Question
Note: Summarize it with your own wording. Macroeconomic factors explaining stock volatility: multi-country empirical evidence from the auto industry Jana Vychytilov, Drahomra Pavelkov, Ha Pham
Note: Summarize it with your own wording.
Macroeconomic factors explaining stock volatility: multi-country empirical evidence from the auto industry
Jana Vychytilov, Drahomra Pavelkov, Ha Pham & Tom
ABSTRACT
This paper aims to explore which macroeconomic factors affect the volatility of the automakers stock prices by employing a multifactor model. The study uses quarterly panel data of 39 automakers quoted on the stock exchanges in the 11 countries. It studies the effects of 19 macroeconomic variables from January 2000 to December 2017, and proposes the mixed-effect model constructed based on employing genetic algorithm and AIC criterion, and compares its explanatory power with the existing multifactor model (El Khoury, 2015). This paper suggests that the proposed model can shed more light on explaining the variability of stock prices of the quoted automakers. The findings show there are positive linkages between automaker's stock return volatility and explanatory variables such as stock market development, GDP and unemployment. Conversely, an inverse linkage between the dependent variable and money supply and IPI was found. The study demonstrates that selected macroeconomic factors can also be used as predictors.
1. Introduction
The car industry is an important sector of the global economy. This sector contributes by almost 3 percent of the global GDP with sales reaching a record of 88 million cars in 2016. The profit margins of suppliers and automakers are at 10-year-high levels, with capital spending (R&D) reaching the level of 95 billion USD in 2016.1 The car industry is a very progressive sector. According to Cosentino (2009) the environmental regulations have been tightening at the beginning of the third millennium, pushing auto industry into the period of transformative change. Moreover, the key innovations are driven by Industry 4.0 principles (such as diverse mobility, electrified vehicles, connectivity and IoT solutions, self-driving and autonomous driving technology, big data value creation, digitalisation of manufacturing processes and faster construction cycles using cloud manufacturing and smart automatisation, cobotisation, mass customisation oriented on customers and caring of customers in real time over the whole vehicle lifetimes, falling margins
from sales of vehicles run on diesel, changing global value chain collaborating with new sectors such as healthcare, insurance and ICT firms, multi-tier partnership, and more recent trends transforming the auto industry in the recent years).1
Beginning with Chen, Roll, and Ross (1986), various papers have tried to identify associations between macroeconomic variables and changing stock prices (Flannery & Protopapadakis, 2002). However, Bilson, Brailsford, and Hooper (2001) point out that the potential impact of macroeconomic variables has not been still appropriately examined and, furthermore, add that the literature is primarily focused on microeconomic factors. The question of whether economic conditions can influence the real-investment opportunities available (Flannery & Protopapadakis, 2002) arose. Cheung and Ng (1998) and lately El Khoury (2015) state that the economic theory does not clearly state which factors and how many factors should be used to explain the stock volatility.
The current literature which deals with the impact of macroeconomic variables on stock prices volatility of automakers is mostly conducted as a single-country study.
While taking into account (i) the global importance of auto industry, widely considered as sensitive to economic conditions and entering a period of disruptive technological changes and environmental issues (ii) the absence of related cross-continental study examining changing stock prices in the macroeconomic context, this makes interesting to investigate this field. This paper aims to fill the abovementioned gap and contributes to the existing literature in several ways; firstly by selecting explanatory macroeconomic variables for the model that explains the stock volatility of automakers, using data of 39 automakers in 11 countries in the period of tightening environmental issues of 2000-2017; second by proposing a multifactor model consisting of macroeconomic variables that appropriately explain the stock volatility of automakers and by verifying its statistical significance. Finally, evidence of a higher explanatory power of the proposed model compared to the El Khoury's (2015) is presented. The El Khoury's model explains stock prices of nine European automakers by macroeconomic variables from 2003 to 2012, in which the automotive industry was not hit by disruptive technological changes and environmental issues.
