Answered step by step
Verified Expert Solution
Question
1 Approved Answer
Machine learning question, use jupyter lab, no AI plz: Import this: import sys from packaging import version import sklearn import matplotlib.pyplot as plt import numpy
Machine learning question, use jupyter lab, no AI plz: Import this: import sys from packaging import version import sklearn import matplotlib.pyplot as plt import numpy as np import tensorflow as tf from sklearn.preprocessing import adddummyfeature from sklearn.datasets import makeblobs from sklearn import metrics #Import scikitlearn metrics module for accuracy calculation from sklearn.modelselection import traintestsplit from sklearn.metrics import confusionmatrix, ConfusionMatrixDisplay printSklearn package",sysversioninfo printSklearn package",sklearn.version printTensorFlow version:", tfversion assert sysversioninfo assert version.parsesklearnversion version.parse pltrcfont size pltrcaxes labelsize titlesize pltrclegend fontsize pltrcxtick labelsize pltrcytick labelsize Questions: Q Finetune learning rate, and number of hidden units using the training set. Use a two layer model with one hidden layer. Store your best trained model in bestmodel, Store the best number of hidden units in besthu Store the best number of hidden units in bestlr For learning rate, try some values from to For hidden units, try some values from Other parameters should be: epochs batchsize and 'adam' as the optimizer Print the accuracy of the model on the testing dataset, xtest Hints: You have to use two for loops for the two parameters you are trying to finetune. You can get a testing accuracy above #Q bestmodel None bestaccuracy bestlr besthu ###Write your code here##### #uncomment these two line to test your bestmodel #acc bestmodel.evaluatextest, ytest, verbose #printlearning rate", bestlr "hidden units", besthu "Testing Accuracy", acc SEE Q PIC Q Calculate the gradient of with respect to and using the backpropagation algorithm Calculate the gradient of with respect to and using the computation graph in tensorflow Declare intermediate variables, and so on Remember to use is needed when is used in multiple paths of the computation similarly dfdy x y #Forward pass #Backward pass #printf #printdfdxdfdy #f #dfdx #dfdy #using computation graph from tensorflow x tfVariable y tfVariable #printf #printgradxgrady See Q pic Calculate the gradient of with respect to and using the backpropagation algorithm cdots # Using backpropagation, construct some intermediate # variables for $qy$ and z xq then finally f npsumz x nparray y nparray m xshape printx printy #Forward Pass #Backward Pass #printff #printdfdxdfdx #printdfdydfdy #Sample output #f #dfdq #dfdx #dfdy see q pic Calculate the gradient of with respect to and using the computation graph in tensorflow #using tensorflow x tfVariable trainable True, namex y tfVariable trainable True, namey #tfprintff #gradx grady tpgradientfxy #tfprintgradxgradx #tfprintgradygrady #Sample output #f #gradx #grady
Machine learning question, use jupyter lab, no AI plz:
Import this:
import sys
from packaging import version
import sklearn
import matplotlib.pyplot as plt
import numpy as np
import tensorflow as tf
from sklearn.preprocessing import adddummyfeature
from sklearn.datasets import makeblobs
from sklearn import metrics #Import scikitlearn metrics module for accuracy calculation
from sklearn.modelselection import traintestsplit
from sklearn.metrics import confusionmatrix, ConfusionMatrixDisplay
printSklearn package",sysversioninfo
printSklearn package",sklearn.version
printTensorFlow version:", tfversion
assert sysversioninfo
assert version.parsesklearnversion version.parse
pltrcfont size
pltrcaxes labelsize titlesize
pltrclegend fontsize
pltrcxtick labelsize
pltrcytick labelsize
Questions:
Q
Finetune learning rate, and number of hidden units using the training set.
Use a two layer model with one hidden layer.
Store your best trained model in bestmodel,
Store the best number of hidden units in besthu
Store the best number of hidden units in bestlr
For learning rate, try some values from to
For hidden units, try some values from
Other parameters should be: epochs batchsize and 'adam' as the optimizer
Print the accuracy of the model on the testing dataset, xtest
Hints:
You have to use two for loops for the two parameters you are trying to finetune.
You can get a testing accuracy above
#Q
bestmodel None
bestaccuracy
bestlr
besthu
###Write your code here#####
#uncomment these two line to test your bestmodel
#acc bestmodel.evaluatextest, ytest, verbose
#printlearning rate", bestlr "hidden units", besthu "Testing Accuracy", acc
SEE Q PIC
Q
Calculate the gradient of with respect to and using the backpropagation
algorithm
Calculate the gradient of with respect to and using the computation graph in
tensorflow
Declare intermediate variables, and so on Remember to use is needed when is used in
multiple paths of the computation similarly dfdy
x
y
#Forward pass
#Backward pass
#printf
#printdfdxdfdy
#f
#dfdx
#dfdy
#using computation graph from tensorflow
x tfVariable
y tfVariable
#printf
#printgradxgrady
See Q pic
Calculate the gradient of with respect to and using the backpropagation
algorithm
cdots
# Using backpropagation, construct some intermediate
# variables for $qy$ and z xq then finally f npsumz
x nparray
y nparray
m xshape
printx
printy
#Forward Pass
#Backward Pass
#printff
#printdfdxdfdx
#printdfdydfdy
#Sample output
#f
#dfdq
#dfdx
#dfdy
see q pic
Calculate the gradient of with respect to and using the computation graph in
tensorflow
#using tensorflow
x tfVariable trainable True, namex
y tfVariable trainable True, namey
#tfprintff
#gradx grady tpgradientfxy
#tfprintgradxgradx
#tfprintgradygrady
#Sample output
#f
#gradx
#grady
Step by Step Solution
There are 3 Steps involved in it
Step: 1
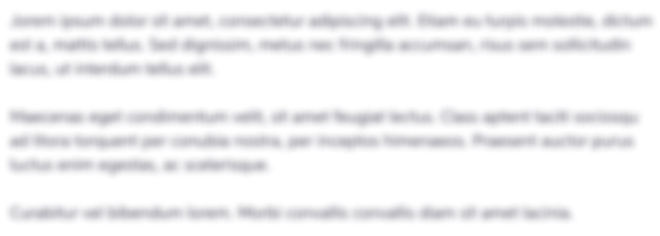
Get Instant Access with AI-Powered Solutions
See step-by-step solutions with expert insights and AI powered tools for academic success
Step: 2

Step: 3

Ace Your Homework with AI
Get the answers you need in no time with our AI-driven, step-by-step assistance
Get Started