Answered step by step
Verified Expert Solution
Question
1 Approved Answer
ID Salary Compa Midpoint Age 4 5 6 7 8 9 10 11 12 13 14 15 19 20 21 22 26 27 30 31
ID Salary Compa Midpoint Age 4 5 6 7 8 9 10 11 12 13 14 15 19 20 21 22 26 27 30 31 32 43 44 49 50 1 2 3 16 17 18 23 24 25 28 29 33 34 35 36 37 38 39 40 41 42 45 46 47 48 75.4 72 46 26.9 59.8 65.4 24.2 22.7 77.2 63.2 51 62.9 23 22.6 23.4 63.7 39.4 24 23.3 36.1 63.5 26.5 61.4 45.2 69.6 74.2 23.4 24.5 26.8 24.9 66.5 23.6 61.7 34.3 57.9 22.9 60 34.7 42 46.6 36.3 76.7 57.3 22.5 54.7 24.5 48.5 74.3 22.6 41.3 1.125 67 67 48 31 57 57 23 23 67 57 48 57 23 23 23 57 40 23 23 31 57 31 57 40 57 67 23 23 31 23 57 23 57 31 57 23 57 31 40 40 31 67 48 23 48 23 48 67 23 40 44 52 45 25 35 45 24 32 42 45 36 37 22 23 27 39 35 29 22 27 41 26 38 25 34 49 41 32 52 32 27 32 52 44 42 32 34 30 32 44 31 43 48 36 30 41 36 36 30 30 1.075 0.958 0.867 1.049 1.147 1.053 0.989 1.152 1.108 1.062 1.104 0.998 0.982 1.017 1.117 0.985 1.045 1.014 1.164 1.114 0.856 1.078 1.130 1.221 1.107 1.018 1.064 0.866 1.084 1.166 1.025 1.082 1.107 1.016 0.997 1.053 1.120 1.050 1.166 1.170 1.145 1.193 0.979 1.140 1.067 1.010 1.109 0.984 1.033 Performance Service Gender Rating 95 95 90 95 90 95 90 100 95 90 95 95 95 90 75 75 80 60 95 90 95 80 80 80 90 100 100 85 80 80 55 90 95 70 100 90 85 75 100 90 80 95 65 65 75 70 90 70 80 100 9 5 18 4 9 11 2 8 20 16 8 5 2 4 3 20 7 4 2 6 21 2 12 5 11 10 19 1 7 8 3 9 22 16 16 12 8 5 8 4 11 13 6 6 9 4 16 12 7 2 1 0 0 0 0 0 0 1 1 0 1 0 1 1 1 0 0 1 1 1 0 0 0 0 1 0 1 0 0 1 1 1 0 1 0 1 0 1 1 0 1 0 1 1 1 0 0 0 1 1 Raise ID Salary Compa Midpoint Age 4.4 5.4 4.3 5.6 5.5 4.5 6.3 5.7 5.5 5.2 5.2 5.5 6.2 5.3 4.3 3.9 3.9 3.9 6.2 5.5 6.6 4.9 4.6 4.3 5.3 4 4.8 4.6 3.9 4.9 3 5.8 4.5 4.8 5.5 6 5.7 3.6 5.7 5.7 5.6 6.3 3.8 3.3 3.8 4 5.7 4.5 4.7 4.7 8 10 11 14 15 23 26 31 35 36 37 42 3 18 20 39 7 13 22 24 45 17 48 28 43 19 25 40 2 32 34 16 27 41 5 30 1 4 12 33 38 44 46 47 49 50 6 9 21 29 23.6 22.6 23.4 22.9 24.9 22.5 23 24 22.6 23.4 23.3 22.7 34.7 36.3 34.3 36.1 42 41.3 57.3 54.7 51 66.5 69.6 75.4 77.2 24.5 24.5 24.2 26.8 26.9 26.5 46.6 39.4 45.2 48.5 46 60 57.9 61.7 59.8 65.4 63.2 63.7 62.9 63.5 61.4 74.3 74.2 76.7 72 1.025 23 23 23 23 23 23 23 23 23 23 23 23 31 31 31 31 40 40 48 48 48 57 57 67 67 23 23 23 31 31 31 40 40 40 48 48 57 57 57 57 57 57 57 57 57 57 67 67 67 67 32 30 41 32 32 36 22 29 23 27 22 32 30 31 44 27 32 30 48 30 36 27 34 44 42 32 41 24 52 25 26 44 35 25 36 45 34 42 52 35 45 45 39 37 41 38 36 49 43 52 0.984 1.018 0.997 1.084 0.979 0.998 1.045 0.982 1.017 1.014 0.989 1.120 1.170 1.107 1.164 1.050 1.033 1.193 1.140 1.062 1.166 1.221 1.125 1.152 1.064 1.067 1.053 0.866 0.867 0.856 1.166 0.985 1.130 1.010 0.958 1.053 1.016 1.082 1.049 1.147 1.108 1.117 1.104 1.114 1.078 1.109 1.107 1.145 1.075 Performa Service nce Rating 90 9 80 7 100 19 90 12 80 8 65 6 95 2 60 4 90 4 75 3 95 2 100 8 75 5 80 11 70 16 90 6 100 8 100 2 65 6 75 9 95 8 55 3 90 11 95 9 95 20 85 1 70 4 90 2 80 7 95 4 80 2 90 4 80 7 80 5 90 16 90 18 85 8 100 16 95 22 90 9 95 11 90 16 75 20 95 5 95 21 80 12 70 12 100 10 95 13 95 5 Gender Raise 1 1 1 1 1 1 1 1 1 1 1 1 1 1 1 1 1 1 1 1 1 1 1 1 1 0 0 0 0 0 0 0 0 0 0 0 0 0 0 0 0 0 0 0 0 0 0 0 0 0 5.8 4.7 4.8 6 4.9 3.3 6.2 3.9 5.3 4.3 6.2 5.7 3.6 5.6 4.8 5.5 5.7 4.7 3.8 3.8 5.2 3 5.3 4.4 5.5 4.6 4 6.3 3.9 5.6 4.9 5.7 3.9 4.3 5.7 4.3 5.7 5.5 4.5 5.5 4.5 5.2 3.9 5.5 6.6 4.6 4.5 4 6.3 5.4 Degree Gender1 1 1 1 1 1 0 0 1 0 0 0 1 1 0 0 0 1 0 1 0 1 1 1 0 0 1 0 0 0 0 1 0 1 0 1 0 0 1 0 1 0 1 1 1 0 0 1 1 1 0 F F F F F F F F F F F F F F F F F F F F F F F F F M M M M M M M M M M M M M M M M M M M M M M M M M Gr A A A A A A A A A A A A B B B B C C D D D E E F F A A A B B B C C C D D E E E E E E E E E E F F F F 1.053 0.087 1.073 0.076 Week 1. Measurement and Description - chapters 1 and 2 1 The goal this week is to gain an understanding of our data set - what kind of data we are looking at, some descriptive measurse, and a look at how the data is distributed (shape). Measurement issues. Data, even numerically coded variables, can be one of 4 levels nominal, ordinal, interval, or ratio. It is important to identify which level a variable is, as this impact the kind of analysis we can do with the data. For example, descriptive statistics such as means can only be done on interval or ratio level data. Please list under each label, the variables in our data set that belong in each group. Nominal Ordinal Interval Ratio ID Degree Perf. Rat. Salary Gender Grade Compa Gender 1 Age Midpoint Service b. For each variable that you did not call ratio, why did you make that decision? For Nominal, the ID Gender and Gender 1 are labels with no actual order to them For Ordinal, the Degree and Grade are rank ordered labels without a fixed differeance between levels For Interval, the Pref Rat. is done once than a break in the activity before completed again. It has no fixed 0 point, but differences are the same between values. 