Question
Given the following silhouette scores, how many clusters should you choose? Silhouette scores of 2 clusters: 1. 0.8 2. 0.6 Silhouette scores of 3 clusters:
- Given the following silhouette scores, how many clusters should you choose?
Silhouette scores of 2 clusters:
1. 0.8
2. 0.6
Silhouette scores of 3 clusters:
1. 0.6
2. 0.5
3. 0.4
Silhouette scores of 4 clusters:
1. 0.7
2. 0.8
3. 0.6
4. 0.7
Given the following silhouette scores, how many clusters should you choose?
Silhouette scores of 2 clusters:
1. 0.8
2. 0.6
Silhouette scores of 3 clusters:
1. 0.6
2. 0.5
3. 0.4
Silhouette scores of 4 clusters:
1. 0.7
2. 0.8
3. 0.6
4. 0.7
1 or 2
1
1 or 3
3
2
- or 3
- What risks could bootstrapping result in, with regard to ensemble learning? Select the best answer. What risks could bootstrapping result in, with regard to ensemble learning? Select the best answer.
Inefficiency due to looping through observations
Excluding some observations altogether
Impure splits
Computational complexity
- In which of the following scenarios would ward linkage work best? Select all that apply
Noisy data
Cylindrical data
Cleanly separable data
Highly random data
- In which of these scenarios should we use a decision tree over another model?
Predicting which products a customer is likely to buy
Blocking a credit card based on fraudulent transactions
Predicting which ads to display to a customer
- A decision tree with high depth is likely to suffer from ________. Select the best answer.
Underfitting
High RMSE
Overfitting
Low accuracy
- In which of the following scenarios would you expect DBSCAN to work better than other clustering techniques?
When trying to cluster time series data
When trying to cluster customer data where a lot of features are available
When trying to cluster categorical data
When trying to cluster customer data where a lot of features are not available
- In model-based recommendation engines, the algorithm tests iterative values of feature matrices until it's able to predict a ratings matrix accurately. What happens when the SSE is high?
The algorithm attempts one final iteration
The algorithm reverts to the last set of values
The algorithm tries a new set of feature values
Nothing - a high SSE means the ratings are fairly accurate
- Which of the following is a scenario where the kernel trick in a support vector model is helpful? Select the best answer.
When data follows a straight line pattern
When data has clearly defined, round clusters
When data is scattered in an undefined pattern
When data is not linearly separable
- How does shrinkage help combat overfitting in boosting algorithms?
It removes unnecessary branches from tree models
It reduces the influence of each model on the overall result
It regularizes insignificant coefficients to 0
It minimizes insignificant coefficients
- What are support vectors? Select the best answer.
The examples furthest from the hyperplane.
The examples which influence the position of the hyperplane.
The examples which influence the decision boundary.
The examples closest to the hyperplane.
- How does an AdaBoost model make a prediction?
Soft voting for classification and averaging for regression problems
Majority voting for classification and regression problems
Majority voting for classification and averaging for regression problems
Weighted average voting for classification and regression problems
- What depth of trees does an AdaBoost model use?
1
2
Any depth
0
Step by Step Solution
There are 3 Steps involved in it
Step: 1
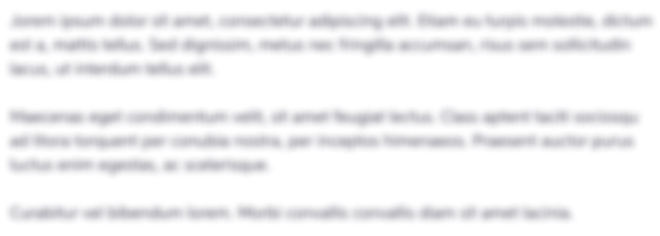
Get Instant Access to Expert-Tailored Solutions
See step-by-step solutions with expert insights and AI powered tools for academic success
Step: 2

Step: 3

Ace Your Homework with AI
Get the answers you need in no time with our AI-driven, step-by-step assistance
Get Started