Answered step by step
Verified Expert Solution
Question
1 Approved Answer
Explain how GPU and CPUs are jointly used for self-driving cars? As CPU makers can technically replace the position of Nvidia in the future, the
Explain how GPU and CPUs are jointly used for self-driving cars? As CPU makers can technically replace the position of Nvidia in the future, the expansion of complementary businesses is integral to the continuous success of NVIDIA. Identify a complementary business and explain how it enhances the competitive advantage of NVIDIA.
NVIDIA looked forward to a future of rapidly expanding potential for its technologies in artificial intelligence (AI). Yet, the company's success and the rising stakes in achieving dominance in Al were attracting many new competitors. The big question was how should the company position itself to continue to survive and thrive in the unfolding Al revolution? Jen-Hsun Huang founded NVIDIA in 1993 to produce leading-edge graphics processing units for powering computer games. The company grew rapidly to become the dominant player in the design and marketing of graphics processing units (GPUs) and cards for gaming and computer-aided design, as well as multimedia editing. More recently the company had diversified into building system-on-a-chip (SOC) solutions for researchers and analysts focused on Al, deep learning and big data, as well as cloud-based visual computing. In addition, NVIDIA's processors increasingly provided supercomputing capabilities for autonomous robots, drones and cars. Though it was at the forefront of GPUs and SoCs, NVIDIA's position in Al was being threatened by the likes of Google, Intel, AMD and a host of Silicon Valley start-ups keen on challenging the company's dominance in the field. The company's early successes in chips for self- driving cars was also being threatened, not only by Intel but also by customers such as Tesla that had announced it would be developing its own technologies The key question facing NVIDIA was: Would the lessons learned from its origins to its current position prove to be a strong roadmap for building NVIDIA into an Al platform company? What strategic choices would the senior team have to make? Fundamentally, what would NVIDIA need to do to tactically win against these competitive threats? NVIDIA: Background in word for my by 30-year Malachows NVIDIA, pronounced "Invidia" from the Latin word for "envy," was a Californian graphics card company. It was founded in 1993 with just $40,000 in capital by 30-year-old former AMD chip designer Jen-Hsun Huang (who would become CEO), Sun engineer Chris Malachowsky, and Sun graphics chip designer Curtis Priem. The three saw an opportunity in the burgeoning 3D computer-game market. The first two years were tough. NVIDIA struggled with an over-ambitious first product, its own programming standard and a lack of market demand for its premium products. At the end of 1995, all but 35 cmployees were laid off. Ditching its own standard, the company switched to Microsoft's new DirectX software standard. It invested 33% ($1 million) of its remaining cash on graphics card emulation - a technology that allowed NVIDIA's engineers to test their designs in a virtual environment before incurring any production costs. This decision, which enabled rapid design and development iterations, meant NVIDIA was able to release a new graphics chip every six to nine months - an unprecedented pace. In 1998, the company announced a strategic partnership with Taiwan semiconductor manufacturer TSMC to manufacture all of NVIDIA'S GPUs using a fabless approach.' (Refer to Exhibit 1 for a timeline of events and key competitor moves.) NVIDIA went public in 1999, raising $42 million, with shares closing 64% up on its first day of trading. Later that year NVIDIA released the GeForce 256, billed as the world's first GPU, which offloaded all graphics processing from the CPU (central processing unit). It outperformed its 3dfx rival by 50%. The built-in transform and lighting (T&L) module also allowed NVIDIA to enter the computer-aided design (CAD) market for the first time, with its new Quadro range. Becoming a Leader In 1999, Sony successfully launched its PlayStation 2 (PS2) in Japan, which the press predicted would destroy PC gaming. Threatened, Microsoft decided it had to rapidly launch its own games console, preferably before the US debut of the PS2 in 2000. Its trump card was DirectX, a Windows technology that allowed 3D games to run without developers having to port their games to every graphics card. Microsoft knew that Sony was dependent on third-party publishers for content-publishers that could be lured away to another platform, with the right incentives. Microsoft initially approached NVIDIA for the Xbox GPU, but NVIDIA's quote was too high. Huang said, "I wanted to do the deal, but not if it was going to kill the company." card. Misat could be lured for the Xbox GPO Microsoft, instead, decided to acquire unknown graphics chip design house Gigapixel and bring it in-house in the hope of manufacturing its own chips and saving on cost. Yet Microsoft was new to the nuances of semiconductor cconomics, where the unit price was dependent on size, yield, design complexity and manufacturing facilities. Ultimately, Gigapixel's increasing lead times pushed the Xbox launch deep into 2001. Facing a revolt from game developers unhappy with an unknown new chip, Microsoft signed a last-minute deal with NVIDIA on the eve of the Xbox announcement. To meet the Xbox launch deadline and ensure support for DirectX, NVIDIA's proposal was to fuse two GeForce 256 cores together to create a custom GPU for the Xbox. Microsoft paid NVIDIA S200 million up front and $48 per unit for the first 5 million GPUs. Later, in 2002, Microsoft would take NVIDIA to court, demanding a discount on further supply." A last-minute meeting between Intel's Paul Otellini and Bill Gates on the night of the NVIDIA deal, meant Microsoft also made a switch from AMD to Intel CPUs for the Xbox. Both Gigapixel and AMD employees were left stunned by the news. Gigapixel was snapped up by NVIDIA's once dominant 1990s rival, but now struggling, 3dfx." Soon after that, NVIDIA acquired 3dfx and all of Gigapixel's assets. This left