Answered step by step
Verified Expert Solution
Question
1 Approved Answer
Lady Tasting Tea1 R. A. Fisher was one of the founding fathers of modern statistics. One of his early, and perhaps the most famous, experiments
\"Lady Tasting Tea\"1 R. A. Fisher was one of the founding fathers of modern statistics. One of his early, and perhaps the most famous, experiments was to test an English lady's claim that she could tell whether milk was poured before tea or not. Here's an account of the seemingly trivial event that had the most profound impact on the history of modern statistics, and hence arguably, modern quantitative science (Box 1978). Already, quite soon after he had come to Rothamstead, his presence had transformed one commonplace tea time to an historic event. It happened one afternoon when he drew a cup of tea from the urn and offered it to the lady beside him, Dr. B. Muriel Bristol, an algologist. She declined it, stating that she preferred a cup into which the milk had been poured first. \"Nonsense,\" returned Fisher, smiling, \"Surely it makes no difference.\" But she maintained, with emphasis, that of course it did. From just behind, a voice suggested, \"Let's test her.\" It was William Roach who was not long afterward to marry Miss Bristol. Immediately, they embarked on the preliminaries of the experiment, Roach assisting with the cups and exulting that Miss Bristol divined correctly more than enough of those cups into which tea had been poured first to prove her case. Miss Bristol's personal triumph was never recorded, and perhaps Fisher was not satisfied at that moment with the extempore experimental procedure. One can be sure, however, that even as he conceived and carried out the experiment beside the trestle table, and the onlookers, no doubt, took sides as to its outcome, he was thinking through the questions it raised: How many cups should be used in the test? Should they be paired? In what order should the cups be presented? What should be done about chance variations in the temperature, sweetness, and so on? What conclusion could be drawn from a perfect score or from one with one or more errors? The real scientific significance of this experiment is in these questions. These are, allowing incidental particulars, the questions one has to consider before designing an experiment. We will look at these questions as pertaining to the \"lady tasting tea,\" but you can imagine how these questions should be adapted to different situations. What should be done about chance variations in the temperature, sweetness, and so on? Ideally, one would like to make all cups of tea identical except for the order of pouring milk first or tea first. But it is never possible to control all of the ways in which the cups of tea can differ from each other. If we cannot control these variations, then the best we can do-we do mean the \"best\"- is by randomization. How many cups should be used in the test? Should they be paired? In what order should the cups be presented? The key idea here is that the number and ordering of the cups should allow a subject ample opportunity to prove his or her abilities and keep a fraud from easily succeeding at correctly discriminating the the order of pouring in all the cups of tea served. What conclusion could be drawn from a perfect score or from one with one or more errors? If the lady is unable to discriminate between the different orders of pouring, then by guessing alone, it should be highly unlikely for that person to determine correctly which cups are which for all of the cups tested. Similarly, if she indeed possesses some skill at differentiating between the orders of pouring, then it may be unreasonable to require her to make no mistakes so as to distinguish her ability from a pure guesser. An actual scenario described by Fisher and told by many others as the \"lady tasting tea\" experiment is as follows. 1 Adapted from Stat Labs: Mathematical statistics through applications by D. Nolan and T. Speed, SpringerVerlag, New York, 2000 1 For each cup, we record the order of actual pouring and what the lady says the order is. We can summarize the result by a table like this: Lady says Tea first Milk first Order of Actual Pouring Tea first Milk first a b c d a+c b+d a+b c+d n Here n is the total number of cups of tea made. The number of cups where tea is poured first is a + c and the lady classifies a + b of them as tea first. Ideally, if she can taste the difference, the counts b and c should be small. On the other hand, if she can't really tell, we would expect a and c to be about the same. Suppose now that to test the lady, 8 cups of tea are prepared, 4 tea first, 4 milk first, and she is informed of the design (that there are 4 cups milk first and 4 cups tea first). Suppose also that the cups are presented to her in random order. Her task