Question
I attached the entire code but the only parts that need to be fixed are the def calc_movie_feature_matrix in class ContentBased, calc_item_item_similarity in class ContentBased,
I attached the entire code but the only parts that need to be fixed are the def calc_movie_feature_matrix in class ContentBased, calc_item_item_similarity in class ContentBased, and predict_from_sim in class RecSys. I also attached the test cells for this part, please make sure these are running. I have the data and the libraries I uploaded as well here. I feel I have tried so many times so please help me :) It is okay if you need to completely change code instead of just fixing it. Test cells cannot be changed.
import pandas as pd
import matplotlib.pyplot as plt
import numpy as np
import time
from sklearn.model_selection import train_test_split
from scipy.sparse import coo_matrix, csr_matrix
from scipy.spatial.distance import jaccard, cosine
from pytest import approx
MV_users = pd.read_csv('data/users.csv')
MV_movies = pd.read_csv('data/movies.csv')
train = pd.read_csv('data/train.csv')
test = pd.read_csv('data/test.csv')
from collections import namedtuple
Data = namedtuple('Data', ['users','movies','train','test'])
data = Data(MV_users, MV_movies, train, test)
#entire code for reference
import numpy as np
from scipy.sparse import coo_matrix
class RecSys():
def __init__(self, data):
self.data = data
self.allusers = list(self.data.users['uID'])
self.allmovies = list(self.data.movies['mID'])
self.genres = list(self.data.movies.columns.drop(['mID', 'title', 'year']))
self.mid2idx = dict(zip(self.data.movies.mID, list(range(len(self.data.movies)))))
self.uid2idx = dict(zip(self.data.users.uID, list(range(len(self.data.users)))))
self.Mr = self.rating_matrix()
self.Mm = None
self.sim = np.zeros((len(self.allmovies), len(self.allmovies)))
def rating_matrix(self):
"""
Convert the rating matrix to numpy array of shape (#allusers, #allmovies)
"""
ind_movie = [self.mid2idx[x] for x in self.data.train.mID]
ind_user = [self.uid2idx[x] for x in self.data.train.uID]
rating_train = list(self.data.train.rating)
return np.array(coo_matrix((rating_train, (ind_user, ind_movie)), shape=(len(self.allusers), len(self.allmovies))).toarray())
def predict_everything_to_3(self):
"""
Predict everything to 3 for the test data
"""
return np.full(len(self.data.test), 3)
def predict_to_user_average(self):
"""
Predict average rating for the user.
Returns numpy array of shape (#users,)
"""
# Average Rating Computation:
sum_of_ratings_for_allUsers = self.Mr.sum(axis=1)
count_of_valid_ratings_for_allUsers = (self.Mr!=0).sum(axis=1)
# Stores avg rating for all users
avg_rating_for_allUsers = sum_of_ratings_for_allUsers / count_of_valid_ratings_for_allUsers
# Retireve rating for data.test.uID from 'avg_rating_for_allUsers'
# Hint: We pass indexes in above array because it stores indexes instead of actual UID or MIDs.
index_of_testUsers = [self.uid2idx[actual_uid] for actual_uid in self.data.test.uID]
# Rating for all test users:
avg_rating_for_testUsers = avg_rating_for_allUsers[index_of_testUsers]
return avg_rating_for_testUsers
def predict_from_sim(self, uid, mid):
user_idx = self.uid2idx[uid]
movie_idx = self.mid2idx[mid]
user_ratings = self.Mr[user_idx, :]
sim_ratings = self.Mr[user_idx] * self.sim[:, movie_idx]
return np.dot(user_ratings, sim_ratings) / np.sum(sim_ratings)
def predict(self):
"""
Predict ratings in the test data. Returns predicted rating in a numpy array of size (# of rows in testdata,)
"""
predictions = []
for i in range(len(self.data.test)):
uid = self.data.test.iloc[i]['uID']
mid = self.data.test.iloc[i]['mID']
predictions.append(self.predict_from_sim(uid, mid))
return np.array(predictions)
def rmse(self, yp):
yp[np.isnan(yp)] = 3 # In case there are NaN values in prediction, impute them with 3
yt = np.array(self.data.test.rating)
return np.sqrt(((yt - yp) ** 2).mean())
from scipy.spatial.distance import pdist, squareform
class ContentBased(RecSys):
from sklearn.metrics.pairwise import pairwise_distances
def __init__(self, data):
super().__init__(data)
self.data = data
self.Mm = self.calc_movie_feature_matrix()
def calc_movie_feature_matrix(self):
"""
Create movie feature matrix in a numpy array of shape (#allmovies, #genres)
"""
movie_features = np.zeros((len(self.allmovies), len(self.genres)))
for i, movie in self.data.movies.iterrows():
movie_id = movie['mID']
movie_idx = self.mid2idx[movie_id]
for j, genre in enumerate(self.genres):
if movie[genre] == 1:
movie_features[movie_idx, j] = 1
return coo_matrix(movie_features)
def calc_item_item_similarity(self):
"""
Create item-item similarity using Jaccard similarity
"""
self.sim = 1 - pairwise_distances(self.Mm, metric="jaccard")
def jaccard_similarity(self, X):
"""
Calculates item-item similarity for all pairs of items using Jaccard similarity (values from 0 to 1)
X is the feature matrix.
