Answered step by step
Verified Expert Solution
Question
1 Approved Answer
In the course, you learned about different techniques for dimensionality reductions that embed high dimensional data into a low dimensional space. Explain in at most
In the course, you learned about different techniques for dimensionality reductions that embed high dimensional data into a low dimensional space.
Explain in at most words, how PCA and tSNE approach dimensionality reduction respectively. Name one disadvantage for each of the methods, again using a maximum of words.
Why is it important to normalize the data before applying PCA?
Given a dataset of observations and a column wise mean of zero, the first principal component is the direction of maximum variance in the data, ie
Show that the first principal component also corresponds to the eigenvector with the largest eigenvalue of the covariance matrix of the data.
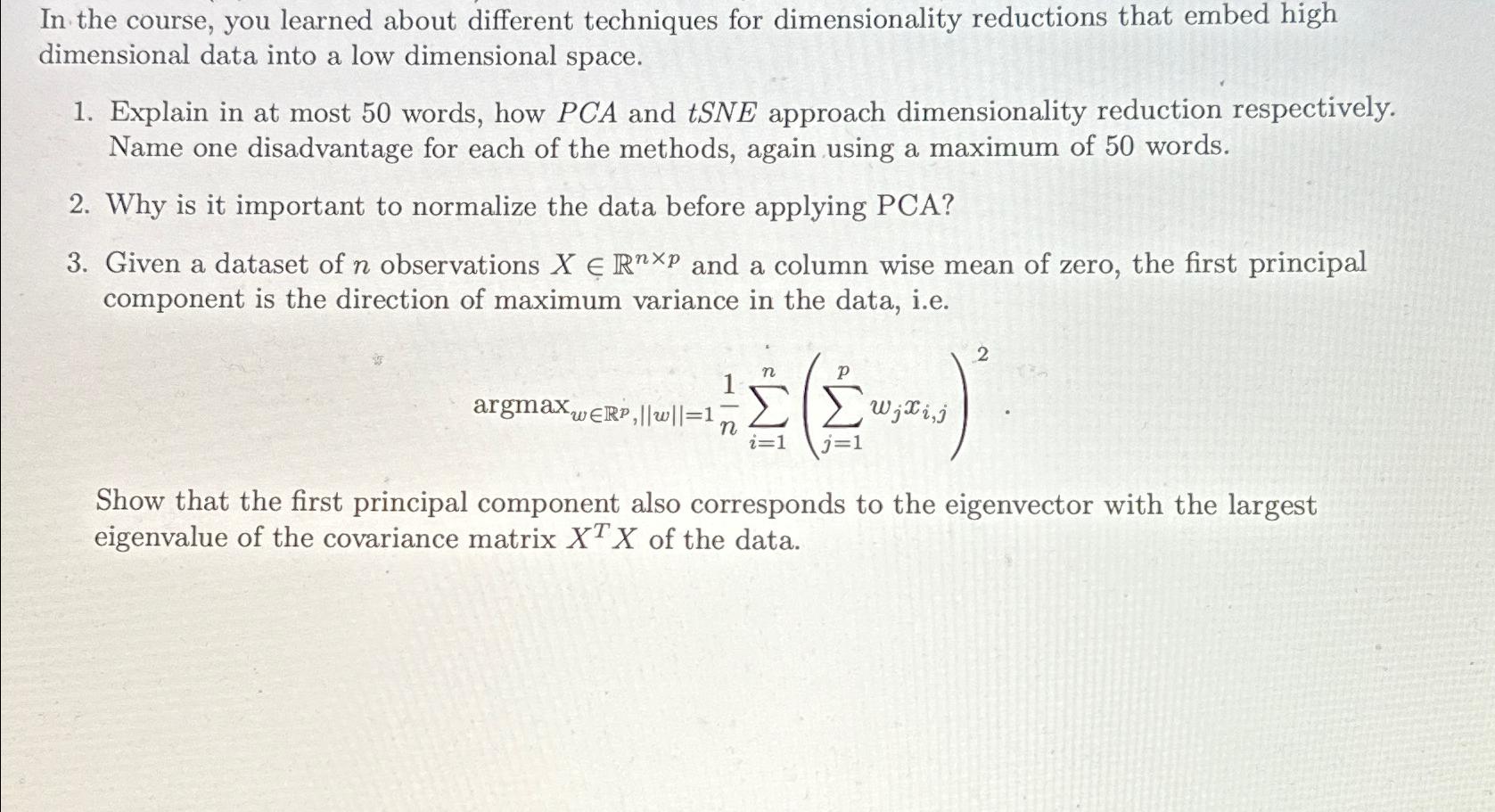
Step by Step Solution
There are 3 Steps involved in it
Step: 1
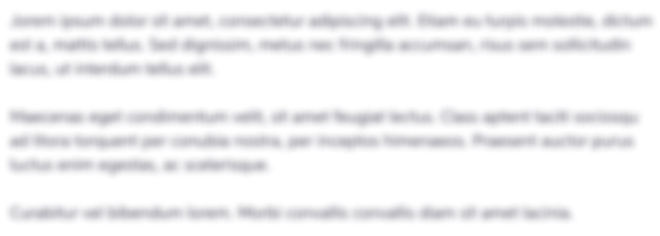
Get Instant Access to Expert-Tailored Solutions
See step-by-step solutions with expert insights and AI powered tools for academic success
Step: 2

Step: 3

Ace Your Homework with AI
Get the answers you need in no time with our AI-driven, step-by-step assistance
Get Started