mean square F squares Regression 1111.930 1 111.930 4.328 Residual 15311900.419 59597 256.924 Total 15313012.350 59598 Sig .037 By discussing these results in more detail we will be able to answer whether there is a relationship between excessive drinking and income. Our main source for determining whether there is a relationship between the two is our R value. In this case, our R value is .009. If an R value is closer to -1, the two variables have a negative relationship. If the R value is closer to +1, the two variables have a positive relationship. If it is closer to 0 there is no relationship. In this case, our R value is almost zero but slightly positive. Because our p-value is smaller than 0.05, we must reject the null hypothesis. However, there is no evidence that there is a negative relationship between the two variables as one of our alternative hypotheses states, because the regression slope equation is positive and our R value is slightly positive. Therefore, we must turn to our other alternative hypothesis, that there is a positive relationship between the two variables, as our appropriate hypothesis. However, as our R^2 and adjusted R^2 indicates, this data is not an adequate portrayal of this relationship because both values are 0. If there was a strong association between the two variables, R^2 would be much closer to 1. Because of the values in our regression analysis, we ultimately have to reject our null hypothesis and the hypothesis that there is a negative relationship between excessive drinking and income. However, we accept the hypothesis that there is a positive relationship between the two variables tentatively because our dataset is not an appropriate reflection of the relationship between the two variables. How many drinks r have on a day when drinking Cumulative Frequency Valid Not applicable Percent Valid Percent Percent 59351 99.6 99.6 99.6 1 119 .2 .2 99.8 2 66 .1 .1 99.9 3 27 .0 .0 99.9 4 12 .0 .0 100.0 5 8 .0 .0 100.0 6 5 .0 .0 100.0 7 4 .0 .0 100.0 8 1 .0 .0 100.0 12 1 .0 .0 100.0 Dont know 2 .0 .0 100.0 No answer 3 .0 .0 100.0 59599 100.0 100.0 Total Through the use of the GSS dataset and SPSS we were able to test our null and alternative hypotheses, and determine whether there is a relationship between excessive drinking and income. According to our literature reviews, there have been studies done that show how excessive drinking and income could have a positive relationship and also how they could have a negative relationship. Our research question was \"what is relationship between regularly excessive drinking and annual earnings?\" Through proper data analysis from the GSS, we ultimately rejected the null hypothesis and found that there was a slightly positive relationship between rincome and drinkday. Originally, our plan was to find the mean number of drinks someone had in a day and double it to define \"excessive drinking.\" However, because there were so many respondents who were \"not applicable\" for the drinkday variable, the mean ended up being 0. Obviously this dataset is not an appropriate reflection of the relationship between the two variables because of how many respondents were invalid for the drinkday variable. However, according to our slope equation, for every drink a respondent has their income goes up .149 units on average. If this dataset was an accurate reflection of the relationship between rincome and drinkday this equation would mean that there is a positive relationship between the two. Sociology 120 Spring 2016 Professor Charles FINAL PAPER GUIDELINES I This is a preliminary, very rough guide to your final paper. It is important to begin thinking about your research ideas early in the semester, so that you have adequate time to do a literature review and think about your methods of analysis. This is only a rough guide for what your final paper should look like, but your paper should include the bold-faced sections. It is important to study something you are interested in. To make this as interesting as possible, you will utilize the full General Social Survey (1972-2014), rather than the truncated data sets you use for your assignments. The entire GSS is available on-line through Van Pelt. Someone from the library will come to class and talk to you about accessing the data and what tools are available. More than likely, this person will make a return visit to recitation later in the semester when you're likely to have more concrete questions. And, you should take note of how to contact this person so that you can contact them with questions as you write your paper (keeping in mind that there are 80 of you, and that many others will have the same plan!). Your teaching assistant will also be able to assist you with this. To get started, an outline similar to the one below will be helpful. It can be very informal and you can change your ideas later if necessary. I realize that you may not be able to explain what methods of analysis you will use this early in