2 The first step in analyzing data sets is to find some summary descriptive statistics for key variables. For salary, compa, age, performance rating, and service; find the mean, standard deviation, and range for 3 groups: overall sample, Females, and Males. You can use either the Data Analysis Descriptive Statistics tool or the Fx =average and =stdev functions. (the range must be found using the difference between the =max and =min functions with Fx) functions. Note: Place data to the right, if you use Descriptive statistics, place that to the right as well. Some of the values are completed for you - please finish the table. Salary Compa Age Perf. Rat. Service Overall Mean 45.02 1.0632 35.7 85.9 9.0 Standard Deviation 19.2083 0.0816 8.2513 11.4147 5.7177 Note - data is a sample from the larger company population Range 54.7000 0.3650 30 45 21 Female Mean 38.2120 1.0734 32.5 84.2 7.9 Standard Deviation 18.5321 0.0758 6.9 13.6 4.9 Range 54.7000 0.2420 26.0 45.0 18.0 Male Mean 51.8320 1.0530 38.9 87.6 10.0 Standard Deviation 17.6984 0.0873 8.4 8.7 6.4 Range 52.5000 0.3100 28.0 30.0 21.0 3 What is the probability for a: Probability a. Randomly selected person being a male in grade E? b. Randomly selected male being in grade E? Note part b is the same as given a male, what is probabilty of being in grade E? c. Why are the results different? 4 5. A key issue in comparing data sets is to see if they are distributed/shaped the same. We can do this by looking at some measures of where some selected values are within each data set - that is how many values are above and below a comparable value. For each group (overall, females, and males) find: Overall Female Male A The value that cuts off the top 1/3 salary value in each group 59.1667 41.3000 62.9000 "=large" function i The z score for this value within each group? 0.7364 0.1666 0.6254 Excel's standize function ii The normal curve probability of exceeding this score: 0.9894 0.9800 0.9981 1-normsdist function iii What is the empirical probability of being at or exceeding this salary value? B The value that cuts off the top 1/3 compa value in each group. 1.1077 1.1200 1.1070 i The z score for this value within each group? 0.5447 0.6145 0.6179 ii The normal curve probability of exceeding this score: 1.0000 1.0000 1.0000 iii What is the empirical probability of being at or exceeding this compa value? C How do you interpret the relationship between the data sets? What do they mean about our equal pay for equal work question? What conclusions can you make about the issue of male and female pay equality? Are all of the results consistent? What is the difference between the sal and compa measures of pay? Conclusions from looking at salary results: Men are generally paid higher Conclusions from looking at compa results: The male and female compa results look closer to each other. Slightly higher for the female Do both salary measures show the same results? No they do not. Can we make any conclusions about equal pay for equal work yet? Females are still not making the same amount as their male counterparts. ID Salary 1 2 3 4 5 6 7 8 9 10 11 12 13 14 15 16 17 18 19 20 21 22 23 24 25 26 27 28 29 30 31 32 33 34 35 36 37 38 39 40 41 42 43 44 45 46 47 48 49 50 60 26.8 34.7 57.9 48.5 74.3 42 23.6 74.2 22.6 23.4 61.7 41.3 22.9 24.9 46.6 66.5 36.3 24.5 34.3 76.7 57.3 22.5 54.7 24.5 23 39.4 75.4 72 46 24 26.9 59.8 26.5 22.6 23.4 23.3 65.4 36.1 24.2 45.2 22.7 77.2 63.2 51 63.7 62.9 69.6 63.5 61.4 Compa Midpoint 1.053 0.866 1.120 1.016 1.010 1.109 1.050 1.025 1.107 0.984 1.018 1.082 1.033 0.997 1.084 1.166 1.166 1.170 1.064 1.107 1.145 1.193 0.979 1.140 1.067 0.998 0.985 1.125 1.075 0.958 1.045 0.867 1.049 0.856 0.982 1.017 1.014 1.147 1.164 1.053 1.130 0.989 1.152 1.108 1.062 1.117 1.104 1.221 1.114 1.078 57 31 31 57 48 67 40 23 67 