NVIDIA with only one real competitor- ATI Technologies. Rivalry with ATI ATI was founded in Toronto in 1985 and went public in 1993. Its answer to NVIDIA's GeForce was the ATI Radeon line of graphics cards. The two companies went on to dominate the graphics card market in a closely fought battle between 2000 and 2006. It was ATI that won the contract to design the Xbox 360's Xenon GPU. Microsoft demanded full control of production and manufacturing to avoid a repeat of the perceived high price of the original Xbox NVIDIA GPUs. When the console launched in 2005, however, it was plagued by hardware defects and an abnormally high failure rate, which was remembered years later is the infamous "Red Rings of Death." Meanwhile, in 2004, NVIDIA had quietly signed a deal to provide Sony with a GeForce-based GPU design named RSX for its new PlayStation 3 console. It was a coup for NVIDIA, and a first for Sony, which usually designed and manufactured its own hardware - a sign of the rapidly increasing complexity of producing a competitive GPU. The RSX GPU silicon Wis produced at Sony Group's own Nagasaki Fab2 foundry. In July 2006, Advanced Micro Devices (AMD) announced it was buying ATI Technologies for $5.4 billion in cash and stock, sending shockwaves through the industry. AMD believed that shrinking CPU sizes would leave room for fusing a CPU with a GPU and allow it to outmaneuver Intel, which did not have any comparable capabilities. AMD's first target was NVIDIA, but NVIDIA'S CEO Huang, a former AMD employee, insisted he become CEO of the joint company, so AMD turned to ATI. The merger between AMD and ATI was complex, not least because AMD was involved in an antitrust suit against Intel and weighed down by the cost of running its own foundries." CUDA Cores: The Game Changer While AMD/ATI were distracted by their integration, NVIDIA launched the GeForce G80, featuring its new "Tesla" microarchitecture. This was considered a gamechanger in the industry. The G80 offered a 100% performance improvement over the previous generation, redefining what GPUs were capable of. NVIDIA realized that if it switched its GPU architecture from arrays of specialized cores, dedicated to specific tasks, to an array of much "simpler" cores, it could include many more cores and run them at double the clock-speed. Crucially, they could be programmed to perform whatever calculations were required at any particular moment. The Glo's revolutionary "compute" approach carried a big risk: NVIDIA was adding extra features that were unrelated to gaming, at substantial extra cost, to a product that was primarily aimed at the gaming market. We definitely felt that compute was a risk. Both in terms of area - we were adding area that all of our gaming products would have to carry even though it wouldn't be used for gaming - and in terms of complexity of taking on compute as a parallel operating model for the chip. Johan Alben, Senior VP, GPU Engineering To ensure all developers could take full advantage of the revolutionary G80 architecture to accelerate their programs, now that any task could be parallelized, NVIDIA launched a new programming model named the Compute Unified Device Architecture (CUDA). At the heart of CUDA's design was NVIDIA's belief that the world needed a new parallel programming model. CUDA provided an abstraction that allowed developers to ignore the underlying hardware completely, which made developing on it quicker and cheaper. It turned out that programs written using CUDA, and accelerated using a G80, were not just a little faster they were 100-400 times faster (refer toe Table 1). This heralded the era of general-purpose GPU (GPGPU) programming No Greeks Greeks Intel Xeon 18.15 26.95 ClearSpeed Advance 2 CSX600 2.95 6x 6.45 NVIDIA 8800 GTX 0.045 400% 0.18 149 Table 1: Example of CUDA performance gains (Source: NVIDIA Corporation, 2006-2008) CUDA had applications across multiple fields, including medicine, chemistry, biology, physics and finance, NVIDIA was able to ensure widespread adoption of CUDA through its heavy investment in a CUDA ccosystem, and its existing network of developer programs and university partnerships. It also spurred the development of competing GPGPU standards like the ATI- supported open-standard, OpenCL, which worked with multiple graphics card brands, unlike CUDA. The GeForce 8 series was not without its problems. Laptop varieties of the G80 started failing at extremely high rates, due potentially to a manufacturing defect. NVIDIA tried to cover it up, but eventually admitted the problem and took a $200 million write-down. It then became the subject of a class-action lawsuit by investors, claiming NVIDIA had lost $3 billion in market capitalization due to the way it had handled the problem. NVIDIA's G80 "Tesla" microarchitecture started the GPGPU revolution. The next generation "Fermi" microarchitecture cemented NVIDIA's leading position It is completely clear that GPUs are now general-purpose parallel computing processors with amazing graphics, and not just graphics chips anymore. ** Jen-Hsun Huang, CEO, NVIDIA Unveiled in September 2009, Fermi had 512 CUDA cores compared to the G80's 128 and eight times the peak arithmetic performance. Enter Artificial Intelligence The GeForce 580 GTX, the top Fermi-based consumer graphics card, launched in 2010. It was on a pair of these cards that University of Toronto PhD student Alex Krizhevsky started training carly versions of what would become AlexNet - a deep-learning algorithm dramatically better than any algorithm created earlier. Using the Fermi CGU, Krizhevsky joined up with fellow student Ilya Sutskever and Professor Geoffrey Hinton to enter an Al image recognition competition - ImageNet - in 2012. Their submission blew the competition away. With 85% accuracy, it was the first algorithm to breach the critical sub-25% error threshold and was 41% better than the nearest competition. (Refer to Exhibir 2 for background on machine learning GPUs and AlexNet) It was a defining moment in artificial intelligence, and it put the spotlight and deep-learning Al leadership firmly on NVIDIA. AlexNet was later bought by Google, which saw an opportunity to take Al to the next level of performance. Strategic Steps ... and Missteps In 