Question
Instruction: your report (in PDF format) must be professional-looking not exceeding 4 pages, including tables and figures (Times New Roman 12-pt font, single-spaced with 1-inch
Instruction: your report (in PDF format) must be professional-looking not exceeding 4 pages, including tables and figures (Times New Roman 12-pt font, single-spaced with 1-inch margins on all sides). Use of any software other than EXCEL will lead to automatic ZERO for the short Report. Need my feedback? I am more than glad to give you feedback and advice on your preliminary report. This way, you can not only maximize your grade, you may also significantly improve the quality of the report. I can give you a meaningful and useful feedback only after you have made a genuine effort (well ahead of the project due date) to come up with a detailed report. My feedback uses your existing report as a basis. Please seek help early. Please make an appointment via email so that I open a Zoom session to discuss about your preliminary report (first draft).
Background
A recent study found that American consumers are making average monthly debt payments of around $1,000 (Source: Experian.com, November 11, 2010). However, the study of 26 metropolitan areas reveals quite a bit of variation in debt payments, depending on where the consumer lives. For instance, in Washington DC, residents pay the most ($1,285 per month), while Pittsburgh residents pay the least ($763 per month). Madelyn Davis, an economist in a large bank, believes that income differences between cities are the primary reason for the disparate debt payments. For example, Washington DCs high incomes have likely contributed to it placement on the list. She is also unsure about the likely effect of unemployment rate on consumer debt payments.
Statistical Analysis
In order to analyze the relationship between income, unemployment rate, and consumer debt payments, Madelyn collected data from the same 26 metropolitan cities used in the earlier debt payment study. Specifically, she gathered each areas 2010 2011 median household income as well as the monthly unemployment rate and average consumer debt for August 2010. Data is available in CANVAS.
Taking Madelyns role, you (the student) would like to use this sample data to understand the relationships between (1) Debt Payments and Income, and (2) Debt Payments and Unemployment Rate. To that purpose, you will employ data visualization and regression analysis. Conduct the following data analysis details (instructions). Please do not simply follow them. Try to understand why they help address the issue at hand. In your analysis, please use =0.1 when conducting hypothesis testing.
Metropolitan area | Unemployment | Debt | Income | ||||||||
Washington, D.C. | 6.3 | 1,285 | 103500 | ||||||||
Seattle | 8.5 | 1,135 | 81700 | ||||||||
Baltimore | 8.1 | 1,133 | 82200 | ||||||||
Boston | 7.6 | 1,133 | 89500 | ||||||||
Denver | 8.1 | 1,104 | 75900 | Description of Varaibles | |||||||
San Francisco | 9.3 | 1,098 | 93400 | ||||||||
San Diego | 10.6 | 1,076 | 75500 | Unemployment | The unemployment rate in a city (this is in percentage or %) | ||||||
Sacramento | 12.4 | 1,045 | 73100 | Debt | Average household debt (in dollars) | ||||||
Los Angeles | 12.9 | 1,024 | 68200 | Income | Median household income (in dollars) | ||||||
Chicago | 9.7 | 1,017 | 75100 | ||||||||
Philadelphia | 9.2 | 1,011 | 78300 | ||||||||
Minneapolis | 7 | 1,011 | 84000 | ||||||||
New York | 9.3 | 989 | 78300 | ||||||||
Atlanta | 10.3 | 986 | 71800 | ||||||||
Dallas | 8.4 | 970 | 68300 | ||||||||
Phoenix | 9.1 | 957 | 66600 | ||||||||
Portland | 10.2 | 948 | 71200 | ||||||||
Cincinnati | 9.3 | 920 | 69500 | ||||||||
Houston | 8.7 | 889 | 65100 | ||||||||
Columbus | 8.3 | 888 | 68600 | ||||||||
St. Louis | 9.9 | 886 | 68300 | ||||||||
Miami | 14.5 | 867 | 60200 | ||||||||
Detroit | 15.7 | 832 | 69800 | ||||||||
Cleveland | 9.6 | 812 | 64800 | ||||||||
Tampa | 12.6 | 791 | 59400 | ||||||||
Pittsburgh | 8.3 | 763 | 63000 |
Using a scatter plot, visualize the pair-wise relationship, i.e. Debt Payments versus Income and Debt Payments versus Unemployment Rate. For better exposition, format the x-axis and y-axis to accurately reflect the true range of the values. Simply right-click on any axis and adjust the min (or max) as needed. Also superimpose a simple linear
1
ISDS 513: Statistical Analysis
Modified from the original case study by Dawit Zerom
regression line on the scatters. Simply right-click on the data cloud and select add
trend. You go from there. Please also add R-square.