23 23 57 40 23 23 40 57 31 23 31 67 48 23 48 23 23 40 67 67 48 23 31 57 31 23 23 23 57 31 23 40 23 67 57 48 57 57 57 57 57 Age Perform ance Rating Service Gender Raise 34 52 30 42 36 36 32 32 49 30 41 52 30 32 32 44 27 31 32 44 43 48 36 30 41 22 35 44 52 45 29 25 35 26 23 27 22 45 27 24 25 32 42 45 36 39 37 34 41 38 85 80 75 100 90 70 100 90 100 80 100 95 100 90 80 90 55 80 85 70 95 65 65 75 70 95 80 95 95 90 60 95 90 80 90 75 95 95 90 90 80 100 95 90 95 75 95 90 95 80 8 7 5 16 16 12 8 9 10 7 19 22 2 12 8 4 3 11 1 16 13 6 6 9 4 2 7 9 5 18 4 4 9 2 4 3 2 11 6 2 5 8 20 16 8 20 5 11 21 12 0 0 1 0 0 0 1 1 0 1 1 0 1 1 1 0 1 1 0 1 0 1 1 1 0 1 0 1 0 0 1 0 0 0 1 1 1 0 1 0 0 1 1 0 1 0 0 1 0 0 5.7 3.9 3.6 5.5 5.7 4.5 5.7 5.8 4 4.7 4.8 4.5 4.7 6 4.9 5.7 3 5.6 4.6 4.8 6.3 3.8 3.3 3.8 4 6.2 3.9 4.4 5.4 4.3 3.9 5.6 5.5 4.9 5.3 4.3 6.2 4.5 5.5 6.3 4.3 5.7 5.5 5.2 5.2 3.9 5.5 5.3 6.6 4.6 Degree Gender1 0 0 1 1 1 1 1 1 1 1 1 0 0 1 1 0 1 0 1 0 1 1 0 0 0 0 1 0 0 0 1 0 1 1 0 0 0 0 0 0 0 1 0 1 1 1 1 1 0 0 M M F M M M F F M F F M F F F M F F M F M F F F M F M F M M F M M M F F F M F M M F F M F M M F M M Gr E B B E D F C A F A A E C A A C E B A B F D A D A A C F F D A B E B A A A E B A C A F E D E E E E E The ongoing question that the weekly assignm Note: to simplfy the analysis, we will assume The column labels in the table mean: ID - Employee sample numberSalary - Sa Age - Age in years Performan Service - Years of service (rou Gender - 0 Midpoint - salary grade midpoin Raise - pe Grade - job/pay grade Degree (0= Gender1 (Male or Female) Compa - sa ments will focus on is: Are males and females paid the same for equal work (under the Equal Pay Act)? e that jobs within each grade comprise equal work. Salary in thousands nce Rating - Appraisal rating (employee evaluation score) 0 = male, 1 = female ercent of last raise = BS\\BA 1 = MS) salary divided by midpoint Week 2 1 Testing means - T-tests In questions 2, 3, and 4 be sure to include the null and alternate hypotheses you will be testing. In the first 4 questions use alpha = 0.05 in making your decisions on rejecting or not rejecting the null hypothesis. Below are 2 one-sample t-tests comparing male and female average salaries to the overall sample mean. (Note: a one-sample t-test in Excel can be performed by selecting the 2-sample unequal variance t-test and making the second variable = Ho value - a Note: These values are not the same as the data the assignment uses. The purpose is to analyze the results of t-tests rather than directly answer our eq Based on these results, how do you interpret the results and what do these results suggest about the population means for male and female average sal Males Ho: Mean salary = Ha: Mean salary =/= Females Ho: Mean salary = Ha: Mean salary =/= 45.00 45.00 45.00 45.00 Note: While the results both below are actually from Excel's t-Test: Two-Sample Assuming Unequal Variances, having no variance in the Ho variable makes the calculations default to the one-sample t-test outcome - we are tricking Excel into doing a one sample Male Ho Female Ho Mean 52 45 Mean 38 45 Variance 316 0 Variance 334.66667 0 Observations 25 25 Observations 25 25 Hypothesized Mean D 0 Hypothesized Mean D 0 df 24 df 24 t Stat 1.9689038266 t Stat -1.913206 P(T<=t) one-tail 0.0303078503 P(T<=t) one-tail 0.0338621 t Critical one-tail 1.7108820799 t Critical one-tail 1.7108821 P(T<=t) two-tail 0.0606157006 P(T<=t) two-tail 0.0677242 t Critical two-tail 2.0638985616 Conclusion: Do not reject Ho; mean equals 45 t Critical two-tail 2.0638986 Conclusion: Do not reject Ho; mean equals 45 Note: the Female results are done for you, please complete the male results. Is this a 1 or 2 tail test? Is this a 1 or 2 tail test? 2 tail 2 tail - why? - why? Ho contains = P-value is: Is P-value < 0.05 (one tail test) or 0.025 (two tail test)? 