"""
intersection = X.dot(X.T)
union = np.expand_dims(X.any(axis=1), axis=1) + np.expand_dims(X.any(axis=1), axis=0) - intersection
similarity = intersection / union
np.fill_diagonal(similarity, 0) # Set diagonal elements to 0
return similarity
class Collaborative(RecSys):
import numpy as np
def __init__(self, data):
super().__init__(data)
def calc_item_item_similarity(self, simfunction, *X):
"""
Create item-item similarity using similarity function.
X is an optional transformed matrix of Mr
"""
if len(X) == 0:
self.sim = simfunction()
else:
self.sim = simfunction(X[0])
def cossim(self):
"""
Calculates item-item similarity for all pairs of items using cosine similarity (values from 0 to 1) on utility matrix
Returns a cosine similarity matrix of size (#all movies, #all movies)
"""
norm = np.linalg.norm(self.Mr, axis=1, keepdims=True)
self.sim = self.Mr.dot(self.Mr.T) / (norm * norm.T)
np.fill_diagonal(self.sim, 0) # Set diagonal elements to 0
return self.sim
def jacsim(self, Xr):
"""
Calculates item-item similarity for all pairs of items using Jaccard similarity (values from 0 to 1)
Xr is the transformed rating matrix.
"""
intersection = Xr.dot(Xr.T)
union = np.expand_dims(Xr.sum(axis=1), axis=1) + np.expand_dims(Xr.sum(axis=1), axis=0) - intersection
similarity = intersection / union
np.fill_diagonal(similarity, 0) # Set diagonal elements to 0
return similarity
#test cells for 2a
cb = ContentBased(data)
# tests calc_movie_feature_matrix in the class ContentBased
assert(cb.Mm.shape==(3883, 18))
#test cells for 2b
cb.calc_item_item_similarity()
# Sample tests calc_item_item_similarity in ContentBased class
sample_cb = ContentBased(sample_data)
sample_cb.calc_item_item_similarity()
# print(np.trace(sample_cb.sim))
# print(sample_cb.sim[10:13,10:13])
assert(sample_cb.sim.sum() > 0), "Check calc_item_item_similarity."
assert(np.trace(sample_cb.sim) == 3152), "Check calc_item_item_similarity. What do you think np.trace(cb.sim) should be?"
ans = np.array([[1, 0.25, 0.],[0.25, 1, 0.],[0., 0., 1]])
for pred, true in zip(sample_cb.sim[10:13, 10:13], ans):
assert approx(pred, 0.01) == true, "Check calc_item_item_similarity. Look at cb.sim"
#test cells for 2c
# for a, b in zip(sample_MV_users.uID, sample_MV_movies.mID):
# print(a, b, sample_cb.predict_from_sim(a,b))
# Sample tests for predict_from_sim in RecSys class
assert(sample_cb.predict_from_sim(245,276)==approx(2.5128205128205128,abs=1e-2)), "Check predict_from_sim. Look at how you predicted a user rating on a movie given UserID and movieID."
assert(sample_cb.predict_from_sim(2026,2436)==approx(2.785714285714286,abs=1e-2)), "Check predict_from_sim. Look at how you predicted a user rating on a movie given UserID and movieID."
