Answered step by step
Verified Expert Solution
Question
00
1 Approved Answer
Ill be needing the folloiwng done A description of the significance of your data science case study in the chosen domain; A section that highlights
Ill be needing the folloiwng done A description of the significance of your data science case study in the chosen domain; A section that highlights the aim and objectives of the case study; Here is my case study so far please tell provide me with a dataset that: The selected dataset is significant to the domain and a detailed description of the dataset is included. Note: Your chosen dataset should be compact with no fewer than variables and no more than variables. You will be using the same dataset in Assessment This assessment serves as the planning document for Assessment Suggest you read the Assessment and resources in the website Exploratory Data Analysis Tutorial in Python, before selecting the dataset. Recommend you choose the dataset in csv format. The datasets used in this website are excluded to be used for both Assessments and Here is my case study so far Abstract Within a month after a patient is discharged it's crucial to predict whether they might need to be readmitted a concern, in healthcare management. In this case study, an analysis of hospital records using regression is conducted to identify the main factors influencing readmission rates. The goal is to create a model that can help healthcare providers intervene early leading to readmission rates and better patient outcomes. Additionally ethical considerations, like safeguarding patient privacy and data are discussed. This research holds significance as it has the potential to enhance healthcare effectiveness and patient care through data driven insights. Introduction Data science has developed into an essential component of contemporary medical treatment, providing very effective tools for the examination of extensive datasets and the extraction of insightful conclusions. In this particular case study, the issue of patient readmissions within thirty days after release is the subject of discussion. This is a prevalent and expensive problem in hospitals. Because they are able to determine which patients are most likely to need readmission, healthcare practitioners are able to more efficiently allocate resources and put preventative measures into practice. The purpose of this research is to construct a prediction model by using logistic regression, which will provide a solution that is driven by data to enhance the results of healthcare. One of the many issues that the healthcare sector must contend with is the high incidence of patients who need readmission back to the hospital. Not only do these readmissions put a drain on the resources of the hospital, but they also bring to light significant deficiencies in patient care. For the purpose of establishing solutions to address readmissions, it is helpful to have an understanding of the causes that contribute to readmissions. A method that may be used to anticipate readmissions and allow proactive healthcare management is provided by data science, which is characterised by its sophisticated analytical tools. Problem Definition Within the first thirty days after being discharged from the hospital, the prediction of patient readmissions is the key issue that is addressed in this case study. The early identification of patients who are at risk may assist healthcare practitioners in increasing the efficiency with which they allocate resources and in putting preventative measures into practice. We hope that by establishing a predictive model, we will be able to improve patient care, lower the number of readmissions, and maximise the efficiency of hospital operations. Significance The significance of this research lies in the fact that it tackles a fundamental problem in the administration of healthcare. The ability to anticipate readmissions may result in improved resource allocation, greater patient monitoring, and enhancements to the overall quality of healthcare outcomes. It is possible for healthcare practitioners to establish targeted treatments by understanding the variables that lead to readmissions. This will eventually result in better patient satisfaction and a reduction in the expenditures associated with healthcare. Aims and Objectives Aims: A predictive model for patient readmissions within thirty days after release is the primary objective of this case study, which aims to construct such a model. For the purpose of preventing readmissions, this model will assist healthcare practitioners in identifying patients who are at a high risk and in taking preventative measures. Objectives: Identify the key predictors of patient readmissions. Apply logistic regression to develop the predictive model. Validate the model using appropriate metrics such as accuracy, precision, and recall. Provide actionable insights to healthcare providers based on the model's predictions. Dataset For the purpose of this case study, the information that was obtained from a public healthcare database that contained hospital discharge records was utilised. Patient demographics such as age and gender medical history including past diseases and medications discharge specifications such as the reason for discharge and the length of stay and followup data are some of the characteristics that are covered. Eight variables make up the dataset, which is not only simple to manage but also highly pertinent to the investigation that is now being conducted. Throughout the entirety of the research, the dataset will be utilised on a consistent basis for Assessment This particular dataset was selected because it provides a thorough coverage of patient histories and outcomes, which makes it an excellent candidate for the development of a prediction model. A thorough screening process was employed to guarantee that the variables, in the dataset accurately depict the factors influencing readmissions. To ensure the studys precision and dependability the dataset undergoes a cleaning and preprocessing method. This approach is crafted to rectify any instances of conflicting data that might have existed. Analytics Approach In the course of this investigation, the most important statistical