Question
INSTRUCTIONS : For this assignment you have to execute and interpret one regression model. The objective is to determine how much will be saved if
INSTRUCTIONS : For this assignment you have to execute and interpret one regression model. The objective is to determine how much will be saved if 10% of complicated appendicitis cases were prevented, meaning they were instead "non-complicated." The dependent variable is defined as the total charge associated with the inpatient episode (TCHGS). The "treatment variable" of interest is complicated versus uncomplicated appendicitis. Pay special attention to it when discussing the results and provide an explanation for the outcomes between the models. Is it statistically significant? Why is it positive or negative and does this make sense? In addition to the main variable of interest, don't forget to discuss (very briefly) the other explanatory variables. In the model, in addition to the treatment variable, control for the influence of the following confounding variables (specifications are discussed in the short video): age, race, gender, ethnicity, insurance status, and severity. In your results section, provide both (a) the difference in total cost per case, e.g. complicated vs. non-complicated, and (b) the total savings is 10% of complicated cases were instead treated as non-complicated. To figure out how many cases are complicated versus non-complicated, you can use a frequency statement as shown below. 2. Which data set to use? Appendicitis.SAS7BDat. 3. Turn in a structured abstract (no more than 300 words, not including the title and section headers, so 307 in total) with the format shown below. a. A point will be deducted if you exceed the word limit. Adhering to the word limit is essential as it forces you to get to the point; at the same time you have to be careful not to omit anything of importance. i. Do not include tables or graphs in the abstract (that would go in the main text or presentation - if you were to do one)! Determine which values from your test(s) are most important to the reader and report those in the abstract. ii. Since the word limit applies to everyone equally, no exceptions will be made!!! 4. What is important or relevant information is by definition, at least partially, subjective. However, there are certain rules that have to be followed. i. For example, in the results section you should definitely discuss the significance of your test-statistic (at minimum you should provide the p-value associated with the treatment variable). If you do not, at least one point will be deducted (yes, this is a big oversight). Given the word limit, you may want to group the other variables and report as such. ii. In the methods section, provide a rationalization for using the specific method. 5. Special importance is attached to the discussion section of the abstract (see below). This is where you have to apply your creativity as a story teller. This is where you help make information out of data as it involves the reader. Try to convey why the hypothesis and the analysis are important (if you personally don't care, ask yourself why anyone would). Imagine how and what decisions could be affected by the analysis and findings and communicate this to your intended audience. Simple repetitive statements already expressed elsewhere in the abstract will result in deductions, so do not just repeat the conclusions! Abstract Objective: This contains a brief statement describing the general objective of the analysis. For example "The objective of this analysis is to examine the impact of ..." Side note: each independent variable included in the model is associated with a hypothesis. For example, a hypothesis (in alternative form) is that Hispanics have a lower percentage of charge associated with labs because (you fill in the blank). Given your word limit, you should just focus on the treatment variable. Data and methods: This contains a brief description of the particular dataset used to test the hypothesis. For example, concerning the dataset briefly mention the unit of measurement, whether the data is publicly available, and what types of variables it includes. You should also discuss what you are controlling for (e.g. age). Results: This contains a brief layman's description of the least squares results and whether or not each variable (or groups of were) was significant. Conclusions: A very brief statement indicating your conclusions pertaining to the hypothesis - did your results reject the null hypothesis or not? Discussion: This is perhaps the most important section. Here you tell the story of why you believe the analysis was important - why is it "information" and not just "data"? Why would anyone within related professional or policy circles find your analysis and results interesting? How could your analysis help such individuals in terms of decision making? You may want to select one of the listed independent variables and assume that it is your treatment variable in an experiment. This will help focus your abstract. As part of the discussion, indicate whether you would have liked to test the influence of additional variables if they had been available .
*************************Data output of SAS FOR LINEAR REGRESSION **************************
Dependent Variable is Operating room Total charges
The REG Procedure
Model: MODEL1
Dependent Variable: TCHGS TCHGS
Number of Observations Read | 47460 |
Number of Observations Used | 47460 |
Analysis of Variance | |||||
---|---|---|---|---|---|
Source | DF | Sum of Squares | Mean Square | F Value | Pr>F |
Model | 8 | 1.842725E13 | 2.303406E12 | 2473.72 | <.0001 |
Error | 47451 | 4.418411E13 | 931152403 | ||
Corrected Total | 47459 | 6.261136E13 |
Root MSE | 30515 | R-Square | 0.2943 |
Dependent Mean | 50896 | Adj R-Sq | 0.2942 |
Coeff Var | 59.95464 |
Parameter Estimates | ||||||
---|---|---|---|---|---|---|
Variable | Label | DF | Parameter Estimate | Standard Error | tValue | Pr>|t| |
Intercept | Intercept | 1 | 271956 | 2177.14211 | 124.91 | <.0001 |
AGE | AGE | 1 | 91.20939 | 7.26987 | 12.55 | <.0001 |
black | 1 | 5684.95352 | 470.91645 | 12.07 | <.0001 | |
otherNW | 1 | 6813.95711 | 425.26911 | 16.02 | <.0001 | |
female | 1 | 311.73308 | 284.31449 | 1.10 | 0.2729 | |
hispanic | 1 | 2457.95591 | 342.49490 | 7.18 | <.0001 | |
uninsured | 1 | 1136.03849 | 366.21885 | 3.10 | 0.0019 | |
complicated | complicated | 1 | 11983 | 313.34904 | 38.24 | <.0001 |
SeverityOverall | SeverityOverall | 1 | -237142 | 2110.40017 | -112.37 | <.0001 |
Logistic regression with Dependent variable =Emergency
The LOGISTIC Procedure
Model Information | ||
---|---|---|
Data Set | WORK.APPEND | |
Response Variable | postOperative | postOperative |
Number of Response Levels | 2 | |
Model | binary logit | |
Optimization Technique | Fisher's scoring |
Number of Observations Read | 28736 |
Number of Observations Used | 28736 |
Response Profile | ||
---|---|---|
Ordered Value | postOperative | Total Frequency |
1 | 1 | 1848 |
2 | 0 | 26888 |
Probability modeled is postOperative='1'.