# test cells for 2d
# Sample tests method predict in the RecSys class
sample_yp = sample_cb.predict()
sample_rmse = sample_cb.rmse(sample_yp)
print(sample_rmse)
assert(sample_rmse==approx(1.1962537249116723, abs=1e-2)), "Check method predict in the RecSys class."
# Hidden tests method predict in the RecSys class
yp = cb.predict()
rmse = cb.rmse(yp)
print(rmse)
I attached screenshots here of the error messages I am getting.
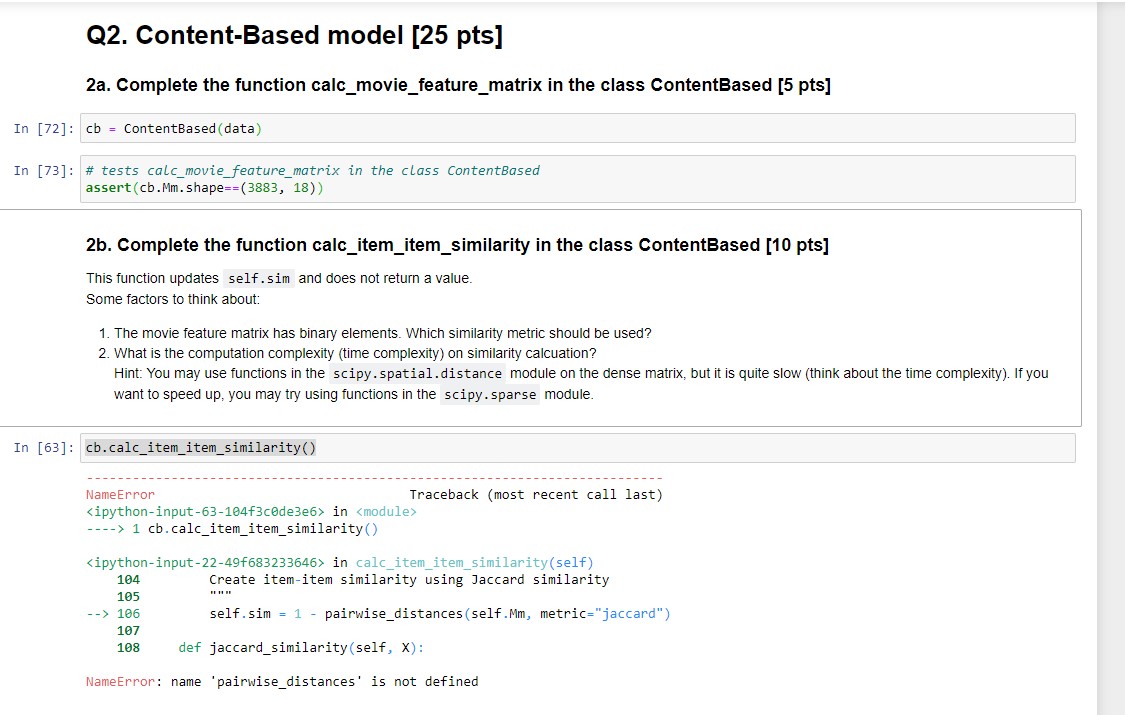
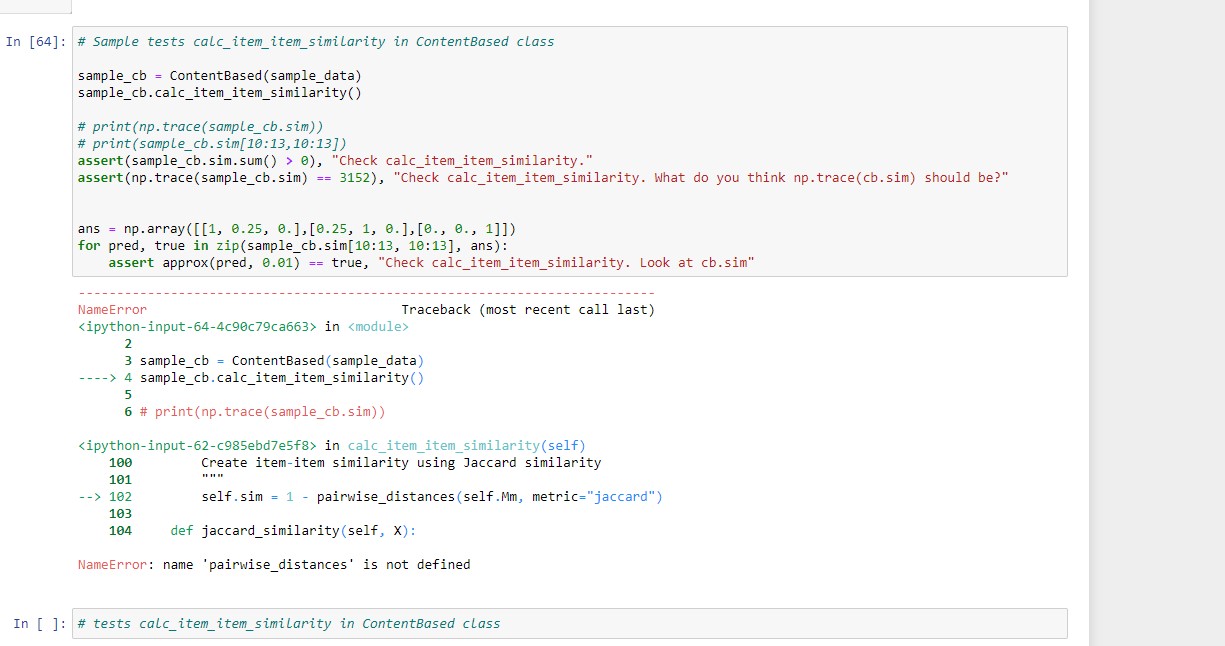
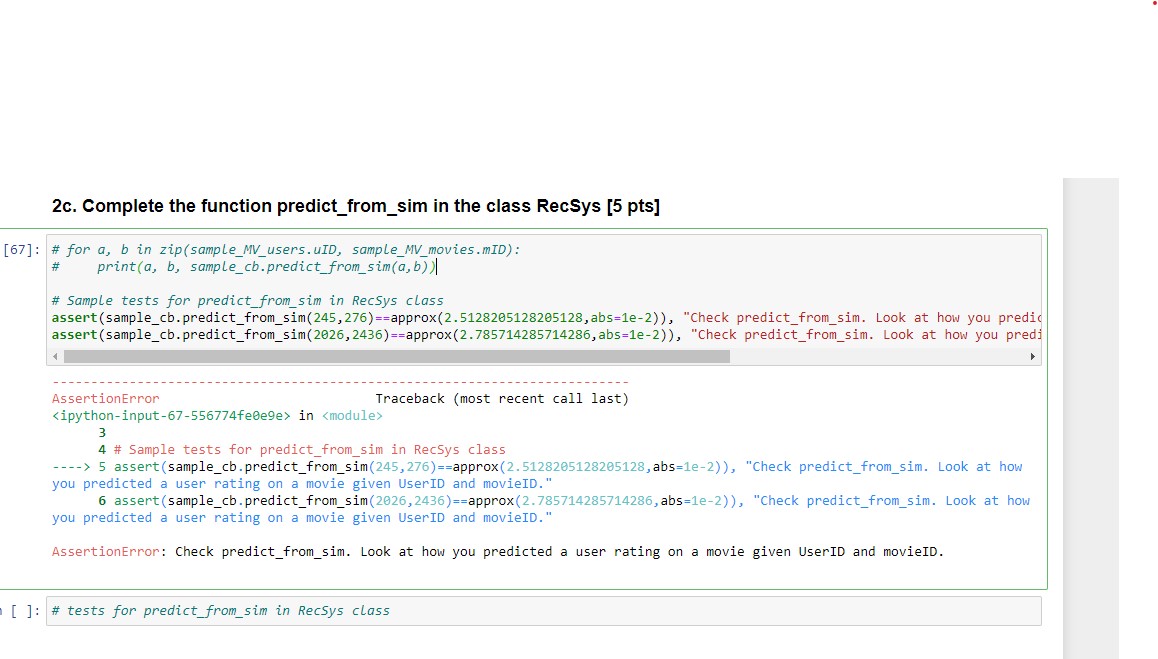
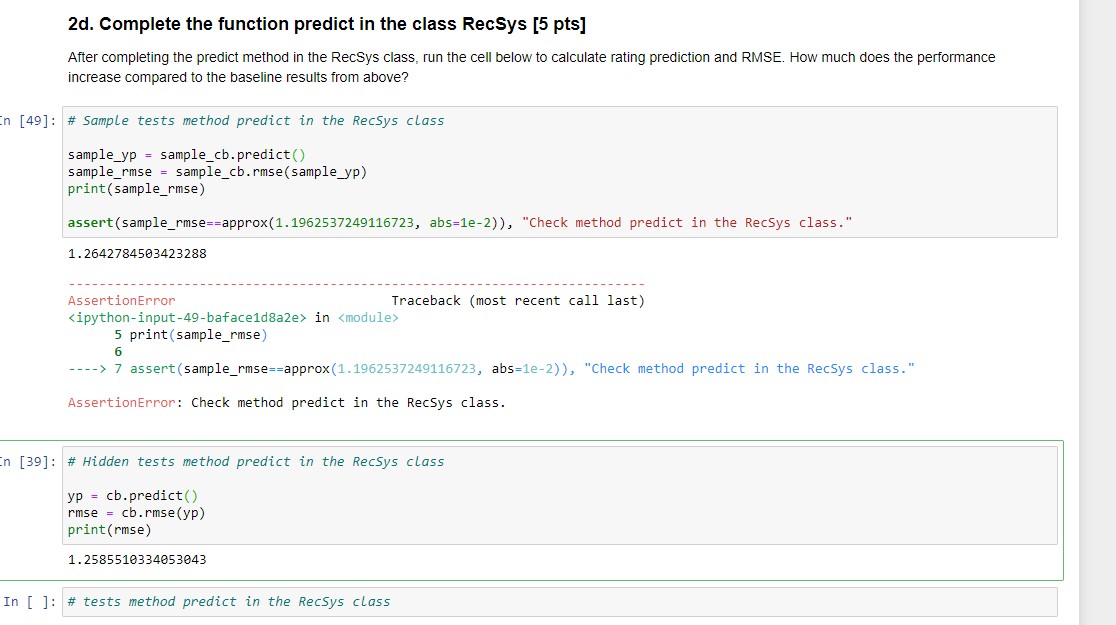
Q2. Content-Based model [25 pts] 2a. Complete the function calc_movie_feature_matrix in the class ContentBased [5 pts] In [72]: cb= ContentBased (data) In [73] # tests calc_movie_feature_matrix in the