method that was utilised was logistic regression. Logistic regression is an approach that works well for issues that include binary classification, such as determining whether or not a patient will be readmitted within the next thirty days. We are able to estimate the chance of readmission using this strategy, which takes into account a number of different predictor variables. The following is a list of the steps that are involved in the analytics approach: Data Preprocessing: The dataset is being cleaned up in order to address missing values, outliers, and inconsistencies respectively. The data will be suitable for analysis as a result of this decision. Feature Selection: Finding the most important predictor variables that are responsible for readmissions is a focus of this study. Both exploratory data analysis and statistical tests are utilised in order to accomplish this goal. Model Development: The development of the prediction model by the application of logistic regression. It is necessary to train the model on a subset of the dataset and then validate it on the remaining data in order to guarantee that it is accurate and reliable. Model Evaluation: For the purpose of assessing the performance of the model, measurements such as accuracy, precision, recall, and the Area Under the Receiver Operating Characteristic Curve AUCROC are utilised. Interpretation and Insights: The data are being analysed in order to give healthcare practitioners with insights that can be put into action. This includes determining the most important variables and the influence those predictors have on readmissions. Ethical Issues When it comes to managing healthcare data ethical considerations play a role. In our studies we take steps to ensure that patient data is anonymized to safeguard their privacy rights. We strictly follow healthcare laws, like HIPAA at every stage of processing and analysing data. Moreover we evaluate the model to detect any biases and ensure that its predictions remain fair and unbiased, across all groups. Determining if a patient might need to return to the hospital within a month after being discharged is an aspect of healthcare management. This research project focuses on identifying the factors that impact readmission rates through analysing hospital data using regression analysis. The goal is to create a model that enables doctors to take measures leading to readmission rates and better outcomes through their interventions. Additionally ethical considerations such, as safeguarding data privacy and maintaining confidentiality are addressed. This study holds significance as it utilises data driven insights that could enhance the quality of patient care and the efficiency of healthcare services.
Ill be needing the folloiwng done
A description of the significance of your data science case study in the chosen domain;
A section that highlights the aim and objectives of the case study;
Here is my case study so far please tell provide me with a dataset that:
The selected dataset is significant to the domain and a detailed description of the dataset is included.
Note: Your chosen dataset should be compact with no fewer than variables and no more than
variables. You will be using the same dataset in Assessment This assessment serves as the planning
document for Assessment Suggest you read the Assessment and resources in the website
Exploratory Data Analysis Tutorial in Python, before selecting the dataset. Recommend you
choose the dataset in csv format. The datasets used in this website are excluded to be used for both
Assessments and
Here is my case study so far
Abstract
Within a month after a patient is discharged it's crucial to predict whether they might need to be readmitted a concern, in healthcare management. In this case study, an analysis of hospital records using regression is conducted to identify the main factors influencing readmission rates. The goal is to create a model that can help healthcare providers intervene early leading to readmission rates and better patient outcomes. Additionally ethical considerations, like safeguarding patient privacy and data are discussed. This research holds significance as it has the potential to enhance healthcare effectiveness and patient care through data driven insights.
Introduction
Data science has developed into an essential component of contemporary medical treatment, providing very effective tools for the examination of extensive datasets and the extraction of insightful conclusions. In this particular case study, the issue of patient readmissions within thirty days after release is the subject of discussion. This is a prevalent and expensive problem in hospitals. Because they are able to determine which patients are most likely to need readmission, healthcare practitioners are able to more efficiently allocate resources and put preventative measures into practice. The purpose of this research is to construct a prediction model by using logistic regression, which will provide a solution that is driven by data to enhance the results of healthcare.
One of the many issues that the healthcare sector must contend with is the high incidence of patients who need readmission back to the hospital. Not only do these readmissions put a drain on the resources of the hospital, but they also bring to light significant deficiencies in patient care. For the purpose of establishing solutions to address readmissions, it is helpful to have an understanding of the causes that contribute to readmissions. A method that may be used to anticipate readmissions and allow proactive healthcare management is provided by data science, which is characterised by its sophisticated analytical tools.
Problem Definition
Within the first thirty days after being discharged from the hospital, the prediction of patient readmissions is the key issue that is addressed in this case study. The early identification of patients who are at risk may assist healthcare practitioners in increasing the efficiency with which they allocate resources and in putting preventative measures into practice. We hope that by establishing a predictive model, we will be able to improve patient care, lower the number of readmissions, and maximise the efficiency of hospital operations.