Employing a mixed effect multifactor model and genetic algorithm, the study finds that the volatility of 39 auto stocks in 11 countries can be appropriately explained by five macroeconomic explanatory factors, such as GDP, unemployment, money supply, inflation measured by industrial production index and stock market index development. The empirical results can be of interest of stakeholders and shareholders of auto companies, academicians or policymakers.
The paper is organised as follows. Section 2 presents a comprehensive theoretical review with a discussion of macroeconomic variables and pricing models designed to macroeconomic factors affecting stock prices. The research methodology and data collection are described in Section 3. The research results and discussion of the empirical findings are provided in Section 4, and Section 5 concludes the paper.
2. Literature review
Macroeconomic variables are candidates for explaining stock prices movements as the macroeconomic changes simultaneously affect firms' cash flows and may influence the risk-adjusted discount rate (Flannery & Protopapadakis, 2002).
Factor models analyse sensitivity of asset returns as a function of one or more factors (Dubravka & Posedel, 2010). The existence of the relation between changing stock prices and one factor has been widely used since the proposed Sharpe-LintnerBlack SLB (CAPM) model. This model assumes that just one factor is significant. In particular it indicates a simple positive relation between average stock returns and the stock market, in the sense of Markowitz (1959). This approach was supported by Black, Jensen, and Scholes (1972), or Fama and MacBeth (1973).
However, lately SLB (CAPM) model was not fully supported by Fama and French (1989) who pointed out that this model has its limitations. Their tests do not support the basic assumption of the SLB model, that average stock returns are positively related to market betas. Since then, the attention of related studies has progressively moved to multifactor approach aiming to explain the sensitivity of stock prices movements as a function of more factors (Bilson et al., 2001; Chen et al., 1986; Cheung & Ng, 1998; Fama, 1981, 1990; Flannery & Protopapadakis, 2002; Rapach, Wohar, & Rangvid, 2005; Ross, 1976; among others); providing evidence that there is a linkage between changing stock prices and macroeconomic variables.
Ross (1976) proposed the arbitrage pricing theory and the arbitrage model (APT) as an alternative to the capital pricing model. Fama and French (1989) pointed out that the expected returns from changing stock prices are lower when economic conditions are strong and higher when conditions are weak. Fama (1990) further suggests that annual stock variances can be traced to forecasts of variables ("three proxies for equity variation") such as real-GNP industrial production, and investment that are important determinants of cash flows to firms; and declares that U.S. stock returns and its aggregate real activity were correlated. Meanwhile, Chen et al. (1986) hypothesise and evidence economic variables such as interest rates, inflation, industrial production or bond spreads as the significant factors for stock markets, in the APT context. They concluded that between macroeconomic variables and changing stock prices is a strong relationship, however, they stress that they cannot specify what macroeconomic factors are suitable for asset pricing, creating the core gap in the literature.
Cheung and Ng (1998) lately provide evidence of a long run co-movements among five stock indices (namely in Canada, Germany, Italy, Japan and U.S.) and countryspecific measures of aggregate real activity; such as the real oil price, real GNP, real money supply and real consumption, that was not fully captured previously by Fama (1990). Flannery and Protopapadakis (2002) then highlight that the changing stock prices are widely seen in the literature to that time as to be negatively correlated with inflation (CPI, PPI) and money growth, and support this finding empirically. They furthermore point out, that they have evidence of more candidates for priced factors, such as a balance of trade, employment or housing starts. Rapach et al. (2005) examines whether macroeconomic factors such as interest rates, inflation, industrial production, unemployment among other can predict stock returns in twelve countries. He emphasises that interest rates and inflation seem to be the significant factors, while contrary, the predictive ability of industrial production and the unemployment to assess changing stock prices is limited.
Since the third millennium various multifactor- based studies on examining relationships between stock prices and macroeconomic variables have been frequently published (Buy uksalvarci & Abdioglu, 2010; Czapkiewicz & Wojtowicz, 2014; Dubravka & Posedel, 2010; Hsing, 2011; Hsing & Hsieh, 2012; Khoon & Gupta, 2001; Kyereboah-Coleman & Agyire-Tettey, 2008; Maysami & Koh, 2000; Rjoub, Tursoy, & Gunsel, 2009; among others), majority of the papers has been conducted as singlecountry study.
