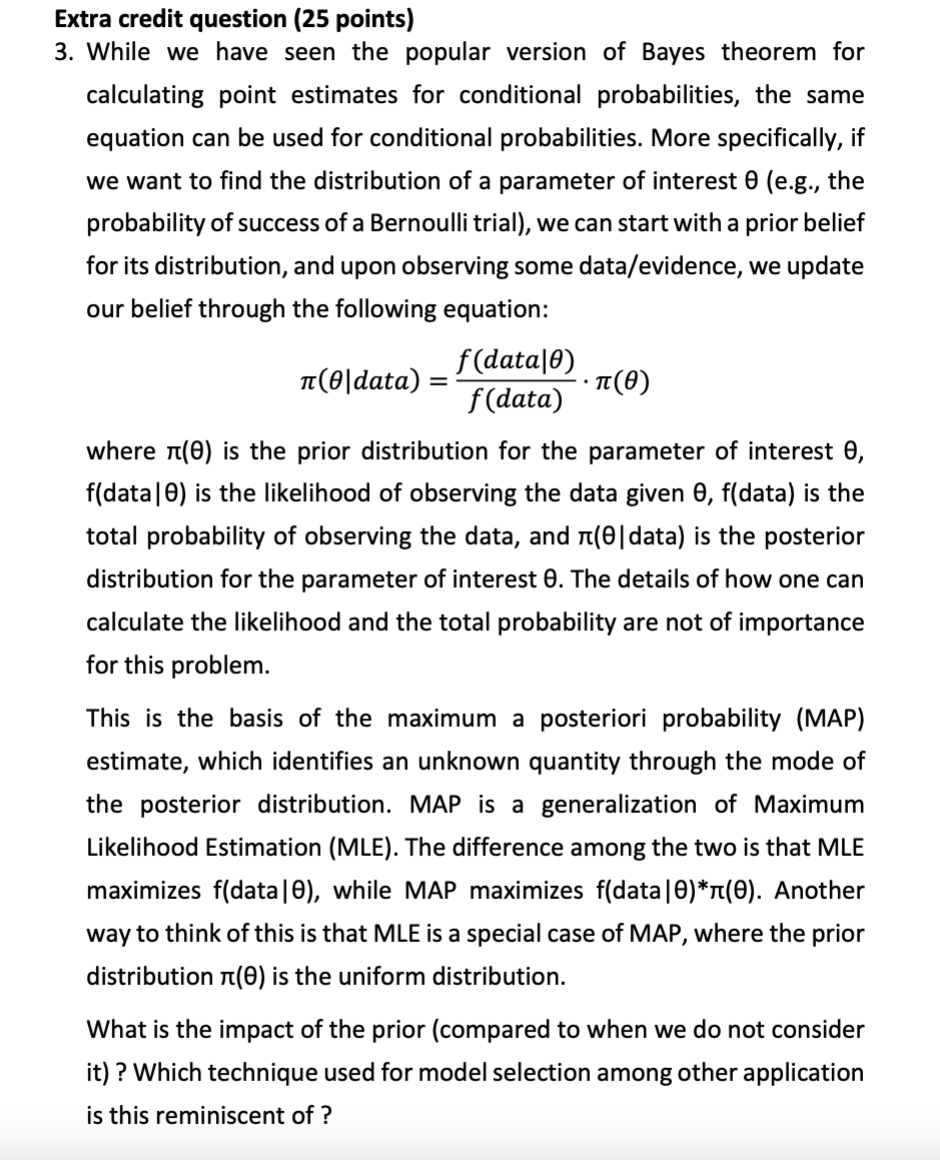
Extra credit question (25 points) 3. While we have seen the popular version of Bayes theorem for calculating point estimates for conditional probabilities, the same equation can be used for conditional probabilities. More specifically, if we want to find the distribution of a parameter of interest 9 (e.g., the probability of success of a Bernoulli trial), we can start with a prior belief for its distribution, and upon observing some data/evidence, we update our belief through the following equation: f(data|0) T(@data) = 0(0) f(data) where r(0) is the prior distribution for the parameter of interest 0, f(data|0) is the likelihood of observing the data given 0, f(data) is the total probability of observing the data, and r(0|data) is the posterior distribution for the parameter of interest 8. The details of how one can calculate the likelihood and the total probability are not of importance for this problem. This is the basis of the maximum a posteriori probability (MAP) estimate, which identifies an unknown quantity through the mode of the posterior distribution. MAP is a generalization of Maximum Likelihood Estimation (MLE). The difference among the two is that MLE maximizes f(data|0), while MAP maximizes f(data|0)*7(0). Another way to think of this is that MLE is a special case of MAP, where the prior distribution 1(O) is the uniform distribution. What is the impact of the prior (compared to when we do not consider it) ? Which technique used for model selection among other application is this reminiscent of ? Extra credit question (25 points) 3. While we have seen the popular version of Bayes theorem for calculating point estimates for conditional probabilities, the same equation can be used for conditional probabilities. More specifically, if we want to find the distribution of a parameter of interest 9 (e.g., the probability of success of a Bernoulli trial), we can start with a prior belief for its distribution, and upon observing some data/evidence, we update our belief through the following equation: f(data|0) T(@data) = 0(0) f(data) where r(0) is the prior distribution for the parameter of interest 0, f(data|0) is the likelihood of observing the data given 0, f(data) is the total probability of observing the data, and r(0|data) is the posterior distribution for the parameter of interest 8. The details of how one can calculate the likelihood and the total probability are not of importance for this problem. This is the basis of the maximum a posteriori probability (MAP) estimate, which identifies an unknown quantity through the mode of the posterior distribution. MAP is a generalization of Maximum Likelihood Estimation (MLE). The difference among the two is that MLE maximizes f(data|0), while MAP maximizes f(data|0)*7(0). Another way to think of this is that MLE is a special case of MAP, where the prior distribution 1(O) is the uniform distribution. What is the impact of the prior (compared to when we do not consider it) ? Which technique used for model selection among other application is this reminiscent of