then is to identify the 4 cups milk first and 4 cups tea first. This design fixes the row and column totals in the table above to be 4 each. That is, a + b = a + c = c + d = b + d = 4. With these constraints, when any one of a, b, c, d is specified, the remaining three are uniquely determined: b = 4 a, c = 4 a, and d = a In general, for this design, no matter how many cups (n) are served, the row total a + b will equal a + c because the subject knows how many of the cups are \"tea first\" (or one kind as supposed to the other). So once a is given, the other three counts are specified. We can test the discriminating skill of the lady, if any, by randomizing the order of the cups served. If we take the position that she has no discriminating skill, then the randomization of the order makes the 4 cups chosen by her as tea first equally likely to be any 4 of the 8 cups served. There are 84 = 70 (in R, choose(8,4)-see also Devore pp. 71-73) possible ways to classify 4 of the 8 cups as tea first. If the subject has no ability to discriminate between two preparations, then by the randomization, each of these 70 ways is equally likely. Only one of 70 ways leads to a completely correct classification. So someone with no discriminating skill has 1/70 chance of making no errors. It turns out that, if we assume that she has no discriminating skill, the number of correct classifications of tea first (\"a\" in the table) has \"hypergeometric\" probability distribution (see help(dhyper) in R or Devore pp. 128-129). There are 5 possibilities: 0, 1, 2, 3, 4 for a and the corresponding probabilities (and R commands for computing the probabilities) are tabulated below. Number of correct calls 0 1 2 3 4 R command dhyper(0,4,4,4) dhyper(1,4,4,4) dhyper(2,4,4,4) dhyper(3,4,4,4) dhyper(4,4,4,4) 2 Probability 1/70 16/70 36/70 16/70 1/70 With these probabilities, we can compute the p-value for the test of the hypothesis that the lady cannot tell between the two preparations. Recall that the p-value is the probability of observing a result as extreme or more extreme than the observed result assuming the null hypothesis. If she makes all correct calls,the p-value is 1/70 and if she makes one error (3 correct calls) then the p-value is 1/70 + 16/70 0.24. The test described above is known as \"Fisher's exact test.\" References Box, J. F. (1978). R. A. Fisher: The Life of a Scientist. John Wiley & Sons, Inc., New York. 3 Hi all, We have now gone through a milestone with the midterm. Our focus now will be on inferential statistics, which simply means taking a sample and making an educated guess about the true nature of the parameters. To help you understand we have PQ4 (see also my car detailing example) and exercise 2. Parameters as opposed to "statistics" are those measurements of a population and the most common is the population mean (and sometimes variance). So we ask ourselves does our "statistic" really represent the parameter? In simple terms is our sample mean (or variance) close to the population mean (or variance)? We do this by hypothesis testing. i know your are inundated with various distributions to do this but the basic concept is about the same and if you understand that then you will be able to handle any new distribution that comes along. Some of you have struggled but you are not alone because the giants of statistics struggled, got confused and also picked the wrong stat method but they worked until they got it (mostly) right. Lady Tasting Tea (see also Wikipedia) Let's have a moment for reflection. Where did we get to this point of hypothesis testing? It's actually a rather fascinating history. We have been on this road for hundreds of years but let's start with a nice summer day in England in the 1920's and believe it or not, A Lady Tasting Tea. Fun reading and don't worry not a bunch of math and formulas. From the publisher: At a summer tea party in Cambridge, England, a lady states that tea poured into milk tastes differently than that of milk poured into tea. Her notion is shouted down by the scientific minds of the group. But one guest, by the name Ronald Aylmer Fisher, proposes to scientifically test the lady's hypothesis. There was no better person to conduct such a test, for Fisher had brought to the field of statistics an emphasis on controlling the methods for obtaining data and the importance of interpretation. He knew that how the data was gathered and applied was as important as the data themselves. In The Lady Tasting Tea, readers will encounter not only R.A. Fisher's theories (and their repercussions), but the ideas of dozens of men and women whose revolutionary work affects our everyday lives. Salsburg traces the rise and fall of Karl Pearson's theories, explores W. Edwards Deming's statistical methods of quality control (which rebuilt postwar Japan's economy), and