Question
NCG deep learning is available on services from Amazon, Google, IBM and Microsoft. In Japan, Fujitsu announced a deep learning system for Riken, a privately
NCG deep learning is available on services from Amazon, Google, IBM and Microsoft. In Japan, Fujitsu announced a deep learning system for Riken, a privately held Japanese research foundation, which in terms of operations will be one of the largest-scale supercomputers in Japan to accelerate research and development in AI technology. On the software side, the Google Brain Team has contributed significantly to conduct machine learning and deep neural networks research with the open source TensorFlow software. The flexible architecture runs on one or more CPUs or GPUs in a desktop, server or mobile device with a single API. The system underpins a range of industry-specific deep learning offerings. In the legal field, Intraspexion uses TensorFlow as the core of an early warning system to investigate and prevent potential litigation. Cognitive analytics Cognitive analytics relies on processing and storage combinations to mimic the human brain in making deductions from vast amounts of data. This brings us back to the world of the mainframe and the supercomputer. Recently, the US Air Force and IBM announced a plan to build the world's first cognitive analytics supercomputer to enable analysis of multiple big data feeds (audio, video or text). It will run on an array of 64 TrueNorth Neurosynaptic processors. Each core is part of a distributed network and operates in parallel on an event-driven basis, not clock-based like conventional CPUs. The processing power is equivalent to 64 million neurons and 16 billion synapses. Estimates of the average human brain indicate 100 billion neurons and 100 trillion synapses - so cognitive analytics is still in its infancy. Commercially, the financial sector is at the forefront of cognitive analytics adoption and financial services company Opimas predicts that in 2017, finance firms in the investment sector will spend $1.5bn on robotic process automation, machine learning, deep learning and cognitive analytics, with that sum increasing by 75% to $2.8bn in 2021. Caveats in data management Given the breadth of data management issues, overall maturity is a slow process. Driving forces include different technologies, platforms, and capabilities that influence how this field is practised. Continued use of SQL (and NoSQL for unstructured data) to access new data storage and data structures is essential to ensuring easy access for developers, adoption affordability, and integration with existing enterprise infrastructure. The NoSQL scalability is crucial, as is the ability to enhance corporate data management strategies. Likewise, open source developments in Hadoop for big data and Spark for processing will enable companies to expand their data management functionality, while reducing costs. Their focus can then be on business issues rather than technology. With huge repositories of unstructured big data, especially in data lakes, the need for consistent frameworks and data governance increases. Companies need to develop business rules and glossaries, and clearly identify governance responsibility roles, before making seismic changes to their data management.
What do you believe are the future trends of data management?
Step by Step Solution
There are 3 Steps involved in it
Step: 1
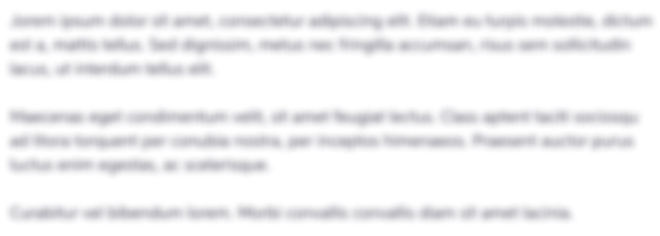
Get Instant Access to Expert-Tailored Solutions
See step-by-step solutions with expert insights and AI powered tools for academic success
Step: 2

Step: 3

Ace Your Homework with AI
Get the answers you need in no time with our AI-driven, step-by-step assistance
Get Started