2006, NVIDIA had acquired Indian-US company PortalPlayer, a designer of SoC hardware for personal media players, for $357 million.PortalPlayer had recently been dropped by Apple's iPod line, which had accounted for most of its revenue. Leveraging the PortalPlayer acquisition, NVIDIA launched its brand new "Tegra" SoC product line for mobile devices. Despite much lauded designs and great performance, it struggled in a competitive market and had limited success. In 2011, NVIDIA launched the Tegra 3 quad-core SoC. It was only when Audi and Tesla Motors both announced plans to use the Tegra 3 processor to power their vehicles' infotainment systems across their entire ranges worldwide that it became a strategic product for NVIDIA. Tegra gave NVIDIA entry into the automotive industry. Four years later the company would further develop this market with the launch of its Tegra-based Drive PX automotive platform. Not every strategic move resulted in success. Attempting to compete with mobile giant Qualcomm, NVIDIA acquired loera for $376 million in 2011." It planned to incorporate an LTE modem into its next-gen Tegra 4 SoC. Four years later, with only a negligible presence in the market, NVIDIA announced plans to sell icera and exit the mobile processor market completely. Our vision was right, but the mobile business is not for us. NVIDIA is not in the commodity chip business that the smartphone market has become ... our mobile experience was far from a bust. The future of computing is constrained by energy. The technology, methodologies, and design culture of energy efficiency have allowed us to build the world's most efficient GPU architecture, Jen-Hsun Huang, CEO, NVIDIA NVIDIA's Tegra SoC put it squarely in the running for the next generation of gaming consoles. Microsoft and Sony both wanted to increase the market share of their new consoles, the Xbox One and PlayStation 4. The key to achieving this would be to increase the number of applications that could run on their consoles and lower the development costs of third-party publishers. There were two options for Microsoft and Sony: ARM or x86, the only two CPU architectures that had the performance and developer support to achieve their goals. They also both wanted an SoC solution, as it used less power and space and generated less heat. ARM could not compete on pure performance and lacked a viable 64-bit option, important for memory-hungry games. That eliminated the ARM-based NVIDIA Tegra. Intel's potential solutions lacked the required graphics power to run games. In 2013, at "E3," the world's biggest computer game show, Microsoft and Sony announced their new consoles would both be using an SoC from AMD that would be custom-designed for cach console. NVIDIA claimed it had chosen not to compete as the financial rewards were insufficient. The Rise of Deep Learning Despite exiting the mobile phone business and losing out on both new gaming consoles, NVIDIA had an ace up its sleeve-its new Pascal microarchitecture. First announced by Huang at its GPU Technology conference in 2015, Pascal took three years to develop and was heavy on features designed to increase deep learning performance. The first Pascal-based product announcement in 2016 was not a gaming card, but the Tesla P100, designed for high- performance computing. It cost just under $10,000, had 3,584 CUDA cores, and promised a massive increase in neural network training performance compared to the previous generation. Included in the announcement was CUDA version 8 and new software called nvGRAPH that was aimed at accelerating big-data analytics. Huang announced to investors in 2017: Al fueled the rapid growth of our datacenter business, which tripled year over year in the fourth quarter, bringing total annual revenue to $830 million. We have a multi-year lead in Al computing (and we've built an end-to-end platform that starts with a GPU optimized for AL Our single architecture spans computers of every shape and size-PCs, servers, loT devices - and is available from every cloud service provider - Alibaba, Amazon, Google, IBM, Microsoft GPU deep learning is the most advanced, most accessible platform for Al. Jen-Hsun Huang, CEO, NVIDIA At the same time, in a world-first for both NVIDIA and the industry, the company launched the NVIDIA DGX-1, billed as an "Al super-computer in a box.* The off-the-shelf solution contained cight Tesla P100 cards in a standard medium-sized rack server enclosure costing $129,000. (Refer to Figure 1 for performance comparisons based on the workload of Reser 50, 90 epochs to solution, CPU server: Dual Xeon E5-26994, 2.6 GHz.) Google's Raja Monga also attended the press conference, talking about the DGX-l's use of TensorFlow, Google's open-source machine learning framework. 711 hours Figure 1: DGX-1 performance comparisons (Source: reproduced from NVIDIA calculations) The first DGX-1 produced was donated to Elon Musk's non-profit Al research company, OpenAL NVIDIA also gave nine others to prominent universities known for their deep- learning research departments, including: New York University, Stanford and the University of Toronto. Some companies, such as London-based BenevolentAl, considered the DGX-l's premium price to be worth the money when compared to a year's worth of the equivalent Amazon Web Services computer resource. Others, such as Baidu Al Labs' Greg Diamos, thought it likely that large customers would want to build their own custom servers. Both Microsoft and Facebook went on to create the HGX-1 and Big Basin respectively, with each server featuring eight Tesla P100 cards like the DGX-1. Meanwhile IBM created its IS822LC server, which used only four Tesla P100 cards mixed with Power CPUs, and an open-reference design that anyone could copy. Google on the other hand, announced its own data center hardware to accelerate machine learning, called the Tensor Processing Unit (TPU), although it was not commercially available. NVIDIA in 2018 In 1998, NVIDIA had 117 cmployees, and by January 2018, that number had grown to more than 8,000. The company had expanded beyond its original market of 3D graphics cards to four markets: gaming, professional visualization, datacenter and automotive. Despite the four different markets, the company