relates the story of Stella Cunliffe's early work on the capacity of small beer casks at the Guinness brewing factory. The Lady Tasting Tea is not a book of dry facts and figures, but the history of great individuals who dared to look at the world in a new way. From Wikipedia: The lady in question claimed to be able to tell whether the tea or the milk was added first to a cup. Fisher proposed to give her eight cups, four of each variety, in random order. One could then ask what the probability was for her getting the number she got correct, but just by chance. The experiment provided the Lady with 8 randomly ordered cups of tea - 4 prepared by first adding milk, 4 prepared by first adding the tea. She was to select the 4 cups prepared by one method. This offered the Lady the advantage of judging cups by comparison. The Lady was fully informed of the experimental method. The null hypothesis was that the Lady had no such ability. Note that in Fisher's approach, there is no alternative hypothesis;[2] this is instead a feature of the Neyman-Pearson approach. The test statistic was a simple count of the number of successes in selecting the 4 cups. The null hypothesis distribution was computed by the number of permutations. The number of selected permutations equaled the number of unselected permutations. Tea-Tasting Distribution Success Number of Permutations of selection count permutations 0 oooo 11=1 1 ooox, ooxo, oxoo, xooo 4 4 = 16 ooxx, oxox, oxxo, xoxo, xxoo, 2 6 6 = 36 xoox 3 oxxx, xoxx, xxox, xxxo 4 4 = 16 4 xxxx 11=1 2 Total 70 There were no z tables but as you see by the permutations (you now have a basic understanding) there is only 1 in 70 chance that the lady could have guessed so a rough alpha/p value of 1.4 %. Thus the start of hypothesis testing but also a feud between Karl Pearson and Fisher (remember the F test). Pearson believed in a a null hypothesis AND the alternative hypothesis while Fisher believed in only using the null hypothesis. This deep philosophical discussion still divides stats today but beyond the scope of this course. The feud escalated when the upstart Fisher showed that some of Pearson's distribution tables were in error! Using difficult to understand, n-diiminsional geometry Fisher showed you needed a correction and introduced a new concept called degrees of freedom which you use today for a correction of some of the tables. NO you don't need to know about n-dminsional geometry in this course!!!! We now go from tea to beer or well from tea to t. As you know you use the Student t test for samples less than 30 but how did it come about? This is discussed on page 311 and William Gosset a humble man. My first textbook as a student covered the t distribution but didn't even bother to mention his name. Gosset took some of the distributions created by Pearson and saw they explained the error of small sample sizes. Finally, let's not forget Carl Gauss. He basically introduced the Gaussian distribution (z) that we use today and commonly call the standard distribution (see p. 221). The normal distribution is so important in the world today that the German government issued a 10 mark note (before the euro) with his image. Note the curve on the left of his image. I lived in Germany during this time and I could always pull out a 10 Mark note to the class (military students). 3 Finally, I've enclosed an explanation of the Lady tasting tea but this is for your fun and certainly not mandatory reading. If you look at the history of statistics, a young science you will see this math whizzes made mistakes like you and struggled in getting the right methodologies and distributions/tables to eliminate errors in predicting the reliability of samples. We didn't get for example, the degrees of freedom overnight. It was developed when individuals saw errors. What you have and they didn't have was a computer and software that you can now simulate thousands of random samples that might have taken them a year or even years of work and I guess, lots of graph paper. Anyway, if you do struggle remember you have some pretty smart company who struggled as well! Tony 4 \"Lady Tasting Tea\"1 R. A. Fisher was one of the founding fathers of modern statistics. One of his early, and perhaps the most famous, experiments was to test an English lady's claim that she could tell whether milk was poured before tea or not. Here's an account of the seemingly trivial event that had the most profound impact on the history of modern statistics, and hence arguably, modern quantitative science (Box 1978). Already, quite soon after he had come to Rothamstead, his presence had transformed one commonplace tea time to an historic event. It happened one afternoon when he drew a cup of tea from the urn and oered it to the lady beside him, Dr. B. Muriel Bristol, an algologist. She declined it, stating that she preferred a cup into which the milk had been poured first. \"Nonsense,\" returned Fisher, smiling, \"Surely it makes no dierence.