Maysami and Koh (2000) suggest that inflation, money supply growth, interest rate, and variations in exchange rate do form a cointegrating relation with changes in Singapore's stock market levels. Khoon and Gupta (2001) attempt to examine APT by providing evidence from Malaysia and show that the APT model is quite robust, pointing out the importance of macroeconomic variables such as inflation. Al-Sharkas (2004) evidences long-term equilibrium relations between selected macroeconomic variables such as real-economic activity, money supply, inflation, and interest rate, on the Amman Stock Exchange. Kyereboah-Coleman and Agyire-Tettey (2008) find in Ghana that lending rates have an adverse effect on stock prices, while the inflation rate has a negative effect. Tursoy Gunsel, and Rjoub (2008) followed by Rjoub et al. (2009) test APT in Istanbul Stock Exchange, declaring a significant relationship between the changing stock prices and the selected and tested macroeconomic variables. Dubravka and Posedel (2010) propose that market index is highly important factor followed by interest rates, oil prices and industrial production with having a positive relation to changing stock prices in Croatian stock market, while inflation has a negative relation. Hsing (2011) examined the relationship between the Croatian stock market index and relevant macroeconomic variables such as real GDP, stock market development, government bond yield, real-interest rates, exchange rates or the expected inflation. Hsing and Hsieh (2012) suggest the Poland stock market is positively associated with industrial production, real GDP, stock market index, interest rate, nominal effective exchange rate, inflation rate, and government bond yield.
A very limited number of studies aiming to examine the stock volatility of the automotive industry by using macroeconomic variables is available (El Khoury, 2015; Kruger & Rhein, 2015; Mazzucato & Semmler, 1999). Mazzucato and Semmler (1999) examine whether there is a linkage between market share instability and stock price volatility in the U.S. automobile industry. However they conclude that the stock price volatility is indeed partly affected by industry-specific factors. Kruger and Rhein (2015) find out that macroeconomic factors like income, inflation, exports and interest rates can be suitable explanatory variables for the German automobile industry.
The paper of El Khoury (2015) is dedicated directly to automakers and investigates the changing stock prices using the multifactor model incorporating only macroeconomic variables. The six-factor model that explain changing stock prices of nine European automakers between January 2003 and April 2012 by studying 16 macroeconomic factors is proposed in this study, giving evidence that macroeconomic variables such as stock market index development, exchange rate, exports, platinum, aluminium, and unemployment can explain the stock variability of studied automakers. Furthermore, the study points out that the stock market index development, exchange rate, exports and platinum affect the changing stock prices positively, while aluminium and unemployment negatively. There are, however, some weak points in the mentioned study. For example, the OLS method which was used does neither take an individual firm nor the time effect into account. The study does not consider the country effect although the data come from several European countries.
The mentioned and the other related studies mentioning the macroeconomic factor(s) as the explanatory variable(s) of stock volatility are summarised in Appendix A. They serve as theory-based support for choosing the macroeconomic variables in this study to develop a model explaining automakers' stock volatility within the macroeconomic context. There is still no cross-continental empirical evidence based on the multifactor model explaining stock volatility of automakers using macroeconomic variables. This study seeks therefore to fill this knowledge gap by investigating whether, and if so, which macroeconomic factors significantly affect changing stock prices tested on 39 automakers across 11 countries, taking individual-firm effect, country effect and time effect into account; and to compare the explanatory power of the developed multifactor model based on using these macroeconomic variables with the model incorporating macroeconomic variables suggested as significant in the study of El Khoury (2015).
3. Data and methodology
3.1. Data
Average changes of the real-stock return volatility measured by standard deviation during a year were used as the dependent variable. Daily closing market prices of 39 automakers' stocks quoted in 11 countries (namely in Brazil, France, Germany, Hong Kong, China, India, Italy, Portugal, Singapore, Switzerland and the U.S.) were available and collected from Yahoo Finance Database for the whole period of 2000-2017. Quarterly standard deviations of daily returns (OEMSD) were computed as a proxy for risk to hold a stock.