employed a single-microarchitecture across all its products (Pascal and Volta). The strategy was to focus on markets where its architecture gave it a competitive edge, which meant a strong return on research and development (R&D). NVIDIA spent its entire R&D budget on researching new micro-architectures across a three-year horizon, then producing a new range of GPU products based on that architecture. NVIDIA'S GPU design realized strong year-over-year growth of 39% in FY 2017, representing 84.3% of total revenues. (Refer to Exhibir 3 for key financials and share price.) Gaming was the company's main revenue carner, but it was also riding on the wave of cryptocurrency miners. JPR research estimated that 3 million GPU's were bought by miners in 2017. NVIDIA's GeForce NOW application provided cloud gaming services. The global cloud computing market was expected to reach $411 billion by 2020. In early 2018, NVIDIA tried to enforce a GeForce Partner Program, obliging PC and add-in board vendors to use only NVIDIA'S GPUs in their high-end gaming brands, claiming it was an unfair use of their marketing support for vendors to use other GPUs. The company was targeted for being anti-competitive shortly afterwards. Professional visualization (virtualization, mobility, virtual reality and AI) was another market that NVIDIA was successfully advancing, working with customers by providing a visualization platform powered by advanced GPUs. Customers were using the platform to power a variety of medical advances. Arterys, for example, was using NVIDIA GPU-powered deep leaming to speed up analysis of medical images, which were deployed in GE Healthcare MRI machines to help diagnose heart disease. In 2017, Japan's Komatsu selected NVIDIA to provide GPUs to its construction sites to transform them into jobsites of the future that would be safer and more efficient For automotive, NVIDIA had launched its Drive PX1 Successor, the PX2, some months prior to the launch of the Tesla P100 filled DGX-1. It was aimed at autonomous driving and featured two Tegra-based SoCs and a Pascal-based GPU. NVIDIA's early automotive partner, Tesla Motors, having split from long-time partner Mobileye, announced that all future vehicles would include a Drive PX2 to enable "enhanced autopilot." Taking a platform approach, NVIDIA also partnered with Volvo, Audi, Daimler, BMW and Ford to test the PX2. It supplied Toyota, Volkswagen, Volvo, BMW, Daimler, Honda, Renault-Nissan, Bosch, Baidu and others. Despite NVIDIA's success, Tesla announced in a quarterly earnings call in August 2018 that it would build its own custom chips for its self-driving hardware. One analyst suggested that Tesla thought that chip vendors were slowing them down" or "locking them into a particular architecture, and it needed to own the technology. NVIDIA responded that it had already identified the issue and had successfully improved it, achieving 30 trillion operations per second (TOPS) with just 30 Watts. Tesla's custom version of the PX2 produced between 8- 10 TOPS. Further, in 2019, NVIDIA planned to launch the Drive Pegasus, which would achieve 320 TOPS at 500 Watts. Also threatening NVIDIA's dominance was rival Intel. Intel bought Israeli automotive company Mobileye in 2018. Mobileye's EyeQ3 chip powered Tesla's first generation of autopilot hardware, enabling Intel to enter the automotive market. Mobileye's latest chip. EyeQ5 was its third generation, and it had signed a deal for it to be deployed in eight million vehicles in 2019. Intel's approach differed from NVIDIA, preferring to co-develop processors optimized for integrated software applications, allowing the needs of the software algorithm to determine what goes into the hardware for a more efficient implementation. For data centers, NVIDIA worked with Alibaba Cloud, Baidu and Tencent to upgrade from Pascal to Volta-based platforms, giving them increased processing speed and scalability for Al inferencing and training. Amazon Web Services and Microsoft used NVIDIA GPUs for their deep learning applications. While Google continued to use NVIDIA GPUs in its cloud, despite announcing it would create its own hardware Cloud TPU. In March 2019, NVIDIA won a bidding war against Intel and Microsoft for Isracli chipmaker, Mellanox, for $6.9 billion. Mellanox produced chips for very high-speed networks, critical for expanding cloud services. Together, NVIDIA and Mellanox would power over 50% of the world's 500 biggest computers and cover every computer producer and major cloud service provider. In the press release, Huang explained: The emergence of Al and data science, as well as billions of simultaneous computer users, is fueling skyrocketing demand on the world's datacenters. Addressing this demand will require holistic architectures that connect vast numbers of fast computing nodes over intelligent networking fabrics to form a giant datacenter-scale computer engine. We're excited to unite NVIDIA's accelerated computing platform with Mellanox's world-renowned accelerated networking platform under one roof to create next-generation datacenter-scale computing solutions and I am particularly thrilled to work closely with the visionary leaders of Mellanox and their amazing people to invent the computers of tomorrow." Jen-Hsun Huang, CEO, NVIDIA Leading the Future of AI? In 2018, NVIDIA was dominant and growing rapidly in the deep learning Al space. Yet, there were competitive threats on the horizon that could challenge NVIDIA's position. These came from Google's Tensor flow processing unit (TPU) and companies including Intel, AMD and Silicon Valley start-ups, such as Wave computing, that claimed to be working on chips that were 10 times faster than NVIDIA's with less power consumption. China's Cambricon, backed by the Chinese government, while not targeting deep-learning training, could change strategy and was a potential threat One commentator suggested that he viewed NVIDIA not as a GPU company but as a "platform company with an insatiable appetite for growth and an ability to pivot quickly when threatened. The question facing the company was: Did NVIDIA have a clear strategy and roadmap to grow as an Al platform company? Was that the right strategy or should it continue to explore and exploit