\" But she maintained, with emphasis, that of course it did. From just behind, a voice suggested, \"Let's test her.\" It was William Roach who was not long afterward to marry Miss Bristol. Immediately, they embarked on the preliminaries of the experiment, Roach assisting with the cups and exulting that Miss Bristol divined correctly more than enough of those cups into which tea had been poured first to prove her case. Miss Bristol's personal triumph was never recorded, and perhaps Fisher was not satisfied at that moment with the extempore experimental procedure. One can be sure, however, that even as he conceived and carried out the experiment beside the trestle table, and the onlookers, no doubt, took sides as to its outcome, he was thinking through the questions it raised: How many cups should be used in the test? Should they be paired? In what order should the cups be presented? What should be done about chance variations in the temperature, sweetness, and so on? What conclusion could be drawn from a perfect score or from one with one or more errors? The real scientific significance of this experiment is in these questions. These are, allowing incidental particulars, the questions one has to consider before designing an experiment. We will look at these questions as pertaining to the \"lady tasting tea,\" but you can imagine how these questions should be adapted to dierent situations. What should be done about chance variations in the temperature, sweetness, and so on? Ideally, one would like to make all cups of tea identical except for the order of pouring milk first or tea first. But it is never possible to control all of the ways in which the cups of tea can dier from each other. If we cannot control these variations, then the best we can do-we do mean the \"best\"- is by randomization. How many cups should be used in the test? Should they be paired? In what order should the cups be presented? The key idea here is that the number and ordering of the cups should allow a subject ample opportunity to prove his or her abilities and keep a fraud from easily succeeding at correctly discriminating the the order of pouring in all the cups of tea served. What conclusion could be drawn from a perfect score or from one with one or more errors? If the lady is unable to discriminate between the dierent orders of pouring, then by guessing alone, it should be highly unlikely for that person to determine correctly which cups are which for all of the cups tested. Similarly, if she indeed possesses some skill at dierentiating between the orders of pouring, then it may be unreasonable to require her to make no mistakes so as to distinguish her ability from a pure guesser. An actual scenario described by Fisher and told by many others as the \"lady tasting tea\" experiment is as follows. 1 Adapted from Stat Labs: Mathematical statistics through applications by D. Nolan and T. Speed, SpringerVerlag, New York, 2000 1 5 Hi class, Here is an example for question 1 in Exercise 1, which would be similar to questions 2 and 3. I will first tell you a made up story that hopefully will be fun to read but will help you understand the hypothesis process. We have to start with some management question or issue. Use a little creativity and come up with one related to your work on business. The other day, I was asked if I wanted my car \"detailed.\" Well, I never heard of it and I guess it's a fancy name for cleaning the inside of your car. So let's just start with thatsomething I have almost zero knowledge about. I was wondering who would pay for thisnot me but there must be people who would be willing to pay for it. If I remember the price was $250. No way I would pay but someone must be willing to pay. So I will use this idea for a management scenario. Just use your imagination and story telling skills and you can come up with your own...no math needed for this exercise. Now I have magically given someone a new job at Don's Details which was also made up in my mind. Let's say he is the executive director and operating manager for a chain of car detailing shops in Annapolis. We will call him John. I will let John take over now. I am considering expanding to Baltimore but concerned about this issue: can I (John) charge enough to stay in business in Baltimore, which was hit harder economically than Annapolis. I cannot assume what is successful in Annapolis would be successful in Baltimore. Our service is a luxury item that people tend to forgo when having financial problems (at least that's what I am assuming). I looked at trade journals (not really but I am pretending in this role) but couldn't find any data that would help me make a knowledge-based decision. I