Answered step by step
Verified Expert Solution
Question
1 Approved Answer
king rook vs king problem using nlp and neural networks math numpy as np | ort pandas as pd ort matplotlib.pyplot as plt sklearn.model_selection import
king rook vs king problem using nlp and neural networks
math numpy as np | ort pandas as pd ort matplotlib.pyplot as plt sklearn.model_selection import train test_split sklearn.preprocessing import LabelEncoder sklearn. neural network import ML.PRegressor sklearn.metrics import mean_squared_error sklearn.metrics import r2_score Class NeuralNet: def init (self, datafile, header=True): self.raw input = pd.read _csv(datafile, sep=,;, names [a,1,b,,3,c,c,, draw ]) \# To00: Write code for pre-processing the dataset, which would include * standardization, normalization, \# categorical to numerical, etc def preprocess(self): self.processed data = self.raw input \# setup dataframe for pandas self. df = pd.DataFrame(self, processed_data, columns ( d a ,1;,b,,3,c,2, , draw')) A preprocess categorical variables into numerical variables number = Labelencoder () self.df[' a '] = number, fit transform(self.processed data [ 'a']. astype('st 'r ' ')) self. df ' "b' - number. fit transform(self.processed_dataf 'b'].astype('str') ) self.df [ c '] - number. fit transform(self.processed datal 'c']. astype('str') ') \# TO00: Train and evaluate models for all combinations of parameters \# specified in the init method. We would like to obtain following outputs: \# 1. Training Accuracy and Error (Loss) for every model \# 2. Test Accuracy and Error (Loss) for every model 3. History Curve (Plot of Accuracy against training steps) for all the models in a single plot. The plot should be color coded i.e. different color for each model def train evaluate(self): ncols = len(self.df. columns) nrows = len(self.df.index) x= self.df.iloc [:,0:( ncols 1)] y= self.df.iloc [:, (ncols-1) ] X_train, X_test, y _train, y_test = train_test_split (x,y) \# Below are the hyperparameters that you need to use for model I evaluation activations = ['logistic', 'tanh', 'relu'] learning rate =[0.01,0.1] max iterations =[100,200] also known as epochs num_hidden_layers =[2,3] \# create the neural network and be sure to keep track of