other core markets? What did NVIDIA need to do tactically to retain its leadership? NVIDIA looked forward to a future of rapidly expanding potential for its technologies in artificial intelligence (AI). Yet, the company's success and the rising stakes in achieving dominance in Al were attracting many new competitors. The big question was how should the company position itself to continue to survive and thrive in the unfolding Al revolution? Jen-Hsun Huang founded NVIDIA in 1993 to produce leading-edge graphics processing units for powering computer games. The company grew rapidly to become the dominant player in the design and marketing of graphics processing units (GPUs) and cards for gaming and computer-aided design, as well as multimedia editing. More recently the company had diversified into building system-on-a-chip (SOC) solutions for researchers and analysts focused on Al, deep learning and big data, as well as cloud-based visual computing. In addition, NVIDIA's processors increasingly provided supercomputing capabilities for autonomous robots, drones and cars. Though it was at the forefront of GPUs and SoCs, NVIDIA's position in Al was being threatened by the likes of Google, Intel, AMD and a host of Silicon Valley start-ups keen on challenging the company's dominance in the field. The company's early successes in chips for self- driving cars was also being threatened, not only by Intel but also by customers such as Tesla that had announced it would be developing its own technologies The key question facing NVIDIA was: Would the lessons learned from its origins to its current position prove to be a strong roadmap for building NVIDIA into an Al platform company? What strategic choices would the senior team have to make? Fundamentally, what would NVIDIA need to do to tactically win against these competitive threats? NVIDIA: Background in word for my by 30-year Malachows NVIDIA, pronounced "Invidia" from the Latin word for "envy," was a Californian graphics card company. It was founded in 1993 with just $40,000 in capital by 30-year-old former AMD chip designer Jen-Hsun Huang (who would become CEO), Sun engineer Chris Malachowsky, and Sun graphics chip designer Curtis Priem. The three saw an opportunity in the burgeoning 3D computer-game market. The first two years were tough. NVIDIA struggled with an over-ambitious first product, its own programming standard and a lack of market demand for its premium products. At the end of 1995, all but 35 cmployees were laid off. Ditching its own standard, the company switched to Microsoft's new DirectX software standard. It invested 33% ($1 million) of its remaining cash on graphics card emulation - a technology that allowed NVIDIA's engineers to test their designs in a virtual environment before incurring any production costs. This decision, which enabled rapid design and development iterations, meant NVIDIA was able to release a new graphics chip every six to nine months - an unprecedented pace. In 1998, the company announced a strategic partnership with Taiwan semiconductor manufacturer TSMC to manufacture all of NVIDIA'S GPUs using a fabless approach.' (Refer to Exhibit 1 for a timeline of events and key competitor moves.) NVIDIA went public in 1999, raising $42 million, with shares closing 64% up on its first day of trading. Later that year NVIDIA released the GeForce 256, billed as the world's first GPU, which offloaded all graphics processing from the CPU (central processing unit). It outperformed its 3dfx rival by 50%. The built-in transform and lighting (T&L) module also allowed NVIDIA to enter the computer-aided design (CAD) market for the first time, with its new Quadro range. Becoming a Leader In 1999, Sony successfully launched its PlayStation 2 (PS2) in Japan, which the press predicted would destroy PC gaming. Threatened, Microsoft decided it had to rapidly launch its own games console, preferably before the US debut of the PS2 in 2000. Its trump card was DirectX, a Windows technology that allowed 3D games to run without developers having to port their games to every graphics card. Microsoft knew that Sony was dependent on third-party publishers for content-publishers that could be lured away to another platform, with the right incentives. Microsoft initially approached NVIDIA for the Xbox GPU, but NVIDIA's quote was too high. Huang said, "I wanted to do the deal, but not if it was going to kill the company." card. Misat could be lured for the Xbox GPO Microsoft, instead, decided to acquire unknown graphics chip design house Gigapixel and bring it in-house in the hope of manufacturing its own chips and saving on cost. Yet Microsoft was new to the nuances of semiconductor cconomics, where the unit price was dependent on size, yield, design complexity and manufacturing facilities. Ultimately, Gigapixel's increasing lead times pushed the Xbox launch deep into 2001. Facing a revolt from game developers unhappy with an unknown new chip, Microsoft signed a last-minute deal with NVIDIA on the eve of the Xbox announcement. To meet the Xbox launch deadline and ensure support for DirectX, NVIDIA's proposal was to fuse two GeForce 256 cores together to create a custom GPU for the Xbox. Microsoft paid NVIDIA S200 million up front and $48 per unit for the first 5 million GPUs. Later, in 2002, Microsoft would take NVIDIA to court, demanding a discount on further supply." A last-minute meeting between Intel's Paul Otellini and Bill Gates on the night of the NVIDIA deal, meant Microsoft also made a switch from AMD to Intel CPUs for the Xbox. Both Gigapixel and AMD employees were left stunned by the news. Gigapixel was snapped up by NVIDIA's once dominant 1990s rival, but now struggling, 3dfx." Soon after that, NVIDIA acquired 3dfx and all of Gigapixel's assets. This left NVIDIA with only one real competitor- ATI Technologies. Rivalry with ATI ATI was founded in Toronto in 1985 and went public in 1993. Its answer to NVIDIA's GeForce was the ATI Radeon line of graphics cards. The two companies went on to dominate the graphics card market in a closely fought battle between 2000 and 2006. It was ATI that won the contract to design the Xbox 360's Xenon GPU. Microsoft demanded full control of production and manufacturing to avoid a