go into Mary's office the accountant and CFO. We like to always banter each other...since we seem to be the oldest employees and old school types. She has also kept me out of trouble, as she is one heck of an accountant and CFO with a vision. Mary: (Mary looks up from her computer) Hi John. (Looks at me appraisingly): what's that look on your face? Every time I see that look I know it's going to cost the company money or give me an ulcer (she grins). John: No, no Mary, it's about Baltimore... Mary: (she rolls her eyes): Ohhh yes let me get out my Tums (she laughs). It's not the let's expand to Baltimore story. We have just begun to succeed in Annapolis and the economy...shaky shaky and the uncertain government regulations not to mention the uncertainty over the federal and state HR laws. How about a more pleasant subject, would you like me to bore you with my indexes and news clippings? John: Mary, good old Baltimore just makes it more exciting...we are big and bold! Besides, we've shown we have a very robust business model if we can get beyond the cost threshold of what customers are willing to pay.... and no, spare me the indices and your cutouts. Mary: Business model or not, risky, risky, risky but let's talk (she sits back on her Aeron chair (claims a good management investment by buying these expensive chairs). She stares out between the myriad of Excel printouts stacked like mountains on her desk (likes hard copies) and arcane books on accounting and fiduciary matters). Mary: And, they are not cutouts but clippings! Cutouts are those spy thingys. John: well, since there is no specific marketing data I want to propose to the directors that we conduct a survey in Baltimore to determine if it is economically feasible to start a pilot store in this market. What I need from you is the absolute minimum cost it takes to detail a car so I can compare to what people are willing to pay in Baltimore. Mary: I anticipated that you would ask me that question and the answer is $90 but that's based on the Annapolis market. I would guess that labor, indirect, real estate costs, etc. would be lower in Baltimore but just can't say. I am comfortable with $90 but you (pointing an accusatory finger at me) don't even think about anything lower! John: No, no that number is fine. $90 will be my conservative number so the board of directors will see I am erring on the lower risk side and you will back me up on that number. Mary: Of course I will if I said it I back it up. Now I got some real work to do to so leave me alone. (She swivels around back to her computer, which is like her pet dog. John is used to such abruptness and amused by it). John: Thanks Mary, you are cheerful as always. I will let you get back to Rover (the name he gives to her computer). Mary: (she mutters with her back to him) Ruff, ruff. I go back to my office, thinking how will I convince the board of directors (mainly investors) to spend the money on a survey. I will do a telephone survey although knowing I may underrepresent younger people who tend to use mobile phones. However, I am not after perfection and I think it will give me a good enough answer. So now I have $90 from Mary so let me now do question 1. I will take the $90 and add a profit margin ROI (return on investment) of about 20%, which is enough to make these investors/directors happy. Story is over so let's now do question 1 of the assignment. Red text will be my comments/instructions and would not be included if I submitted it as a student. 2 1a. Preparation: Explain the problem you would test for (make up numbers) and state the hypotheses in both numeric and narrative form. Explain why this would be a upper tailed test. At this time you have not collected any data in your pretend scenario. State what course of action your management team will make if (1) Ho is rejected. From the operating manager to board of directors of Don's Details: 1a. For the last three years, Don's Details has used is a viable business model that specializes in detailing cars as demonstrated in the Annapolis market. The market there appears to be saturated based on recent accounting reports (by Mary). With approval we will conduct a survey to show this business model is also viable in the Baltimore market. We know according to accounting, the cost of a typical detail on a car costs us $90, which includes indirect and labor costs. This cost may be less in the Baltimore market but to reduce risk we will keep the $90 estimate. We also know that the directors expect a ROI of at least 20% so we will round this up $110 that we must charge as a minimum. The research question then is simple: will those potential customers in Baltimore be willing to pay at least $110 for quality detailing? Since this is a risky, volatile market and an especially unknown market in Baltimore we want to minimize