the performance " metrics mlp = MLPRegressor (hidden_layer_sizes (4,4,4), activation-'relu', solver learning rate init =0,1) mlp2 = MLPRegressor(hidden_layer_sizes =(4,4,4), activation='tanh', solven learning rate init 9.1) mlp3 - MLPRegressor(hidden_layer_sizes (4,4,4), activation- 'logistic', so learning rate init-0.1) print ('RMSE is \{\}. format (rmse)) print('R2 score is \{\}. format (r2)) print(" 1n ) mlp3.fit (x train, y_train) predict_train =mlp3, predict (X_train ) predict_test = mlp 3 . predict ( X__est ) rmse = math.sqrt(mean_squared_error_(y_test, predict_test)) r2=r2 score(y_test, predict_test) print("The model performance for training set logistic") print (" -....................... print ('R2 score is (\}, format (r2)) print ( n) \# Plot the model history for each model in a single plot. \# model history is a plot of accuracy vs number of epochs \# you may want to create a large sized plot to show multiple lines Hi in a same figure. plt.plot(mlp.loss_curve) plt.plot(mlp2.loss_curve) plt.plot (mlp3.loss_curve) plt.legend(['Relu', 'Tanh', 'Logistic'], loc- 'upper left') plt. show() return 0 if nane =" " main ": neural network = Neuralwet ("https://archive.ic5.uci.edu/ml/machine-learning-databases/chess/king-rook-vs-king/krkopt. data") a put in pa neural network.preprocess() neural_network,train_evaluate() C. The model performance for training set ietu POSE is 5.167195300172008 8) score is 0.025785467419110 end The model performance for training set tanh Petst is 5,2481794675579465 R2 score is 0.004991074263591575 The nodel performance for training set logistic PSE is 5.23603.463036178 R2 score is 0.03034525075617336244 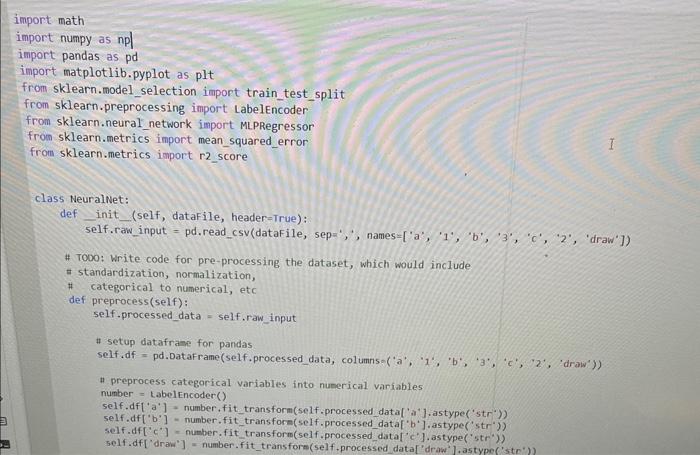
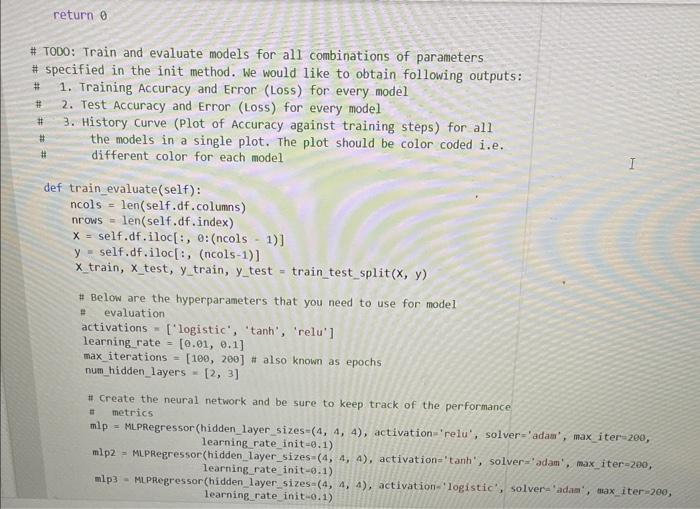
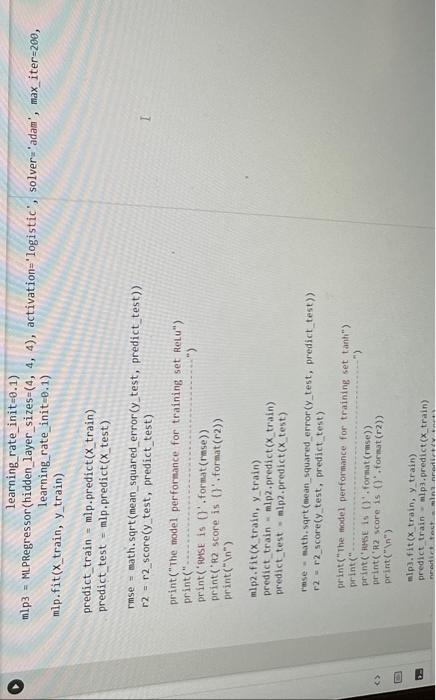
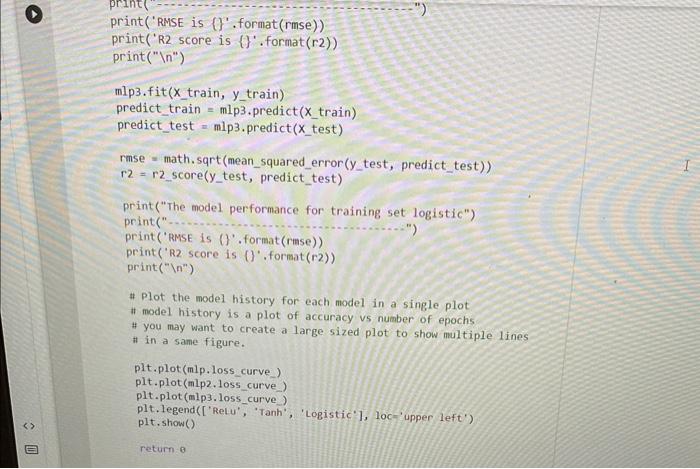
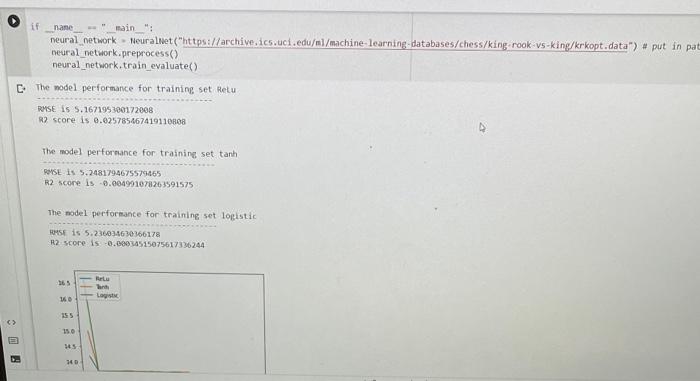
Step by Step Solution
There are 3 Steps involved in it
Step: 1
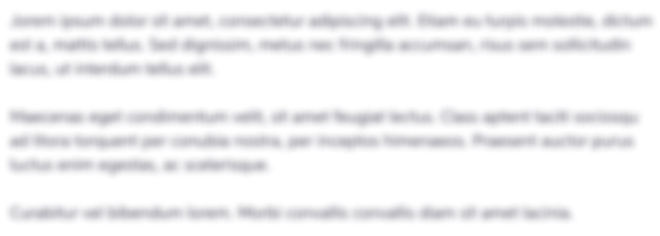
Get Instant Access to Expert-Tailored Solutions
See step-by-step solutions with expert insights and AI powered tools for academic success
Step: 2

Step: 3

Ace Your Homework with AI
Get the answers you need in no time with our AI-driven, step-by-step assistance
Get Started