Answered step by step
Verified Expert Solution
Question
1 Approved Answer
In this question, you will use the scikit-learn package to perform PCA. (1 point) Perform PCA on the data frame df with the parameter as
In this question, you will use the scikit-learn package to perform PCA.
(1 point) Perform PCA on the data frame df with the parameter as n_components = 3,
(1 point) create a figure that includes the first three principal components over time,
(3 points) print out the principal components, explained variances, proportions of explained variances, and cumulative proportions of explained variances. For cumulative proportions, use the .cumsum() function.
from sklearn.decomposition import PCA pca = PCA(????? = ?????) X_pca = pca.?????(df) pc1 = X_pca[?????,?????] pc2 = X_pca[?????,?????] pc3 = X_pca[?????,?????] fig = plt.figure(figsize=(15, 10)) plt.plot(?????, ?????, label='?????') plt.plot(?????, ?????, label='?????') plt.plot(?????, ?????, label='?????') plt.legend(loc="upper right") plt.grid() plt.show() print("Principal Components: ", np.round(pca.?????,2)) print(f"Explained variance: {np.round(pca.?????,2)}") print(f"Explained Variance %: {np.round(pca.?????,2)}") print(f"Cumulative Variance %: {np.round(100*pca.?????.?????(),2)} ") print("Eigenvalues: ", ?????) print("Eigenvectors: ", ?????)
Step by Step Solution
There are 3 Steps involved in it
Step: 1
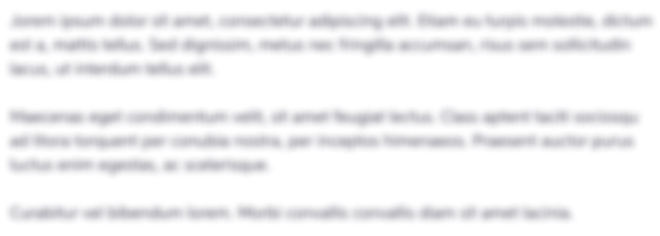
Get Instant Access to Expert-Tailored Solutions
See step-by-step solutions with expert insights and AI powered tools for academic success
Step: 2

Step: 3

Ace Your Homework with AI
Get the answers you need in no time with our AI-driven, step-by-step assistance
Get Started