0.0606157006 P-value is: 0.0677242 No Is P-value < 0.05 (one tail test) or 0.025 (two tail test)? No Why do we not reject the null hypothesis? Pvalue Why do we not reject the null hypothesis? P-value is > 0.05 greater than (>) rejection alpha Interpretation of test outcomes: 2 Based on our sample data set, perform a 2-sample t-test to see if the population male and female average salaries could be equal to each other. (Since we have not yet covered testing for variance equality, assume the data sets have statistically equal variances.) Ho: Ha: Test to use: Male salary mean = Female salary mean Male salary mean =/= Female salary mean t-Test: Two-Sample Assuming Equal Variances t-Test: Two-Sample Assuming Equal Variances Variable 1 Mean Variable 2 51.832 Variance 38.212 313.233933333 343.436933 Observations Pooled Variance 25 25 328.335433333 Hypothesized Mean Dif 0 df 48 t Stat 2.6574997806 P(T<=t) one-tail 0.005329795 t Critical one-tail 1.6772241966 P(T<=t) two-tail 0.01065959 t Critical two-tail 2.0106347219 P-value is: 0.01065959 Is P-value < 0.05 (one tail test) or 0.025 (two tail test)? yes Reject or do not reject Ho: Reject Ho If the null hypothesis was rejected, calculate the effect size value: 0.5314999561 If calculated, what is the meaning of effect size measure: large Interpretation: There is significant associationg between salary and gender b. Is the one or two sample t-test the proper/correct apporach to comparing salary equality? Why? Yes. The sample size is small and the sample is taken from normal population and population standard deviation is not known 3 Based on our sample data set, can the male and female compas in the population be equal to each other? (Another 2-sample t-test.) Again, please assume equal variances for these groups. Ho: Male compas mean = female compas mean Ha: male compas mean =/= female compas mean Statistical test to use: t-Test: Two-Sample Assuming Equal Variances Variable 1 Mean Variable 2 1.05304 Variance 1.0734 0.0076257067 0.00575142 Observations 25 Pooled Variance 25 0.0066885617 Hypothesized Mean Dif 0 df 48 t Stat -0.8801696972 P(T<=t) one-tail 0.1915765138 t Critical one-tail 1.6772241966 P(T<=t) two-tail 0.3831530276 t Critical two-tail 2.0106347219 What is the p-value: 0.383 Is P-value < 0.05 (one tail test) or 0.025 (two tail test)? No Reject or do not reject Ho: Do not reject If the null hypothesis was rejected, calculate the effect size value: If calculated, what is the meaning of effect size measure: - Interpretation: There is no effect size 4 Since performance is often a factor in pay levels, is the average Performance Rating the same for both genders? NOTE: do NOT assume variances are equal in this situation. Ho: Males performance rating mean = femlae performance rating mean Ha: Males performance rating mean =/= femlae performance rating mean Test to ust-Test: Two-Sample Assuming Unequal Variances t-Test: Two-Sample Assuming Unequal Variances Variable 1 Mean Hypothesized Mean Dif 184.75 25 Observations 84.2 75.25 Variance df Variable 2 87.6 25 0 41 t Stat 1.054295244 P(T<=t) one-tail 0.1489606745 t Critical one-tail 1.6828780026 P(T<=t) two-tail 0.297921349 t Critical two-tail 2.0195409483 What is the p-value: 0.29792 Is P-value < 0.05 (one tail test) or 0.025 (two tail test)? No Do we REJ or Not reject the null? Do not reject If the null hypothesis was rejected, calculate the effect size value: If calculated, what is the meaning of effect size measure: - Interpretation: - 5 If the salary and compa mean tests in questions 2 and 3 provide different results about male and female salary equality, which would be more appropriate to use in answering the question about salary equity? Why? What are your conclusions about equal pay at this point? Salary becoause there is large association between salary and gender lue - a constant.) our equal pay question. age salaries? sample test for us. Week 3 Paired T-test and ANOVA For this week's work, again be sure to state the null and alternate hypotheses and use alpha = 0.05 for our decision value in the reject or do not reject decision on the null hypothesis. Salary Midpoint diff 23 0.6 22.6 23 -0.4 23.4 23 0.4 22.9 23 -0.1 24.9 23 1.9 22.5 23 -0.5 23 23 0 24 23 1 22.6 23 -0.4 23.4 23 0.4 23.3 23 0.3 22.7 23 -0.3 34.7 31 3.7 36.3 31 5.3 41.76 34.3 31 3.3 368.95726122 263.45143 36.1 31 5.1 42 40 2 Pearson Correlati 0.9889305701 41.3 40 1.3 Hypothesized Mea 1 23.6 0 57.3 48 9.3 Many companies consider the grade midpoint to be the "market rate" - the salary needed to hire a new employee. Does the company, on average, pay its existing employees at or above the market rate? Use the data columns at the right to set up the paired data set for the analysis. Null Hypothesis: Salaries are < = to market rate dbar< =0 Alt. Hypothesis: Salaries are > market rate dbar>0 Statistical test to use: t-Test: Paired Two Sample for Means Salary Mean