risk so we will use an alpha of .05 so as to minimize the probability of a Type I error. The hypotheses for a onesample test are as follows: Hypothesis (numeric expression) Ho: u<=$110 Ha: u>$110 Narrative Ho: population mean is less than or equal to $110 the maximum Baltimore residents would be willing to pay for expert car detailing. Ha: population mean is greater than $110 the maximum Baltimore residents would be willing to pay for expert car detailing. This is a one tailed test upper since we are interested in a population mean being greater than a value ($110) which would then be to the right half of the distribution and the far right would be the rejection region, alpha. If we reject Ho then we recommend opining up a pilot store in Baltimore after conducting the appropriate site surveys. As you see, I have made it clear that before the survey what management action I will recommend we take BEFORE we have even conducted the survey. I haven't said what I would recommend if we fail to reject Ho and I may do that because of management or strategic reasonsI may want to keep my options open. It is perfectly all right to say what you will do or recommend if you fail to reject Hathat's up to you. As you see, math isn't always the primary driver. Now let's go to 1b. 3 1b. A telephone survey will be conducted where numbers will be randomly selected from the Baltimore phone book. We will ask about ten questions but the first three are important to us: (1) Do you have a car? (2) If question one is yes would you be willing to pay for detailing for your car at least once a year? (3) If answer 2 is yes, what would be the maximum you would pay for expert quality detailing of your car? The 3rd question is the variable of interest needed to test our Ha. The person must be at least 18 years of age or that observation will not be used. To limit survey costs, we will terminate the survey when we have a sample size of n=200 adults who would consider paying for car detailing. The population of interest is the adults (18 years or older) of Baltimore and Baltimore County. There could be some bias since we are using a telephone survey and younger people tend not to have land lines but we think it is worth the risk and not practical to do a door to door or other expensive sampling methodology. As described above we are requiring a higher mean and a conservative alpha (not .1) to reduce the risk of a type one error. So, let's stop a moment. At this point we haven't done a survey, but let me ask you: Do you have a pretty good idea of what I plan to do? Do you know what I will be asking the citizens of Baltimore and do you know what I plan to recommend to the board of directors if we do reject the null hypotheses? Do you know at least in broad terms how I will conduct the experiment? Do you at least have some confidence that I have done a reasonable job of minimizing risk as for as a Type 1 error? Could you take what I written and produce the same experiment? Have I missed telling you so information that you need like what is the sample size or what is the is the population or how do I obtain the sample? If this was a real report I would have to have much more detail on my sampling methodology but that is beyond the scope of this course and all I ask of you is a very basic sampling methodology that has the word \"random\" and at least a rudimentary methods to attempt a random sample. Whole courses however could be taught on insuring this and way beyond the scope of this course. Also, we must be careful how we phrase the survey questions but we assume in this course we would phrase them properly (not always the case in the real world). Now we are ready for the execution and reporting. Here would be my question 1 if I was a student, which would include 1a and 1b and none of this red text of course. Let's now finish up question 1. 1c. This test is worth conducting because like Annapolis there may be great opportunities to expand in a growing market. However, we should not even start a pilot store in Baltimore without at least a simple market survey. This survey will be reasonably inexpensive and could provide valuable information that confirms our belief we should go into this market but the test can also stop us from making a costly business mistake if we are unable to reject Ho (see 1a) that in essence means not a good business decision to expand to the Baltimore market. Now we actually conduct the test. The alpha value for a upper one tailed test at .05 is z=1.645. We will then reject if our test statistic is z>1.645. Since our computed test statistic from our actual data is z=2.85 (sample mean is $129, i.e., the mean response on how much a Baltimore respondent would be willing to pay for detailing), we reject Ho because our test statistic (z=2.85) is 4 greater than our test statistic (z=1.645). We