Question
K-means Algorithm Implementation Question. Really need your help please help me out. In this assignment, you are asked to implement the k-means algorithm based on
K-means Algorithm Implementation Question. Really need your help please help me out.
In this assignment, you are asked to implement the k-means algorithm based on the following pseudocode.
Two java source codes are provided with the assignment. (1) kmeans.java: the core java code for k-means algorithm. This code is incomplete and you need to fill out two parts: Step1 and Step2b. All other steps are complete and you dont need to modify or update. (2) KmeansRun.java: it reads data from text file and executes the k-means algorithm. You dont need to change this code. Also, you dont need to submit this code.
Java and text files are given below.
=========================================================================
kmeans.java
import java.util.Arrays;
public class kmeans {
double[][] centers; // center of the clusters, centers[c][0],
centers[c][1], ..., centers[c][numColumns] are the centers of Cluster c
int numClusters; // number of clusters
double[][] data;
int numRows; // number of rows
int numColumns; // number of columns
int[] clustersInfo; // cluster information for each data point. if
clusterInfo[i] == c, then data point i belongs to cluster c
int kmeans_num_iter; // count the current iteration number of k-
means algorithm
final double Epsilon = 0.0001; // tolerance for comparing two double
numbers
public void kmeans_algorithm(int number_of_clusters, double[][]
data) {
kmeans_num_iter = 0;
numClusters = number_of_clusters;
this.data = data;
numColumns = data[0].length;
numRows = data.length;
centers = new double[numClusters][numColumns];
clustersInfo = new int[numRows];
//Step0: Initial Centers are set: the first numClusters
data points
for(int c=0; c centers[c] = Arrays.copyOf(data[c], data[c].length); } double Current_totalDist = Double.MAX_VALUE; double Prev_totalDist = Double.MAX_VALUE; boolean continueAlgorithm = true; while(continueAlgorithm) { kmeans_num_iter++; // Step1: Assign to the closest centers. Use calculateDistance method to calculate the distance. .... // Step2: Update cluster centers // Step2a: first initializing the current array for centers for(int c=0; c Arrays.fill(centers[c], 0); // Delete the current values } // Step2b: Write code to calculate the new centers .... // Step3: Update total distance Prev_totalDist = Current_totalDist; // before updating the total distance, save it as prev_totalDist Current_totalDist = calculateTotalDistance(); System.out.println("Iteration " + kmeans_num_iter + ": total distance = " + Current_totalDist); // Step4: terminate algorithm if the improvement in total distance between two consecutive iterations is less than Epsilon if(Prev_totalDist - Current_totalDist Epsilon) { continueAlgorithm = false; } } System.out.println(); } // Calculating the distance between two data points. You can assume array a is the data point and array b is the center. public double calculateDistance(double[] a, double[] b) { double dist = 0; for(int j=0; j dist += Math.pow(a[j]-b[j], 2); } return dist; } // Returns the total distance of your current clusters solution. public double calculateTotalDistance() { double totalDist = 0; for(int i=0; i totalDist += calculateDistance(data[i],centers[clustersInfo[i]]); } return totalDist; } } ========================================================================= ========================================================================= KmeansRun.java import java.io.*; import java.util.