Model Convergence Status |
---|
Convergence criterion (GCONV=1E-8) satisfied. |
Model Fit Statistics | ||
---|---|---|
Criterion | Intercept Only | Intercept and Covariates |
AIC | 13718.520 | 10969.309 |
SC | 13726.786 | 11043.702 |
-2 Log L | 13716.520 | 10951.309 |
Testing Global Null Hypothesis: BETA=0 | |||
---|---|---|---|
Test | Chi-Square | DF | Pr>ChiSq |
Likelihood Ratio | 2765.2111 | 8 | <.0001 |
Score | 4864.3455 | 8 | <.0001 |
Wald | 1982.3999 | 8 | <.0001 |
Analysis of Maximum Likelihood Estimates | |||||
---|---|---|---|---|---|
Parameter | DF | Estimate | Standard Error | Wald Chi-Square | Pr>ChiSq |
Intercept | 1 | 7.5077 | 0.3847 | 380.8706 | <.0001 |
AGE | 1 | 0.0101 | 0.00227 | 19.9878 | <.0001 |
black | 1 | 0.1945 | 0.0808 | 5.7930 | 0.0161 |
otherNW | 1 | -0.1207 | 0.0913 | 1.7481 | 0.1861 |
female | 1 | -0.4945 | 0.0558 | 78.4029 | <.0001 |
hispanic | 1 | -0.1497 | 0.0716 | 4.3635 | 0.0367 |
uninsured | 1 | -0.0698 | 0.0639 | 1.1918 | 0.2750 |
SeverityOverall | 1 | -11.3226 | 0.3626 | 975.2230 | <.0001 |
complicated | 1 | 1.1893 | 0.0551 | 466.5174 | <.0001 |
Odds Ratio Estimates | |||
---|---|---|---|
Effect | Point Estimate | 95% Wald Confidence Limits | |
AGE | 1.010 | 1.006 | 1.015 |
black | 1.215 | 1.037 | 1.423 |
otherNW | 0.886 | 0.741 | 1.060 |
female | 0.610 | 0.547 | 0.680 |
hispanic | 0.861 | 0.748 | 0.991 |
uninsured | 0.933 | 0.823 | 1.057 |
SeverityOverall | <0.001 | <0.001 | <0.001 |
complicated | 3.285 | 2.949 | 3.659 |
Association of Predicted Probabilities and Observed Responses | |||
---|---|---|---|
Percent Concordant | 85.6 | Somers' D | 0.712 |
Percent Discordant | 14.4 | Gamma | 0.712 |
Percent Tied | 0.0 | Tau-a | 0.086 |
Pairs | 49689024 | c | 0.856 |
Frequency distribution of complicated versus uncomplicated Appendicitis
The FREQ Procedure
complicated | ||||
---|---|---|---|---|
complicated | Frequency | Percent | Cumulative Frequency | Cumulative Percent |
0 | 33068 | 69.68 | 33068 | 69.68 |
1 | 14392 | 30.32 | 47460 | 100.00 |
Step by Step Solution
There are 3 Steps involved in it
Step: 1
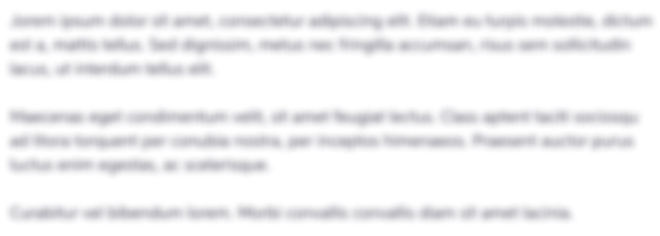
Get Instant Access to Expert-Tailored Solutions
See step-by-step solutions with expert insights and AI powered tools for academic success
Step: 2

Step: 3

Ace Your Homework with AI
Get the answers you need in no time with our AI-driven, step-by-step assistance
Get Started