Answered step by step
Verified Expert Solution
Question
1 Approved Answer
MGS 3100 PROJECT 2 Regression Model (Group Project - Due 12th (Sec 040) or 13th April (Sec 010 and 050)) Purpose of the Project The
MGS 3100 PROJECT 2 Regression Model (Group Project - Due 12th (Sec 040) or 13th April (Sec 010 and 050)) Purpose of the Project The purpose of this project is for you to gain experience in constructing and applying a simple regression model using spreadsheet. To finish the project without major hiccups and headaches strictly follow the timeline specified below March: 11th March: Create your groups for Project 2 and email me the member names. March: 25th March Data collection should be finished (if you are collecting your own data). Last date to discuss with me issues related to your dataset. To discuss these issues write a one page clear description of the question that you want to answer using your data. This write up should contain a detailed description of each of your variables. Prior to meeting with me also send me a copy of your full dataset by email. March: 30th March: Last date to inform me about problems within the group. th April Last date to discuss with me any issues with your estimation results or 8 April: their interpretation. advice: A Strong word of advice Do not break the project into parts and assign each part to a group member. This is always the major source of acrimony, animosity and tears during the semester. Fix a time by consensus for each week when your entire group meets and work together on the project by bringing in your collective understanding and problem solving abilities. OPTION A: Gender and Racial differences in payments in federal jobs Mr. Cartwright is the director of the Office of Personnel and Management (OPM). One employee recently complained to him that even in Federal jobs there exist unexplained salary differences between male and female employees and employees of different racial background. Mr. Cartwright is of the opinion that educational and other 'quality' measures are reasons of such differences and there is no gender or race bias in the federal salaries. He has asked you, the brilliant analysts from MGS 3100 to create a regression model to prove or disprove his claim. He has supplied you with the dataset salary_federal.xls which contains the salary and other information about some randomly sampled federal employees whose names are withheld for reasons of anonymity and to protect their privacy. Your task is to create a regression model that contains at least FIVE (more would be better, but five is the minimum necessary) explanatory variables (that explain/predict) salary of these employees. From this regression model you have to conclude whether you have found any evidence to support the claim of gender and 1 racial discrimination in federal payment structure or not. Description of the dataset is provided at the end of this document. (If OPTION B (If done correctly choosing this option will reward you with points. bonus 10 points. But if done wrong - wrong data, wrong dependent twice.) variable etc. - you may have to do the project twice.) Collect non-time-series data (to be specific, cross section data) on any topic of interest to you (sports, business, banking, finance, government etc. etc.), preferably something related to your work (application of the techniques from this course to your work will add value to your organization and to you.) You must have at least 5 independent variables (can be a mix of categorical and numeric) in your final model and a dependent variable (numeric). The number of observations will depend on the circumstances. However, there should be at least 50 observations. Some interesting starter questions (each can be used as a unique starting point for your project and data collection, but chose just one, no mixing) may be a. What properties/factors of a baseball team (or Football or Soccer or Basketball or Ice Hockey etc.) predict and explain its success over the course of a season? [Here you need to collect data about several teams playing the same sports, and your dependent variable should be the percentage of wins of each team in a particular season.] OR b. What properties/factors of a college are related to its rank in the US News and World Report Ranking in a particular year? OR c. What are the properties/factors of a city to which the unemployment rate (or crime rate) in that city is related to? OR d. What properties/factors (from the previous season) affect the salary of players (NFL or baseball etc.)? OR e. What factors affect the prices of cars/houses? What you need to do in your project as part of the data analysis (whether you choose Option A or Option B) 1. Show descriptive statistics of all the variables. [to get some feel for the data]. Descriptive statistics are average or mean, standard deviation, maximum, minimum, etc. 2 2. Show relationships of each independent variable individually with the dependent variable using scatter plots. Remember to correctly label the horizontal and vertical axes in each diagram. [this is to get some initial feeling about which variables are more related to the dependent variables, and look for possible outliers or influence points] (In Excel: Insert - Charts - Scatter) 3. Perform regression analysis to show overall model for predicting the value of the dependent variable. (You might need to activate the Analysis toolpack Excel plug-in/addin in your Excel. Once you have done that regression can be run by Data - Analysis - Regression). But as we discussed in class, the first model that you estimate is most likely not be your final model. You will need to drop (sometimes add as well) bad variables from the model and reestimate the model again. This is a crucial step. Do not ask me how many times you should reestimate or how many models should be there - there is no answer to that. What I want to see indication of the fact that you have understood the idea of how regression analysis is done through trial and error of including and dropping of variables. So start with a handful (much more than 5) of variables that can be expected to affect your dependent variable. Then drop the ones that does not seem good after you do your initial regression or regressions. This is an iterative process and will take both time and patience. We can talk more about this step during office hour. 4. Interpret the results and write a report using those interpretations that conforms to the sample memo format. Submittals: Report - Single spaced one or two pages Word document (do not embed in Excel) that conforms to the sample memo format and contains: Introduction should contain 1. Why you are interested in the topic: the background of your project 2. What you are trying to predict - your dependent variable. 