Our main focus on the standard deviation of close prices for each country and manufacturer over a year was to select a set of macroeconomic variables which would allow us to investigate the stock volatility. The reason for using this measure is that there is no quarter to quarter data available for countries like China or Taiwan. The 19 macroeconomic variables chosen for this research to explain changing stock prices of automakers are based on the existing literature (see Appendix A). Macroeconomic data were sourced from various databases (see Appendix B).
The final dataset consists of 621 observations and 19 explanatory variables studied in 11 countries over the period of 2000-2017. This is an unbalanced panel as the several country-level observations are not available in every year in the studied period.
3.2. Methodology
The aim of this study is (i) to find out whether, and if so, what macroeconomic factors affect the volatility of stock prices of automakers, (ii) to propose a multifactor model that explains the volatility of stock prices of automakers by incorporating explanatory macroeconomic factors statistically significant, and iii) to test if the proposed model has higher explanatory power than the multifactor model proposed in this sector by El Khoury (2015).
Table 1. The settings of GA.
Parameter
Value
Generation
20
Population
50
Cr
0.8
F
0.1
Iteration
70
Source: Authorswork.
To identify significant macroeconomic factors, we conducted the following procedure. All 19 explanatory variables were normalised and then a feature selection algorithm was used. As the feature selection algorithm, we used genetic algorithm (GA) method (advocated as an adequate practical method for feature and model selection for prediction of financial time series by Atsalakis & Valavanis, 2009; Balcombe, 2005; Huang, 2012; Kapetanios, 2007; among others). The settings of the genetic algorithm can be seen in Table 1.
All results were cross-validated by 10-fold cross-validation. The 14 variables were preselected by GA. Then we used these 14 variables to make all combinations of fixed effects. From these combinations the model with the lowest AIC criterion was identified.
To propose a multifactor model that explains the volatility of stock prices of automakers by incorporating explanatory macroeconomic factors statistically significant, and to test its explanatory power, we constructed three mixed effect models. The first null model (nullModel) without explanatory variables was created for comparison purposes and to show that explanatory variables were statistically significant. This model serves as our null hypothesis. The second model (kbModel) was created based on the study of El Khoury (2015). Finally, the third model (fsModel) includes only explanatory variables selected by the abovementioned feature selection procedure. The hypothesis that fsModel has higher explanatory power than kbModel was tested by using the likelihood ratio test.
Furthermore, we used standard statistical test ANOVA and AIC information criterion to assess which model is statistically more significant. R software in version 3.5 with lme4 package version 1.1-12 and caret package version 6.0.81 were applied.
3.2.1 Economic model
We compare three mixed effect models in the following way. All models contain three levels. An automaker is considered as the first level, a country as the second level, and time as the third level. Time is used as a random slope, and country and automaker are random intercepts. We also used correlation structure AR(1) to model first-order autoregressive residuals. This setup was the same for all models for comparison purposes.
Yi, j, k b0, j, k b1, j, kx1b2, j, kx2 bn, j, kxn ei, j, k
with,
ei, j, k N0, X,(1)
Table 2. Descriptive statistics of dependent variable.
N
Mean
Sd
Min
Max
Range
SD
621
0.082
0.113
0
0.81
0.81
Source: Authorswork.
where Yijk is an average change of the market standard deviation during a year of an individual country and automaker, xn are explanatory variables (macroeconomic factors) which vary for each model, P is AR(1) correlation structure.