-
Describe what you observe. Do the observed simple (or marginal) relationships make
economic (or business) sense? Why? Why not?
-
Run the simple linear regression between Debt Payments versus Income. Also do the
same for Debt Payments versus Unemployment Rate. Note that these will give you same results (i.e. regression coefficients) as the trend lines you have included in the scatter plots. But, the full regression output also gives other important details. Are the observed relationships statistically significant? The p-values of the regression coefficients will help you answer the question.
-
Up on close investigation, one cant help wonder if the Unemployment Rate is TRULY negatively associated with Debt Payments. Does this really conform to reality?
-
Please note that simple linear regression will only tell you about the marginal relationship between two variables. Real life is more complicated than this because several variables are jointly associated.
-
Believing your intuition, i.e. simple linear regression may give an incomplete picture of reality, you decide to conduct multiple linear regression. In particular, you run the regression relationship between Debt Payments (dependent variable), and Income & Unemployment Rate jointly as independent variables. Hence, we have two independent (or predictor) variables.
-
Up on close inspection of the result, it appears that the regression coefficient of Unemployment Rate has a positive sign now. This is more realistic. But, the problem is that the p-value is too high in fact very close to 1 telling us the relationship does not exist. Very Confusing!! It was negative when only marginal relationships were considered and now the relationship is not there anymore. In contrast, Income is still very significant and in fact its regression coefficient remains almost the same.
-
Analytics requires great patience and being critical on any data analysis result. You must dig in and try to understand what is really happening here!!
-
Now let me think as a business student. What happens if I look at Debt Payment Income Ratio? , i.e. Debt Payment divided by Income.
-
Please create this variable in column E in Excel.
-
Plot the scatter of Debt Payment/Income versus Unemployment Rate. As usual format
the x-axis and y-axis properly. Also superimpose a linear trend including the R-square.
-
Now notice the relationship is positive, i.e. see the coefficient. However, looking
carefully at the data clouds, see some of the points when Unemployment rate is very high. In fact, these values are pooling the regression line towards them and in the process the relationship may weaken.
-
Continuing, click on the point where Unemployment Rate is the highest. Note that this point belongs to the city of Detroit in the state of Michigan. Unlike the other cities with relatively elevated Unemployment Rate, the Debt Payment/Income is much lower.
-
Having observed this, while you are in the same scatter, right-click on the Detroit and select delete. Now, the observation will be deleted. What do you notice on the trend line? The regression coefficient for the Unemployment rate increased. Further, the R-
2
ISDS 513: Statistical Analysis
Modified from the original case study by Dawit Zerom
square increased. Also notice that the scatter plot now shows a much more convincing
positive relationship.
-
In statistics, we may call the observation for the city of Detroit a possible OUTLIER.
What can we do with this observation? We have two possible options: 1) remove it
altogether or 2) replace it with a value that is more consistent with the rest of the data.
-
Lets say we decide to replace the Unemployment Rate of Detroit from 15.7% to 7.5%.
You may also try by removing Detroit but do not include in your report.
-
After adjusting the Unemployment Rate of Detroit, now, go back to our original analysis
i.e. ignore Debt Payment/Income ratio. In particular, you run the regression relationship between Debt Payments (dependent variable), and Income & Unemployment Rate jointly as the independent variables.
-
Please explore this regression result. Clearly, both Income and Unemployment Rate are statistically significant. Further, the sign of the coefficient of Unemployment Rate is positive as expected. Things are looking up!
-
Try also interpreting the regression coefficients.
Report Writing
After you have gone through the above tasks (and other tasks you may have conducted), please organize your short report using the following sections arranged in that order:
1. Problem background 2. Business questions to be answered 3. Data Analysis 4. Conclusion and Recommendation
Step by Step Solution
There are 3 Steps involved in it
Step: 1
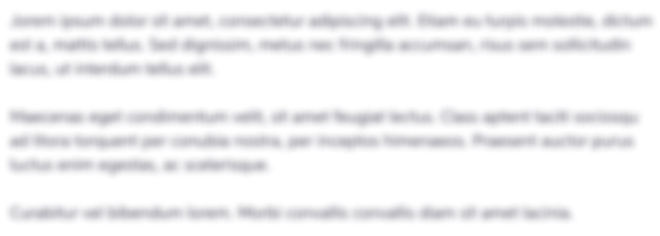
Get Instant Access to Expert-Tailored Solutions
See step-by-step solutions with expert insights and AI powered tools for academic success
Step: 2

Step: 3

Ace Your Homework with AI
Get the answers you need in no time with our AI-driven, step-by-step assistance
Get Started