3. Why you choose certain independent variables for your project. Why do you think those independent or explanatory variables are going to affect the dependent of explained variable? [Logical explanation is required.] 4. How you collected the data (e.g., survey or from Web or from some book) [You should clearly write the source of the data.] Analysis [do not insert any graph or diagram] 3 5. Findings from descriptive statistics and scatter plot [It does not have to be thorough; write what variables are expected to have relationship with the dependent variable.] 6. List of insignificant independent variables in a full model (i.e., a model with all the independent variables) 7. Order of the dropped independent variables in a subsequent regression analyses with reasoning of such order 8. Equation of the final model (i.e., a model with only significant independent variable(s)) 9. Performance of the final model. i.e., how good your model is? Look at your F-significance number and R-squared value for answering this. [Low R-squared does not mean low grade.] 10. Findings from the final model. i.e., interpretation of the coefficients of the independent variables [Make sure that all the coefficients make sense. If it does not, explain further how such odd coefficient can be justified] Excel file Your excel file should have raw data and outputs of all the regression analyses you did. Each worksheet should be clearly named "raw data," "scatter plot," "regression 1, (Full Model)" "regression 2," and so on... finishing with \"Final Regression\". But note that I am not saying you will run three regressions only (Full Model, Regression 2, and final model). You may need more depending on the iterative process that I discussed in point 3 above. [Example] Your report should be written clearly about what you want to do in the project, what you have found, and how you have found. Your report should be easy to read and understand without referring to your Excel file. Email your project to me using the email address specified in the syllabus (kkarmakar1@gsu.edu). Only one submission per group is required. The submitted subm MS Word/Excel/PDF files must contain the full name of all the group members. members Moreover, when emailing me the documents, also forward the same to all of your group members. I will not accept any submission if these two points are not adhered penalties. to. In such cases your submission will incur late penalties. In other words, the submission email that I receive, must contain the email address of all your group members in the \"To\" address field. 4 Grading Rubric to be added soon. Data Set: salary_federal.xls This is a dataset of 523 federal employees. The following variables are there in the dataset. sal: Salary in $. grade: job grade (General Schedule and Equivalent Grade) patco: occupation category (only two categories are present in this dataset) major: In what subject did the employee majored in his/her bachelor's degree age: age in years male: male or female vet: veteran or not *yea-no) yos: years of service edyrs: Completed years of education. promo: was the employee promoted in the immediately preceeding year (yes-no) supmgr: Job position is of Supervisor or Manager type (yes-no) race: white or black To complete the analysis for Project 2 you have to do several things with this dataset. 1. First, you need to decide which variables you want to include in your model. Remember that your final model must have at least four explanatory variables. So your starting point should be more than four variables. 2. Second, several of the variables contain textual information. In the language of statistical packages such variables are called 'string' variable (originates from 'string of characters'). Such variables cannot be used directly into regression. You have to convert them into numeric variables. For example the male variable contains the two words 'male' and 'female' as its values. You have to convert the male variable into a numeric variable (say, male_numeric) which has only 0 and 1 as values. Where 0 means 'female' and 1 means 'male'. You have to do similar conversion for several other variables which takes only two values to prepare the data for analysis. [hint: =IF() function in Excel will be useful] 3. Third, several of the variables contain missing values - empty cells. Excel (unlike more advanced statistical packages) cannot handle such missing values in regression analysis. So you have to drop (delete) the observations (the complete row) that contain such missing values. [hint: do not try to manually search and delete these observations. Think carefully how you can bring all the missing observations on a particular variable on either top of the dataset or at the bottom of the dataset. You have to do this one variable at a time - but for only those variables that will be there in the regression analysis. If a variable is not going to be in the regression, there is no need to delete the entire observations for missing values on that variable.] 5 4. Once all the above steps have been completed only then your data is suitable for a regression analysis (in Excel). Now, copy the appropriate variables (that you want in your regression model to start with) into a new worksheet and start your regression analysis. However, do not forget point 1 above. 6
Step by Step Solution
There are 3 Steps involved in it
Step: 1
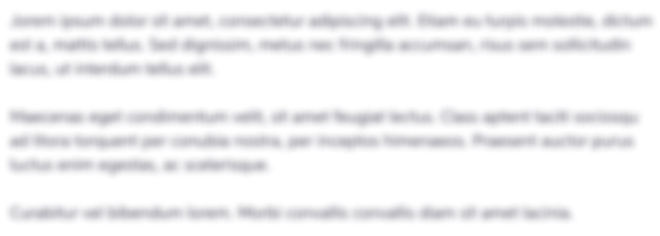
Get Instant Access to Expert-Tailored Solutions
See step-by-step solutions with expert insights and AI powered tools for academic success
Step: 2

Step: 3

Ace Your Homework with AI
Get the answers you need in no time with our AI-driven, step-by-step assistance
Get Started