The first null model (nullModel) contains no explanatory variables. This model was created only for comparison purposes:
OEMSD b0, j, k ei, j, k(2)
The second mixed model (kbModel) was inspired by El Khoury (2015). This model suggests the presence of long run comovements between changing stock prices and macroeconomic variables such as stock market development, exchange rate, platinum, exports and aluminium:
OEMSD b0, j, k b1, j, kSPXb2, j, kERb3, j, k P b4, j, k Exp
(3)b5, j, kUnempb6, j, k A ei, j, k
The third - proposed feature selection-based model (fsModel) contains five explanatory macroeconomic variables selected from 19 macroeconomic variables by mentioned feature selection procedure:
OEMSD b0, j, k b1, j, kMb2, j, kGDPb3, j, kIPIb4, j, kUnemp
(4)b5, j, kSPX ei, j, k
4. Results and discussion
4.1. Results
The main aim of this paper is to test whether, and if so, what macroeconomic factors affect the volatility of stock prices of automakers; to propose a multifactor model that explains the volatility of stock prices of automakers by incorporating explanatory macroeconomic factors statistically significant; and to test its explanatory power.
We summarise the collected data in the form of descriptive statistics in Tables 2 and 3 and the findings in the remaining tables.
Table 2 shows the descriptive statistics for the dependent variable which is the yearly standard deviation of the auto market of automakers. Standard deviation range shows that this market is highly volatile.
Table 3 shows the descriptive statistics for explanatory macroeconomic variables. Some variables have a much higher mean than others (as, for example, M and NX). This is the reason why we normalised all variables for the upcoming feature selection procedure.
Table 3. Descriptive statistics of explanatory variables.
N
Mean
Sd
Min
Max
Range
O
M
GDP
C
CPI
IPI
Unemp
621
621
621
621
621
621
621
2.912
805.542
1.856 1.524 3.863 2.459
5.859
7.908
2758.376
2.569
2.72
3.308 4.045
2.766
13.451.2
5.55 8.15
3.69
17.5
1.9
15.975
16104.8
15.24
11.33
14.775 25
16.182
29.365 16156.04
20.788 19.477
18.465
42.5
14.282
Ex
Imp
NX
IR
G
S P
PA ST
A
SPX
621
621
621
621
621
621
621
621
621
621
621
5.683
5.004
24.59 1.439
2.336 2.514 2.383
3.564 2.51
0.717
0.011
8.51
8.068
186.124
38.278
3.989 6.393 6.589
11.361 5.85
4.624
0.004
18.114.79
292.52
255.695
9.09
10.165
9.715
15.287
4.61
6.84 0
31.432
35.809 2728.99
236.42
7.99
12.883 16.738 25.54
18.913
12.255
0.022
49.483
50.599 3021.51
492.115
17.078 23.047 26.453
40.828
23.518 19.09
0.022
ER
621
0.004
0.005
0
0.018
0.018
Source: Authorswork.
Table 4. The resampled explanatory variables from the feature selection procedure.
Fold01Fold02
Fold03
Fold04
Fold05
Fold06
Fold07
Fold08
Fold09
Fold10
MM
M
M
M
M
M
M
M
M
GDPGDP
GDP
GDP
GDP
GDP
GDP
GDP
GDP
GDP
CC
C
C
C
C
C
C
C
C
CPICPI
IPI
CPI
IPI
IPI
CPI
CPI
CPI
IPI
IPIO
O
IPI
Imp
Ex
Ex
IPI
IPI
Ex
ExIPI
Ex
Ex
NX
ER
Imp
Ex
Ex
ER
NXUnemp
Imp
Imp
Imp
GEx
ST
G
NX
ERImp
ER
ER
SPX
Source: Authorswork.
Table 4 shows selected variables during resampling for each fold. As can be seen, the most often selected variables were GDP, M, C and IPI. On average the GA selected approximately seven explanatory variables with min six and max nine variables. Average R2 during resampling was 0.39. Some explanatory variables such as A, PA, P, S and IR were not identified as significant macroeconomic factors explaining stock volatility of automakers.
Table 5 shows the ANOVA results with the likelihood ratio test for the proposed model and null model. These two models are nested thus we can calculate the likelihood ratio test. As can be seen the p-value is lower than 0.05. The statistically significant difference between these two models (nullModel, fsModel) is declared. Lower AIC can be found for fsModel, which means that this model is statistically more appropriate than nullModel, favouring our proposed model.