therefore accept Ha and recommend to management (see part 1a) that we start a pilot store in Baltimore. We also see that the computed p value is .0325, which is much less than the threshold value of our alpha, which was .05. That's about it! You didn't need advanced math or really any math at all and you only needed to look up one thing in a table and for a one tailed test at .05, z is always 1.645. If you had a sample size of less than 30 then you would use a t test for a simple onesample test. If you have qualitative data you would test proportions (not asked in this assignment). I will not do an example for 1TL or 2T but would be similar. I am reluctant to give examples however, since it could limit flexibility and one challenge I have for you is to use your imagination and creativity to come up with management scenarios as I did. Your examples didn't have to be a marketing example, it could be based on looking at the effectiveness of training or a management decision or even a quality control question. The common element is that for whatever reason you can't query an entire population (as you define a population, in my case the adults in Baltimore who would consider auto detailing) and therefore need to take a sample that tried to answer a question (Ha) based on a management issue. Notice, I didn't lecture on statistics although I may have \"reminded\" the reader or board of directors a little about the significance of a Type I error. Also, you were not expected to know ROI or even if paying $110 is even a realistic number for car detailingI don't care since we just make up those numbers except for the alpha z value at .05 which is z=1.645 (always). It's the same for you as long as within reason, for example, 2 cents for detailing of course would be stretching credibility but if you said $20 or even $400 that would be OK since we are just practicing. Like some DVD's let's provide an alternative ending to this story. You only have to say fail to reject or reject in your assignment and discuss one or the other scenario. Let's now provide an alternative ending and go back to Mary's office starting at 1c: The alpha value for a upper one tailed test at .05 is z=1.645. We will then reject if our test statistic is z>1.645. Since our computed test statistic from our actual data is z=1.25 (sample mean is $112, i.e., the mean response on how much a Baltimore respondent would be willing to pay for detailing), we fail to reject Ho because our test statistic (z=2.85) is less than our alpha value @ .05 (z=1.645). We therefore cannot accept Ha and recommend to management (see part 1a) that we hold off on a pilot store in Baltimore and do further market research next year. We think our sample size was large enough (n=200) and our methodology sound, that a further test would product similar results. However, we recommend another survey next year. We also see that the computed p value is .0637, which is more than the threshold value of our alpha, which was .05. Since you failed to reject Ho you could have your own recommendation not just what I said. Perhaps you think a competitor may beat us to the market and believe a sample 5 size of 500 might get better results and not wait next year. As long as you logically explain your reasoning it's OK if your recommendation is different. Also, you need to listen and read carefully any company policy or desire that might influence how you frame your Ha. However, what is not OK is to state Ho is true. You don't know if it is true or not and your focus has always been on Ha which is your hypothesis you think is true or hope to be true. You may assume as for as your decision that Ho is the status quo but you have not proven Ho and you don't have enough proof for Ha. Never state you have proven Ho!!! You just know there is too much of a risk not to assume you would have a Type 1 error if you accept Ha. Now for fun, let's go back to Mary's office with the scenario that we failed to reject Ho. John goes to Mary's office about three months after the detailing discussion. John: Hi Mary, stepped in to let you know that I have recommended to the board of directors not to at this time open up a pilot store in Baltimore. Mary: (she does her characteristic chair swivel from her computer (Rover) behind her-removes her reading glasses and says sarcastically in what she takes for humor), Well less work for me. All I need is more books to balance. Well seriously are people in Baltimore cheapskates or really still hurting from the economy? (Back to her sarcastic mode:) Or perhaps people in Baltimore have no cars? Maybe we could detail pets? John: Hey don't diss Baltimore some of my best friends live there. It's just that we found that the mean cost people were willing to pay was only $112, which was not significant enough. Mary: Wait, didn't you say based on