Question
Needed solution in RStudio. Wine Quality Testing fixed acidity,volatile acidity,citric acid,residual sugar,chlorides,free sulfur dioxide,total sulfur dioxide,density,pH,sulphates,alcohol,quality 6.6,0.26,0.31,4.8,0.138,41,168,0.9951,3.2,0.38,9.3,Bad 7.6,0.36,0.49,11.3,0.046,87,221,0.9984,3.01,0.43,9.2,Bad 6.7,0.41,0.24,5.4,0.035,33,115,0.9901,3.12,0.44,12.8933333333,Good 7.3,0.19,0.27,13.9,0.057,45,155,0.99807,2.94,0.41,8.8,Good 5.7,0.29,0.16,7.9,0.044,48,197,0.99512,3.21,0.36,9.4,Bad 6.3,0.37,0.28,6.3,0.034,45,152,0.9921,3.29,0.46,11.6,Good 9,0.55,0.3,8.1,0.026,14,71,0.993,2.94,0.36,11.8,Bad 6.5,0.32,0.12,11.5,0.033,35,165,0.9974,3.22,0.32,9,Bad 6.2,0.25,0.47,11.6,0.048,62,210,0.9968,3.19,0.5,9.5,Bad
Needed solution in RStudio.
Wine Quality Testing
fixed acidity,volatile acidity,citric acid,residual sugar,chlorides,free sulfur dioxide,total sulfur dioxide,density,pH,sulphates,alcohol,quality 6.6,0.26,0.31,4.8,0.138,41,168,0.9951,3.2,0.38,9.3,Bad 7.6,0.36,0.49,11.3,0.046,87,221,0.9984,3.01,0.43,9.2,Bad 6.7,0.41,0.24,5.4,0.035,33,115,0.9901,3.12,0.44,12.8933333333,Good 7.3,0.19,0.27,13.9,0.057,45,155,0.99807,2.94,0.41,8.8,Good 5.7,0.29,0.16,7.9,0.044,48,197,0.99512,3.21,0.36,9.4,Bad 6.3,0.37,0.28,6.3,0.034,45,152,0.9921,3.29,0.46,11.6,Good 9,0.55,0.3,8.1,0.026,14,71,0.993,2.94,0.36,11.8,Bad 6.5,0.32,0.12,11.5,0.033,35,165,0.9974,3.22,0.32,9,Bad 6.2,0.25,0.47,11.6,0.048,62,210,0.9968,3.19,0.5,9.5,Bad 7.1,0.28,0.44,1.8,0.032,32,107,0.9907,3.25,0.48,12.2,Good 6.9,0.54,0.26,12.7,0.049,59,195,0.99596,3.26,0.54,10.5,Good 7.1,0.32,0.4,1.5,0.034,13,84,0.9944,3.42,0.6,10.4,Bad 7,0.26,0.26,10.8,0.039,37,184,0.99787,3.47,0.58,10.3,Good 5.8,0.13,0.22,12.7,0.058,24,183,0.9956,3.32,0.42,11.7,Good 7,0.39,0.31,5.3,0.169,32,162,0.9965,3.2,0.48,9.4,Bad 7.5,0.41,0.23,14.8,0.054,28,174,0.99898,3.18,0.49,9.7,Bad 8.3,0.21,0.49,19.8,0.054,50,231,1.0012,2.99,0.54,9.2,Bad 6.7,0.12,0.36,2.3,0.039,43,125,0.99229,3.07,0.67,10.1,Good 7.6,0.18,0.49,18.05,0.046,36,158,0.9996,3.06,0.41,9.2,Bad 6.7,0.28,0.28,2.4,0.012,36,100,0.99064,3.26,0.39,11.7,Good 8.1,0.45,0.34,8.3,0.037,33,216,0.9976,3.31,0.64,9.7,Bad 7,0.35,0.24,1.9,0.04,21,144,0.9923,3.35,0.38,11,Bad 8.6,0.18,0.28,0.8,0.032,25,78,0.99104,2.99,0.38,11.1,Bad 6.4,0.29,0.2,15.6,0.04,20,142,0.9962,3.1,0.54,10.6,Bad 6.2,0.29,0.32,3.6,0.026,39,138,0.9892,3.31,0.37,13.1,Good 9.6,0.23,0.4,1.5,0.044,19,135,0.9937,2.96,0.49,10.9,Bad 6.8,0.31,0.32,7.6,0.052,35,143,0.9959,3.14,0.38,9,Bad 6.5,0.36,0.31,13.55,0.053,20,113,0.99544,3.2,0.56,11,Good
Wine Quality Training
fixed acidity,volatile acidity,citric acid,residual sugar,chlorides,free sulfur dioxide,total sulfur dioxide,density,pH,sulphates,alcohol,quality 9.1,0.27,0.45,10.6,0.035,28,124,0.997,3.2,0.46,10.4,Good 6.6,0.36,0.29,1.6,0.021,24,85,0.98965,3.41,0.61,12.4,Good 7.4,0.24,0.36,2,0.031,27,139,0.99055,3.28,0.48,12.5,Good 7.1,0.26,0.49,2.2,0.032,31,113,0.9903,3.37,0.42,12.9,Good 6.2,0.66,0.48,1.2,0.029,29,75,0.9892,3.33,0.39,12.8,Good 6.2,0.66,0.48,1.2,0.029,29,75,0.9892,3.33,0.39,12.8,Good 6.8,0.26,0.42,1.7,0.049,41,122,0.993,3.47,0.48,10.5,Good 6.7,0.23,0.31,2.1,0.046,30,96,0.9926,3.33,0.64,10.7,Good 5.2,0.44,0.04,1.4,0.036,43,119,0.9894,3.36,0.33,12.1,Good 5.2,0.44,0.04,1.4,0.036,43,119,0.9894,3.36,0.33,12.1,Good 6.8,0.53,0.35,3.8,0.034,26,109,0.9906,3.26,0.57,12.7,Good 7,0.24,0.36,2.8,0.034,22,112,0.99,3.19,0.38,12.6,Good 6.1,0.31,0.58,5,0.039,36,114,0.9909,3.3,0.6,12.3,Good 5,0.55,0.14,8.3,0.032,35,164,0.9918,3.53,0.51,12.5,Good 