Significance
The significance of this research lies in the fact that it tackles a fundamental problem in the administration of healthcare. The ability to anticipate readmissions may result in improved resource allocation, greater patient monitoring, and enhancements to the overall quality of healthcare outcomes. It is possible for healthcare practitioners to establish targeted treatments by understanding the variables that lead to readmissions. This will eventually result in better patient satisfaction and a reduction in the expenditures associated with healthcare.
Aims and Objectives
Aims: A predictive model for patient readmissions within thirty days after release is the primary objective of this case study, which aims to construct such a model. For the purpose of preventing readmissions, this model will assist healthcare practitioners in identifying patients who are at a high risk and in taking preventative measures.
Objectives:
Identify the key predictors of patient readmissions.
Apply logistic regression to develop the predictive model.
Validate the model using appropriate metrics such as accuracy, precision, and recall.
Provide actionable insights to healthcare providers based on the model's predictions.
Dataset
For the purpose of this case study, the information that was obtained from a public healthcare database that contained hospital discharge records was utilised. Patient demographics such as age and gender medical history including past diseases and medications discharge specifications such as the reason for discharge and the length of stay and followup data are some of the characteristics that are covered. Eight variables make up the dataset, which is not only simple to manage but also highly pertinent to the investigation that is now being conducted. Throughout the entirety of the research, the dataset will be utilised on a consistent basis for Assessment
This particular dataset was selected because it provides a thorough coverage of patient histories and outcomes, which makes it an excellent candidate for the development of a prediction model. A thorough screening process was employed to guarantee that the variables, in the dataset accurately depict the factors influencing readmissions. To ensure the studys precision and dependability the dataset undergoes a cleaning and preprocessing method. This approach is crafted to rectify any instances of conflicting data that might have existed.
Analytics Approach
In the course of this investigation, the most important statistical method that was utilised was logistic regression. Logistic regression is an approach that works well for issues that include binary classification, such as determining whether or not a patient will be readmitted within the next thirty days. We are able to estimate the chance of readmission using this strategy, which takes into account a number of different predictor variables.
The following is a list of the steps that are involved in the analytics approach:
Data Preprocessing: The dataset is being cleaned up in order to address missing values, outliers, and inconsistencies respectively. The data will be suitable for analysis as a result of this decision.
Feature Selection: Finding the most important predictor variables that are responsible for readmissions is a focus of this study. Both exploratory data analysis and statistical tests are utilised in order to accomplish this goal.
Model Development: The development of the prediction model by the application of logistic regression. It is necessary to train the model on a subset of the dataset and then validate it on the remaining data in order to guarantee that it is accurate and reliable.
Model Evaluation: For the purpose of assessing the performance of the model, measurements such as accuracy, precision, recall, and the Area Under the Receiver Operating Characteristic Curve AUCROC are utilised.
Interpretation and Insights: The data are being analysed in order to give healthcare practitioners with insights that can be put into action. This includes determining the most important variables and the influence those predictors have on readmissions.
Ethical Issues
When it comes to managing healthcare data ethical considerations play a role. In our studies we take steps to ensure that patient data is anonymized to safeguard their privacy rights. We strictly follow healthcare laws, like HIPAA at every stage of processing and analysing data. Moreover we evaluate the model to detect any biases and ensure that its predictions remain fair and unbiased, across all groups.
Determining if a patient might need to return to the hospital within a month after being discharged is an aspect of healthcare management. This research project focuses on identifying the factors that impact readmission rates through analysing hospital data using regression analysis. The goal is to create a model that enables doctors to take measures leading to readmission rates and better outcomes through their interventions. Additionally ethical considerations such, as safeguarding data privacy and maintaining confidentiality are addressed. This study holds significance as it utilises data driven insights that could enhance the quality of patient care and the efficiency of healthcare services.
Step by Step Solution
There are 3 Steps involved in it
Step: 1
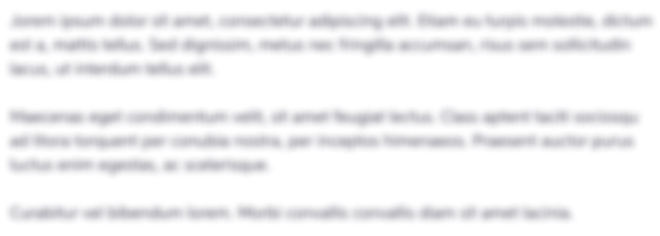
Get Instant Access with AI-Powered Solutions
See step-by-step solutions with expert insights and AI powered tools for academic success
Step: 2

Step: 3

Ace Your Homework with AI
Get the answers you need in no time with our AI-driven, step-by-step assistance
Get Started