*; /** * Code for Extra Credit Assignment * @author */ public class KmeansRun { public static void main(String[] args) throws FileNotFoundException { // TODO Auto-generated method stub String filename = "customers_std.txt"; double[][] data = new double[100][4]; int cnt = 0; Scanner inFile = new Scanner(new File(filename)); while (inFile.hasNextLine()) { String str = inFile.nextLine(); Scanner lineScanner = new Scanner(str); double age = Double.parseDouble(lineScanner.next()); double salary = Double.parseDouble(lineScanner.next()); double married = Double.parseDouble(lineScanner.next()); double numVisit = Double.parseDouble(lineScanner.next()); data[cnt][0] = age; data[cnt][1] = salary; data[cnt][2] = married; data[cnt][3] = numVisit; cnt++; lineScanner.close(); } inFile.close(); int numClusters = 4; kmeans km = new kmeans(); km.kmeans_algorithm(numClusters, data); System.out.println("Market Segmentation Analysis by Kmeans: Your Output"); for(int c = 0; c System.out.print("Cluster " + c + ":"); System.out.print("age = " + Math.round(km.centers[c][0]*14.95 + 45.74) + ", "); System.out.print("salary = " + Math.round(km.centers[c][1]*65032 + 173750) + ", "); System.out.print("married = " + Math.round(km.centers[c][2]*0.423 + 0.77) + ", "); System.out.println("numVisit = " + Math.round(km.centers[c][3]*14.95 + 45.74)); } System.out.println(); System.out.println("Expected Output"); System.out.println("Cluster 0:age = 62, salary = 204600, married = 1, numVisit = 65"); System.out.println("Cluster 1:age = 39, salary = 167492, married = 1, numVisit = 36"); System.out.println("Cluster 2:age = 60, salary = 213420, married = 0, numVisit = 33"); System.out.println("Cluster 3:age = 29, salary = 123603, married = 0, numVisit = 39"); } } ========================================================================= ========================================================================= customers_std.txt 0.82 1.42 0.54 0.88 - 1.59 -0.41 0.54 0.02 1.29 1.39 -1.82 -0.59 - 1.05 0.77 -1.82 -0.07 1.62 -0.46 0.54 0.88 0.82 1.79 -1.82 -1.11 - 1.05 0.87 0.54 0.36 0.89 -0.27 0.54 1.05 0.75 0.96 0.54 1.48 - 0.38 -0.07 0.54 -1.11 - 0.45 0.55 0.54 -0.68 0.02 0.79 0.54 -1.11 - 0.25 1.26 0.54 -1.11 - 1.12 -0.97 -1.82 0.19 1.22 -0.30 0.54 1.23 - 0.52 -0.68 0.54 -0.68 - 0.25 -0.60 0.54 -0.68 0.62 0.10 0.54 1.23 - 1.25 -0.30 0.54 0.10 0.95 -1.05 0.54 1.48 0.55 -1.16 0.54 -0.85 1.15 2.27 0.54 1.92 - 1.05 -1.27 0.54 -0.85 - 0.45 -0.09 -1.82 -0.50 - 1.25 -1.77 -1.82 -0.50 0.02 -1.48 0.54 -0.94 - 0.05 0.82 0.54 -1.19 - 1.59 -0.82 0.54 -0.42 1.62 -1.01 -1.82 -1.19 1.49 -0.10 0.54 1.40 0.75 0.62 0.54 1.40 0.28 -0.18 0.54 -1.11 - 0.65 -0.73 0.54 -1.11 0.15 -1.29 0.54 -0.94 1.49 2.37 0.54 2.52 0.82 0.62 0.54 1.40 1.49 1.46 0.54 0.97 - 0.25 1.09 0.54 -1.02 - 0.72 -1.39 -1.82 -1.02 0.55 0.37 0.54 1.57 0.62 -0.89 0.54 1.40 0.02 -1.20 0.54 -0.94 0.95 -1.22 0.54 -0.94 - 0.12 -1.51 0.54 -0.94 - 1.25 -0.82 0.54 -0.33 - 1.12 -1.25 -1.82 -1.02 0.82 -0.27 0.54 0.88 - 0.25 0.01 0.54 -0.94 - 1.39 -1.27 -1.82 -1.11 - 1.65 -0.84 0.54 -0.07 1.42 0.20 0.54 1.14 0.22 -0.10 0.54 -0.50 - 1.19 0.41 -1.82 0.36 1.49 1.39 0.54 0.88 - 0.99 -0.14 0.54 0.02 1.22 0.54 0.54 1.57 0.55 -0.80 0.54 1.23 1.36 0.41 0.54 1.23 1.62 1.02 0.54 1.48 - 0.18 0.76 0.54 -0.68 - 1.32 0.54 -1.82 0.36 1.36 2.33 0.54 2.09 - 1.52 -0.30 -1.82 -0.07 - 1.52 -1.64 -1.82 -0.85 1.29 1.93 0.54 1.40 - 1.45 -1.19 -1.82 -0.68 0.08 1.40 0.54 -1.02 0.15 -0.10 0.54 -1.11 - 1.19 -0.56 -1.82 0.10 - 0.58 -0.97 -1.82 -0.50 1.36 0.44 0.54 1.48 - 0.85 -0.01 0.54 0.02 0.55 0.56 0.54 1.48 - 1.59 -1.53 -1.82 -1.19 0.89 -0.12 0.54 0.88 1.36 0.34 0.54 1.14 - 0.25 1.26 0.54 -0.94 - 1.32 0.15 -1.82 -0.16 0.35 -0.27 0.54 0.88 - 1.32 -1.60 -1.82 -0.68 - 0.38 1.04 0.54 -0.68 - 0.85 0.65 0.54 -0.42 - 1.05 -0.14 0.54 -0.07 1.42 -0.10 -1.82 -0.59 0.89 -0.89 0.54 1.14 - 0.25 -0.43 0.54 -0.50 1.42 0.07 0.54 0.88 1.56 1.18 0.54 1.48 - 0.05 0.68 0.54 -0.68 - 0.99 -0.95 0.54 -0.42 - 0.45 -1.22 -1.82 -1.02 0.22 -0.24 0.54 -1.02 - 1.52 -1.08 0.54 -0.16 0.02 1.31 0.54 -1.02 0.02 1.37 0.54 -0.68 - 0.58 -0.78 0.54 -0.85 - 0.25 -0.54 0.54 -0.68 - 0.05 0.87 0.54 -0.68 - 0.38 0.98 -1.82 -0.85 - 0.79 0.03 0.54 0.10 ========================================================================= Thank you.
Step by Step Solution
There are 3 Steps involved in it
Step: 1
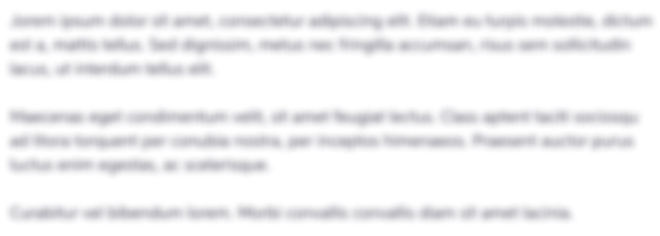
Get Instant Access to Expert-Tailored Solutions
See step-by-step solutions with expert insights and AI powered tools for academic success
Step: 2

Step: 3

Ace Your Homework with AI
Get the answers you need in no time with our AI-driven, step-by-step assistance
Get Started