the semester so begin by jotting down what types of analyses might answer your research question. (For example, if you are interested in studying gender differences in earnings, a good place to start might be to compare the average hourly pay of women and men). As you become more comfortable and knowledgeable, you can update your outline and add details. Finally, keep in mind that the focus of this course is on items III, IV, V, and, to a lesser extent VI. These are the sections that I want you to spend the most time on. Your literature review, therefore, should not be exhaustive and in fact can be limited to 3-5 articles/books written in the last 10 years. Suggested Outline for Final Paper: I. INTRODUCTION Introduce topic State research question II. THEORETICAL BACKGROUND Discussion/Summary of previous research on your topic (literature review) 1 III. HYPOTHESES Statement of research and null hypotheses IV. DATA/MEASUERS Description of data source Description of methods V. RESULTS/DISCUSSION Discuss each procedure Present and discuss findings Decision about the null hypothesis Conclusions about associations Link back to theoretical background VI. CONCLUSION Restate hypotheses Summarize major findings Explain if previous research supports your findings Implications, thoughts on future research, limitations 2 Statistics Gss year for this respondent N Valid Missing 59599 0 Mean 1993.02 Median 1994.00 Mode 2006 Std. Deviation Variance 12.296 151.200 Statistics Respondents income N Valid Missing 59599 0 Mean 8.49 Median 8.00 Mode 0 Std. Deviation Variance 16.029 256.938 Respondents income Cumulative Frequency Valid Not applicable Percent Valid Percent Percent 21149 35.5 35.5 35.5 Lt $1000 1248 2.1 2.1 37.6 $1000 to 2999 1782 3.0 3.0 40.6 $3000 to 3999 1179 2.0 2.0 42.5 $4000 to 4999 1004 1.7 1.7 44.2 $5000 to 5999 1039 1.7 1.7 46.0 $6000 to 6999 950 1.6 1.6 47.6 $7000 to 7999 939 1.6 1.6 49.1 $8000 to 9999 1678 2.8 2.8 52.0 $10000 - 14999 4686 7.9 7.9 59.8 $15000 - 19999 3665 6.1 6.1 66.0 $20000 - 24999 3593 6.0 6.0 72.0 $25000 or more 13129 22.0 22.0 94.0 1919 3.2 3.2 97.2 Don't know 517 .9 .9 98.1 No answer 1122 1.9 1.9 100.0 59599 100.0 100.0 Refused Total Statistics How many drinks r have on a day when drinking N Valid 59599 Missing 0 Mean .02 Median .00 Mode 0 Std. Deviation .918 Variance .842 .02 Mean Median Mode Std. Deviation .00 0 .918 How many drinks r have on a day when drinking Cumulative Frequency Valid Not applicable Percent Valid Percent Percent 59351 99.6 99.6 99.6 1 119 .2 .2 99.8 2 66 .1 .1 99.9 3 27 .0 .0 99.9 4 12 .0 .0 100.0 5 8 .0 .0 100.0 6 5 .0 .0 100.0 7 4 .0 .0 100.0 8 1 .0 .0 100.0 12 1 .0 .0 100.0 Dont know 2 .0 .0 100.0 No answer 3 .0 .0 100.0 59599 100.0 100.0 Total Case Processing Summary Cases Valid N Missing Percent N Total Percent N Percent Respondents income * How many drinks r have on a day 59599 100.0% 0 0.0% 59599 100.0% when drinking Respondents income * How ma Not applicable Respondents income Not applicable Count % within How many drinks r have on a day when drinking Lt $1000 Count % within How many drinks r have on a day when drinking $1000 to 2999 Count % within How many drinks r have on a day when drinking $3000 to 3999 Count % within How many drinks r have on a day when drinking $4000 to 4999 Count % within How many drinks r have on a day when drinking $5000 to 5999 Count % within How many drinks r have on a day when drinking $6000 to 6999 Count 1 2 21047 53 35.5% 44.5% 1245 1 2.1% 0.8% 1777 3 3.0% 2.5% 1177 2 2.0% 1.7% 1003 0 1.7% 0.0% 1036 2 1.7% 1.7% 946 0 3 % within How many drinks r have on a day when drinking $7000 to 7999 Count % within How many drinks r have on a day when drinking $8000 to 9999 Count % within How many drinks r have on a day when drinking $10000 - 14999 Count % within How many drinks r have on a day when drinking $15000 - 19999 Count % within How many drinks r have on a day when drinking $20000 - 24999 Count % within How many drinks r have on a day when drinking $25000 or more Count % within How many drinks r have on a day when drinking Refused Count % within How many drinks r have on a day when drinking Don't know Count % within How many drinks r have on a day when drinking No answer Count % within How many drinks r have on a day when drinking Total Count % within How many drinks r have on a day when drinking 1.6% 0.0% 937 0 1.6% 0.0% 1675 0 2.8% 0.0% 4674 9 7.9% 7.6% 3649 6 6.1% 5.0% 3578 5 6.0% 4.2% 13060 32 22.0% 26.9% 1913 4 3.2% 3.4% 513 2 0.9% 1.7% 1121 0 1.9% 0.0% 59351 119 100.0% 100.0% Descriptives How many drinks r have on a day when drinking N Mean Std. Deviation Std. Error 95% Confidence Interval for Mean Minimum 3 10 Lower Bound Not applicable Upper Bound 21149 .02 .971 .007 .01 .03 0 Lt $1000 1248 .00 .085 .002 .00 .01 0 $1000 to 2999 1782 .00 .095 .002 .00 .01 0 $3000 to 3999 1179 .00 .041 .001 .00 .00 0 $4000 to 4999 1004 .00 .095 .003 .00 .01 0 $5000 to 5999 1039 .00 .076 .002 .00 .01 0 $6000 to 6999 950 .02 .302 .010 .00 .04 0 $7000 to 