Midpoint 45.022 Variance Observations 50 df 50 49 54.7 48 6.7 t Stat 5.8092273485 51 48 3 P(T<=t) one-tail 2.29930E-007 66.5 57 9.5 t Critical one-tail 1.6765508931 69.6 57 12.6 P(T<=t) two-tail 4.59860E-007 75.4 67 8.4 t Critical two-tail 2.0095751993 77.2 67 10.2 24.5 23 1.5 24.5 23 1.5 24.2 23 1.2 26.8 31 -4.2 26.9 31 -4.1 26.5 31 -4.5 46.6 40 6.6 39.4 40 -0.6 45.2 40 5.2 48.5 48 0.5 46 48 -2 60 57 3 57.9 57 0.9 61.7 57 4.7 59.8 57 2.8 What is the p-value: 2.30E-07 Is P-value < 0.05 (one tail test) or 0.025 (two tail test)? Yes What else needs to be checked on a 1-tail test in t stat > tcritical for one taileed test order to reject the null? Do we REJ or Not reject the null? If the null hypothesis was rejected, what is the REJ effect effect size meaning of size value: 0.821549 measure: Effect size is large. There is storng association between salaries and midpoint Interpretation of test results: The mean salaries are greater than market rate 65.4 Last week, we found that average performance ratings do not differ between males and females in the population. Now we need to see if they differ among the grades. Is the average performace rating the same for all grades? (Assume variances are equal across the grades for this ANOVA.) Here are the data values sorted by grade level. The rating values sorted by grade have been placed in columns I - N for you. A B C D E 90 80 100 90 85 Null Hypothesis: Ho: means equal for all grades 80 75 100 65 100 Alt. Hypothesis: Ha: at least one mean is unequal 100 80 90 75 95 Place B17 in Outcome range box. 90 70 80 90 55 Anova: Single Factor 80 95 80 95 90 85 80 95 SUMMARY 65 90 90 Groups Count Sum Average Variance 70 75 A 15 1265 84.3333333 153.0952381 95 95 B 7 570 81.4285714 72.61904762 60 90 C 5 450 90 100 90 95 D 5 415 83 157.5 75 80 E 12 1045 87.0833333 152.0833333 95 F 6 550 91.6666667 116.6666667 90 100 ANOVA Source of Variation SS Between Groups 519.20238095 Within Groups Total 5865.297619 6384.5 df MS F P-value F crit 5 103.840476 0.778985356 0.570215477 2.427040114 44 133.302219 49 Interpretation of test results: What is the p-value: 0.57 If the ANVOA was done correctly, this is the p-value shown. Is P-value < 0.05? No Do we REJ or Not reject the null? Not Reject 6.2 57 6.7 62.9 57 5.9 63.5 57 6.5 61.4 F 70 100 95 95 95 95 8.4 57 63.7 2 57 63.2 Let's look at some other factors that might influence pay - education(degree) and performance ratings. 57 4.4 74.3 67 7.3 74.2 67 7.2 76.7 67 9.7 72 67 5 Mean 3.262 Stdev 3.9705492 If the null hypothesis was rejected, what is the effect size value (eta squared): 0.08132232 Meaning of effect size measure: Effect size is small There is no association between grades and performance rating What does that decision mean in terms of our equal pay question: The performance rating is sam for all the grades 3 While it appears that average salaries per each grade differ, we need to test this assumption. Is the average salary the same for each of the grade levels? Use the input table to the right to list salaries under each grade level. (Assume equal variance, and use the analysis toolpak function ANOVA.) Null Hypothesis: The mean salaries are same for all grades If desired, place salaries per grade in these columns A B C D E Alt. Hypothesis: Atleast one mean is different from others 24.5 26.8 46.6 48.5 60 24.5 26.9 39.4 46 57.9 Place B51 in Outcome range box. 24.2 26.5 45.2 57.3 61.7 Anova: Single Factor 23.6 34.7 42 54.7 59.8 22.6 36.3 41.3 51 65.4 SUMMARY 23.4 34.3 63.2 Groups Count Sum Average Variance 22.9 36.1 63.7 A 15 352.1 23.4733333 0.616380952 24.9 62.9 B 7 221.6 31.6571429 21.72619048 22.5 63.5 C 5 214.5 42.9 8.65 23 61.4 D 5 257.5 51.5 20.845 24 66.5 E 12 755.6 62.9666667 10.16787879 22.6 69.6 F 6 449.8 74.9666667 3.602666667 23.4 50 23.3 22.7 ANOVA Source of Variation SS Between Groups 17692.079324 Within Groups Total 386.82647619 18078.9058 df MS F P-value F 74.3 74.2 76.7 72 75.4 77.2 F crit 5 3538.41586 402.480977 1.5137E-035 2.427040114 44 8.79151082 49 Note: Sometimes we see a p-value in the format of 3.4E-5; this means move the decimal point left 5 places. In this example, the p-value is 0.000034 What is the p-value: 1.514E-035 Is P-value < 0.05? Yes Do we REJ or Not reject the null? REJ If the null hypothesis was rejected, calculate the effect size value (eta squared): 0.97860344 If calculated, what is