repeat of the perceived high price of the original Xbox NVIDIA GPUs. When the console launched in 2005, however, it was plagued by hardware defects and an abnormally high failure rate, which was remembered years later is the infamous "Red Rings of Death." Meanwhile, in 2004, NVIDIA had quietly signed a deal to provide Sony with a GeForce-based GPU design named RSX for its new PlayStation 3 console. It was a coup for NVIDIA, and a first for Sony, which usually designed and manufactured its own hardware - a sign of the rapidly increasing complexity of producing a competitive GPU. The RSX GPU silicon Wis produced at Sony Group's own Nagasaki Fab2 foundry. In July 2006, Advanced Micro Devices (AMD) announced it was buying ATI Technologies for $5.4 billion in cash and stock, sending shockwaves through the industry. AMD believed that shrinking CPU sizes would leave room for fusing a CPU with a GPU and allow it to outmaneuver Intel, which did not have any comparable capabilities. AMD's first target was NVIDIA, but NVIDIA'S CEO Huang, a former AMD employee, insisted he become CEO of the joint company, so AMD turned to ATI. The merger between AMD and ATI was complex, not least because AMD was involved in an antitrust suit against Intel and weighed down by the cost of running its own foundries." CUDA Cores: The Game Changer While AMD/ATI were distracted by their integration, NVIDIA launched the GeForce G80, featuring its new "Tesla" microarchitecture. This was considered a gamechanger in the industry. The G80 offered a 100% performance improvement over the previous generation, redefining what GPUs were capable of. NVIDIA realized that if it switched its GPU architecture from arrays of specialized cores, dedicated to specific tasks, to an array of much "simpler" cores, it could include many more cores and run them at double the clock-speed. Crucially, they could be programmed to perform whatever calculations were required at any particular moment. The Glo's revolutionary "compute" approach carried a big risk: NVIDIA was adding extra features that were unrelated to gaming, at substantial extra cost, to a product that was primarily aimed at the gaming market. We definitely felt that compute was a risk. Both in terms of area - we were adding area that all of our gaming products would have to carry even though it wouldn't be used for gaming - and in terms of complexity of taking on compute as a parallel operating model for the chip. Johan Alben, Senior VP, GPU Engineering To ensure all developers could take full advantage of the revolutionary G80 architecture to accelerate their programs, now that any task could be parallelized, NVIDIA launched a new programming model named the Compute Unified Device Architecture (CUDA). At the heart of CUDA's design was NVIDIA's belief that the world needed a new parallel programming model. CUDA provided an abstraction that allowed developers to ignore the underlying hardware completely, which made developing on it quicker and cheaper. It turned out that programs written using CUDA, and accelerated using a G80, were not just a little faster they were 100-400 times faster (refer toe Table 1). This heralded the era of general-purpose GPU (GPGPU) programming No Greeks Greeks Intel Xeon 18.15 26.95 ClearSpeed Advance 2 CSX600 2.95 6x 6.45 NVIDIA 8800 GTX 0.045 400% 0.18 149 Table 1: Example of CUDA performance gains (Source: NVIDIA Corporation, 2006-2008) CUDA had applications across multiple fields, including medicine, chemistry, biology, physics and finance, NVIDIA was able to ensure widespread adoption of CUDA through its heavy investment in a CUDA ccosystem, and its existing network of developer programs and university partnerships. It also spurred the development of competing GPGPU standards like the ATI- supported open-standard, OpenCL, which worked with multiple graphics card brands, unlike CUDA. The GeForce 8 series was not without its problems. Laptop varieties of the G80 started failing at extremely high rates, due potentially to a manufacturing defect. NVIDIA tried to cover it up, but eventually admitted the problem and took a $200 million write-down. It then became the subject of a class-action lawsuit by investors, claiming NVIDIA had lost $3 billion in market capitalization due to the way it had handled the problem. NVIDIA's G80 "Tesla" microarchitecture started the GPGPU revolution. The next generation "Fermi" microarchitecture cemented NVIDIA's leading position It is completely clear that GPUs are now general-purpose parallel computing processors with amazing graphics, and not just graphics chips anymore. ** Jen-Hsun Huang, CEO, NVIDIA Unveiled in September 2009, Fermi had 512 CUDA cores compared to the G80's 128 and eight times the peak arithmetic performance. Enter Artificial Intelligence The GeForce 580 GTX, the top Fermi-based consumer graphics card, launched in 2010. It was on a pair of these cards that University of Toronto PhD student Alex Krizhevsky started training carly versions of what would become AlexNet - a deep-learning algorithm dramatically better than any algorithm created earlier. Using the Fermi CGU, Krizhevsky joined up with fellow student Ilya Sutskever and Professor Geoffrey Hinton to enter an Al image recognition competition - ImageNet - in 2012. Their submission blew the competition away. With 85% accuracy, it was the first algorithm to breach the critical sub-25% error threshold and was 41% better than the nearest competition. (Refer to Exhibir 2 for background on machine learning GPUs and AlexNet) It was a defining moment in artificial intelligence, and it put the spotlight and deep-learning Al leadership firmly on NVIDIA. AlexNet was later bought by Google, which saw an opportunity to take Al to the next level of performance. Strategic Steps ... and Missteps In 2006, NVIDIA had acquired Indian-US company PortalPlayer, a designer of SoC hardware for personal media players, for $357 million.PortalPlayer had recently been dropped by Apple's iPod line, which had accounted for most of its revenue. Leveraging the PortalPlayer acquisition, NVIDIA launched its brand new "Tegra" SoC product line for mobile devices. Despite much lauded designs and great performance, it struggled in a competitive market and had limited