6,0.25,0.28,2.2,0.026,54,126,0.9898,3.43,0.65,12.9,Good 5.9,0.27,0.29,11.4,0.036,31,115,0.9949,3.35,0.48,10.5,Good 6.5,0.36,0.28,3.2,0.037,29,119,0.9908,3.25,0.65,12.4,Good 6.2,0.24,0.29,13.3,0.039,49,130,0.9952,3.33,0.46,11,Good 7.1,0.32,0.34,14.5,0.039,46,150,0.995,3.38,0.5,12.5,Good 6.6,0.33,0.31,1.3,0.02,29,89,0.99035,3.26,0.44,12.4,Good 8.1,0.17,0.44,14.1,0.053,43,145,1.0006,3.28,0.75,8.8,Good 8.1,0.17,0.44,14.1,0.053,43,145,1.0006,3.28,0.75,8.8,Good 6,0.18,0.31,1.4,0.036,14,75,0.99085,3.34,0.58,11.1,Good 7.5,0.42,0.34,4.3,0.04,34,108,0.99155,3.14,0.45,12.8,Good 7.3,0.25,0.36,2.1,0.034,30,177,0.99085,3.25,0.4,11.9,Good 7.3,0.25,0.36,2.1,0.034,30,177,0.99085,3.25,0.4,11.9,Good 7.3,0.25,0.36,2.1,0.034,30,177,0.99085,3.25,0.4,11.9,Good 7.3,0.21,0.29,1.6,0.034,29,118,0.9917,3.3,0.5,11,Good
3. Predicting wine quality by using support vector machine classification algorithm. (a) Download the full wine quality training and testing datasets from Moodle, and use the training dataset to find out the optimal value of hyperparameter C for a linear kernel-based svm. (b) Train a svm classifier by using the linear kernel and the corresponding optimal value of hyperparameter C, then make predictions on the testing dataset, report the classification accuracy. (c) Use the training dataset to find out the optimal values of hyperparameters C and y for an RBF kernel-based svm. (d) Train a svm classifier by using the RBF kernel and the corresponding optimal values of hyperparameters C and 7, then make predictions on the testing dataset, report the classification accuracy. (e) Train a logistic regression model. Then use the testing dataset to conduct an ROC curve analysis to compare the predictive performance of the trained logistic regression model and those two svm classifiers trained by using linear and RBF kernels respectively. Hint: Define set.seed (1). Given a pre-defined hyperparameter space - C: (0.01, 0.1, 1, 10, 100), and 7: (0.01, 0.1, 1, 10, 100). 3. Predicting wine quality by using support vector machine classification algorithm. (a) Download the full wine quality training and testing datasets from Moodle, and use the training dataset to find out the optimal value of hyperparameter C for a linear kernel-based svm. (b) Train a svm classifier by using the linear kernel and the corresponding optimal value of hyperparameter C, then make predictions on the testing dataset, report the classification accuracy. (c) Use the training dataset to find out the optimal values of hyperparameters C and y for an RBF kernel-based svm. (d) Train a svm classifier by using the RBF kernel and the corresponding optimal values of hyperparameters C and 7, then make predictions on the testing dataset, report the classification accuracy. (e) Train a logistic regression model. Then use the testing dataset to conduct an ROC curve analysis to compare the predictive performance of the trained logistic regression model and those two svm classifiers trained by using linear and RBF kernels respectively. Hint: Define set.seed (1). Given a pre-defined hyperparameter space - C: (0.01, 0.1, 1, 10, 100), and 7: (0.01, 0.1, 1, 10, 100)Step by Step Solution
There are 3 Steps involved in it
Step: 1
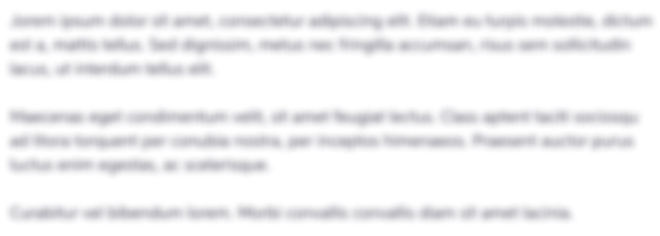
Get Instant Access to Expert-Tailored Solutions
See step-by-step solutions with expert insights and AI powered tools for academic success
Step: 2

Step: 3

Ace Your Homework with AI
Get the answers you need in no time with our AI-driven, step-by-step assistance
Get Started