Question
Modify the EM implementation (see the notebook file EM_algorithm.ipynb shared with you on the google drive: https://drive.google.com/drive/folders/1_rehaUOvRAp_uKK3_A2Hqak1BI8qIfQ L?usp=share_link) to allow for three classes (each following
Modify the EM implementation (see the notebook file EM_algorithm.ipynb shared with you on the google drive: https://drive.google.com/drive/folders/1_rehaUOvRAp_uKK3_A2Hqak1BI8qIfQ L?usp=share_link) to allow for three classes (each following a Gaussian distribution with different mean and standard deviation) in the data. You should modify the code to generate three classes of the data and use the EM algorithm to learn the parameters for the three Gaussian distribution. You should submit your modified implementation in a notebook file through canvas, which should include the implementation as well as a short description of your simulation result.
HERE IS CODE
-----------------------------------------------------------
import numpy as np
import matplotlib.pyplot as plt
# set random seed so that everytime we get the same result
np.random.seed(1)
# prepare simulation data
N1 = 1000
N2 = 1000
N = N1 + N2
real_mu1 = 0.2
real_std1 = 1
real_mu2 = 0.8
real_std2 = 1
y = np.concatenate((np.random.normal(real_mu1, real_std1, N1), np.random.normal(real_mu2, real_std2, N2)))
# EM algorithm
# Initialization
nits = 1000
count = 0 # should be initialized as 0
p1 = 0.5
p2 = 0.5
mu1 = np.random.random()
mu2 = np.random.random()
s1 = np.std(y)
s2 = s1
ll = np.zeros(nits)
gamma1 = np.zeros(N)
gamma2 = np.zeros(N)
while count < nits:
count = count + 1
# E-step
for i in range(N):
num1 = p1 * np.exp(-(y[i]-mu1)**2/(2*s1)) / np.sqrt(s1)
num2 = p2 * np.exp(-(y[i]-mu2)**2/(2*s2)) / np.sqrt(s2)
gamma1[i] = num1 / (num1 + num2)
gamma2[i] = num2 / (num1 + num2)
# M-step
mu1 = np.sum(gamma1*y) / np.sum(gamma1)
mu2 = np.sum(gamma2*y) / np.sum(gamma2)
s1 = np.sum(gamma1*(y-mu1)**2) / np.sum(gamma1)
s2 = np.sum(gamma2*(y-mu2)**2) / np.sum(gamma2)
p1 = np.sum(gamma1) / N
p2 = np.sum(gamma2) / N
ll[count - 1] = np.sum(np.log(p1*np.exp(-(y-mu1)**2/(2*s1)) / np.sqrt(s1) + p2*np.exp(-(y-mu2)**2/(2*s2)) / np.sqrt(s2)))
print('Estimated (mu1, sigma1): (%s, %s), (mu2, sigma2): (%s, %s)' % (mu1, s1, mu2, s2))
plt.plot(range(nits), ll)
Step by Step Solution
There are 3 Steps involved in it
Step: 1
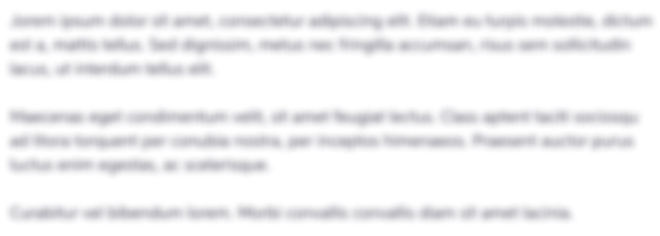
Get Instant Access to Expert-Tailored Solutions
See step-by-step solutions with expert insights and AI powered tools for academic success
Step: 2

Step: 3

Ace Your Homework with AI
Get the answers you need in no time with our AI-driven, step-by-step assistance
Get Started