success. In 2011, NVIDIA launched the Tegra 3 quad-core SoC. It was only when Audi and Tesla Motors both announced plans to use the Tegra 3 processor to power their vehicles' infotainment systems across their entire ranges worldwide that it became a strategic product for NVIDIA. Tegra gave NVIDIA entry into the automotive industry. Four years later the company would further develop this market with the launch of its Tegra-based Drive PX automotive platform. Not every strategic move resulted in success. Attempting to compete with mobile giant Qualcomm, NVIDIA acquired loera for $376 million in 2011." It planned to incorporate an LTE modem into its next-gen Tegra 4 SoC. Four years later, with only a negligible presence in the market, NVIDIA announced plans to sell icera and exit the mobile processor market completely. Our vision was right, but the mobile business is not for us. NVIDIA is not in the commodity chip business that the smartphone market has become ... our mobile experience was far from a bust. The future of computing is constrained by energy. The technology, methodologies, and design culture of energy efficiency have allowed us to build the world's most efficient GPU architecture, Jen-Hsun Huang, CEO, NVIDIA NVIDIA's Tegra SoC put it squarely in the running for the next generation of gaming consoles. Microsoft and Sony both wanted to increase the market share of their new consoles, the Xbox One and PlayStation 4. The key to achieving this would be to increase the number of applications that could run on their consoles and lower the development costs of third-party publishers. There were two options for Microsoft and Sony: ARM or x86, the only two CPU architectures that had the performance and developer support to achieve their goals. They also both wanted an SoC solution, as it used less power and space and generated less heat. ARM could not compete on pure performance and lacked a viable 64-bit option, important for memory-hungry games. That eliminated the ARM-based NVIDIA Tegra. Intel's potential solutions lacked the required graphics power to run games. In 2013, at "E3," the world's biggest computer game show, Microsoft and Sony announced their new consoles would both be using an SoC from AMD that would be custom-designed for cach console. NVIDIA claimed it had chosen not to compete as the financial rewards were insufficient. The Rise of Deep Learning Despite exiting the mobile phone business and losing out on both new gaming consoles, NVIDIA had an ace up its sleeve-its new Pascal microarchitecture. First announced by Huang at its GPU Technology conference in 2015, Pascal took three years to develop and was heavy on features designed to increase deep learning performance. The first Pascal-based product announcement in 2016 was not a gaming card, but the Tesla P100, designed for high- performance computing. It cost just under $10,000, had 3,584 CUDA cores, and promised a massive increase in neural network training performance compared to the previous generation. Included in the announcement was CUDA version 8 and new software called nvGRAPH that was aimed at accelerating big-data analytics. Huang announced to investors in 2017: Al fueled the rapid growth of our datacenter business, which tripled year over year in the fourth quarter, bringing total annual revenue to $830 million. We have a multi-year lead in Al computing (and we've built an end-to-end platform that starts with a GPU optimized for AL Our single architecture spans computers of every shape and size-PCs, servers, loT devices - and is available from every cloud service provider - Alibaba, Amazon, Google, IBM, Microsoft GPU deep learning is the most advanced, most accessible platform for Al. Jen-Hsun Huang, CEO, NVIDIA At the same time, in a world-first for both NVIDIA and the industry, the company launched the NVIDIA DGX-1, billed as an "Al super-computer in a box.* The off-the-shelf solution contained cight Tesla P100 cards in a standard medium-sized rack server enclosure costing $129,000. (Refer to Figure 1 for performance comparisons based on the workload of Reser 50, 90 epochs to solution, CPU server: Dual Xeon E5-26994, 2.6 GHz.) Google's Raja Monga also attended the press conference, talking about the DGX-l's use of TensorFlow, Google's open-source machine learning framework. 711 hours Figure 1: DGX-1 performance comparisons (Source: reproduced from NVIDIA calculations) The first DGX-1 produced was donated to Elon Musk's non-profit Al research company, OpenAL NVIDIA also gave nine others to prominent universities known for their deep- learning research departments, including: New York University, Stanford and the University of Toronto. Some companies, such as London-based BenevolentAl, considered the DGX-l's premium price to be worth the money when compared to a year's worth of the equivalent Amazon Web Services computer resource. Others, such as Baidu Al Labs' Greg Diamos, thought it likely that large customers would want to build their own custom servers. Both Microsoft and Facebook went on to create the HGX-1 and Big Basin respectively, with each server featuring eight Tesla P100 cards like the DGX-1. Meanwhile IBM created its IS822LC server, which used only four Tesla P100 cards mixed with Power CPUs, and an open-reference design that anyone could copy. Google on the other hand, announced its own data center hardware to accelerate machine learning, called the Tensor Processing Unit (TPU), although it was not commercially available. NVIDIA in 2018 In 1998, NVIDIA had 117 cmployees, and by January 2018, that number had grown to more than 8,000. The company had expanded beyond its original market of 3D graphics cards to four markets: gaming, professional visualization, datacenter and automotive. Despite the four different markets, the company employed a single-microarchitecture across all its products (Pascal and Volta). The strategy was to focus on markets where its architecture gave it a competitive edge, which meant a strong return on research and development (R&D). NVIDIA spent its entire R&D budget on researching new micro-architectures across a three-year horizon, then producing a new range of GPU products based on that architecture. NVIDIA'S GPU design realized strong year-over-year growth