Answered step by step
Verified Expert Solution
Question
1 Approved Answer
Needour task is to create a visual report for these data. You will create a single Matplotlib Figure containing the following subplots: A scatter plot
Needour task is to create a visual report for these data. You will create a single Matplotlib Figure containing the following subplots:
A scatter plot with allweights on the xaxis and allmpgs on the yaxis
A line plot with uniquemodelyears with separate yaxes for yearlymeanmpgs and yearlymeanhorsepower. Include markers for each data point.
Two pie charts, one each for the number of models by origin in origins and origins
A series of bar plots with originmeanmpgs originmeanhorsepower, originmeancylinders, originmeandisplacement, originmeanweight, and originmeanacceleration on yaxis, and each should have uniqueorigins on the xaxis.
The subplots should use a layout with rows:
Row three subplots: Scatter plot item above pie charts item
Row subplot stretched across the figure: Line plot item
Row subplots: bar plots item
Be sure to give each subplot a title and label the axes with appropriate descriptor and units. You may customize the appearance colors marker types, etc. in any way you think looks appealing to be written with python code, and here is the start codeimport pandas as pd
import seaborn as sns
data snsloaddatasetmpg
allmpgs datampgtolist
allweights dataweighttolist
uniquemodelyears listdatamodelyear'unique
uniqueorigins sortedlistdataoriginunique
yearlymeanmpgs data.groupbymodelyear'meannumericonlyTruempgtolist
yearlymeanhorsepower data.groupbymodelyear'meannumericonlyTruehorsepowertolist
origins data.locdatamodelyear' 'origin'
origins data.locdatamodelyear' 'origin'
originmeanmpgs data.groupbyoriginmeannumericonlyTruempgtolist
originmeanhorsepower data.groupbyoriginmeannumericonlyTruehorsepowertolist
originmeancylinders data.groupbyoriginmeannumericonlyTruecylinderstolist
originmeandisplacement data.groupbyoriginmeannumericonlyTruedisplacementtolist
originmeanweight data.groupbyoriginmeannumericonlyTrueweighttolist
originmeanacceleration data.groupbyoriginmeannumericonlyTrueaccelerationtolist
Step by Step Solution
There are 3 Steps involved in it
Step: 1
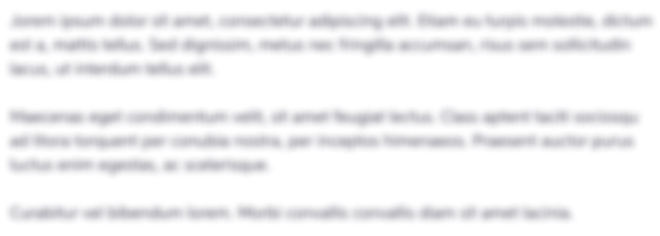
Get Instant Access to Expert-Tailored Solutions
See step-by-step solutions with expert insights and AI powered tools for academic success
Step: 2

Step: 3

Ace Your Homework with AI
Get the answers you need in no time with our AI-driven, step-by-step assistance
Get Started