Answered step by step
Verified Expert Solution
Question
1 Approved Answer
No dataset provided. Based on the question asked you should still be able to fill in the correct code. In python please [10 points] Problem
No dataset provided. Based on the question asked you should still be able to fill in the correct code. In python please
[10 points] Problem 2 - Building a Naive Bayes Classifier A sample dataset has been provided to you in the '/data/dataset.csv" path. Here are the attributes for the dataset. Use this dataset to test your functions. Age - ["40") Income - ["low", "medium", "high"] Student - ['no". "yes"] Credit Rating - ["fair", 'excellent") Loan - ['no", "yes"] . Note: A sample dataset to test your code has been provided in the location "data/dataset.csv". Please maintain this as it would be necessary while grading. Do not change the variable names of the returned values. After calculating each of those values, assign them to the corresponding value that is being returned. The 'Loan' attribute should be used as the target variable while making calculations for your naive bayes classifier. : from collections import defaultdiet def naive_bayes (dataset_file, attributes, attribute_values): Input: 1. dataset_file - A string variable which references the path to the dataset file. 2. attributes - A python list which has all the attributes of the dataset 3. attribute_values - A python dictionary representing the values each attribute can hold. Output: A proabbilities dictionary which contains the counts of when the Loan target variable is yes or no depending on the input attribute. Hint: Starter code has been provided to you to calculate the counts. Your code is very similar to the previous prot probabilities - "Age": { 40": {"yes": 0, "no": 0) }, "Income": { "low": {"yes": 0, "no":0}, "medium": {"yes": 0, "no": 0, "high": {"yes": 0, "no":0}}, "Student": { "yes" : {"yes": 0, "no":0},"no": {"yes": 0, "no":0} }, "Credit Rating": { "fair". ("yes": 0, "no":0}, "excellent": {"yes": 0, "no": 0) }, "Loan": {"yes": 0, "no": 0) > df - pd.read_csv (dataset_file) d_range - len(df) vcount - df [ "Loan").value_counts() vcount_loan_yes - veount [ "yes" ] vcount_loan_no - veount("no"] probabilities("Loan" ( "yes") - vcount_loan_yes/d_range probabilities("Loan"]"no") - vcount_loan_no/d_range for attribute in attributes: value_counts - dict() vcount - df[ attribute).value_counts() for att_value in attribute_values[attribute): your code here [10 points] Problem 2 - Building a Naive Bayes Classifier A sample dataset has been provided to you in the '/data/dataset.csv" path. Here are the attributes for the dataset. Use this dataset to test your functions. Age - ["40") Income - ["low", "medium", "high"] Student - ['no". "yes"] Credit Rating - ["fair", 'excellent") Loan - ['no", "yes"] . Note: A sample dataset to test your code has been provided in the location "data/dataset.csv". Please maintain this as it would be necessary while grading. Do not change the variable names of the returned values. After calculating each of those values, assign them to the corresponding value that is being returned. The 'Loan' attribute should be used as the target variable while making calculations for your naive bayes classifier. : from collections import defaultdiet def naive_bayes (dataset_file, attributes, attribute_values): Input: 1. dataset_file - A string variable which references the path to the dataset file. 2. attributes - A python list which has all the attributes of the dataset 3. attribute_values - A python dictionary representing the values each attribute can hold. Output: A proabbilities dictionary which contains the counts of when the Loan target variable is yes or no depending on the input attribute. Hint: Starter code has been provided to you to calculate the counts. Your code is very similar to the previous prot probabilities - "Age": { 40": {"yes": 0, "no": 0) }, "Income": { "low": {"yes": 0, "no":0}, "medium": {"yes": 0, "no": 0, "high": {"yes": 0, "no":0}}, "Student": { "yes" : {"yes": 0, "no":0},"no": {"yes": 0, "no":0} }, "Credit Rating": { "fair". ("yes": 0, "no":0}, "excellent": {"yes": 0, "no": 0) }, "Loan": {"yes": 0, "no": 0) > df - pd.read_csv (dataset_file) d_range - len(df) vcount - df [ "Loan").value_counts() vcount_loan_yes - veount [ "yes" ] vcount_loan_no - veount("no"] probabilities("Loan" ( "yes") - vcount_loan_yes/d_range probabilities("Loan"]"no") - vcount_loan_no/d_range for attribute in attributes: value_counts - dict() vcount - df[ attribute).value_counts() for att_value in attribute_values[attribute): your code hereStep by Step Solution
There are 3 Steps involved in it
Step: 1
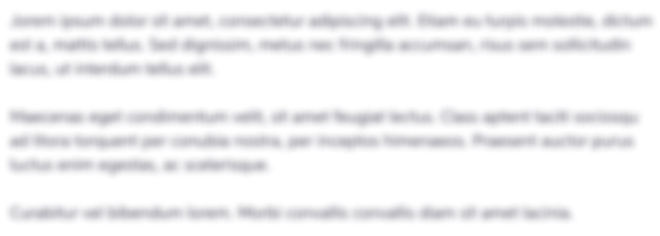
Get Instant Access to Expert-Tailored Solutions
See step-by-step solutions with expert insights and AI powered tools for academic success
Step: 2

Step: 3

Ace Your Homework with AI
Get the answers you need in no time with our AI-driven, step-by-step assistance
Get Started