Table 6 shows the ANOVA results with the likelihood ratio test for the proposed model and model inspired by El Khoury (2015). The statistically significant difference between these two models (kbModel, fsModel) is declared. Lower AIC and df can be found for fsModel, which means that this model is statistically more appropriate than kbModel, favouring the explanatory power of our proposed model (fsModel).
Table 5. ANOVA results for comparing proposed mixed effect model (fsModel) with nullModel.
Model
df
AIC
BIC
logLik
Test
L.Ratio
p-value
nullModel fsModel
1
2
9
14
1422.21428.5
1382.41366.4
720.12
728.23
1 vs 2
16.229
0.00621
Source: Authorswork.
Table 6. ANOVA results for comparing proposed mixed effect model (fsModel) with kbModel.
Model
df
AIC
BIC
logLik
TestL.Ratio
p-value
kbModel 1 fsModel 2
15
14
1422.21428.5
1355.71366.4
726.1
728.2
1 vs 24.307
0.03796
Source: Authorswork.
Table 7. Fixed effects of our pr
oposed mode
l (fsModel).
Value
Std.Error
DF
t-value
p-value
(Intercept)0.0162571
0.0211744
577
0.767770
0.4429
M0.0000039
GDP0.0051352
0.0000033
0.0021341
577
577
1.1940612.406304
0.2329
0.0164
IPI0.0018897
Unemp0.0042851
0.0011117
0.0027842
577
577
1.6998611.539108
0.0897
0.1243
SPX2.0886598
0.6560219
577
3.183827
0.0015
Source: Authorswork.
In addition, the estimation results are also provided by Table 7 which shows the coefficient of fixed effects for the fsModel. We find a positive linkage between automaker's stock volatility and explanatory variables, such as SPX, GDP and Unemp. Conversely, we evidence an inverse linkage between the dependent variable and factors such as M and IPI.
All five explanatory variables selected by GA and considering the AIC criterion, incorporated in our proposed fsModel can potentially shed some more light as predictors in forecasting models of stock volatility of companies operating in the auto industry.
4.2. Discussion
There has been very little research done to explain stock volatility of automakers in the macroeconomic context while taking into account the global importance of auto industry, widely considered as sensitive to economic conditions and entering a period of disruptive technological changes and environmental issues.
In general, the empirical research on the multifactor approach and APT has grown since the pioneering work of Ross (1976). While the original asset pricing theory does not specify what the underlying economic forces are that drive the changing asset prices (Chen et al., 1986; Cheung & Ng, 1998; El Khoury, 2015; among others), it has been declared by various studies that the stock prices tend to fluctuate positively or negatively with economic indicators (Kyereboah-Coleman & Agyire-Tettey, 2008). Nevertheless, an appropriate number of explanatory factors incorporated in the multifactor model of changing asset prices is an important issue (Connor & Korajczyk, 1993).
We developed the five-factor mixed effect model (fsModel) consisting of macroeconomic factors explaining significantly the volatility of stock prices of automakers quoted in various countries (namely in Brazil, France, Germany, Hong Kong, China, India, Italy, Portugal, Singapore, Switzerland and US), providing a cross-continental empirical evidence. We finally found that the macroeconomic variables such as stock market development, GDP, unemployment, money aggregate and inflation measured by IPI are significant explanatory factors of stock volatility of automakers.
The number of factors incorporated in the proposed fsModel is consistent with the study of Trzcinka (1986) as the first five factors are the most distinct. Or, coherently with Connor and Korajczyk (1993) who defends in maximum up to six factors, by providing robust evidence on NYSE and AMEX stock exchanges.
Our findings about inflation and money growth affecting stock prices are consistent with the papers on factor models (for example Chen et al., 1986; Cheung & Ng, 1998; Flannery & Protopapadakis, 2002; Mukherjee & Naka, 1995; among others), highlighting these factors to be significantly correlated with changing stock prices. We documented in Table 6 that the changing stock prices negatively relate to inflation and money growth. This result is in accordance with the study of Flannery and Protopapadakis (2002). Another finding about GDP is consistent with findings in studies such as Fama (1990), Cheung and Ng (1998), while inconsistent with Rapach et al. (2005) who points out that the predictive ability of industrial production and the unemployment to assess changing stock prices is limited. Furthermore, a summary of previous studies using macroeconomic variables to explain changing stock prices is provided in Appendix A and enables in-depth comparison of our results, which can also serve for the future research.