7999 939 .00 .092 .003 .00 .01 0 $8000 to 9999 1678 .01 .197 .005 .00 .02 0 $10000 - 14999 4686 .01 .145 .002 .00 .01 0 $15000 - 19999 3665 .01 .246 .004 .00 .02 0 $20000 - 24999 3593 .04 1.644 .027 -.02 .09 0 $25000 or more 13129 .01 .169 .001 .01 .01 0 1919 .06 2.262 .052 -.05 .16 0 Don't know 517 .20 4.355 .192 -.18 .58 0 No answer 1122 .00 .119 .004 .00 .01 0 59599 .02 .918 .004 .01 .02 0 Refused Total ANOVA How many drinks r have on a day when drinking Sum of Squares Between Groups df Mean Square 24.488 15 1.633 Within Groups 50172.034 59583 .842 Total 50196.522 59598 Between Groups Within Groups Total Sum of Squares 24.488 Df 15 Mean Square 1.633 50172.034 59583 .842 50196.522 59598 F Sig. 1.939 .016 F Sig. 1.939 .016 Correlations How many drinks r have on Respondents income Pearson Correlation Respondents a day when income drinking 1 Sig. (2-tailed) N .037 59599 59599 * 1 How many drinks r have on Pearson Correlation .009 a day when drinking Sig. (2-tailed) .037 N *. Correlation is significant at the 0.05 level (2-tailed). .009* 59599 59599 Variables Entered/Removeda Variables Variables Entered Removed Model 1 Method How many drinks r have on . Enter a day when drinkingb a. Dependent Variable: Respondents income b. All requested variables entered. Model Summary Std. Error of the Model R 1 R Square a .009 Adjusted R Square .000 Estimate .000 16.029 a. Predictors: (Constant), How many drinks r have on a day when drinking Model R R square Adjusted R square 1 .009 .000 .000 Std. Error of the Estimate 16.029 ANOVAa Model 1 Sum of Squares Regression df Mean Square 1111.930 1 1111.930 Residual 15311900.419 59597 256.924 Total 15313012.350 59598 F 4.328 Sig. .037b a. Dependent Variable: Respondents income b. Predictors: (Constant), How many drinks r have on a day when drinking Model Regression Residual Sum of Df squares 1111.930 1 15311900.419 59597 Mean square F Sig 111.930 256.924 4.328 .037 Total 15313012.35 0 59598 Coefficientsa Standardized Unstandardized Coefficients Model 1 B (Constant) How many drinks r have on a day when drinking Std. Error 8.485 .066 .149 .072 Coefficients Beta t .009 Sig. 129.210 .000 2.080 .037 a. Dependent Variable: Respondents income Descriptive statistics: In this case we have two variables Respondents income (though it is in ratio scale but here it is grouped in categories) and How many drinks r have on a day when drinking (Discrete variable). The frequency, percentage and cumulative percentage for each category (for both variables) are given in the beginning of the analysis. For example from the results we can see that 1248 (almost 2.1%) of the respondents have income less than $1000. 1782 (almost 3%) have in between $1000 to 2999. And so on. Similarly the percentage distribution for the number of drinks is also given. We also have the descriptive statistics which is giving us the Mean, Median, mode, standard deviation etc. values for each category. But as they are not so important in this case so I am not analyzing them in details. The cross-tabulation between these two considered variables is also given in the results which can be used to see whether there is an association between the variables, but as we have better measures to measure that so I am considering those measures. ANOVA: An ANOVA is run to see whether there is a significant difference between the mean number of drinks r have on a day when drinking in different categories. The P-value of 0.016 (smaller than 0.05) is implying to reject the null hypothesis and conclude that there is a significant difference in group means. Regression Analysis: Now lets look at the most important part of the analysis. The regression analysis answers the question we are trying to answer. It gives the relationship between the two considered variables. Before the actual analysis lets look at the scatter diagram and the correlation. Though the correlation coefficient value of 0.009 is coming out to be significant at 0.05 significance level but that is not practically significant. Moreover the scatter diagram indicates that there are quite few outliers in the dataset which might be affecting our result. Now the regression model is significant at 0.05 significance level as p-value is 0.037. But the R2 and Adjusted R2 value indicates that the model is not accurate at all and thus using this model for the prediction purpose might not be realistic. Moreover we saw presence of outliers which affects the regression model. The slope and intercept both are significant. The slope value of 0.149 implies that per 1 extra drink is expected to increase the income by 0.149 unit on average
Step by Step Solution
There are 3 Steps involved in it
Step: 1
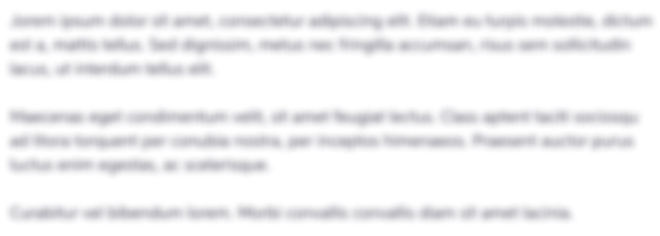
Get Instant Access to Expert-Tailored Solutions
See step-by-step solutions with expert insights and AI powered tools for academic success
Step: 2

Step: 3

Ace Your Homework with AI
Get the answers you need in no time with our AI-driven, step-by-step assistance
Get Started