class ContentBased assert(cb.Mm.shape==(3883, 18)) 2b. Complete the function calc_item_item_similarity in the class ContentBased [10 pts] This function updates self.sim and does not return a value. Some factors to think about: 1. The movie feature matrix has binary elements. Which similarity metric should be used? 2. What is the computation complexity (time complexity) on similarity calcuation? Hint: You may use functions in the scipy.spatial.distance module on the dense matrix, but it is quite slow (think about the time complexity). If you want to speed up, you may try using functions in the scipy.sparse module. In [63]: cb.calc_item_item_similarity() NameError Traceback (most recent call last) in ----> 1 cb.calc_item_item_similarity() in calc_item_item_similarity(self) Create item-item similarity using Jaccard similarity 104 105 --> 106 107 108 self.sim = 1 pairwise distances (self.Mm, metric="jaccard") def jaccard similarity (self, x): NameError: name 'pairwise_distances' is not defined
Step by Step Solution
There are 3 Steps involved in it
Step: 1
address the issues youre encountering lets focus on fixing the calcmoviefeaturematrix calcitemitemsimilarity and predictfromsim methods as per your request Below are the modifications import numpy as ...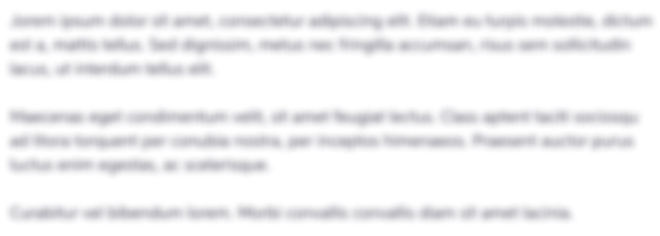
Get Instant Access to Expert-Tailored Solutions
See step-by-step solutions with expert insights and AI powered tools for academic success
Step: 2

Step: 3

Ace Your Homework with AI
Get the answers you need in no time with our AI-driven, step-by-step assistance
Get Started