Question
Goal: Develop an appreciation for using deep learning solutions for different types of problems (regression, binary classification, multiclass classification). Learning objectives: Learn how to implement
Goal: Develop an appreciation for using deep learning solutions for different types of problems (regression, binary classification, multiclass classification). Learning objectives: Learn how to implement deep learning solutions to classical problems in regression and (binary and multiclass) classification using Python, Keras, TensorFlow, and Jupyter Notebooks. Get acquainted with representative datasets and problems in deep learning. Learn how to implement, train, and evaluate fully connected neural networks. Starter package Starter code: A2_starter_CAP6619.ipynb (available on Google Colab check link on Canvas) Instructions: Document all your findings, steps, conclusions, lessons learned, insights, etc. in your report (think of it as a lab notebook) Add your answers to the numbered questions in (or right before) the Conclusions section of your report. Procedure: 1. Access the instructor's notebook containing the starter code on Google Colab. NB: You must use your FAU account! 2. Make a copy of the notebook and save it to your Google Drive. 3. Run the starter code and ensure that it works as intended. 4. (OPTIONAL) Work on TODO items marked as OPTIONAL in the starter code notebook (for a maximum of 10% bonus on your grade for the assignment). 5. Answer the questions below: PART 1: (1) What type of preprocessing was applied to the raw input data? Why was it necessary to do so? (2) Does your model suffer from overfitting? If so, what would you suggest doing about it? (3) Is accuracy a good metric of success in this case? Why (not)?
CAP 6619 Deep Learning Dr. Marques Project 2 Spring 2023 2 PART 2: (1) What type of preprocessing was applied to the raw input data? Why was it necessary to do so? (2) How many categories are there in this case? (3) Does your model suffer from overfitting? If so, what would you suggest doing about it? (4) Is accuracy a good metric of success in this case? Why (not)? PART 3: (1) What type of preprocessing was applied to the raw input data? Why was it necessary to do so? (2) Why is this problem a case of regression (rather than classification)? (3) Does your model suffer from overfitting? If so, what would you suggest doing about it? (4) Is mean absolute error (MAE) a good metric of success in this case? Why (not)? 6. Prepare your report, with all relevant plots, code snippets, numerical values and most importantly your insights and lessons learned. 7. Save your report as a PDF file. 8. Submit the PDF file and the URL of your Google Colab notebook (*) via Canvas. ________________________________ (*) Make sure to choose the option Share with anyone with the link (with viewer privileges) rather than restricting it to FAU email addresses. See screenshot below.
Step by Step Solution
There are 3 Steps involved in it
Step: 1
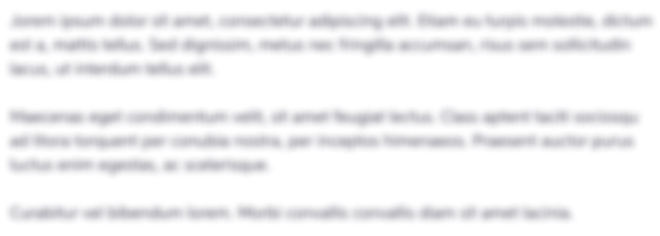
Get Instant Access to Expert-Tailored Solutions
See step-by-step solutions with expert insights and AI powered tools for academic success
Step: 2

Step: 3

Ace Your Homework with AI
Get the answers you need in no time with our AI-driven, step-by-step assistance
Get Started