the data I gave you that $110 was a viable plan and in fact, quite conservative? That's 2 dollars over the minimum so did you change the threshold? John: No not at all, we kept the $110 but that wasn't the problem. Yes, if we knew beyond a reasonable doubt (what's called a Type 1 error) that $110 reflected the Baltimore adult population who wanted detailing for $110 or more we would go with the pilot store. We just couldn't be sure enough of our sample and the board of directors tend to be low risk takers. You know that and how they drive you crazy. I can't fight that. I could not prove my hypothesis (Ha) that customers would pay $110 and yes, the mean was a $112 but there as a possibility that $112 was just \"luck\" or what you and I call bogus and not the true population. The p value was .0637 which means that there is close t a 7% chance my sample could just have a high mean that doesn't reflect reality. It's like the one time I won fifty bucks on the lotto and therefore based on that one \"sample\" take all my money out of the bank and play lotto. More or less there is a 7% that our sample is a fluke and not the representative of the Baltimore population. Do you remember Dewey? Mary: Who? Who we? 6 John: Never mind. Like a murder trial you have to go beyond a reasonable doubt when you do hypothesis testing on a sample. Mary: Hmmm, I am old school and never took a stat class and please don't make me watch \"Show me your stats\" again on You Tube, but isn't 7% good enough? I would take that bet in Atlantic City. John: Well changing the alpha after the fact, to let's say to an alpha of .1 (z=1.282) so you can reject Ho is considered unethical and is really just bad decision making and not professional. If we really think 7% is acceptable risk then we should have picked another alpha instead of .05 such as .1. It's like playing a game and saying the best out of 3, then if you don't win, best out of 5, then out of 7 until you finally win. If you really thought .1 was acceptable then pick .1 before the experiment. If you then change it really shows you aren't that professional because you should have thought it through at the beginning. Also, what if you then make a decision based on that and we lose lots of money on the pilot store? Oh, Mr and Ms. Directors, sorry I did the experiment so I didn't believe in the results so I changed the rules so I can have my pilot store because I am a greedy manager who wants to expand my domainblah blah blah. Although a pilot store it could seriously impact our reputation with the stockholders and future capital spending. Does that make sense to you? In know I don't understand some of your arcane accounting practices and I am now ranting. Mary: Yes, that makes sort of sense. I think I am going to take a stat course to fill in some of the gaps of my knowledge and then I'll know if you are giving me just a lot of whoowie? John: Who? You mean Dewey? Mary: Shut up with the Dewey. John: Sure, but on your great suggestion, I think a basic stat course for all managers would be helpful. It's really not that complicated to get a basic understanding. Of course, statistics will not replace experience and good management but good management includes objectively looking at the data and not just wishful thinking. Mary: OK, enough get out of here unless you have accounting business with me! Of course, \"Mary\" may know more about statistics in real life management, but I wanted her to not have knowledge of stats, as she was my proxy for a student. Blame her for her disparaging remarks about the people of Baltimore. She was actually born in Baltimorewell if any of this was real. When you wrote up parts 1, 2, 3 I doubt you had such dialogs in your mind and I just did this not only to attempt to add a little humor to the subject but also such general dialogs do exist in organizations and businesses. These are not math professors that are generating the genesis for hypothesis testing. The alternatives would be to base such decisions on emotion, intuition, and wishful 7 thinking. Statistics isn't replacing your experience but offers a moment to reflect on the questions, are making a wise decision? It can also show you data that might say, you should take this action or explore this avenue. This is also true in research or in government decision making so it just doesn't apply only in our excample about financials. It might be about making better decision to improve your business process. 8
Step by Step Solution
There are 3 Steps involved in it
Step: 1
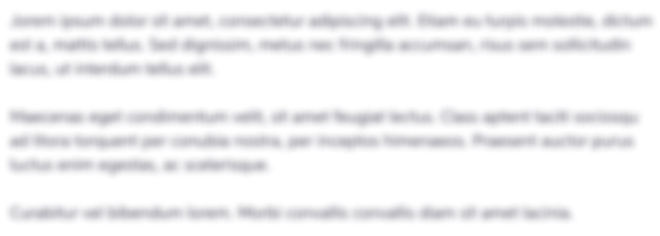
Get Instant Access to Expert-Tailored Solutions
See step-by-step solutions with expert insights and AI powered tools for academic success
Step: 2

Step: 3

Ace Your Homework with AI
Get the answers you need in no time with our AI-driven, step-by-step assistance
Get Started