of 39% in FY 2017, representing 84.3% of total revenues. (Refer to Exhibir 3 for key financials and share price.) Gaming was the company's main revenue carner, but it was also riding on the wave of cryptocurrency miners. JPR research estimated that 3 million GPU's were bought by miners in 2017. NVIDIA's GeForce NOW application provided cloud gaming services. The global cloud computing market was expected to reach $411 billion by 2020. In early 2018, NVIDIA tried to enforce a GeForce Partner Program, obliging PC and add-in board vendors to use only NVIDIA'S GPUs in their high-end gaming brands, claiming it was an unfair use of their marketing support for vendors to use other GPUs. The company was targeted for being anti-competitive shortly afterwards. Professional visualization (virtualization, mobility, virtual reality and AI) was another market that NVIDIA was successfully advancing, working with customers by providing a visualization platform powered by advanced GPUs. Customers were using the platform to power a variety of medical advances. Arterys, for example, was using NVIDIA GPU-powered deep leaming to speed up analysis of medical images, which were deployed in GE Healthcare MRI machines to help diagnose heart disease. In 2017, Japan's Komatsu selected NVIDIA to provide GPUs to its construction sites to transform them into jobsites of the future that would be safer and more efficient For automotive, NVIDIA had launched its Drive PX1 Successor, the PX2, some months prior to the launch of the Tesla P100 filled DGX-1. It was aimed at autonomous driving and featured two Tegra-based SoCs and a Pascal-based GPU. NVIDIA's early automotive partner, Tesla Motors, having split from long-time partner Mobileye, announced that all future vehicles would include a Drive PX2 to enable "enhanced autopilot." Taking a platform approach, NVIDIA also partnered with Volvo, Audi, Daimler, BMW and Ford to test the PX2. It supplied Toyota, Volkswagen, Volvo, BMW, Daimler, Honda, Renault-Nissan, Bosch, Baidu and others. Despite NVIDIA's success, Tesla announced in a quarterly earnings call in August 2018 that it would build its own custom chips for its self-driving hardware. One analyst suggested that Tesla thought that chip vendors were slowing them down" or "locking them into a particular architecture, and it needed to own the technology. NVIDIA responded that it had already identified the issue and had successfully improved it, achieving 30 trillion operations per second (TOPS) with just 30 Watts. Tesla's custom version of the PX2 produced between 8- 10 TOPS. Further, in 2019, NVIDIA planned to launch the Drive Pegasus, which would achieve 320 TOPS at 500 Watts. Also threatening NVIDIA's dominance was rival Intel. Intel bought Israeli automotive company Mobileye in 2018. Mobileye's EyeQ3 chip powered Tesla's first generation of autopilot hardware, enabling Intel to enter the automotive market. Mobileye's latest chip. EyeQ5 was its third generation, and it had signed a deal for it to be deployed in eight million vehicles in 2019. Intel's approach differed from NVIDIA, preferring to co-develop processors optimized for integrated software applications, allowing the needs of the software algorithm to determine what goes into the hardware for a more efficient implementation. For data centers, NVIDIA worked with Alibaba Cloud, Baidu and Tencent to upgrade from Pascal to Volta-based platforms, giving them increased processing speed and scalability for Al inferencing and training. Amazon Web Services and Microsoft used NVIDIA GPUs for their deep learning applications. While Google continued to use NVIDIA GPUs in its cloud, despite announcing it would create its own hardware Cloud TPU. In March 2019, NVIDIA won a bidding war against Intel and Microsoft for Isracli chipmaker, Mellanox, for $6.9 billion. Mellanox produced chips for very high-speed networks, critical for expanding cloud services. Together, NVIDIA and Mellanox would power over 50% of the world's 500 biggest computers and cover every computer producer and major cloud service provider. In the press release, Huang explained: The emergence of Al and data science, as well as billions of simultaneous computer users, is fueling skyrocketing demand on the world's datacenters. Addressing this demand will require holistic architectures that connect vast numbers of fast computing nodes over intelligent networking fabrics to form a giant datacenter-scale computer engine. We're excited to unite NVIDIA's accelerated computing platform with Mellanox's world-renowned accelerated networking platform under one roof to create next-generation datacenter-scale computing solutions and I am particularly thrilled to work closely with the visionary leaders of Mellanox and their amazing people to invent the computers of tomorrow." Jen-Hsun Huang, CEO, NVIDIA Leading the Future of AI? In 2018, NVIDIA was dominant and growing rapidly in the deep learning Al space. Yet, there were competitive threats on the horizon that could challenge NVIDIA's position. These came from Google's Tensor flow processing unit (TPU) and companies including Intel, AMD and Silicon Valley start-ups, such as Wave computing, that claimed to be working on chips that were 10 times faster than NVIDIA's with less power consumption. China's Cambricon, backed by the Chinese government, while not targeting deep-learning training, could change strategy and was a potential threat One commentator suggested that he viewed NVIDIA not as a GPU company but as a "platform company with an insatiable appetite for growth and an ability to pivot quickly when threatened. The question facing the company was: Did NVIDIA have a clear strategy and roadmap to grow as an Al platform company? Was that the right strategy or should it continue to explore and exploit other core markets? What did NVIDIA need to do tactically to retain its leadershipStep by Step Solution
There are 3 Steps involved in it
Step: 1
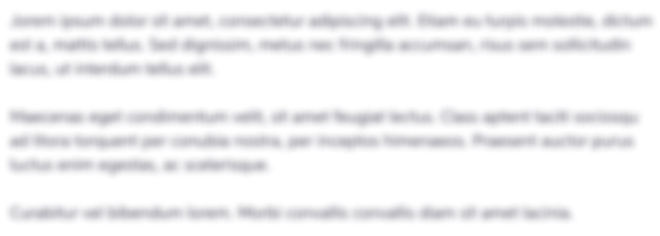
Get Instant Access to Expert-Tailored Solutions
See step-by-step solutions with expert insights and AI powered tools for academic success
Step: 2

Step: 3

Ace Your Homework with AI
Get the answers you need in no time with our AI-driven, step-by-step assistance
Get Started