the meaning of effect size measure: Very large. The salaries are dependent on grades Interpretation: The mean salary of atleat one grade is different from others 4 The table and analysis below demonstrate a 2-way ANOVA with replication. Please interpret the results. Note: These values are not the same as the data the assignment uses. The purpose of this question is to analyze the result of a 2-way ANOVA test rather than directly answer our equal pay question. BA MA Ho: Average compas by gender are equal Male 1.017 1.157 Ha: Average compas by gender are not equal 0.870 0.979 Ho: Average compas are equal for each degree 1.052 1.134 Ha: Average compas are not equal for each degree 1.175 1.149 Ho: Interaction is not significant 1.043 1.043 Ha: Interaction is significant 1.074 1.134 1.020 1.000 Perform analysis: 0.903 1.122 0.982 0.903 Anova: Two-Factor With Replication 1.086 1.052 1.075 1.140 SUMMARY BA MA Total 1.052 1.087 Male 1.096 1.050 Female Count 12 12 24 1.025 1.161 Sum 12.349 12.9 25.249 1.000 1.096 Average 1.029083333 1.075 1.0520417 0.956 1.000 Variance 0.006686447 0.006519818 0.006866 1.000 1.041 1.043 1.043 Female 1.043 1.119 Count 12 12 24 1.210 1.043 Sum 12.791 12.787 25.578 1.187 1.000 Average 1.065916667 1.065583333 1.06575 1.043 0.956 Variance 0.006102447 0.004212811 0.0049334 1.043 1.129 1.145 1.149 Total Count Sum 24 24 25.14 25.687 Average 1.0475 1.070291667 Variance 0.006470348 0.005156129 ANOVA Source of Variation SS Sample 0.002255021 Columns 0.006233521 (This is the row variable or gender.) 1 0.0062335 1.060054 0.3088296 4.0617065 (This is the column variable or Degree.) Interaction 0.006417188 1 0.0064172 1.0912878 0.3018915 4.0617065 Within Total 0.25873675 0.273642479 df MS F P-value F crit 1 0.002255 0.3834821 0.538939 4.0617065 44 0.0058804 47 Interpretation: For Ho: Average compas by gender are equal Ha: Average compas by gender are not equal What is the p-value: 0.53893895 Is P-value < 0.05? No Do you reject or not reject the null hypothesis:Do not Reject If the null hypothesis was rejected, what is the effect size value (eta squared): 0.00824076 Meaning of effect size measure: Very small For Ho: Average compas are equal for all degrees Ha: Average compas are not equal for all grades What is the p-value: 0.30882956 Is P-value < 0.05? No Do you reject or not reject the null hypothesis:Do not Reject If the null hypothesis was rejected, what is the effect size value (eta squared): 0.0227798 Meaning of effect size measure: Small For: Ho: Interaction is not significant Ha: Interaction is significant What is the p-value: 0.30189151 Is P-value < 0.05? No Do you reject or not reject the null hypothesis:Do not Reject If the null hypothesis was rejected, what is the effect size value (eta squared): 0.02345099 Meaning of effect size measure: Small What do these three decisions mean in terms of our equal pay question: The mean compas are equal across differetn grades and gender Place data values in these columns 5. Using the results up thru this week, what are your conclusions about gender equal pay for equal work at this point? t-Test: Two-Sample Assuming Equal Variances Female Mean Variance Male 38.212 51.832 343.43693333 313.23393 Observations 25 Null hypothesis: Mean salaries for males and females are equal Alternate hypothesis : Mean salaries for males and females are not equal 25 Pooled Variance 328.33543333 pvalue < 0.05 Hypothesized Mea We reject Ho df t Stat P(T<=t) one-tail 0 48 -2.657499781 0.005329795 t Critical one-tail 1.6772241966 P(T<=t) two-tail 0.01065959 t Critical two-tail 2.0106347219 Conclusions Performance rating is independent of gender and grades Salaries differ for grades and gender Female 23.6 22.6 23.4 22.9 24.9 22.5 23 24 22.6 23.4 23.3 22.7 34.7 36.3 34.3 36.1 42 41.3 57.3 54.7 51 66.5 69.6 75.4 77.2 Male 24.5 24.5 24.2 26.8 26.9 26.5 46.6 39.4 45.2 48.5 46 60 57.9 61.7 59.8 65.4 63.2 63.7 62.9 63.5 61.4 74.3 74.2 76.7 72 Dif Week 4 Confidence Intervals and Chi Square (Chs 11 - 12) For questions 3 and 4 below, be sure to list the null and alternate hypothesis statements. Use .05 for your significance level in making your decisions. For full credit, you need to also show the statistical outcomes - either the Excel test result or the calculations you performed. 