The closest paper to ours is El Khoury (2015). El Khoury's paper aims to explain the changing stock prices of nine European automakers from January 2003 to April 2012, by constructing a six-factor model and studying 16 macroeconomic factors, using OLS method. To the best of our knowledge there is no other related paper providing the cross-country evidence on explaining the fluctuation of prices of quoted automakers. Comparing to the results presented in El Khoury's study, stock market development and unemployment are consistently considered as significant explanatory factors. We did not find any evidence that export, exchange rate, platinum and aluminium prices can adequately explain changing stock prices of automakers, as proposed by El Khoury (2015).
5. Conclusion
The asset pricing theories do not specify what economic forces affect the stock prices (Cheung & Ng, 1998). This paper has attempted to address the question of whether the volatility of stock prices of automakers is affected by macroeconomic variables, and if so, to identify these variables. The five-factor model was developed in this study for 39 stocks of automakers in 11 countries, listed endlessly from January 2000 to December 2017, testing 19 macroeconomic variables identified by literature, and provides evidence that the proposed model can shed more light on explaining the automakers stock price volatility in comparison with previous studies. This research finds that the stock volatility can be explained by stock market development, GDP, unemployment, money supply and inflation measured by the industrial price index. All five explanatory variables were selected using genetic algorithm and considering the AIC criterion. The positive linkage between automaker's stock return volatility and explanatory variables such as stock market development, GDP and unemployment was found. Conversely, an inverse linkage between the dependent variable and money supply and IPI was identified. The findings drawn from this study are beneficial in several ways. First, the explanatory macroeconomic factors from the employed model can potentially be used as predictors and can serve investors for effective portfolio diversification. Second, the explanatory macroeconomic factors can be used as decision criteria important to the executives of automakers when making market expansion decisions and can be inter alia implemented into a conceptual market expansion decision scorecard. Finally, the explanatory macroeconomic factors used in the proposed model can serve policymakers and also academicians for deeper understanding of the phenomenon of macroeconomic forces affecting the stock volatility.
Note
1. Data was sourced from several databases, particularly the contribution of global auto industry to global GDP from A.T. Kearny; sales and profit margins, and R&D from PwC, the automotive trends from McKinsey&Company, KPMG, Deloitte and PwC.
Acknowledgements
The authors are thankful for the financial support received from the Czech Science Foundation, Grant No. 16-25536S: Methodology of Developing a Predictive Model of Sector and Company Performance in the Macroeconomic Context.
Disclosure statement
No potential conflict of interest was reported by the authors.
Funding
This work was supported by the Grant Agency of the Czech Republic under Grant No. 1625536S: Methodology of Developing a Predictive Model of Sector and Company Performance in the Macroeconomic Context.
Note: Summarize it with your own wording.
Step by Step Solution
There are 3 Steps involved in it
Step: 1
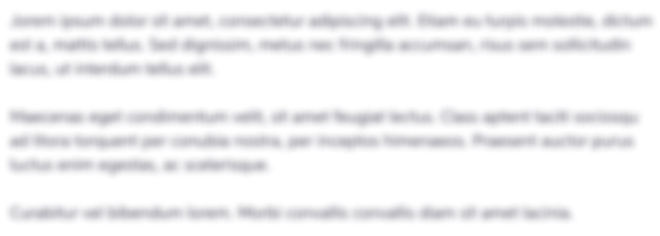
Get Instant Access to Expert-Tailored Solutions
See step-by-step solutions with expert insights and AI powered tools for academic success
Step: 2

Step: 3

Ace Your Homework with AI
Get the answers you need in no time with our AI-driven, step-by-step assistance
Get Started