1 Using our sample data, construct a 95% confidence interval for the population's mean salary for each gender. Interpret the results. Mean St error t value Low to High Males Females Interpretation: 2 Using our sample data, construct a 95% confidence interval for the mean salary difference between the genders in the population. How does this compare to the findings in week 2, question 2? Difference St Err. T value Low to High Yes/No Can the means be equal? Why? How does this compare to the week 2, question 2 result (2 sampe t-testResults are the same - means are not equal. a. Why is using a two sample tool (t-test, confidence interval) a better choice than using 2 one-sample techniques when comparing two samples? 3 We found last week that the degree values within the population do not impact compa rates. This does not mean that degrees are distributed evenly across the grades and genders. Do males and females have athe same distribution of degrees by grade? (Note: while technically the sample size might not be large enough to perform this test, ignore this limitation for this exercise.) Ignore any cell size limitations. What are the hypothesis statements: Ho: Ha: Note: You can either use the Excel Chi-related functions or do the calculations manually. Data InTables The Observed Table is completed for you. OBSERVED A B C D E F Total If desired, you can do manual calculations per cell here. M Grad 1 1 1 1 5 3 12 A B C D E F Fem Grad M Grad 5 3 1 1 1 2 13 Male Und Fem Grad 2 2 2 1 5 1 13 Female Und Male Und 7 1 1 2 1 0 12 Female Und 15 7 5 5 12 6 50 Sum = EXPECTED M Grad Fem Grad Male Und Female Und For this exercise - ignore the requirement for a correction for expected values less than 5. Interpretation: What is the value of the chi square statistic: What is the p-value associated with this value: Is the p-value <0.05? Do you reject or not reject the null hypothesis: If you rejected the null, what is the Cramer's V correlation: What does this correlation mean? What does this decision mean for our equal pay question: 4 Based on our sample data, can we conclude that males and females are distributed across grades in a similar pattern within the population? Again, ignore any cell size limitations. What are the hypothesis statements: Ho: Ha: A OBS COUNT - m OBS COUNT - f B C D E Do manual calculations per cell here (if desired) A B C D E F M F F Sum = EXPECTED What is the value of the chi square statistic: What is the p-value associated with this value: Is the p-value <0.05? Do you reject or not reject the null hypothesis: If you rejected the null, what is the Phi correlation: If calculated, what is the meaning of effect size measure: What does this decision mean for our equal pay question: 5. How do you interpret these results in light of our question about equal pay for equal work? Week 5 Correlation and Regression 1. Create a correlation table for the variables in our data set. (Use analysis ToolPak or StatPlus:mac LE function Correlation.) a. Reviewing the data levels from week 1, what variables can be used in a Pearson's Correlation table (which is what Excel produces)? b. Place table here (C8): c. d. Looking at the above correlations - both significant or not - are there any surprises -by that I mean any relationships you expected to be meaningful and are not and vice-versa? e. 2 Using r = approximately .28 as the signicant r value (at p = 0.05) for a correlation between 50 values, what variables are significantly related to Salary? To compa? Does this help us answer our equal pay for equal work question? Below is a regression analysis for salary being predicted/explained by the other variables in our sample (Midpoint, age, performance rating, service, gender, and degree variables. (Note: since salary and compa are different ways of expressing an employee's salary, we do not want to have both used in the same regression.) Plase interpret the findings. Note: These values are not the same as the data the assignment uses. The purpose is to analyze the result of a regression test rather than directly answer our equal pay question. Ho: The regression equation is not significant. Ha: The regression equation is significant. Ho: The regression coefficient for each variable is not significant Note: technically we have one for each input variable. Ha: The regression coefficient for each variable is significant Listing it this way to save space. Sal SUMMARY OUTPUT Regression Statistics Multiple R 0.99155907 R Square 0.9831894 Adjusted R Square 0.98084373 Standard Error 2.65759257 Observations 50 ANOVA df Regression Residual Total SS MS F Significance F 6 17762.3 2960.383 419.15161 1.812E-036 43 303.70033 7.062798 49 18066 Standard Coefficients Error t Stat P-value Lower 95% Upper 95% Lower 95.0% Upper 95.0% Intercept -1.74962121 3.6183677 -0.483539 0.6311665 -9.046755 5.54751262 -9.0467550427 5.547512618 Midpoint 1.21670105 0.0319024 38.13829 8.66E-035 1.15236383 1.28103827 1.1523638283 1.2810382727 Age -0.00462801 0.0651972 -0.070985 0.943739 -0.1361107 0.1268547 -0.1361107191 0.1268546987 Performace Rating -0.05659644 0.0344951 -1.640711 0.1081532 -0.1261624 0.01296949 -0.1261623747 0.0129694936 Service -0.04250036 0.084337 -0.503935 0.6168794 -0.2125821 0.12758138 -0.2125820912 0.1275813765 Gender 2.420337212 0.8608443 2.811585 0.0073966 0.68427919 4.15639523 0.684279192 4.156395232 Degree 0.27553341 0.7998023 0.344502 0.7321481 -1.3374217 1.88848848 -1.3374216547 1.8884884833 Note: since Gender and Degree are expressed as 0 and 1, they are considered dummy variables and can be used in a multiple regression equation. Interpretation: For the Regression as a whole: What is the value of the F statistic: What is the p-value associated with this value: Is the p-value <0.05? Do you reject or not reject the null hypothesis: What does this decision mean for our equal pay question: For each of the coefficients: Intercept What is the coefficient's p-value for each of the variables: NA Is the p-value < 0.05? NA Do you reject or not reject each null hypothesis: NA What are the coefficients for the significant variables? Midpoint Age Perf. Rat. Service Gender Degree Note: Thes Using the intercept coefficient and only the significant variables, what is the equation? Is gender a significant factor in salary: If so, who gets paid more with all other things being equal? How do we know? 3 Salary = Perform a regression analysis using compa as the dependent variable and the same independent variables as used in question 2. Show the result, and interpret your findings by answering the same questions. Note: be sure to include the appropriate hypothesis statements. Regression hypotheses Ho: Ha: Coefficient hyhpotheses (one to stand for all the separate variables) Ho: Ha: Place c94 in output box. Interpretation: For the Regression as a whole: What is the value of the F statistic: What is the p-value associated with this value: Is the p-value < 0.05? Do you reject or not reject the null hypothesis: What does this decision mean for our equal pay question: For each of the coefficients: Intercept What is the coefficient's p-value for each of the variables: NA Is the p-value < 0.05? NA Do you reject or not reject each null hypothesis: NA What are the coefficients for the significant variables? Midpoint Age Perf. Rat. Service Gender Degree Using the intercept coefficient and only the significant variables, what is the equation? Compa = Is gender a significant factor in compa: Regardless of statistical significance, who gets paid more with all other things being equal? How do we know? 4 Based on all of your results to date, Do we have an answer to the question of are males and females paid equally for equal work? Does the company pay employees equally for for equal work? How do we know? Which is the best variable to use in analyzing pay practices - salary or compa? Why? What is most interesting or surprising about the results we got doing the analysis during the last 5 weeks? 5 Why did the single factor tests and analysis (such as t and single factor ANOVA tests on salary equality) not provide a complete answer to our salary equality question? What outcomes in your life or work might benefit from a multiple regression examination rather than a simpler one variable test? se values are not the same as in the data the assignment uses. The purpose is to analyze the result of a 2-way ANOVA test rather than directly answer our equal pay
Step by Step Solution
There are 3 Steps involved in it
Step: 1
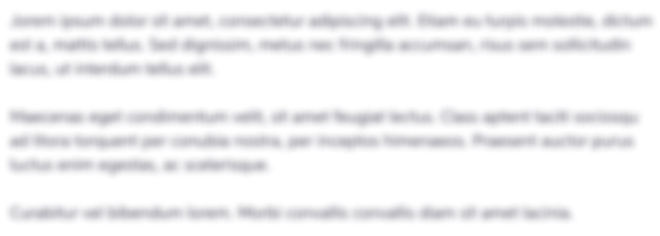
Get Instant Access to Expert-Tailored Solutions
See step-by-step solutions with expert insights and AI powered tools for academic success
Step: 2

Step: 3

Ace Your Homework with AI
Get the answers you need in no time with our AI-driven, step-by-step assistance
Get Started